- 1Univ. Lille, Inserm, CHU-Lille, Lille Neuroscience & Cognition, Lille, France
- 2Département de Pharmacologie de la Faculté de Pharmacie, Univ. Lille, Lille, France
- 3Département de Pharmacologie Médicale, I-SITE ULNE, LiCEND, Lille, France
Neuroinflammation, as defined by the presence of classically activated microglia, is thought to play a key role in numerous neurodegenerative disorders such as Alzheimer’s disease. While modulating neuroinflammation could prove beneficial against neurodegeneration, identifying its most relevant biological processes and pharmacological targets remains highly challenging. In the present study, we combined text-mining, functional enrichment and protein-level functional interaction analyses to 1) identify the proteins significantly associated to neuroinflammation in Alzheimer’s disease over the scientific literature, 2) distinguish the key proteins most likely to control the neuroinflammatory processes significantly associated to Alzheimer's disease, 3) identify their regulatory microRNAs among those dysregulated in Alzheimer's disease and 4) assess their pharmacological targetability. 94 proteins were found to be significantly associated to neuroinflammation in Alzheimer’s disease over the scientific literature and IL4, IL10 and IL13 signaling as well as TLR-mediated MyD88- and TRAF6-dependent responses were their most significantly enriched biological processes. IL10, TLR4, IL6, AKT1, CRP, IL4, CXCL8, TNF-alpha, ITGAM, CCL2 and NOS3 were identified as the most potent regulators of the functional interaction network formed by these immune processes. These key proteins were indexed to be regulated by 63 microRNAs dysregulated in Alzheimer's disease, 13 long non-coding RNAs and targetable by 55 small molecules and 8 protein-based therapeutics. In conclusion, our study identifies eleven key proteins with the highest ability to control neuroinflammatory processes significantly associated to Alzheimer’s disease, as well as pharmacological compounds with single or pleiotropic actions acting on them. As such, it may facilitate the prioritization of diagnostic and target-engagement biomarkers as well as the development of effective therapeutic strategies against neuroinflammation in Alzheimer’s disease.
Introduction
Despite extensive research there is still no cure for Alzheimer’s disease, the commonest cause of dementia. Alzheimer’s disease is characterized by neuronal loss in specific brain regions associated with the formation of amyloid-beta senile plaques and tau-immunoreactive neurofibrillary tangles (Polanco et al., 2018). Another hallmark of the disease is the presence of neuroinflammation as defined by the presence of classically activated microglial cells (Fan et al., 2017; Dani et al., 2018). Microglial cells are the resident immune cells of the central nervous system (Ransohoff and Cardona, 2010). While their activation is needed to defend the brain from injury, it has become clear that a sustained and uncontrolled inflammation could contribute to neurodegeneration, for instance leading to vascular dysfunction, mitochondrial dysfunction and oxidative stress (Sochocka et al., 2013; Orsucci et al., 2013).
Several lines of evidence support that better understanding the neuroinflammatory process in Alzheimer’s disease could lead to the development of new diagnostic and therapeutic tools. Positron emission tomography showed that microglial activation is observed from the earliest stages of Alzheimer's disease and correlates both with amyloid deposition and tau aggregation (Dani et al., 2018). Moreover, exciting data demonstrated that the immune challenges could trigger and exacerbate tau and amyloid pathologies (Bhaskar et al., 2010; Krstic et al., 2012), possibly contributing to a pathological vicious circle that could favor regulated neuronal death. Genetic studies including genome-wide studies have also associated inflammation-related genes to the etiology of Alzheimer’s disease (i.e. ABCA7, CLU, CR1, HLA-DRB1, HLA-DRB5, PICALM, and TREM2) (Lambert et al., 2009; Jun et al., 2010; Guerreiro et al., 2013; Lambert et al., 2013; Jansen et al., 2019), further supporting the concept of neuroinflammation as a pathogenic factor in Alzheimer’s disease. To date, a major challenge is to identify the key processes and proteins linked to neuroinflammation in Alzheimer’s disease. Available information reveals a complex picture with the implication of central and peripheral cell types and of a plethora of proteins including pro-inflammatory cytokines, anti-inflammatory cytokines, chemoattractant molecules, peripheral immune mediators as well as their receptors and downstream signaling targets (Itagaki et al., 1988; Togo et al., 2002; Monsonego et al., 2003). Mining useful information in these data for the development of diagnostic and therapeutic neuroprotective strategies remains highly challenging.
Integrated bioinformatics can help to explore complex biological pathways, by analyzing large amount of data and by performing analyses over many distinct datasets. In the present study, we used integrated bioinformatics to perform a systematic review of the proteins associated to neuroinflammation in Alzheimer’s disease over the scientific literature and then to identify the most represented biological processes and the key candidate proteins to modulate them. We combined text-mining, Gene Ontology and Reactome functional enrichment analysis, protein‐protein functional interaction analysis, non-coding RNA-target interaction analysis and drug–protein interaction analysis and identified eleven proteins with the highest ability to modulate neuroinflammatory processes in Alzheimer’s disease. As such, our study provides data that could help to further explore the time-dependent role of the immune response in Alzheimer’s disease and to prioritize the development of neuroinflammation-centered diagnostic or therapeutic tools against Alzheimer’s disease.
Materials and Methods
Protein Collection (Text-Mining)
A systematic review of the proteins relating to Alzheimer’s disease neuroinflammation was collected using the pubmed2ensembl resource that has been developed as an extension to the BioMart system for mining the biological literature for genes (http://pubmed2ensembl.ls.manchester.ac.uk/) (Baran et al., 2011). The string ‘neuroinflammation alzheimer’ was used to retrieve the Ensembl Gene ID related both to neuroinflammation and Alzheimer’s disease in the Homo sapiens (human) genome assembly GRCh37 from Genome Reference Consortium. We then used UniProtKB Retrieve/ID mapper (https://www.uniprot.org/) to retrieve the UniProtKB protein identifiers associated to these Ensembl Gene ID (UniProt, 2019). All the protein identifiers were imported into Cytoscape 3.7.2 for subsequent analysis.
Gene Set Enrichment
Gene Ontology (GO) enrichment of the collected proteins was first performed using the ClueGo + CluePedia Cytoscape plug-in, with annotation from GO Cellular Component, GO Molecular Function and GO Biological Process categories (Bindea et al., 2009). A p-value <0.05 was considered as the cutoff criterion. The most enriched annotations were then visualized using the ggplot2 package in R language (www.r-project.org). Functional enrichment analysis of the proteins was subsequently performed and visualized using the Reactome Pathway database (https://reactome.org) (Fabregat et al., 2017; Jassal et al., 2020). Proteins from the 10 most significantly enriched biological processes identified with Reactome were selected for subsequent analysis.
Protein-Protein Functional Interaction
The STRING protein query database (http://string-db.org) was used to construct a protein-protein functional interaction network in Cytoscape (Szklarczyk et al., 2019). The minimum required interaction score was set to 0.7 to retain only high-confidence functional interactions. We then used the CentiScaPe Cytoscape plug-in to calculate the node degree and betweenness centrality of each protein. The nodes that had a degree centrality and a betweenness centrality greater than or equal to the mean were identified as key proteins (e.g. more likely to modulate neuroinflammation) and retained for subsequent microRNA-target and drug-protein interaction analyses.
Integration of Regulatory MicroRNAs and Long Non-Coding RNAs (lncRNAs) in the Key Protein Network
The microRNA-target interactions were identified using the miRTarBase Homo sapiens 8.0 database that curates experimentally validated microRNA-target interactions and includes 502,652 microRNA target interactions (Huang et al., 2020). The lncRNA-target interactions were obtained from the LncRNA2Target 2.0 database, by retaining only interactions inferred from the low-throughput experiments such as immunoprecipitation assays, RNA pull down assays, luciferase reporter assays, RT-qPCR or western blot (Cheng et al., 2019). Regulating microRNAs that are significantly dysregulated in the blood of Alzheimer's disease patients were selected based on the data published by Leidinger and coworkers (Additional file 1 of their electronic Supplementary Material; (Leidinger et al., 2013). The microRNA-target and lncRNA-target interactions and the microRNAs relative abundance in Alzheimer's disease (log2(fold change); (Leidinger et al., 2013) were mapped using the CyTargetLinker v4.1.0 Cytoscape application (Kutmon et al., 2018).
Drug-Gene Interaction Analysis
The Drug–Gene Interaction database 3.0 (DGIdb v3.0; http://www.dgidb.org/) was used to search for existing associations between drugs (e.g. small molecule compounds or immunotherapies) and essential proteins from our dataset (Cotto et al., 2018). The results of the search were visualized using Cytoscape so that information on protein targetability, drug-protein interaction, drug nature and drug FDA-approval could be easily apprehended.
Results
Identification of Proteins Associated to Alzheimer’s Neuroinflammation
The strategy of our study to identify key candidate proteins to modulate neuroinflammation in Alzheimer’s disease is depicted in Figure 1. Text-mining using the pubmed2ensembl resource that links over 2,000,000 articles to nearly 150,000 genes (Baran et al., 2011) allowed us to systematically collect a list of 94 unique proteins related to neuroinflammation in Alzheimer’s disease. The list of the 94 proteins with corresponding Gene symbols, Ensembl Gene and UniProtKB identifiers is provided in Supplementary Table 1.
