- 1Macquarie Medical School, Faculty of Medicine, Health and Human Sciences, Macquarie University, Sydney, NSW, Australia
- 2Melanoma Institute Australia, The University of Sydney, Sydney, NSW, Australia
- 3Department of Medical Oncology, Chris O’Brien Lifehouse, Sydney, NSW, Australia
- 4The Faculty of Medicine and Health, The University of Sydney, Sydney, NSW, Australia
- 5Department of Medical Oncology, Northern Sydney Cancer Centre, Royal North Shore Hospital, Sydney, NSW, Australia
- 6Crown Princess Mary Cancer Centre, Westmead and Blacktown Hospitals, Sydney, NSW, Australia
- 7Department of Melanoma and Surgical Oncology, Royal Prince Alfred Hospital, Sydney, NSW, Australia
- 8Charles Perkins Centre, The University of Sydney, Sydney, NSW, Australia
- 9Department of Tissue Pathology and Diagnostic Oncology, Royal Prince Alfred Hospital and NSW Health Pathology, Sydney, NSW, Australia
Detection of melanoma mutations using circulating tumor DNA (ctDNA) is a potential alternative to using genomic DNA from invasive tissue biopsies. To date, mutations in the GC-rich TERT promoter region, which is commonly mutated in melanoma, have been technically difficult to detect in ctDNA using next-generation sequencing (NGS) panels. In this study, we developed a custom melanoma NGS panel for detection of ctDNA, which encompasses the top 15 gene mutations in melanoma including the TERT promoter. We analyzed 21 stage III and IV melanoma patient samples who were treatment-naïve or on therapy. The overall detection rate of the custom panel, based on BRAF/NRAS/TERT promoter mutations, was 14/21 (67%) patient samples which included a TERT C250T mutation in one BRAF and NRAS mutation negative sample. A BRAF or NRAS mutation was detected in the ctDNA of 13/21 (62%) patients while TERT promoter mutations were detected in 10/21 (48%) patients. Co-occurrence of TERT promoter mutations with BRAF or NRAS mutations was found in 9/10 (90%) patients. The custom ctDNA panel showed a concordance of 16/21 (76%) with tissue based-detection and included 12 BRAF/NRAS mutation positive and 4 BRAF/NRAS mutation negative patients. The ctDNA mutation detection rate for stage IV was 12/16 (75%) and for stage III was 1/5 (20%). Based on BRAF, NRAS and TERT promoter mutations, the custom melanoma panel displayed a limit of detection of ~0.2% mutant allele frequency and showed significant correlation with droplet digital PCR. For one patient, a novel MAP2K1 H119Y mutation was detected in an NRAS/BRAF/TERT promoter mutation negative background. To increase the detection rate to >90% for stage IV melanoma patients, we plan to expand our custom panel to 50 genes. This study represents one of the first to successfully detect TERT promoter mutations in ctDNA from cutaneous melanoma patients using a targeted NGS panel.
Introduction
The analysis of circulating tumor DNA (ctDNA) is progressively being integrated into routine clinical care to monitor cancer recurrence, response to therapy, emergence of resistance and to guide therapy (1–3). In melanoma, ctDNA assessment [reviewed in (4, 5)] predicts overall survival of stage IV melanoma patients treated with BRAF and MEK inhibitors or immunotherapy (6–14) and the relapse-free and melanoma-specific survival of patients with high-risk stage III resected melanoma (15–18). ctDNA can also detect the appearance of treatment-resistant melanoma subclones (6), tumor heterogeneity (19), and metabolic tumor burden (20). ctDNA analysis can inform when to cease therapy (21), predicts disease progression after cessation of immunotherapy (22) and differentiates “true progression” from “pseudoprogression” in melanoma patients treated with immunotherapy (23).
