The Impact of COVID-19 on Bank Sector Traditional Business Model Sustainability in China: Bank Branch Versus Fintech
- School of Accounting, Southwestern University of Finance and Economics, Chengdu, China
This paper aims to analyze the impact of COVID-19 on the sustainability of the banking sector and the fintech sector. In China, where banks’ revenue mainly comes from branches, we collect relevant data manually and use the OLS model for empirical analysis. The results show that as the COVID-19 infection rate increases, the number of bank branches decreases significantly, which threatens the banking sector’s sustainability. The fintech sector acts as a competitor to the banking sector. With the increase of COVID-19 infection rate, the public pays more attention to fintech, promoting the development of the fintech sector. Moreover, the impact of COVID-19 on these two sectors will diminish over time. In addition, this paper finds that COVID-19 further weakens the number of bank branches during the epidemic through the mediating effect of fintech. The findings of this paper help to assess the sustainability of the different financial sectors during the epidemic, which is essential for financial stability.
Introduction
The COVID-19 outbreak at the end of 2019 poses a severe threat to human safety and survival [1, 2]. From data published by the World Health Organization, COVID-19 has spread to 190 of 233 countries worldwide by the end of 2020. The cumulative number of confirmed cases reached 80 million and caused more than 1.8 million deaths. Not only that, but the COVID-19 epidemic has also had a significant impact on the global economy. In terms of the banking sector, with the spread of COVID-19, the scale of social financing has been significantly reduced [1], exacerbating the vulnerability of financial institutions, increasing the operational risk of banks [2] and reducing their profitability [3].
Specifically, in China, the banking sector derives its profits mainly from deposits and loans of branches, which is the traditional business development model of banks. The number of bank branches reached a staggering 400,000 at the end of 2020. However, during the epidemic, the daily activities of the population were restricted, the business activities of many enterprises were at a standstill. The banks’ consideration to prevent the epidemic and improve their business performance is likely to slow down their establishment of new branches, which will change their traditional business model.
COVID-19 has negatively impacted many industries but has facilitated the development of the fintech sector.6 Fintech has increased the convenience of the public lifestyle [4, 5] and improved the productivity of businesses [6, 7]. At the same time, consumers will face more serious information security issues. This was more evident during the epidemic. To address information security issues, some fintech sectors have started to use quantum information technology to ensure information security. Quantum key distribution enables secure key sharing between senders and receivers through the preparation, transmission, and measurement of quantum states of single photons, which cannot be eavesdropped, and combines with traditional encryption techniques to complete classical information encryption and secure transmission [8, 9]. As security is enhanced, the public becomes more dependent on fintech, and the sustainability of the banking sector is severely impacted [10]. In the case of Internet payment, for example, by the end of 2020, non-fintech payment methods will be more secure. For example, at the end of 2020, the non-banking sector will have 93% of the market share, which will reduce the frequency of use of bank credit cards by the public and weaken the deposit and loan business of bank branches [11].
COVID-19 negatively affected many industries but facilitated the development of the fintech sector [12]. Fintech increased the convenience of public lifestyles [4, 5] and improved the productivity of businesses [6, 7], which was more evident during the epidemic. Currently, the fintech sector, as the main competitor of banks, has a severe impact on the sustainability of the banking sector [10]. As an example, at the end of 2020, the non-bank sector held 93% of the market share of Internet payment methods, reducing the frequency of public use of bank credit cards and weakening the deposit and loan business of bank branches [11].
Currently, scholars have concentrated on analyzing the short-term impact of COVID-19 on the banking sector and have focused their research on banks’ lending business, business performance, and business risks [3, 13, 14]. In addition, we should also focus on the impact that COVID-19 will have on the branches of the banking sector since this impact will change the business model of banks and have a long-term impact on their profitability. To answer this question: First, this paper will analyze whether COVID-19 will impact the branches of banks? Second, given the intense competition between the fintech industry and banks, has COVID-19 affected the fintech industry [15]. Finally, does COVID-19 further affect banks’ institutions through fintech during the epidemic?
To address the above questions, this paper examines the dual impact of COVID-19 on the banking sector and the fintech sector using provincial panel data for each day between January 2020 and December 2020. First, we construct proxies: 1) the number of branches to measure the sustainability of the banking sector’s business model, 2) the Internet search index for fintech to measure the sustainability of the fintech sector, and 3) the COVID-19 infection rate to measure the severity of the epidemic. Second, the dual impact of COVID-19 on the business model of the banking sector and the fintech sector is analyzed through a structural model. Finally, the mediating effect is used to test whether COVID-19 affects banks’ business models through fintech.