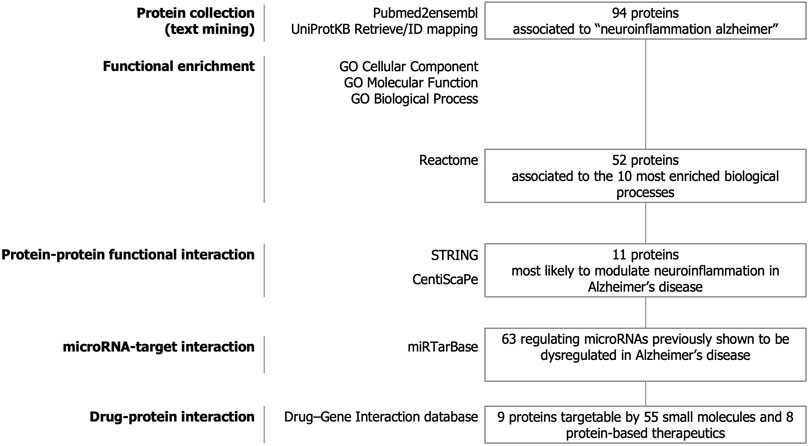
FIGURE 1. Study design. Text-mining was used to support large-scale screening of the scientific literature to collect 94 proteins related to neuroinflammation in Alzheimer’s disease. The entire dataset was analyzed for functional enrichment by using Gene Ontology (GO) cellular localization, molecular functions, biological pathways and by using the Reactome Pathway database and 52 proteins were associated to the 10 most significantly enriched pathways. Protein-protein functional interaction using STRING and CentisScaPe allowed us to identify 11 key proteins most likely to modulate neuroinflammation in Alzheimer’s disease. Finally, non-coding RNA-target interaction and drug-gene interaction analysis showed that the key proteins were indexed to be targetable by a total of 63 microRNAs dysregulated in Alzheimer's disease and by 55 small molecules and 8 protein-based therapeutics.
Gene Ontology (GO) functional enrichment analyses were performed using ClueGo + CluePedia plug-in to explore the cellular localization, molecular functions and biological pathways associated to these proteins. All the collected genes had functional annotations in the selected ontologies. The Cellular Component annotations showed an important enrichment (e.g. over-representation) of the proteins located in the extracellular region, at the cell surface or periphery or at the synapse. The gene ontology enrichment analysis of Biological process annotations showed that the most enriched terms were inflammatory response, positive regulation of cytokine production and response to lipopolysaccharide. Finally, Molecular function annotations revealed an enrichment for proteins with amyloid-beta or peptide binding activities or cytokine receptor binding activities. Other enriched terms were related to arachidonic acid and fatty acid binding, to NADPH oxidoreductase activity or to ionotropic glutamate receptor activity. The top eight enriched terms of the Cellular Component, Biological Process and Molecular Function are shown in Figure 2. The proteins of our dataset that are annotated for the top eight enriched Molecular Function annotations are listed in Figure 3.
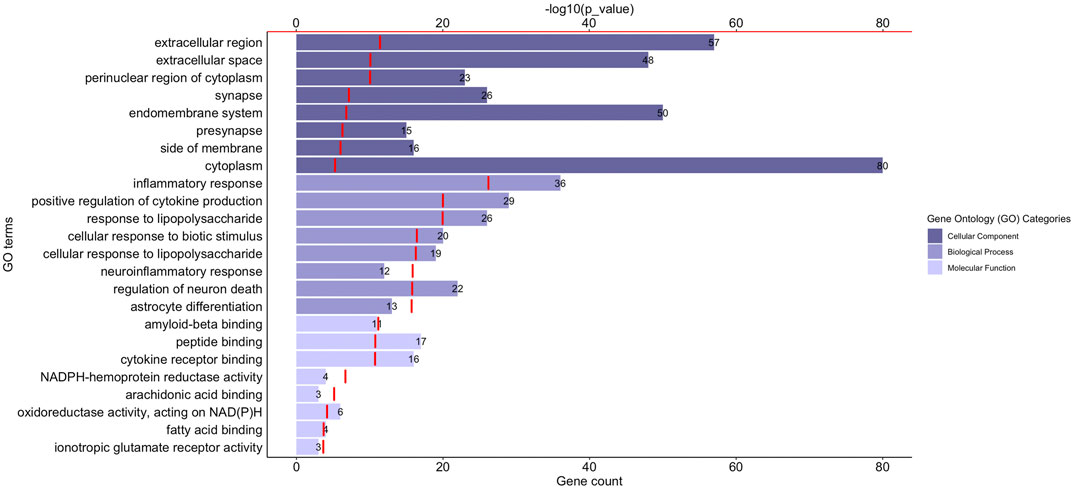
FIGURE 2. Functional enrichment analysis of the proteins associated to neuroinflammation in Alzheimer’s disease. The graph shows the top eight over-represented Gene Ontology (GO) cellular localization, GO molecular functions and GO biological pathways annotations (Y-axis), the number of proteins of our dataset included in each term (length of the histogram bars and number displayed next to each histogram bar, lower X-axis) and the −log10(p_value) levels (red segments, upper X-axis). p-value ≤ 0.05.
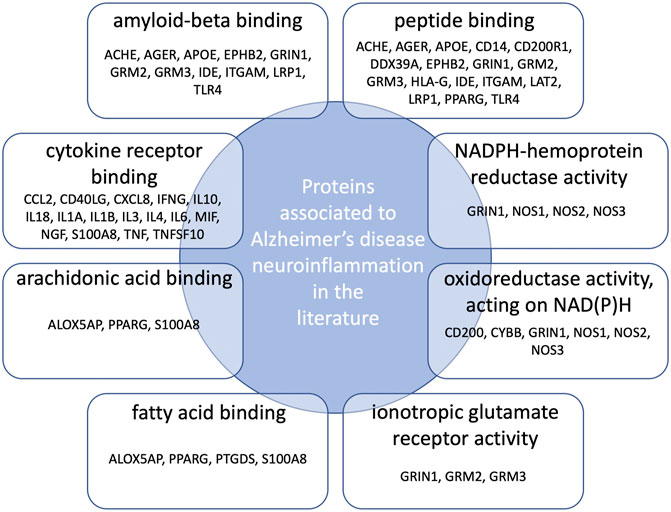
FIGURE 3. Connections between proteins associated to neuroinflammation in Alzheimer’s disease and the top eight over-represented molecular functions annotations. Protein names are those belonging both to our dataset and the enriched GO molecular functions annotations.
Together, our results show that the proteins associated to Alzheimer's disease neuroinflammation are primarily connected to amyloid beta as well as oxidative stress and lipid metabolism that are critically involved in the neuropathogenesis of Alzheimer's disease.
Selection of the Most Relevant Biological Processes
To identify the most relevant biological processes involved in neuroinflammation in Alzheimer’s disease, we performed a functional enrichment of the collected proteins by using the Reactome Pathway database. The 10 most relevant pathways sorted by p-value were Interleukin-4 and Interleukin-13 signaling (p = 1.11 × 10−16), Signaling by Interleukins (p = 1.11 × 10−16), Interleukin-10 signaling (p = 5.77 × 10−15), Immune System (p = 9.30 × 10−14), Cytokine Signaling in Immune system (p = 5.70 × 10−13), Innate Immune System (p = 6.60 × 10−8), Toll-like Receptor Cascades (p = 8.11 × 10−7), TRAF6 mediated induction of NFkB and MAP kinases upon TLR7/8 or 9 activation (p = 1.00 × 10−6), MyD88 dependent cascade initiated on endosome (p = 1.085 × 10−6) and Toll Like Receptor 7/8 (TLR7/8) Cascade (p = 1.085 × 10−6) (Table 1). 52 proteins from the 10 aforementioned most enriched pathways were retained for protein-protein functional interaction analysis.
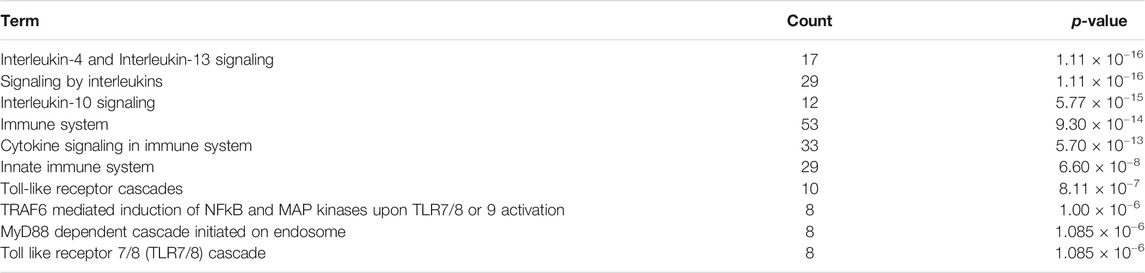
TABLE 1. Function enrichment results of proteins associated to neuroinflammation in Alzheimer’s disease using the Reactome Pathway database. Count: enriched gene number in the category.