To date, a limited number of studies have employed targeted melanoma next generation sequencing (NGS) panels to analyze mutations in ctDNA (24–29). Targeted ctDNA sequencing panels yielded results concordant with other tissue and liquid biopsy approaches (24–29), and detected ctDNA mutations in 52-74% of stage IV melanoma (24, 25, 27) with 0.1-1.0% limit of detection (LOD). A limitation of these ctDNA panels has been the complete inability to detect TERT promoter mutations. This is a significant disadvantage because TERT promoter mutations are the most frequent recurrent mutations in melanoma, occurring in 34-80% of cutaneous melanomas and are associated with poor survival (30). The most frequent TERT mutations C228T (-124 C>T), C250T (-146 C>T) and CC242TT (138/-139CC>TT) (31–42) occur within high GC DNA regions that is difficult to sequence. As a result, most studies continue to rely on droplet digital PCR (ddPCR), which does not have the multiplexing capabilities of targeted NGS panels, to detect TERT promoter mutations (24, 25).
In cutaneous melanoma TERT promoter mutations commonly co-occur (80-90%) with NF1, BRAF or NRAS mutations, and TERT promoter mutations are also found in 15-60% of BRAF/NRAS/NF1 WT cutaneous melanoma background (33, 38–40, 43–46). This highlights the need for any melanoma detection assay to include TERT promoter mutations in order to maximize detection rates in BRAF/NRAS WT patients. The Guardant360 NGS ctDNA assay (Guardant Health, Redwood City, CA, USA) includes TERT promoter mutations (47–50), but this pan-cancer panel is not adjustable or specifically tailored for melanoma and requires an allele frequency above 0.25% to detect mutations with 100% sensitivity. We wanted to explore whether anchored multiplex PCR technology (51), which enables the enrichment of target DNA using gene specific primers located at only one end of the DNA, could concurrently detect TERT promoter mutations and driver oncogenes in melanoma liquid biopsies. In this proof of principle study we developed a pilot melanoma NGS panel for ctDNA analysis incorporating 15 genes and the TERT promoter. The performance of this custom melanoma mutation panel was evaluated in 21 stage III and IV cutaneous melanoma patient blood samples and compared directly to our previous custom melanoma panel which was based on an Ampliseq-HD workflow (25). Our data confirm that anchored multiplex PCR provided a sensitive and specific melanoma liquid biopsy assay that detects common TERT promoter mutations. The design of this panel can be expanded and adjusted to incorporate treatment resistance and predictive mutations and is, therefore, particularly valuable in cutaneous melanoma where most patients will ultimately relapse while on treatment with targeted or immune checkpoint therapies.
Materials and Methods
Human Melanoma Samples
The fresh-frozen tissue and blood samples from melanoma patients used in the current study were obtained from the Melanoma Institute Australia biospecimen bank with written informed patient consent and institutional review board approval (Sydney Local Health District Human Research Ethics Committee, Protocol No. X15–0454 and HREC/11/RPAH/444). Healthy blood samples were obtained with written informed patient consent and institutional review board approval (Macquarie University Human Research Ethics Committee Protocol No. 52020195621941). The Oncofocus/OncoCarta panels v1.0 (Agena Bioscience, San Diego, CA, USA) or Find IT solid tumor panel (Sonic Genetics, Macquarie Park, NSW, Australia) were used for detection of melanoma-associated BRAF, NRAS, KRAS and KIT variants in tissue samples (52, 53). Immunohistochemistry to detect BRAF V600E using VE1 monoclonal antibody (Abcam, Cambridge, UK) was performed as previously described (54).
Blood (10 ml) was either collected in EDTA tubes (Becton Dickinson, Franklin Lakes, NJ, USA) and processed within 4 h from blood draw or Cell-Free DNA collection tubes (Roche, Basel, Switzerland) and processed within 4 days from blood draw. Tubes were spun at 800 g for 15 min at room temperature. Plasma was then removed into new 15 ml tubes without disturbing the buffy coat and respun at 1600 g for 10 min at room temperature to remove cellular debris. Plasma was stored in 1-2 ml aliquots at -80°C.