Compared with the existing literature, the marginal contributions of this paper are mainly in the following aspects: First, most of the literature currently examines the impact of COVID-19 on the non-banking sector. However, as an important financial institution for economic development, banks have received insufficient attention from scholars. The studies that have been conducted also focus only on the short-term effects of COVID-19 on the banking sector. This paper takes the traditional business model of banks as the object of study. It analyzes the long-term impact of COVID-19 on the banking sector, which fills the gaps in the existing literature. The findings of this paper contribute to a more comprehensive understanding of the economic outcomes of COVID-19. Second, most existing literature analyzes the relationship between COVID-19 and the fintech sector in isolation, ignoring the competitive relationship between the banking sector and the fintech sector during the epidemic. In this paper, we illustrate the link between these three through a mediating effects model. Specifically, COVID-19 will have a direct impact on the traditional business model of banks and indirectly affect the traditional business model of banks through the fintech sector. Fourth, FinTech is a complex concept, including technologies such as machine learning, cloud computing, quantum information, etc. These technologies require a close connection between different disciplines, such as computer, physics and biology, etc. However, we need to make further tests whether these technological achievements can be accepted by the public. The findings of this paper fully affirm the role played by these technologies in the financial system and provide a reference for the reform of the banking sector.
The rest of the paper is structured as follows: Part 2 presents the literature review and research hypotheses. Part 3 introduces the research methodology of this paper. Part 4 describes and discusses the empirical results. Part5 concludes.
Literature Review and Research Hypothesis
COVID-19 and the Traditional Business Model of Banks
From the history of banks, the interest difference between obtaining deposits and loans is the primary source of profit for banks [16]. Therefore, the banking sector competed fiercely for customers’ deposits and loans, and the rapid expansion of bank branches was an essential means of bank competition [17–19]. However, bank lending decreases significantly during epidemics, Ҫolak and Öztekin (2021) [13] analyze the impact of COVID-19 on global bank lending using a difference in difference approach and find that bank lending is smaller in countries with severe epidemics. Özlem.D.N and Schandlbauer (2021) take European banks as subjects and develop a similar picture. This effect is more pronounced for fully capitalized banks. The main reason for this result is the significant negative impact of COVID-19 on business production and the daily activities of the public [20, 21]. At the same time, the increased economic uncertainty makes banks more cautious in lending [1, 22]. Thus COVOID-19 affects the number of bank branches from the asset side and changes the traditional business model of banks.
In summary, during the epidemic period, banks will slow down the establishment of bank branches to prevent COVID-19, reduce risks, and improve efficiency. At the same time, the government and the public take various necessary measures to protect against COVID-19, such as: wearing masks and strict disinfection [23, 24]. Therefore, the impact of COVID-19 on bank branches may gradually diminish over time. Based on these analyses, we propose the following hypotheses:
Hypothesis 1a (H1a): There is a negative relationship between COVID-19 and bank branches.
Hypothesis 1b (H1b): The effect of COVID-19 on bank branches gradually decreases over time.
COVID-19 and Fintech Sector
COVID-19 negatively affected the banking sector in China [25] but promoted the development of competitors (fintech sector) [26]. During the epidemic, the public reduced the frequency of going out [27], and increased internet activity to avoid contracting COVID-19 [28–30]. Jiang and Stylos (2021) found that consumer habits changed significantly during the epidemic, with a rapid increase in personal online spending and an essential role for digital technology. There are two sides to everything, and with the rapid development of fintech, frequent information security incidents have raised public concerns. That is, the convenience and security of fintech are two important factors that influence consumer demand [31]. Fintech companies will invest more in big data and quantum information technology to meet consumer-related financial needs and accelerate digital transformation [26, 32]. Therefore, with the COVID-19 outbreak increasing public demand for fintech, the fintech sector will also grow at a faster pace. Likewise, considering that the government and the public have already taken relevant countermeasures [23, 24]. Therefore, the impact of COVID-19 on fintech may also diminish over time.
Hypothesis 2a (H2a): There is a positive relationship between COVID-19 and the fintech sector.
Hypothesis 2b (H2b): The effect of COVID-19 on fintech sector gradually decreases over time.
Mediation Effect of the FinTech Sector
Recently, the fintech sector has become a public concern and has negatively impacted bank branches regarding deposits, loans [33, 34], and payment settlements (e.g., mobile payments) [35, 36]. This negative impact not only reduces bank performance [37] but also changes the traditional business model of banks. Banks have to invest in fintech to improve their performance as the demand for IT by companies and individuals increases [38, 39], which occupies their internal resources and makes them invest less in their branches. This impact is more evident during the COVID-19 outbreak. Specifically, the positive impact of COVID-19 on fintech is likely to stimulate banks to invest more resources in developing their fintech, thus further weakening the investment in bank branches. Thus, fintech will have a mediating effect between COVID-19 and bank branches. Based on these analyses, we propose the following hypotheses:
Hypothesis 3 (H3): COVID-19 affects bank branches indirectly through the mediating effect of the fintech sector.
In response to these theories and hypotheses, we draw the structural framework and present it in Figure 1.
Materials and Methods
Data and Sample
The date of the first confirmed infection with COVID-19 was 20 January 2020, in China, so this paper defined the interval as 20 January 2020-31 December 2020; the frequency of data collection was every day; the sample included 31 provinces in China; the samples with missing data were excluded in this paper, and finally 10,038 observations were obtained.