Identification of High-Confidence Protein Functional Interactions
Figure 4 represents the protein-protein functional interaction network of the aforesaid proteins. In this representation, nodes represent proteins and edges represent functional interactions. There were 47 interconnected nodes (e.g. proteins) and 244 relationship pairs (e.g. functional interactions). Degree average value was 9.38 and betweenness average value was 47.69. We selected nodes with both higher than average degree (e.g. the measure of the total number of edges connected to the protein) and betweenness (e.g. an indicator of how important the protein is to the shortest paths through the network) as key proteins most likely to have the ability to modulate the aforementioned biological processes and thus neuroinflammation in Alzheimer’s disease. The 11 proteins that met this selection criterion sorted by high to low betweenness value were: interleukin (IL)10, Toll-like receptor (TLR)4, IL6, AKT1, C-reactive protein (CRP), IL4, C-X-C motif chemokine ligand (CXCL)8, Tumor necrosis factor (TNF) alpha, integrin alpha M (ITGAM), C-C motif chemokine ligand (CCL)2 and nitric oxide synthase (NOS)3 (Table 2). These proteins should be considered as the most potent modulators of neuroinflammation in Alzheimer’s disease and were next analyzed for microRNA- and drug-protein interaction.
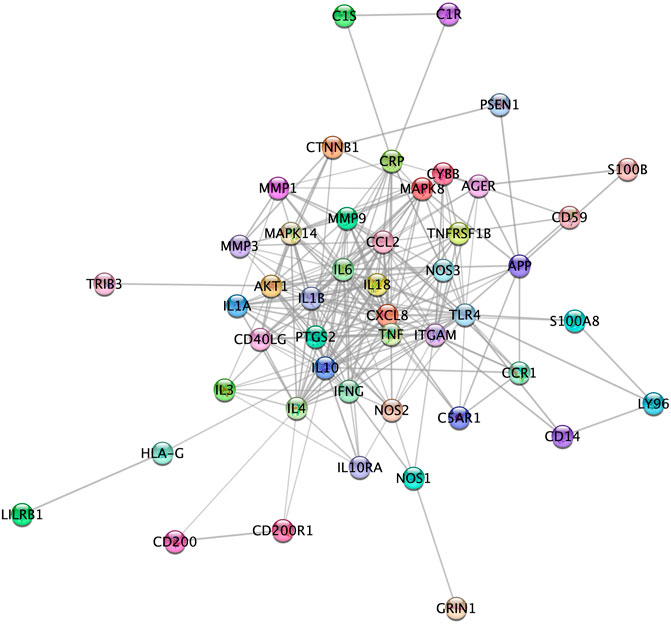
FIGURE 4. High confidence protein-protein functional interaction network of proteins associated to the most relevant biological processes in neuroinflammation in Alzheimer’s disease. Among the 52 proteins associated to the biological processes, 5 (CD4, CHGA, GIG25, LAT2 and MIF) did not show high confidence protein-protein functional interaction in the network and therefore are not represented here.
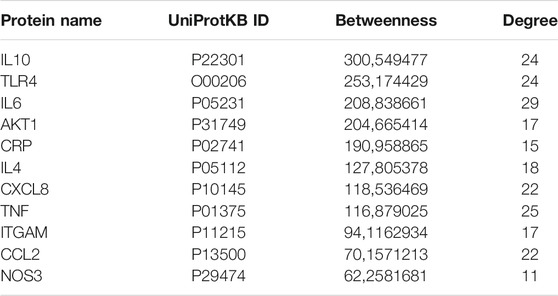
TABLE 2. Proteins with highest than average betweenness and degree in the protein-protein functional interaction network.
Integration of Regulatory Non-Coding RNAs Into the Key Protein Network
Non-coding RNAs including microRNAs and lncRNAs play crucial roles in regulating gene expression and have emerged as key regulators of immune cell functions in innate and adaptive immunity. We first aimed to identify microRNAs both 1) regulating the key protein network as indexed in the experimentally validated gene–microRNA interaction database miRTarBase and 2) dysregulated in Alzheimer's disease blood samples, as reported by Leidiger and coworkers (Leidinger et al., 2013). This led to the identification of 63 dysregulated mature microRNAs including 22 with higher and 41 with lower expression levels in Alzheimer's disease (Figure 5). The corresponding list of mature microRNAs with protein targets is provided in Supplementary Table 2. We also integrated 13 lncRNAs known to regulate the key protein as indexed in the LncRNA2Target database (Figure 5). The corresponding list of lncRNAs with protein targets and bibliographic references is provided in Supplementary Table 3.
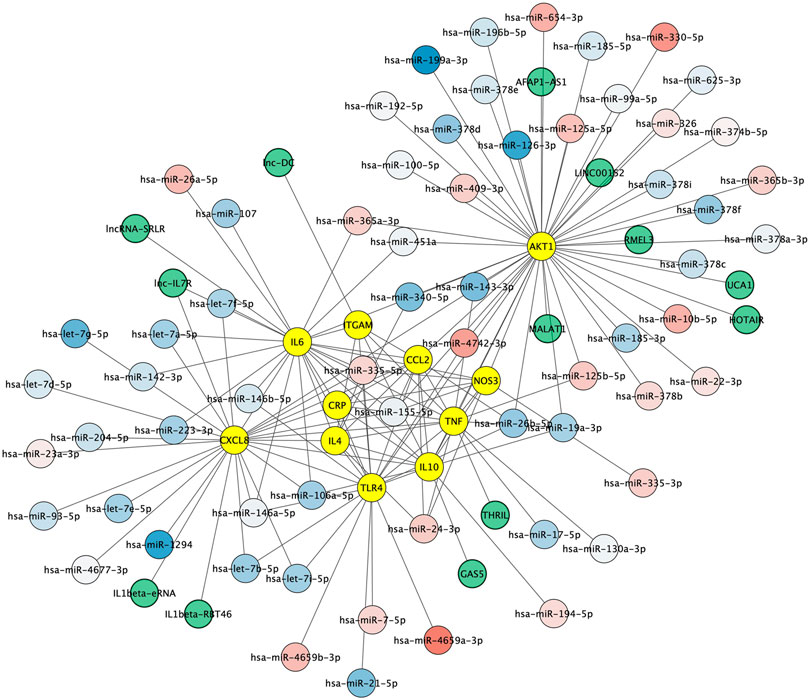
FIGURE 5. Non-coding RNA-protein interaction network. Experimentally validated interactions between microRNAs dysregulated in Alzheimer (blue to red round shapes), lncRNAs (green round shapes) and key proteins in Alzheimer’s disease neuroinflammation (yellow round shapes). Blue to red gradient denotes low to high relative abundance of microRNAs in Alzheimer's disease patient samples according to (Leidinger et al., 2013).
Drug–Protein Network Construction and Identification of Targetable Proteins
To assess to what extent the 11 proteins could be pharmacologically targeted, drug-proteins relationships were predicted using the Drug–Gene Interaction database 3.0. Among the 11 proteins, 9 were indexed to be targeted by a total of 63 drugs. The NOS3 protein had the most interactions with drugs (n = 29), followed by TNF alpha (n = 19), AKT1 (n = 5) and TLR4 (n = 5) and IL6 (n = 4). There was a total of 67 drug–protein relationships in the network. Drugs comprised 8 protein-based therapies of which 6 had a FDA-approval as well as 55 small compounds of which 10 had a FDA-approval. Drug-protein interactions can be visualized in Figure 6.
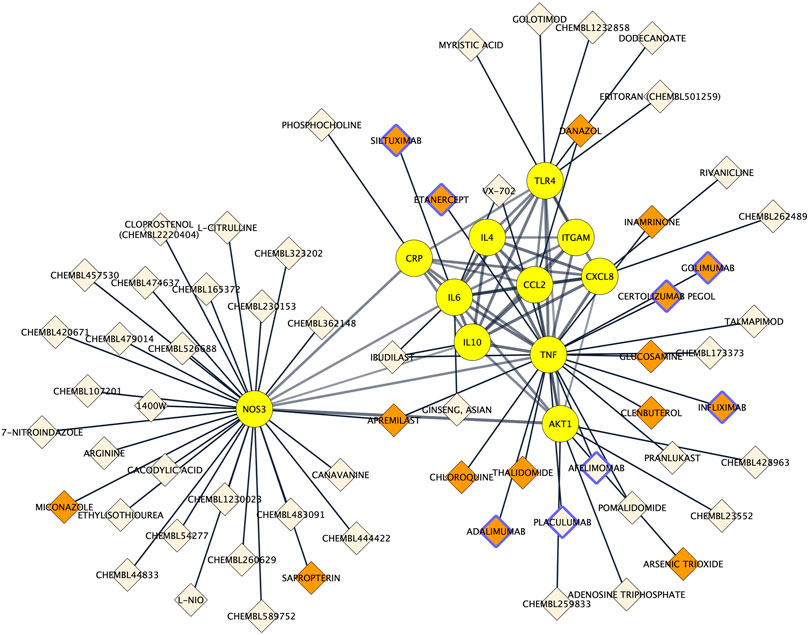
FIGURE 6. Protein-drug interaction network. Existing associations between drugs (e.g. small molecule compounds or immunotherapies; diamond shapes) and key proteins in Alzheimer’s disease neuroinflammation (yellow round shapes). FDA‐approved drugs and non‐FDA‐approved drugs are respectively colored in orange and light beige. Protein-based therapies are bordered with purple.