Measures
Dependent Variables
According to H1, we need to get information about bank branches. In China, the official website of the China Banking and Insurance Regulatory Commission (CBRC) publishes the financial institution license information of bank branches. This license contains information such as the bank’s name, the date of establishing the bank branch, and the geographical location. We collected these data manually, sorted them by province and time, and ordered them into a panel data format. In this paper, we use the number of bank branches added in each Province per day (Brach) as the dependent variable in H1.
According to H2, we need to find an indicator that can measure the level of development of the fintech sector. However, the fintech sector is a vague concept that includes digital payments and wallets, P2P lending platforms, robot-money, intelligent money management, machine learning and cloud computing, financial credit, financial information platforms, Etc. It is not easy to find a comprehensive indicator to measure the level of development of the fintech sector. Even if such a measure exists, the indicator does not match the frequency of collection of COVID-19 data (per day) in a time series. Therefore, this paper uses the public Internet search index for fintech for each Province per day as the dependent variable of H2 to match the bank branch data and COVID-19 data to ensure that the observations of these three key indicators are the same. Finally, we categorized dates by province and time and organized them into a panel data format.
Independent Variables
We need to obtain COVID-19 related information. In China, the health committee of each province publishes COVID-19 related data on the official website every day, such as information on the number of increased infections per day and the cumulative number of infections. We collected these data manually, categorized them by province and time, organized them into panel data format, and calculated the COVID-19 infection rate. This paper used the COVID-19 infection rate (Incrs) per day and the cumulative infection rate (Surv) for each province as independent variables.
Moderating Variables
According to H1b and H2b, the samples need to be divided into two parts in this paper and represented by day30. Counting from the first COVID-19 infected person found in each province, day30 takes the value of 0 if the sample falls within 30 days after the diagnosis; if the sample is more than 30 days after the diagnosis, day30 takes the value of 1.
Control Variables
According to the literature, we control for factors that may impact the number of bank branches and FinTech. Usually, the higher the mortality rate of COVID-19 infected people during an epidemic, the more panicked the population will be, and the frequency of going out will decrease. As a result, the number of bank branches and FinTech is significantly affected. In addition, we control for the financing needs of the society (Loan), marketization index (Mark), the regional industrial structure (Indus), the level of digital trading of companies (Digt), and the level of regional economic development (PGDP).
Table 1 summarizes all of the above variables and presents the measures and data sources for these variables.
Model Design
In this paper, we use ordinary least squares (OLS) to test H1-H3. In the testing process, it should be noted that if the error terms of the model are correlated with each other, the standard error of the OLS estimation results will be biased and will not reflect the true variability of the estimated coefficients well. Hence, the standard error needs to be adjusted. Therefore, this paper uses cluster-adjusted standard errors (cluster) at the province level to solve this problem [40]. We also add time dummy variables to the model to eliminate the interference of time trends on the estimation results. ε represents the error term of the model; i and t denote province and time, respectively.
Model 1: This model is designed to test the effect that COVID-19 would have on the number of bank branches (H1a). The independent variable is incrs (surv), and the dependent variable is Brach.
Model 2: This model is designed to test whether the impact of COVID-19 on bank branches will diminish over time (H1b). We add the intersection terms of incrs (surv) and Day30 to model 1.
Model 3: This model is designed to test the impact that COVID-19 would have on the fintech sector (H2a). The independent variables are incrs and surv, and the dependent variables are Fintech.
Model 4: This model is designed to test whether the effect of COVID-19 on FinTech diminishes over time (H2b). We add to model 3 by increasing the intersection t terms of incrs and Day30, surv and Day30, respectively.
Model 5: This model is designed to test the mediating effect that fintech can have between COVID-19 and bank branches (H3). In model 5, the independent variables are incrs (surv) and Fintech, and the dependent variable is Brach. The mediating effect requires three conditions to be satisfied [40, 41].
1) In model 1, there is a significant association between Brach and incrs (surv). Therefore, the estimated coefficient α1 must pass the significance test.
2) In model 3, there is a significant association between Fintech and incrs (surv). Therefore, the estimated coefficient β1 must pass the significance test.
3) In model 5, there is a significant association between the mediating variable (Fintech) and Brach. Therefore, the estimated coefficient γ2 must pass the significance test. Moreover, in model 5, if the estimated coefficient γ1 of incrs (surv) passes the significance test, it means that model 5 is a partial mediating effect model. Otherwise, it means that model 5 is a fully mediated effects model.
Descriptive Statistics Analysis
Table 2 presents the results of descriptive statistics for all variables in this paper. The mean value of Brach is 0.256, which indicates an average increase of 0.256 bank branches per province per day. The minimum value of Brach is 0, and the maximum value is 243, with a significant difference between them, indicating a sizeable individual difference between each sample. The mean value of Fintech is 0.32, which indicates that 32 out of 10,000 people 32 will search for Fintech information via the Internet every day. The mean value of Incrs is 0.002, which means that 2 out of 10,000 people will be infected with COVID-19 every day. The mean value of Surv is 0.032, which means that 32 out of 10,000 people will be infected with COVID-19 cumulatively.