Discussion
This study used text-mining and data-based bioinformatics to explore the protein network underlying neuroinflammatory processes in Alzheimer’s disease. Text-mining has been recommended for large-scale screening (O'Mara-Eves et al., 2015) and can be used to identify key pathways and candidate genes for drug-discovery and Human health (Singhal et al., 2016; Lever et al., 2019; Pan et al., 2019). In our study, it allowed to collect 94 proteins significantly associated to neuroinflammation in Alzheimer’s disease over the scientific literature (Supplementary Table 1). It is important to note that text-mining has biases due to the imbalance in research attention where some genes are highly investigated while others are ignored (Wang et al., 2015; Oprea et al., 2018). Therefore, the obtained list of proteins is not meant to be exhaustive and may particularly miss proteins still sparsely mentioned in the literature. As such our study may be considered as complementary to other studies such as genome-wide association studies for the further development of diagnostic and target-engagement biomarkers for drug discovery.
Gene Ontology enrichment analysis was used to get first insights into the profile of the 94 collected proteins (Bindea et al., 2009). Cellular localization annotations showed an overrepresentation of cellular compartments essential for communication (e.g. extracellular space, synapse, presynaptic compartment and side of membranes) consistent with the fact that an altered cerebral microenvironment and a dysregulated cell-to-cell crosstalk are critically involved in neuroinflammation (Schwartz and Deczkowska, 2016; Fabiani and Antollini, 2019). Most overrepresented molecular function annotations were amyloid beta binding activity—an activity presented by proteins such as ITGAM (also named CD11b) (Goodwin et al., 1997) and TLR4 (Liu et al., 2020)—as well as cytokine receptor binding activity, further linking the amyloid peptide to the innate immune response in Alzheimer's disease. Molecular function annotations also defined NADPH oxidation, fatty acid and arachidonic acid binding as well as glutamate signaling as significant functions, therefore connecting neuroinflammation to NADPH oxidases and arachidonic acid metabolism critically involved in the neuropathogenesis of Alzheimer's disease (Fricker et al., 2018; Tarafdar and Pula, 2018; Chen et al., 2020). Altogether, the Gene Ontology annotation enrichment helped to capture a coherent picture of neuroinflammation in Alzheimer’s disease and its connections to relevant molecular functions and biological pathways.
Finding the biological processes that are mostly associated to neuroinflammation in Alzheimer’s disease remains highly challenging. We aimed to address this challenge without a priori by analyzing the functional enrichment of our protein dataset using the Reactome Pathway database. Signaling of the anti-inflammatory cytokines IL4, IL10 and IL13 appeared as the most significantly enriched processes. These pathways have been proposed to have protective role in Alzheimer's disease by inducing the clearance of amyloid-beta and the secretion of anti-inflammatory cytokines and neurotrophic factors (Szczepanik et al., 2001; Kawahara et al., 2012; Kiyota et al., 2012; Tang et al., 2019). On the opposite, IL4 and IL13 signaling could exacerbate oxidative stress by activating microglial NADPH oxidases and cyclooxygenase 2 (Park et al., 2009; Jeong et al., 2019). Likewise, TLR signaling as well as the MyD88 and the TNF receptor associated factor (TRAF)6 dependent molecular cascades (that both play an essential role in TLR-elicited intracellular signaling) were identified as important processes. Here again, both beneficial and detrimental roles were attributed to TLRs and for instance TLR7, TLR8 and TLR9 signaling could enhance microglial amyloid beta uptake in the early stage of Alzheimer’s disease, but over time contribute to sustained neuroinflammation (Gambuzza et al., 2014). Taken together, our data highlight that the main processes associated to neuroinflammation in Alzheimer’s disease have both beneficial as well as detrimental roles, and these may be time-dependent. As such, developing strategies to enhance their protective effects or to combat their pathological responses in a disease-stage manner could prove therapeutic potential for Alzheimer’s disease.
Identifying the proteins critically involved in the regulation of the aforementioned processes required to detect key proteins based on the network topology. We found 11 proteins with higher than average degree and betweenness and thus with the highest ability to control the immune processes. These key proteins included the anti-inflammatory cytokines IL4 and IL10 and the pro-inflammatory cytokines TNF-alpha and IL6 that balance appears to be important for maintaining a less pathologic immune profile, as suggested by a recent study of Taipa and coworkers that found that a collection of both pro-inflammatory and anti-inflammatory cytokines were correlated with less cognitive decline in patients after one year (Taipa et al., 2019). Key proteins also included the neutrophil chemoattractant CXCL8 and CCL2 (also named monocyte chemoattractant protein (MCP)1) that exert their effects on target cells via the G protein-coupled receptors CXCL5/CXCL8 receptor (CXCR)1 and 2 and the C-C chemokine receptor (CCR)2, respectively (Zhang et al., 2013; Mamik and Ghorpade, 2016; Chou et al., 2018; Haarmann et al., 2019). Of note, both CXCL8 and CCL2 levels were found to be increased in the cerebrospinal fluid and brain tissue of Alzheimer’s disease patients (Galimberti et al., 2006; Sokolova et al., 2009) and higher CCL2 levels in cerebrospinal fluid were associated with a faster rate of cognitive decline during the early stages of the disease (Westin et al., 2012). Our study also pinpointed at TLR4 and ITGAM (also named CD11b, one protein subunit that forms macrophage antigen complex-1 (Mac-1) or complement receptor 3 (CR3)) that stimulation by amyloid beta leads to microglia activation, resulting in increased cytokine production and oxidative stress (Hong et al., 2016; Yang et al., 2020). Levels of natural CR3 ligands, such as complement fragments, ICAM-1, and fibrin, are also increased in Alzheimer's disease patients (van Oijen et al., 2005; Daborg et al., 2012; Janelidze et al., 2018). Of interest, the activation of CCR2 (Bakos et al., 2017), CXCR1 and CXCR2 (Lane et al., 2006), TLR4 (Fang et al., 2017) and ITGAM (Zhang et al., 2011) all trigger the phosphoinositide 3-kinase (PI3K)/Akt pathway that is perturbated in the brain of patients (Steen et al., 2005; Liu et al., 2011) and that downstream targets include the kinase AKT1, another key protein in our results. Also of interest, the activity of calcium-responsive NOS3, another key node in our study otherwise known as an important regulator of vascular function and of oxidative homeostasis, is also intertwined with that of AKT1 (Dossumbekova et al., 2008; Silva and Garvin, 2009). Finally, our study identified CRP that is increased in brain tissue from Alzheimer’s disease patients (Wood et al., 1993; Iwamoto et al., 1994) and that should be considered not only as a marker but also as a driver of neuroinflammation, since CRP can bind to the complement factor 1q (C1q) and activate the classical complement cascade (Slevin et al., 2015; Braig et al., 2017). The identification of these 11 functionally interconnected proteins provides more specific biomarkers modulating neuroinflammatory processes in Alzheimer's disease. Furthermore, the identification of microRNAs regulating these proteins and known to be deregulated in Alzheimer's disease such as hsa-miR-26a-5p, hsa-miR-107, hsa-miR-26b-5p or hsa-let-7f-5p support their implication in Alzheimer's disease pathogenesis as well as their interest as potential peripheral biomarkers (Wang et al., 2008; Mendes-Silva et al., 2016; Guo et al., 2017; Swarbrick et al., 2019). Likewise, the identification of lncRNAs acting on targets such as CXCL8, TNF-alpha, IL6, IL10 or ITGAM support their role, still largely unexplored, as important regulators of the human innate immune response (Wang et al., 2014a; Cui et al., 2014; Ilott et al., 2014; Li et al., 2014; Li et al., 2017) (Supplementary Table 3).