Correlation Coefficients of Variables
Table 3 shows the correlation coefficients between all variables, the correlation coefficients between Incrs (Surv) and brach are −0.023 and −0.025, the negative relationship between them is consistent with the expectation of H1a. the correlation coefficients between Incrs (Surv) and fintech are 0.194 and 0.327, the positive relationship between them is consistent with the expectation of H2a. However, the statistical correlation does not mean that the variables are economically significant with each other. Multiple regression analysis is still needed to verify this paper H1-H3.
Regression Analysis
Considering that the problem of multicollinearity among variables can lead to bias in the estimation results, we conducted variance factor analysis (VIF) for model 1–model 5. We found that the values of VIF are all much less than 10, indicating that there is no multicollinearity problem among variables in the model.
Effect of COVID-19 on Bank Branches
Table 4 shows the test results for H1a and H1b. Column (1) tests the effect of the control variables in this paper on bank branches, and the estimated coefficient values for death and mark are negative and significant. This indicates that the higher the mortality rate of COVID-19 and the more marketized, the lower the number of bank branches. The coefficient values of loan and pgdp are positive and significant, which indicates that the higher the demand for social financing and the higher the regional economic level, the higher the number of bank branches.
The estimated coefficient value of incrs in column (2) is −0.270. It is significant at the 5% level, implying that a 1% increase in COVID-19 infection rate is associated with a 0.27% decrease in bank branches. The estimated coefficient value of surv in column (3) is −0.043 and is significant at the 1% level. These results indicates that as the COVID-19 epidemic worsens, banks will slow down the establishment of branches. Thus, H1 is verified. It can be seen that COVID-19 can have a significant impact on the traditional business model of banks.
The coefficients of the intersection terms incrs × day30 and surv × day30 in columns (4) and (5) are positive and significant at the 5 and 10% levels. Combined with the estimation results in columns (2) and (3), it indicates that bank branches will be less negatively affected than in the first month of the beginning of the outbreak. This implies that the impact of COVID-19 on the banks gradually weakened over time. Therefore, H2a is verified.
Effect of COVID-19 on Fintech
Table 5 shows the test results for H2a and H2b. Column (1) tests the effect of the control variables of this paper on fintech. The coefficient value of loan is negative and significant at the 1% level, which indicates a negative relationship between social financing demand and fintech. The coefficients of mark and pgdp are positive and significant at the 5 and 10% levels, which means that the more marketized and the higher the level of economic development, the higher the level of fintech development.
The estimated coefficient value of column (2) incrs is 0.010. It is significant at the 1% level, which means that a 1% increase in the infection rate of COVID-19 increases the fintech internet search index by 0.010%. The estimated coefficient value of Surv in column (3) is 0.001 and is significant at the 5% level. Those results of this empirical analysis suggest that as the COVID-19 epidemic worsens, the public becomes more concerned about fintech, which will contribute to the rapid development of the fintech sector. Therefore, H2a is validated.
The coefficients of the intersecting terms incrs × day30 and surv × day30 in columns (4) and (5) are negative and significant at the 1 and 5% levels. Combined with the estimation results in columns (2) and (3), it indicateds that fintech is less positively affected than the first month of the epidemic’s start. This implies that the impact of COVID-19 on FinTech gradually weakened over time. Therefore H2b is verified.
Intermediation Effects in the Fintech Sector
The three conditions for the intermediation effect to hold are described in the model design section. The estimation results in columns (2) and (3) of Table 4 and columns (2) and (3) of Table 5 already confirm that the first two conditions hold, and the third condition (H3) of the mediating effect needs to be tested next. The coefficient values of the mediating variable (fintech) in columns (1) and (2) of Table 6 are −1.757 and −1.749, and both are significant at the 5% level, which satisfies the third condition for the mediating effect. Combined with the previous analysis results, the results of the analysis in Table 6 indicate that the positive effect of COVID-19 on fintech will further reduce the establishment of branches by banks. Therefore, H3 is verified that.
Meanwhile, the estimated coefficients of incrs and surv in columns (1) and (2) are negative and significant at the 5 and 1% levels, which indicates that model 5 is a partial mediating effect model; that means, COVID-19 will have not only a direct impact on bank branches but also an indirect impact through fintech.
Summary of Intermediary Effect Test Results
Through the previous analysis, we know that COVID-19 will have a direct effect on bank branches and indirectly affect bank branches through the fintech sector. In order to better understand the mediating effect, Table 7 summarizes the results of all the above analyses.
Endogeneity Problem and Robustness Test
Endogeneity Problem
There is a possible endogeneity problem of mutual causality between the independent variables (incr, surv) and the dependent variable (brach) in model 1, which could lead to bias in the first conclusion of this paper (negative association between COVID-19 and bank branches). Therefore, this paper uses a two-stage instrumental variable approach [42, 43] to validate the above results. Two conditions of use need to be satisfied in the choice of instrumental variables: first, the dependent variable does not affect the instrumental variables. Second, there is a link between the instrumental variable and the independent variable.
In this paper, the number of tourists in the region is chosen as the instrumental variable because COVID-19 is highly contagious [44, 45], and bank branches do not significantly affect the number of tourists in the region, satisfying the first condition of the instrumental variable. On the other hand, tourists would accelerate the spread of the virus and increase the number of confirmed COVID-19 diagnoses, satisfying the second condition of the instrumental variable.