Drug–protein interactions analysis revealed that 9 of the 11 key proteins could be targeted by a total of 55 small molecules and 8 protein-based therapeutics. The latter comprised FDA approved TNF-alpha antagonists (infliximab, etanercept, adalimumab, golimumab and certolizumab pegol) that were found to be associated with lower Alzheimer’s disease risk in patients with rheumatoid arthritis and psoriasis (Zhou et al., 2020) and proved positive immune and cognitive outcomes in rodent models of Alzheimer’s disease (Kim et al., 2016; Paouri et al., 2017; Park et al., 2019). They also included the IL6-targeting antibody siltuximab that was launched for the treatment of multicentric Castleman but that has not been evaluated to date in the context of Alzheimer’s disease, to our knowledge. Drug-protein interaction analysis also pictured small molecules such as thalidomide that reduces the production of TNF-alpha (Sampaio et al., 1991; Gabbita et al., 2012) and that more potent derivative 3,6-dithiothalidomide ameliorated cognition in a rat models of LPS-induced sustained microglia activation (Belarbi et al., 2012) and in a triple transgenic mouse model of Alzheimer's disease (Tweedie et al., 2012). Likewise, we identified glucosamine that was shown to inhibit in microglial cells lipopolysaccharide-induced TNF-alpha expression, Ca2+ influx and outward K+ currents, which are typically representative of microglial activation (Yi et al., 2005). Interestingly, our analysis also highlighted several phosphodiesterase inhibitors such as apremilast (phosphodiesterase 4 inhibitor) that interferes with NOS3 and cytokines production (Schett et al., 2010; Gulisano et al., 2018), inamrinone (phosphodiesterase 3 inhibitor) or ibudilast (a non-selective 3, 4, 10, 11 phosphodiesterase inhibitor). Of note, ibudilast is currently approved for use as an anti-inflammatory in Japan, improved amyloid beta-induced cognitive impairment in rodent (Wang et al., 2014b) and acts through TLR4 blockade (Schwenkgrub et al., 2017a), reduction of pro-inflammatory cytokines TNF-alpha, IL6 and IL1-beta (Wakita et al., 2003; Schwenkgrub et al., 2017b), up-regulation the anti-inflammatory cytokine IL4 and IL10 and various neurotrophic factors (Mizuno et al., 2004). Although these drug-protein association should overall be taken with caution, given that the drugs' effects on the network may depend on their dose and on the disease stage and severity, they may be useful to explore the neuroinflammatory cascade involved in Alzheimer’s disease.
In conclusion, our study provides a review of proteins associated to neuroinflammation in Alzheimer’s disease and identifies 11 interrelated key proteins with the highest ability to control neuroinflammatory processes in Alzheimer’s disease. While some proteins such as TNF-alpha are largely evaluated, our data provide evidence to encourage further investigation on others such as CCL2, CXCL8, IL-10, TLR4, CRP or AKT1 that appears as a key component for translating extracellular information into downstream biological responses regulating microglia phenotype. Assessing these proteins could then help for the evaluation of disease-modifying properties, for example for assessing the impact of the recently FDA-approved aducanumab on neuroinflammation-related endpoints. A major challenge for future therapies will be to enhance the protective role or combat the pathological roles of immune processes such as IL4, IL10 and IL13 or TLR-mediated MyD88- and TRAF6- dependent signaling, and this will require to better understanding the time-dependent role of these processes in Alzheimer’s disease. Our study prioritizes biomarkers and putative targets and identifies non-coding RNAs and pharmacological compounds with single or pleiotropic actions acting on them. As such, it may facilitate the way to novel diagnostic and therapeutic neuroprotective strategies for Alzheimer’s disease.
Data Availability Statement
The original contributions presented in the study are included in the article/Supplementary Material, further inquiries can be directed to the corresponding author.
Author Contributions
FI contributed to the methodology and the execution of the study. DD and BG revised the manuscript providing expertise in pharmacology and clinical neurology. KB designed the study, contributed to the methodology and execution of the study, analyzed the data and wrote the manuscript.
Funding
This research was funded by Région Hauts-de-France (Projet Santé Environnement; KB), University of Lille and Centre National de la Recherche Scientifique (Projet Exploratoire Premier Soutien PEPS; KB), Lille FHU-VasCog (KB) and Campus France (KB).
Conflict of Interest
The authors declare that the research was conducted in the absence of any commercial or financial relationships that could be construed as a potential conflict of interest.
Supplementary Material
The Supplementary Material for this article can be found online at: https://www.frontiersin.org/articles/10.3389/fphar.2021.630003/full#supplementary-material
References
Bakos, E., Thaiss, C. A., Kramer, M. P., Cohen, S., Radomir, L., Orr, I., et al. (2017). CCR2 Regulates the Immune Response by Modulating the Interconversion and Function of Effector and Regulatory T Cells. J. Immunol. 198 (12), 4659–4671. doi:10.4049/jimmunol.1601458
Baran, J., Gerner, M., Haeussler, M., Nenadic, G., and Bergman, C. M. (2011). pubmed2ensembl: a Resource for Mining the Biological Literature on Genes. PLoS One 6 (9), e24716. doi:10.1371/journal.pone.0024716
Belarbi, K., Jopson, T., Tweedie, D., Arellano, C., Luo, W., Greig, N. H., et al. (2012). TNF-α Protein Synthesis Inhibitor Restores Neuronal Function and Reverses Cognitive Deficits Induced by Chronic Neuroinflammation. J. Neuroinflammation 9, 23. doi:10.1186/1742-2094-9-23
Bhaskar, K., Konerth, M., Kokiko-Cochran, O. N., Cardona, A., Ransohoff, R. M., and Lamb, B. T. (2010). Regulation of Tau Pathology by the Microglial Fractalkine Receptor. Neuron 68 (1), 19–31. doi:10.1016/j.neuron.2010.08.023
Bindea, G., Mlecnik, B., Hackl, H., Charoentong, P., Tosolini, M., Kirilovsky, A., et al. (2009). ClueGO: a Cytoscape Plug-In to Decipher Functionally Grouped Gene Ontology and Pathway Annotation Networks. Bioinformatics 25 (8), 1091–1093. doi:10.1093/bioinformatics/btp101
Braig, D., Nero, T. L., Koch, H.-G., Kaiser, B., Wang, X., Thiele, J. R., et al. (2017). Transitional Changes in the CRP Structure lead to the Exposure of Proinflammatory Binding Sites. Nat. Commun. 8, 14188. doi:10.1038/ncomms14188
Chen, F., Ghosh, A., Lin, J., Zhang, C., Pan, Y., Thakur, A., et al. (2020). 5-lipoxygenase Pathway and its Downstream Cysteinyl Leukotrienes as Potential Therapeutic Targets for Alzheimer's Disease. Brain Behav. Immun. 88, 844–855. doi:10.1016/j.bbi.2020.03.022
Cheng, L., Wang, P., Tian, R., Wang, S., Guo, Q., Luo, M., et al. (2019). LncRNA2Target v2.0: a Comprehensive Database for Target Genes of lncRNAs in Human and Mouse. Nucleic Acids Res. 47 (D1), D140–D144. doi:10.1093/nar/gky1051
Chou, A., Krukowski, K., Morganti, J., Riparip, L.-K., and Rosi, S. (2018). Persistent Infiltration and Impaired Response of Peripherally-Derived Monocytes after Traumatic Brain Injury in the Aged Brain. Int. J. Mol. Sci. 19 (6), 1616. doi:10.3390/ijms19061616
Cotto, K. C., Wagner, A. H., Feng, Y.-Y., Kiwala, S., Coffman, A. C., Spies, G., et al. (2018). DGIdb 3.0: a Redesign and Expansion of the Drug-Gene Interaction Database. Nucleic Acids Res. 46 (D1), D1068–D1073. doi:10.1093/nar/gkx1143
Cui, H., Xie, N., Tan, Z., Banerjee, S., Thannickal, V. J., Abraham, E., et al. (2014). The Human Long Noncoding RNA lnc-IL7R Regulates the Inflammatory Response. Eur. J. Immunol. 44 (7), 2085–2095. doi:10.1002/eji.201344126
Daborg, J., Andreasson, U., Pekna, M., Lautner, R., Hanse, E., Minthon, L., et al. (2012). Cerebrospinal Fluid Levels of Complement Proteins C3, C4 and CR1 in Alzheimer's Disease. J. Neural Transm. 119 (7), 789–797. doi:10.1007/s00702-012-0797-8
Dani, M., Wood, M., Mizoguchi, R., Fan, Z., Walker, Z., Morgan, R., et al. (2018). Microglial Activation Correlates In Vivo with Both Tau and Amyloid in Alzheimer's Disease. Brain 141 (9), 2740–2754. doi:10.1093/brain/awy188
Dossumbekova, A., Berdyshev, E. V., Gorshkova, I., Shao, Z., Li, C., Long, P., et al. (2008). Akt Activates NOS3 and Separately Restores Barrier Integrity in H2O2-Stressed Human Cardiac Microvascular Endothelium. Am. J. Physiology-Heart Circulatory Physiol. 295 (6), H2417–H2426. doi:10.1152/ajpheart.00501.2008
Fabiani, C., and Antollini, S. S. (2019). Alzheimer's Disease as a Membrane Disorder: Spatial Cross-Talk Among Beta-Amyloid Peptides, Nicotinic Acetylcholine Receptors and Lipid Rafts. Front. Cel. Neurosci. 13, 309. doi:10.3389/fncel.2019.00309
Fabregat, A., Sidiropoulos, K., Viteri, G., Forner, O., Marin-Garcia, P., Arnau, V., et al. (2017). Reactome Pathway Analysis: a High-Performance In-Memory Approach. BMC Bioinformatics 18 (1), 142. doi:10.1186/s12859-017-1559-2
Fan, Z., Brooks, D. J., Okello, A., and Edison, P. (2017). An Early and Late Peak in Microglial Activation in Alzheimer's Disease Trajectory. Brain 140 (3), aww349–803. doi:10.1093/brain/aww349
Fang, W., Bi, D., Zheng, R., Cai, N., Xu, H., Zhou, R., et al. (2017). Identification and Activation of TLR4-Mediated Signalling Pathways by Alginate-Derived Guluronate Oligosaccharide in RAW264.7 Macrophages. Sci. Rep. 7 (1), 1663. doi:10.1038/s41598-017-01868-0
Fricker, M., Tolkovsky, A. M., Borutaite, V., Coleman, M., and Brown, G. C. (2018). Neuronal Cell Death. Physiol. Rev. 98 (2), 813–880. doi:10.1152/physrev.0001110.1152/physrev.00011.2017
Gabbita, S. P., Srivastava, M. K., Eslami, P., Johnson, M. F., Kobritz, N. K., Tweedie, D., et al. (2012). Early Intervention with a Small Molecule Inhibitor for Tumor Nefosis Factor-α Prevents Cognitive Deficits in a Triple Transgenic Mouse Model of Alzheimer's Disease. J. Neuroinflammation 9, 99. doi:10.1186/1742-2094-9-99
Galimberti, D., Schoonenboom, N., Scheltens, P., Fenoglio, C., Bouwman, F., Venturelli, E., et al. (2006). Intrathecal Chemokine Synthesis in Mild Cognitive Impairment and Alzheimer Disease. Arch. Neurol. 63 (4), 538–543. doi:10.1001/archneur.63.4.538
Gambuzza, M., Sofo, V., Salmeri, F., Soraci, L., Marino, S., and Bramanti, P. (2014). Toll-like Receptors in Alzheimer's Disease: a Therapeutic Perspective. Cnsnddt 13 (9), 1542–1558. doi:10.2174/1871527313666140806124850
Goodwin, J. L., Kehrli, M. E., and Uemura, E. (1997). Integrin Mac-1 and Beta-Amyloid in Microglial Release of Nitric Oxide. Brain Res. 768 (1-2), 279–286. doi:10.1016/s0006-8993(97)00653-7
Guerreiro, R., Wojtas, A., Bras, J., Carrasquillo, M., Rogaeva, E., Majounie, E., et al. (2013). TREM2 Variants in Alzheimer's Disease. N. Engl. J. Med. 368 (2), 117–127. doi:10.1056/NEJMoa1211851
Gulisano, W., Tropea, M. R., Arancio, O., Palmeri, A., and Puzzo, D. (2018). Sub-efficacious Doses of Phosphodiesterase 4 and 5 Inhibitors Improve Memory in a Mouse Model of Alzheimer's Disease. Neuropharmacology 138, 151–159. doi:10.1016/j.neuropharm.2018.06.002
Guo, R., Fan, G., Zhang, J., Wu, C., Du, Y., Ye, H., et al. (2017). A 9-microRNA Signature in Serum Serves as a Noninvasive Biomarker in Early Diagnosis of Alzheimer's Disease. Jad 60 (4), 1365–1377. doi:10.3233/JAD-170343
Haarmann, A., Schuhmann, M., Silwedel, C., Monoranu, C.-M., Stoll, G., and Buttmann, M. (2019). Human Brain Endothelial CXCR2 Is Inflammation-Inducible and Mediates CXCL5- and CXCL8-Triggered Paraendothelial Barrier Breakdown. Int. J. Mol. Sci. 20 (3), 602. doi:10.3390/ijms20030602
Hong, S., Beja-Glasser, V. F., Nfonoyim, B. M., Frouin, A., Li, S., Ramakrishnan, S., et al. (2016). Complement and Microglia Mediate Early Synapse Loss in Alzheimer Mouse Models. Science 352 (6286), 712–716. doi:10.1126/science.aad8373
Huang, H.-Y., Lin, Y.-C. -D., Li, J., Huang, K.-Y., Shrestha, S., Hong, H.-C., et al. (2020). miRTarBase 2020: Updates to the Experimentally Validated microRNA-Target Interaction Database. Nucleic Acids Res. 48 (D1), D148–D54. doi:10.1093/nar/gkz896
Ilott, N. E., Heward, J. A., Roux, B., Tsitsiou, E., Fenwick, P. S., Lenzi, L., et al. (2014). Long Non-coding RNAs and Enhancer RNAs Regulate the Lipopolysaccharide-Induced Inflammatory Response in Human Monocytes. Nat. Commun. 5, 3979. doi:10.1038/ncomms4979
Itagaki, S., McGeer, P. L., and Akiyama, H. (1988). Presence of T-Cytotoxic Suppressor and Leucocyte Common Antigen Positive Cells in Alzheimer's Disease Brain Tissue. Neurosci. Lett. 91 (3), 259–264. doi:10.1016/0304-3940(88)90690-8
Iwamoto, N., Nishiyama, E., Ohwada, J., and Arai, H. (1994). Demonstration of CRP Immunoreactivity in Brains of Alzheimer's Disease: Immunohistochemical Study Using Formic Acid Pretreatment of Tissue Sections. Neurosci. Lett. 177 (1-2), 23–26. doi:10.1016/0304-3940(94)90035-3
Janelidze, S., Mattsson, N., Stomrud, E., Lindberg, O., Palmqvist, S., Zetterberg, H., et al. (2018). CSF Biomarkers of Neuroinflammation and Cerebrovascular Dysfunction in Early Alzheimer Disease. Neurology 91 (9), e867–e877. doi:10.1212/WNL.0000000000006082
Jansen, I. E., Savage, J. E., Watanabe, K., Bryois, J., Williams, D. M., Steinberg, S., et al. (2019). Genome-wide Meta-Analysis Identifies New Loci and Functional Pathways Influencing Alzheimer's Disease Risk. Nat. Genet. 51 (3), 404–413. doi:10.1038/s41588-018-0311-9
Jassal, B., Matthews, L., Viteri, G., Gong, C., Lorente, P., Fabregat, A., et al. (2020). The Reactome Pathway Knowledgebase. Nucleic Acids Res. 48 (D1), D498–D503. doi:10.1093/nar/gkz1031
Jeong, J. Y., Chung, Y. C., and Jin, B. K. (2019). Interleukin-4 and Interleukin-13 Exacerbate Neurotoxicity of Prothrombin Kringle-2 in Cortex In Vivo via Oxidative Stress. Int. J. Mol. Sci. 20 (8), 1927. doi:10.3390/ijms20081927
Jun, G., Naj, A. C., Beecham, G. W., Wang, L. S., Buros, J., Gallins, P. J., et al. (2010). Meta-analysis Confirms CR1, CLU, and PICALM as Alzheimer Disease Risk Loci and Reveals Interactions with APOE Genotypes. Arch. Neurol. 67 (12), 1473–1484. doi:10.1001/archneurol.2010.201
Kawahara, K., Suenobu, M., Yoshida, A., Koga, K., Hyodo, A., Ohtsuka, H., et al. (2012). Intracerebral Microinjection of Interleukin-4/interleukin-13 Reduces β-amyloid Accumulation in the Ipsilateral Side and Improves Cognitive Deficits in Young Amyloid Precursor Protein 23 Mice. Neuroscience 207, 243–260. doi:10.1016/j.neuroscience.2012.01.049
Kim, D. H., Choi, S.-M., Jho, J., Park, M.-S., Kang, J., Park, S. J., et al. (2016). Infliximab Ameliorates AD-Associated Object Recognition Memory Impairment. Behav. Brain Res. 311, 384–391. doi:10.1016/j.bbr.2016.06.001
Kiyota, T., Ingraham, K. L., Swan, R. J., Jacobsen, M. T., Andrews, S. J., and Ikezu, T. (2012). AAV Serotype 2/1-mediated Gene Delivery of Anti-inflammatory Interleukin-10 Enhances Neurogenesis and Cognitive Function in APP+PS1 Mice. Gene Ther. 19 (7), 724–733. doi:10.1038/gt.2011.126