Table 8 shows the test results, in the first stage of regression analysis, there is a positive correlation between trav and Iv_incr and Iv_surv with estimated coefficients of 0.026 and 0.514, and significant at the 5 and 1% levels. Meanwhile, the Cragg-Donald Wald F statistic of the weak instrumental variable test in the first stage is 21.301, 65.308 greater than the critical values 11.41 of the Stock-Yogo weak ID test at the 10% level, indicating that the instrumental variables selected in this paper are reasonable. In the second stage regression, the coefficients of Iv_incr and Iv_surv are −7.689 and −0.387, respectively. Both are significantly negative at the 5% level, indicating that H1a still holds after accounting for the endogeneity problem.
Robustness Tests
Effect of Data Truncation
Suppose some observations are systematically excluded from the sample. In that case, this phenomenon is called truncation. In this situation, a linear regression of the entire sample using OLS will have its nonlinear perturbation terms included in the perturbation term, leading to inconsistent estimates. Since there are more cases where the number of branches added by the bank (Brach) takes a value equal to zero, it is a case where the sample is truncated. Therefore, we use the Tobit model to test the previous estimation results again. Table 9 shows the results of the robustness test using the Tobit model, and there is a significant negative relationship between columns (1–4) incrs (surv) and Brach, which is consistent with the results of the previous analysis. It indicates that the empirical analysis in this paper is not affected by data truncation.
Effect of Discrete Variables
The dependent and independent variables in this paper are discrete variables. In the empirical analysis using the OLS model, the discrete distribution of the dependent variable may produce estimation bias on the coefficients in the above results. Therefore, we further use the binary choices model (Logit and Probit model) in the generalized linear model to re-examine the original findings. In the Logit and Probit model, we need to construct the dummy dependent variable (Dum_brach), where the value of Dum_brach is equal to 1 if brach >0 and 0 otherwise. Table 10 shows the specific test results, and it can be seen that the coefficients of incrs in columns (1) and (4) are significantly negative (column (2) except), and the coefficient of tech in columns (2) and (4) is also significantly negative, which remains consistent with the results of the previous analysis. Table 11 illustrates that the discrete distribution of the dependent variable does not affect the main conclusions of this paper.
Regional Heterogeneity Test
In China, Hubei Province was the first region where COVID-19 was found. Regions adjacent to Hubei province have more intensive movement between populations, are more threatened by the epidemic, and there are differences in economic level, financial literacy, and culture between regions [46]. Therefore, the impact of COVID-19 on bank branches and financial technology may also exhibit significant regional heterogeneity. To test the geographical differences, we divided the sample into adjacent provinces and non-adjacent provinces. Adjacent provinces include Anhui, Jiangxi, Hunan, Chongqing, Shaanxi, and Henan provinces. Locat takes the value of 1 if the sample belongs to one of them; otherwise, Locat takes 0.
The coefficients of the intersecting terms incrs × Locat and surv × Locat in columns (1) and (2) of Table 11 are negative and significant at the 1% level. Combined with the estimated results in columns (2) and (3) of Table 4, it indicates that provinces closer to Hubei Province are more negatively affected by the traditional business model of banks. The coefficients of the intersecting terms incrs × Locat and surv × Locat in columns (3) and (4) are negative and significant at the 1% level. Combined with the estimation results in columns (2) and (3) of Table 5, it indicates that the promotion effect of COVID-19 on FinTech is more pronounced in provinces that are further away from Hubei Province. These results suggest significant geographical heterogeneity in the impact of COVID-19 on bank branches and fintech.
To better demonstrate this geographic variation, we show it in Figures 2, 3.
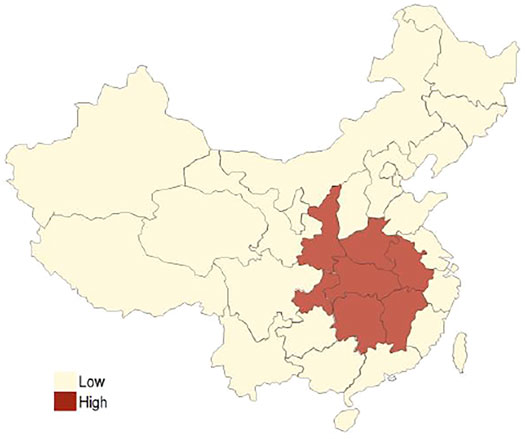
FIGURE 2. The dark color indicates that COVID-19 has a more negative impact on the traditional business model of banks.
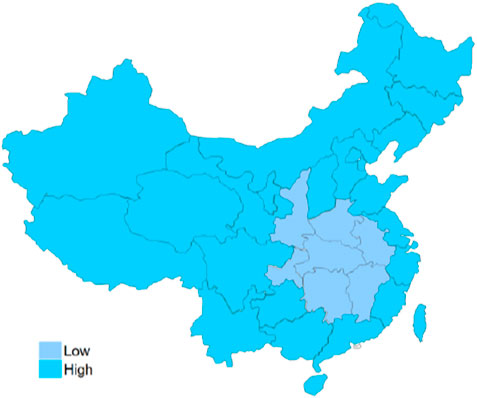
FIGURE 3. The dark color indicates that the positive impact of COVID-19 on fintech is more significant.
Conclusion
Conclusion of This Paper
As technology advances, individuals and businesses become increasingly reliant on fintech, which has had a severe negative impact on traditional bank branches. How will the COVID-19 outbreak at the end of 2019 affect the relationship between these rival fintech and traditional banking sectors? This paper finds that as COVID-19 infection rates increase, fewer bank branches are established. In contrast, the public will pay more attention to the fintech sector. However, these effects diminish in the later stages of the COVID-19 epidemic. At the same time, the fintech sector is also an essential mediating variable for the weakening of bank branches by COVID-19.
Theoretical and Practical Implications
For theoretical implications, the literature mainly focuses on the impact of COVID-19 on the banking sector and the fintech sector as separate studies. In fact, during the COVID-19 epidemic, they were closely linked to each other. Therefore, this paper combines them to confirm the dual impact of COVID-19 on the banking sector and fintech, moreover the existence of a significant mediating effect between them. This has important theoretical implications for a complete understanding of the impact of COVID-19 on the financial system.
The practical significance for developing countries is that banks have an almost monopoly position in the financial system, which leaves them with no incentive to innovate48. However, the unexpected COVID-19 epidemic has had a severe impact on the traditional business model of banks, which has forced them to invest in fintech, and this investment is irreversible under the pressure of external competition. Therefore, banks should develop both fintech and branches and find the best combination of them. This has important practical implications for banks to respond to unexpected public events, improve operational efficiency and enhance long-term competitiveness.
Limitations and Future Research Directions
First, because FinTech is a more complex concept [47], this paper cannot use specific indicators to measure the level of development of the FinTech sector in different regions. Therefore, in the future, more comprehensive indicators need to be constructed to measure the level of fintech in regions. Second, there are differences in the structure of financial systems among different countries, and the findings in this paper may be heterogeneous at the national level. Therefore, the conclusions of this paper need to be tested and enriched by collecting relevant data from other countries in the future. Third, at present, although only a small number of banks have developed their fintech, in the future, banks will be sure to make substantial investments in this area. Then, how banks can achieve optimal investment between branches and fintech is extremely important for banks to improve their operational efficiency. Therefore, in the future, we can continue to conduct in-depth research on this issue.
Data Availability Statement
The raw data supporting the conclusion of this article will be made available by the authors, without undue reservation.
Author Contributions
Conceptualization, PJ and JY; Data Collection, PJ; Formal analysis, JY; Methodology, PJ; Software, PJ; Supervision, JY; Writing—original draft, PJ and JY; Writing—review and editing, PJ and JY. All authors have read and agreed to the published version of the manuscript.
Conflict of Interest
The authors declare that the research was conducted in the absence of any commercial or financial relationships that could be construed as a potential conflict of interest.
Publisher’s Note
All claims expressed in this article are solely those of the authors and do not necessarily represent those of their affiliated organizations, or those of the publisher, the editors and the reviewers. Any product that may be evaluated in this article, or claim that may be made by its manufacturer, is not guaranteed or endorsed by the publisher.
References
1. Li X, Feng H, Zhao S, Carter DA. The Effect of Revenue Diversification on Bank Profitability and Risk during the COVID-19 Pandemic. Finance Res Lett (2021) 43:101957. doi:10.1016/J.FRL.2021.101957
2. Rizwan MS, Ahmad G, Ashraf D. Systemic Risk: The Impact of COVID-19. Finance Res Lett (2020) 36:101682. doi:10.1016/J.FRL.2020.101682
3. Barua B, Barua S. COVID-19 Implications for banks: Evidence from an Emerging Economy. SN Bus Econ (2021) 1(1). doi:10.1007/S43546-020-00013-W