Krstic, D., Madhusudan, A., Doehner, J., Vogel, P., Notter, T., Imhof, C., et al. (2012). Systemic Immune Challenges Trigger and Drive Alzheimer-like Neuropathology in Mice. J. Neuroinflammation 9, 151. doi:10.1186/1742-2094-9-151
Kutmon, M., Ehrhart, F., Willighagen, E. L., Evelo, C. T., and Coort, S. L. (2018). CyTargetLinker App Update: A Flexible Solution for Network Extension in Cytoscape. F1000Res 7, 743. doi:10.12688/f1000research.14613.2
Lambert, J.-C., Heath, S., Heath, S., Even, G., Campion, D., Sleegers, K., et al. (2009). Genome-wide Association Study Identifies Variants at CLU and CR1 Associated with Alzheimer's Disease. Nat. Genet. 41 (10), 1094–1099. doi:10.1038/ng.439
Lambert, J. C., Ibrahim-Verbaas, C. A., Harold, D., Naj, A. C., Sims, R., Bellenguez, C., et al. (2013). Meta-analysis of 74,046 Individuals Identifies 11 New Susceptibility Loci for Alzheimer's Disease. Nat. Genet. 45 (12), 1452–1458. doi:10.1038/ng.2802
Lane, H. C., Anand, A. R., and Ganju, R. K. (2006). Cbl and Akt Regulate CXCL8-Induced and CXCR1- and CXCR2-Mediated Chemotaxis. Int. Immunol. 18 (8), 1315–1325. doi:10.1093/intimm/dxl064
Leidinger, P., Backes, C., Deutscher, S., Schmitt, K., Mueller, S. C., Frese, K., et al. (2013). A Blood Based 12-miRNA Signature of Alzheimer Disease Patients. Genome Biol. 14 (7), R78. doi:10.1186/gb-2013-14-7-r78
Lever, J., Jones, M. R., Danos, A. M., Krysiak, K., Bonakdar, M., Grewal, J. K., et al. (2019). Text-mining Clinically Relevant Cancer Biomarkers for Curation into the CIViC Database. Genome Med. 11 (1), 78. doi:10.1186/s13073-019-0686-y
Li, Y., Li, Y., Huang, S., He, K., Zhao, M., Lin, H., et al. (2017). Long Non-coding RNA Growth Arrest Specific Transcript 5 Acts as a Tumour Suppressor in Colorectal Cancer by Inhibiting Interleukin-10 and Vascular Endothelial Growth Factor Expression. Oncotarget 8 (8), 13690–13702. doi:10.18632/oncotarget.14625
Li, Z., Chao, T.-C., Chang, K.-Y., Lin, N., Patil, V. S., Shimizu, C., et al. (2014). The Long Noncoding RNA THRIL Regulates TNF Expression through its Interaction with hnRNPL. Proc. Natl. Acad. Sci. 111 (3), 1002–1007. doi:10.1073/pnas.1313768111
Liu, Y., Dai, Y., Li, Q., Chen, C., Chen, H., Song, Y., et al. (2020). Beta-amyloid Activates NLRP3 Inflammasome via TLR4 in Mouse Microglia. Neurosci. Lett. 736, 135279. doi:10.1016/j.neulet.2020.135279
Liu, Y., Liu, F., Grundke-Iqbal, I., Iqbal, K., and Gong, C.-X. (2011). Deficient Brain Insulin Signalling Pathway in Alzheimer's Disease and Diabetes. J. Pathol. 225 (1), 54–62. doi:10.1002/path.2912
Mamik, M. K., and Ghorpade, A. (2016). CXCL8 as a Potential Therapeutic Target for HIV-Associated Neurocognitive Disorders. Curr. Drug Targets 17 (1), 111–121. doi:10.2174/1389450116666150626124544
Mendes-Silva, A. P., Pereira, K. S., Tolentino-Araujo, G. T., Nicolau, E. d. S., Silva-Ferreira, C. M., Teixeira, A. L., et al. (2016). Shared Biologic Pathways between Alzheimer Disease and Major Depression: A Systematic Review of MicroRNA Expression Studies. Am. J. Geriatr. Psychiatry 24 (10), 903–912. doi:10.1016/j.jagp.2016.07.017
Mizuno, T., Kurotani, T., Komatsu, Y., Kawanokuchi, J., Kato, H., Mitsuma, N., et al. (2004). Neuroprotective Role of Phosphodiesterase Inhibitor Ibudilast on Neuronal Cell Death Induced by Activated Microglia. Neuropharmacology 46 (3), 404–411. doi:10.1016/j.neuropharm.2003.09.009
Monsonego, A., Zota, V., Karni, A., Krieger, J. I., Bar-Or, A., Bitan, G., et al. (2003). Increased T Cell Reactivity to Amyloid β Protein in Older Humans and Patients with Alzheimer Disease. J. Clin. Invest. 112 (3), 415–422. doi:10.1172/JCI1810410.1172/jci200318104
O'Mara-Eves, A., Thomas, J., McNaught, J., Miwa, M., and Ananiadou, S. (2015). Using Text Mining for Study Identification in Systematic Reviews: a Systematic Review of Current Approaches. Syst. Rev. 4, 5. doi:10.1186/2046-4053-4-5
Oprea, T. I., Bologa, C. G., Brunak, S., Campbell, A., Gan, G. N., Gaulton, A., et al. (2018). Unexplored Therapeutic Opportunities in the Human Genome. Nat. Rev. Drug Discov. 17 (5), 317–332. doi:10.1038/nrd.2018.14
Orsucci, D., Mancuso, M., Caldarazzo Ienco, E., Simoncini, C., Siciliano, G., and Bonuccelli, U. (2013). Vascular Factors and Mitochondrial Dysfunction: a central Role in the Pathogenesis of Alzheimer's Disease. Curr. Neurovasc. Res. 10 (1), 76–80. doi:10.2174/156720213804805972
Pan, Y., Liu, J., and Qi, F. (2019). Identification of Key Candidate Genes and Molecular Pathways in white Fat browning: an Anti-obesity Drug Discovery Based on Computational Biology. Hum. Genomics 13 (1), 55. doi:10.1186/s40246-019-0239-x
Paouri, E., Tzara, O., Kartalou, G.-I., Zenelak, S., and Georgopoulos, S. (2017). Peripheral Tumor Necrosis Factor-Alpha (TNF-α) Modulates Amyloid Pathology by Regulating Blood-Derived Immune Cells and Glial Response in the Brain of AD/TNF Transgenic Mice. J. Neurosci. 37 (20), 5155–5171. doi:10.1523/JNEUROSCI.2484-16.2017
Park, J., Lee, S.-Y., Shon, J., Kim, K., Lee, H. J., Kim, K. A., et al. (2019). Adalimumab Improves Cognitive Impairment, Exerts Neuroprotective Effects and Attenuates Neuroinflammation in an Aβ1-40-Injected Mouse Model of Alzheimer's Disease. Cytotherapy 21 (6), 671–682. doi:10.1016/j.jcyt.2019.04.054
Park, K. W., Baik, H. H., and Jin, B. K. (2009). IL-13-induced Oxidative Stress via Microglial NADPH Oxidase Contributes to Death of Hippocampal Neurons In Vivo. J. Immunol. 183 (7), 4666–4674. doi:10.4049/jimmunol.0803392
Polanco, J. C., Li, C., Bodea, L.-G., Martinez-Marmol, R., Meunier, F. A., and Götz, J. (2018). Amyloid-β and Tau Complexity - towards Improved Biomarkers and Targeted Therapies. Nat. Rev. Neurol. 14 (1), 22–39. doi:10.1038/nrneurol.2017.162
Ransohoff, R. M., and Cardona, A. E. (2010). The Myeloid Cells of the central Nervous System Parenchyma. Nature 468 (7321), 253–262. doi:10.1038/nature09615
Sampaio, E. P., Sarno, E. N., Galilly, R., Cohn, Z. A., and Kaplan, G. (1991). Thalidomide Selectively Inhibits Tumor Necrosis Factor Alpha Production by Stimulated Human Monocytes. J. Exp. Med. 173 (3), 699–703. doi:10.1084/jem.173.3.699
Schett, G., Sloan, V. S., Stevens, R. M., and Schafer, P. (2010). Apremilast: a Novel PDE4 Inhibitor in the Treatment of Autoimmune and Inflammatory Diseases. Ther. Adv. Musculoskelet. 2 (5), 271–278. doi:10.1177/1759720X10381432
Schwartz, M., and Deczkowska, A. (2016). Neurological Disease as a Failure of Brain-Immune Crosstalk: The Multiple Faces of Neuroinflammation. Trends Immunol. 37 (10), 668–679. doi:10.1016/j.it.2016.08.001
Schwenkgrub, J., Zaremba, M., Mirowska-Guzel, D., and Kurkowska-Jastrzebska, I. (2017). Ibudilast: a Nonselective Phosphodiesterase Inhibitor in Brain Disorders. Postepy Hig Med. Dosw (Online) 71 (0), 137–148. doi:10.5604/01.3001.0010.3798
Schwenkgrub, J., Zaremba, M., Joniec-Maciejak, I., Cudna, A., Mirowska-Guzel, D., and Kurkowska-Jastrzębska, I. (2017). The Phosphodiesterase Inhibitor, Ibudilast, Attenuates Neuroinflammation in the MPTP Model of Parkinson's Disease. PLoS One 12 (7), e0182019. doi:10.1371/journal.pone.0182019
Silva, G. B., and Garvin, J. L. (2009). Akt1 Mediates Purinergic-dependent NOS3 Activation in Thick Ascending Limbs. Am. J. Physiol.-Renal Physiol. 297 (3), F646–F652. doi:10.1152/ajprenal.00270.2009
Singhal, A., Simmons, M., and Lu, Z. (2016). Text Mining Genotype-Phenotype Relationships from Biomedical Literature for Database Curation and Precision Medicine. Plos Comput. Biol. 12 (11), e1005017. doi:10.1371/journal.pcbi.1005017