4. Maier E. Supply and Demand on Crowdlending Platforms: Connecting Small and Medium-Sized enterprise Borrowers and Consumer Investors. J Retailing Consumer Serv (2016) 33:143–53. doi:10.1016/J.JRETCONSER.2016.08.004
5. Jagtiani J, John K. Fintech: The Impact on Consumers and Regulatory Responses. J Econ Business (2018) 100:1–6. doi:10.1016/J.JECONBUS.2018.11.002
6. Lv P, Xiong H. Can FinTech Improve Corporate Investment Efficiency? Evidence from China. Res Int Business Finance (2022) 60:101571. doi:10.1016/J.RIBAF.2021.101571
7. Abbasi K, Alam A, DuAnna M, Huynh TLD. FinTech, SME Efficiency and National Culture: Evidence from OECD Countries. Technol Forecast Soc Change (2021) 163:120454. doi:10.1016/J.TECHFORE.2020.120454
8. Xiu X-M, Dong L, Gao Y-J, Chi F. Controlled Deterministic Secure Quantum Communication Using Five-Qubit Entangled States and Two-step Security Test. Opt Commun (2009) 282(2):333–7. doi:10.1016/J.OPTCOM.2008.09.070
9. Elhadad A, Abbas S, Abulkasim H, Hamad S. Improving the Security of Multi-Party Quantum Key Agreement with Five-Qubit Brown States. Comp Commun (2020) 159:155–60. doi:10.1016/J.COMCOM.2020.05.021
10. Yoon C, Lim D. Customers' Intentions to Switch to Internet-Only Banks: Perspective of the Push-Pull-Mooring Model. Sustainability (20212021) 1313(14):80628062. doi:10.3390/SU13148062
11. Jagtiani J, Lemieux C. Do fintech Lenders Penetrate Areas that Are Underserved by Traditional banks. J Econ Business (2018) 100:43–54. doi:10.1016/J.JECONBUS.2018.03.001
12. Daragmeh A, Lentner C, Sági J. FinTech Payments in the Era of COVID-19: Factors Influencing Behavioral Intentions of "Generation X" in Hungary to Use mobile Payment. J Behav Exp Finance (2021) 32:100574. doi:10.1016/J.JBEF.2021.100574
13. Ҫolak G, Öztekin Ö. The Impact of COVID-19 Pandemic on Bank Lending Around the World. J Banking Finance (2021) 133:106207. doi:10.1016/J.JBANKFIN.2021.106207
14. Özlem Dursun-de Neef H, Schandlbauer A. COVID-19 and Lending Responses of European banks. J Banking Finance (2021) 133:106236. doi:10.1016/J.JBANKFIN.2021.106236
15. Joia LA, Cordeiro JPV. Unlocking the Potential of Fintechs for Financial Inclusion: A Delphi-Based Approach. Sustainability (2021) 1313(21):1167511675. doi:10.3390/SU132111675
16. Rogers K, Sinkey JF. An Analysis of Nontraditional Activities at U.S. Commercial banks. Rev Financial Econ (1999) 8(1):25–39. doi:10.1016/S1058-3300(99)00005-1
17. Salas V, Saurina J. Deregulation, Market Power and Risk Behaviour in Spanish banks. Eur Econ Rev (2003) 47(6):1061–75. doi:10.1016/S0014-2921(02)00230-1
18. Demyanyk Y. U.S. Banking Deregulation and Self-Employment: A Differential Impact on Those in Need. J Econ Business (2008) 60(1-2):165–78. doi:10.1016/J.JECONBUS.2007.09.001
19. Chava S, Oettl A, Subramanian A, Subramanian KV. Banking Deregulation and Innovation. J Financial Econ (2013) 109(3):759–74. doi:10.1016/J.JFINECO.2013.03.015
20. Adolph C, Amano K, Bang-Jensen B, Fullman N, Wilkerson J. Pandemic Politics: Timing State-Level Social Distancing Responses to COVID-19. J Health Polit Pol L (2021) 46(2):211–33. doi:10.1215/03616878-8802162
21. Cruz-Cárdenas J, Zabelina E, Guadalupe-Lanas J, Palacio-Fierro A, Ramos-Galarza C. COVID-19, Consumer Behavior, Technology, and Society: A Literature Review and Bibliometric Analysis. Technol Forecast Soc Change (2021) 173:121179. doi:10.1016/J.TECHFORE.2021.121179
22. Beck T, Da-Rocha-Lopes S, Silva AF. Sharing the Pain? Credit Supply and Real Effects of Bank Bail-Ins. Rev Financ Stud (2021) 34(4):1747–88. doi:10.1093/RFS/HHAA067
23. Rahman MS, Peeri NC, Shrestha N, Zaki R, Haque U, Hamid SHA. Defending against the Novel Coronavirus (COVID-19) Outbreak: How Can the Internet of Things (IoT) Help to Save the World. Health Pol Tech (2020) 9(2):136–8. doi:10.1016/J.HLPT.2020.04.005
24. Das S, Sarkar S, Das A, Das S, Chakraborty P, Sarkar J. A Comprehensive Review of Various Categories of Face Masks Resistant to Covid-19. Clin Epidemiol Glob Health (2021) 12:100835. doi:10.1016/J.CEGH.2021.100835
25. Li S, Yan Y. DATA-driven Shock Impact of COVID-19 on the Market Financial System. Inf Process Manage (2022) 59(1):102768. doi:10.1016/J.IPM.2021.102768
26. Amankwah-Amoah J, Khan Z, Wood G, Knight G. COVID-19 and Digitalization: The Great Acceleration. J Business Res (2021) 136:602–11. doi:10.1016/J.JBUSRES.2021.08.011
27. Lu D, Xiao W, Xu G, Ha L, Yang D. Spatiotemporal Patterns and Influencing Factors of Human Migration Networks in China during COVID-19. Geogr Sustainability (2021) 2(4):264–74. doi:10.1016/J.GEOSUS.2021.10.001