Slevin, M., Matou, S., Zeinolabediny, Y., Corpas, R., Weston, R., Liu, D., et al. (2015). Monomeric C-Reactive Protein-A Key Molecule Driving Development of Alzheimer's Disease Associated with Brain Ischaemia? Sci. Rep. 5, 13281. doi:10.1038/srep13281
Sochocka, M., Koutsouraki, E., Gasiorowski, K., and Leszek, J. (2013). Vascular Oxidative Stress and Mitochondrial Failure in the Pathobiology of Alzheimer's Disease: a New Approach to Therapy. CNS Neurol. Disord. Drug Targets 12 (6), 870–881. doi:10.2174/18715273113129990072
Sokolova, A., Hill, M. D., Rahimi, F., Warden, L. A., Halliday, G. M., and Shepherd, C. E. (2009). Monocyte Chemoattractant Protein-1 Plays a Dominant Role in the Chronic Inflammation Observed in Alzheimer's Disease. Brain Pathol. 19 (3), 392–398. doi:10.1111/j.1750-3639.2008.00188.x
Steen, E., Terry, B. M., J. Rivera, E., Cannon, J. L., Neely, T. R., Tavares, R., et al. (2005). Impaired Insulin and Insulin-like Growth Factor Expression and Signaling Mechanisms in Alzheimer's Disease - Is This Type 3 Diabetes? Jad 7 (1), 63–80. doi:10.3233/jad-2005-7107
Swarbrick, S., Wragg, N., Ghosh, S., and Stolzing, A. (2019). Systematic Review of miRNA as Biomarkers in Alzheimer's Disease. Mol. Neurobiol. 56 (9), 6156–6167. doi:10.1007/s12035-019-1500-y
Szczepanik, A., Funes, S., Petko, W., and Ringheim, G. E. (2001). IL-4, IL-10 and IL-13 Modulate Aβ(1-42)-Induced Cytokine and Chemokine Production in Primary Murine Microglia and a Human Monocyte Cell Line. J. Neuroimmunol 113 (1), 49–62. doi:10.1016/s0165-5728(00)00404-5
Szklarczyk, D., Gable, A. L., Lyon, D., Junge, A., Wyder, S., Huerta-Cepas, J., et al. (2019). STRING V11: Protein-Protein Association Networks with Increased Coverage, Supporting Functional Discovery in Genome-wide Experimental Datasets. Nucleic Acids Res. 47 (D1), D607–D613. doi:10.1093/nar/gky1131
Taipa, R., das Neves, S. P., Sousa, A. L., Fernandes, J., Pinto, C., Correia, A. P., et al. (2019). Proinflammatory and Anti-inflammatory Cytokines in the CSF of Patients with Alzheimer's Disease and Their Correlation with Cognitive Decline. Neurobiol. Aging 76, 125–132. doi:10.1016/j.neurobiolaging.2018.12.019
Tang, R. H., Qi, R. Q., and Liu, H. Y. (2019). Interleukin-4 Affects Microglial Autophagic Flux. Neural Regen. Res. 14 (9), 1594–1602. doi:10.4103/1673-5374.25597510.4103/1673-5374.247422
Tarafdar, A., and Pula, G. (2018). The Role of NADPH Oxidases and Oxidative Stress in Neurodegenerative Disorders. Int. J. Mol. Sci. 19 (12), 3824. doi:10.3390/ijms19123824
Togo, T., Akiyama, H., Iseki, E., Kondo, H., Ikeda, K., Kato, M., et al. (2002). Occurrence of T Cells in the Brain of Alzheimer's Disease and Other Neurological Diseases. J. Neuroimmunol 124 (1-2), 83–92. doi:10.1016/s0165-5728(01)00496-9
Tweedie, D., Ferguson, R. A., Fishman, K., Frankola, K. A., Van Praag, H., Holloway, H. W., et al. (2012). Tumor Necrosis Factor-α Synthesis Inhibitor 3,6′-dithiothalidomide Attenuates Markers of Inflammation, Alzheimer Pathology and Behavioral Deficits in Animal Models of Neuroinflammation and Alzheimer's Disease. J. Neuroinflammation 9, 106. doi:10.1186/1742-2094-9-106
UniProt, C. (2019). UniProt: a Worldwide Hub of Protein Knowledge. Nucleic Acids Res. 47 (D1), D506–D515. doi:10.1093/nar/gky1049
van Oijen, M., Witteman, J. C., Hofman, A., Koudstaal, P. J., and Breteler, M. M. B. (2005). Fibrinogen Is Associated with an Increased Risk of Alzheimer Disease and Vascular Dementia. Stroke 36 (12), 2637–2641. doi:10.1161/01.STR.0000189721.31432.26
Wakita, H., Tomimoto, H., Akiguchi, I., Lin, J.-X., Ihara, M., Ohtani, R., et al. (2003). Ibudilast, a Phosphodiesterase Inhibitor, Protects against white Matter Damage under Chronic Cerebral Hypoperfusion in the Rat. Brain Res. 992 (1), 53–59. doi:10.1016/j.brainres.2003.08.028
Wang, H., Mei, Z. l., Zhong, K. L., Hu, M., Long, Y., Miao, M. X., et al. (2014). Pretreatment with Antiasthmatic Drug Ibudilast Ameliorates Aβ1-42-Induced Memory Impairment and Neurotoxicity in Mice. Pharmacol. Biochem. Behav. 124, 373–379. doi:10.1016/j.pbb.2014.07.006
Wang, P., Xue, Y., Han, Y., Lin, L., Wu, C., Xu, S., et al. (2014). The STAT3-Binding Long Noncoding RNA Lnc-DC Controls Human Dendritic Cell Differentiation. Science 344 (6181), 310–313. doi:10.1126/science.1251456
Wang, W.-X., Rajeev, B. W., Stromberg, A. J., Ren, N., Tang, G., Huang, Q., et al. (2008). The Expression of MicroRNA miR-107 Decreases Early in Alzheimer's Disease and May Accelerate Disease Progression through Regulation of -Site Amyloid Precursor Protein-Cleaving Enzyme 1. J. Neurosci. 28 (5), 1213–1223. doi:10.1523/JNEUROSCI.5065-07.2008
Wang, Z., Clark, N. R., and Ma’ayan, A. (2015). Dynamics of the Discovery Process of Protein-Protein Interactions from Low Content Studies. BMC Syst. Biol. 9, 26. doi:10.1186/s12918-015-0173-z
Westin, K., Buchhave, P., Nielsen, H., Minthon, L., Janciauskiene, S., and Hansson, O. (2012). CCL2 Is Associated with a Faster Rate of Cognitive Decline during Early Stages of Alzheimer's Disease. PLoS One 7 (1), e30525. doi:10.1371/journal.pone.0030525
Wood, J. A., Wood, P. L., Ryan, R., Graff-Radford, N. R., Pilapil, C., Robitaille, Y., et al. (1993). Cytokine Indices in Alzheimer's Temporal Cortex: No Changes in Mature IL-1β or IL-1RA but Increases in the Associated Acute Phase Proteins IL-6, α2-macroglobulin and C-Reactive Protein. Brain Res. 629 (2), 245–252. doi:10.1016/0006-8993(93)91327-o
Yang, J., Wise, L., and Fukuchi, K.-i. (2020). TLR4 Cross-Talk with NLRP3 Inflammasome and Complement Signaling Pathways in Alzheimer's Disease. Front. Immunol. 11, 724. doi:10.3389/fimmu.2020.00724
Yi, H. A., Yi, S. D., Jang, B. C., Song, D. K., Shin, D. H., Mun, K. C., et al. (2005). Inhibitory Effects of Glucosamine on Lipopolysaccharide‐induced Activation in Microglial Cells. Clin. Exp. Pharmacol. Physiol. 32 (12), 1097–1103. doi:10.1111/j.1440-1681.2005.04305.x
Zhang, D., Hu, X., Qian, L., Chen, S.-H., Zhou, H., Wilson, B., et al. (2011). Microglial MAC1 Receptor and PI3K Are Essential in Mediating β-amyloid Peptide-Induced Microglial Activation and Subsequent Neurotoxicity. J. Neuroinflammation 8 (1), 3. doi:10.1186/1742-2094-8-3
Zhang, K., Tian, L., Liu, L., Feng, Y., Dong, Y.-B., Li, B., et al. (2013). CXCL1 Contributes to β-Amyloid-Induced Transendothelial Migration of Monocytes in Alzheimer's Disease. PLoS One 8 (8), e72744. doi:10.1371/journal.pone.0072744
Keywords: Alzheimer disease, cytokines, interleukins, microglia, neuroinflammation, toll-like receptors, tumor necrosis factor-alpha, neurodegenerative diseases
Citation: El Idrissi F, Gressier B, Devos D and Belarbi K (2021) A Computational Exploration of the Molecular Network Associated to Neuroinflammation in Alzheimer’s Disease. Front. Pharmacol. 12:630003. doi: 10.3389/fphar.2021.630003
Received: 16 November 2020; Accepted: 29 June 2021;
Published: 15 July 2021.
Edited by:
Claudia Bregonzio, CCT CONICET Córdoba, ArgentinaReviewed by:
Rodrigo Quiroga, National University of Cordoba, ArgentinaSudhanshu P. Raikwar, Barrow Neurological Institute (BNI), United States
Copyright © 2021 El Idrissi, Gressier, Devos and Belarbi. This is an open-access article distributed under the terms of the Creative Commons Attribution License (CC BY). The use, distribution or reproduction in other forums is permitted, provided the original author(s) and the copyright owner(s) are credited and that the original publication in this journal is cited, in accordance with accepted academic practice. No use, distribution or reproduction is permitted which does not comply with these terms.
*Correspondence: Karim Belarbi, karim.belarbi@inserm.fr