28. Chen Q, Min C, Zhang W, Wang G, Ma X, Evans R. Unpacking the Black Box: How to Promote Citizen Engagement through Government Social media during the COVID-19 Crisis. Comput Hum Behav (2020) 110:106380. doi:10.1016/J.CHB.2020.106380
29. Mohammed A, Ferraris A. Factors Influencing User Participation in Social media: Evidence from Twitter Usage during COVID-19 Pandemic in Saudi Arabia. Tech Soc (2021) 66:101651. doi:10.1016/J.TECHSOC.2021.101651
30. Kaya T. The Changes in the Effects of Social media Use of Cypriots Due to COVID-19 Pandemic. Tech Soc (2020) 63:101380. doi:10.1016/J.TECHSOC.2020.101380
31. Merello P, Barberá A, la Poza ED. Is the Sustainability Profile of FinTech Companies a Key Driver of Their Value. Technol Forecast Soc Change (2022) 174:121290. doi:10.1016/J.TECHFORE.2021.121290
32. Papadopoulos T, Baltas KN, Balta ME. The Use of Digital Technologies by Small and Medium Enterprises during COVID-19: Implications for Theory and Practice. Int J Inf Manage (2020) 55:102192. doi:10.1016/J.IJINFOMGT.2020.102192
33. Hodula M. Does Fintech Credit Substitute for Traditional Credit? Evidence from 78 Countries. Finance Res Lett (2021) 102469. doi:10.1016/J.FRL.2021.102469
34. Chueca Vergara C, Ferruz Agudo L, Rambaud C, López Pascual J, Morley B. Fintech and Sustainability: Do They Affect Each Other. Sustainability (2021) 1313(13):70127012. doi:10.3390/SU13137012
35. Yao M, Di H, Zheng X, Xu X. Impact of Payment Technology Innovations on the Traditional Financial Industry: A Focus on China. Technol Forecast Soc Change (2018) 135:199–207. doi:10.1016/J.TECHFORE.2017.12.023
36. A. Basha S, Elgammal MM, Abuzayed BM. Online Peer-To-Peer Lending: A Review of the Literature. Electron Commerce Res Appl (2021) 48:101069. doi:10.1016/J.ELERAP.2021.101069
37. Phan DHB, Narayan PK, Rahman RE, Hutabarat AR. Do financial Technology Firms Influence Bank Performance. Pacific-Basin Finance J (2020) 62:101210. doi:10.1016/J.PACFIN.2019.101210
38. Uddin MH, Mollah S, Ali MH. Does Cyber Tech Spending Matter for Bank Stability. Int Rev Financial Anal (2020) 72:101587. doi:10.1016/J.IRFA.2020.101587
39. Lee C-C, Li X, Yu C-H, Zhao J. Does Fintech Innovation Improve Bank Efficiency? Evidence from China's Banking Industry. Int Rev Econ Finance (2021) 74:468–83. doi:10.1016/J.IREF.2021.03.009
40. Judd CM, Kenny DA. Process Analysis. Eval Rev (2016) 5(5):602–19. doi:10.1177/0193841x8100500502
41. Baron RM, Kenny DA. The Moderator-Mediator Variable Distinction in Social Psychological Research: Conceptual, Strategic, and Statistical Considerations. J Personal Soc Psychol (1986) 51(6):1173–82. doi:10.1037/0022-3514.51.6.1173
42. Andrews DWK. Heteroskedasticity and Autocorrelation Consistent Covariance Matrix Estimation. Econometrica (1991) 59(3):817. doi:10.2307/2938229
43. Newey WK, West KD. Automatic Lag Selection in Covariance Matrix Estimation. Rev Econ Stud (1994) 61(4):631–53. doi:10.2307/2297912
44. He P, Gao Y, Guo L. Evaluating the Disaster Risk of the COVID-19 Pandemic Using an Ecological Niche Model. Sustain (2021) 1313(21):1166711667. doi:10.3390/SU132111667
45. Anand U, Cabreros C, Mal J. Novel Coronavirus Disease 2019 (COVID-19) Pandemic: From Transmission to Control with an Interdisciplinary Vision. Environ Res (2021) 197:111126. doi:10.1016/J.ENVRES.2021.111126
46. Zou J, Deng X. Financial Literacy, Housing Value and Household Financial Market Participation: Evidence from Urban China. China Econ Rev (2019) 55:52–66. doi:10.1016/J.CHIECO.2019.03.008
Keywords: COVID-19, bank sector, branch, finTech, mediating effect
Citation: Yan J and Jia P (2022) The Impact of COVID-19 on Bank Sector Traditional Business Model Sustainability in China: Bank Branch Versus Fintech. Front. Phys. 10:820646. doi: 10.3389/fphy.2022.820646
Received: 23 November 2021; Accepted: 24 January 2022;
Published: 21 February 2022.
Edited by:
Ayse Humeyra Bilge, Kadir Has University, TurkeyReviewed by:
Cristiane Batistela, University of São Paulo, BrazilBaogui Xin, Shandong University of Science and Technology, China
Omer Gebizlioglu, Kadir Has University, Turkey
Copyright © 2022 Yan and Jia. This is an open-access article distributed under the terms of the Creative Commons Attribution License (CC BY). The use, distribution or reproduction in other forums is permitted, provided the original author(s) and the copyright owner(s) are credited and that the original publication in this journal is cited, in accordance with accepted academic practice. No use, distribution or reproduction is permitted which does not comply with these terms.
*Correspondence: Jingshi Yan, 511540072@qq.com