Purification of Circulating Free DNA (cfDNA) From Plasma
Plasma cfDNA was purified using the QIAamp circulating nucleic acid kit (Qiagen, Hilden, Germany) according to the manufacturer’s instructions. cfDNA was purified from to 2-5 mL of plasma. cfDNA was subsequently quantified using a Qubit dsDNA high sensitivity assay kit and a Qubit fluorometer 3 (Life Technologies, Carlsbad, CA, USA), according to the manufacturer’s instructions.
Purification of DNA From Melanoma Cell Lines
Short term cultures (1-2 weeks) of melanoma cell lines were maintained in Dulbecco’s Modified Eagle media supplemented with 10% heat inactivated fetal bovine serum (Sigma-Aldrich, St Louis, MO, USA), 4 mM glutamine (Sigma-Aldrich, St Louis, MO, USA), and 20 mM HEPES (Sigma-Aldrich, St Louis, MO, USA), at 37°C in 5% CO2. Spent medium (4 ml) was harvested 48-72 h after splitting of cells. Medium was centrifuged at 800 g for 15 min and supernatant transferred to a new tube and spun at 1600 g for 10 min. Double spun supernatant was then aliquoted into cryovials and stored at -80°C. DNA was subsequently extracted from harvested medium supernatant as described for plasma cfDNA.
Custom Melanoma Gene Panel for Targeted NGS of cfDNA
A made-to-order melanoma gene panel consisting of individual forward and reverse primers was obtained from ArcherDX a subsidiary of Invitae (San Francisco, CA, USA). The panel covers nucleotide variants which give rise to melanoma-associated amino acid changes across 15 gene targets (42, 55–58), as well as melanoma-associated nucleotide variants in the promoter region of the TERT gene (36, 59) (Table S1).
The targeted NGS workflow was based on anchored multiplex PCR (AMP) (51). This consists of the use of anchored nested gene specific primers coupled with universal primers in two rounds of PCR amplification. NGS library preparation and sequencing workflows were according to the manufacturer’s protocols (ArcherDX Liquidplex protocol for Illumina version LA090.2) with panel-specific volumes and cycling conditions according to the manufacturer’s product insert (ArcherDX Liquidplex Macquarie Melanoma version LA771.1). For the second PCR reaction the number of cycles was reduced from 20 to 19.
Library QC and sequencing was performed by the Australian Genome Research Facility (AGRF) Sydney node (Westmead, NSW, Australia). Library quality was assessed using a high sensitivity D1000 screen tape on an Agilent Tapestation 2200 (Agilent, Santa Clara, CA, USA). Individual libraries were quantified using an NEBNext Library Quant Kit for Illumina (NEB, Ipswich, MA, USA) using a CFX384 Real-Time System (Bio-Rad, Hercules, CA, USA). Library concentrations were calculated using a size of 150 bp and subsequently pooled to 4 nM. The final library concentration used for sequencing was 18 pM and included 10% PhiX. Sequencing was performed using a MiSeq reagent kit V3 (600 cycle) (Illumina, San Diego California) run as 300 cycle (150 bp PE) on a MiSeq instrument (Illumina, San Diego California).
Analysis of fastq sequencing files was performed using Archer suite analysis version 6.2.7 at https://analysis.archerdx.com according to the manufacturer’s protocol (Archer Analysis 6.0 User Manual). The detection of background sequencing noise was performed using a normal data set consisting of cfDNA from three healthy controls. For SNP/indel detection the thresholds included: AO ≥ 5; UAO ≥ 3; gnomAD AF ≤ 5%; AF ≥ 95MDAF. Rather than setting an average static background (cutoff) noise profile across a panel Archer analysis establishes a position-specific background noise profile for a panel, based on a normal data set, and this information is used to determine the 95MDAF for every potential variant covered by the panel. Thus, 95MDAF is a function of the sequencing coverage of the variant position and the likelihood of that variant appearing as a result of noise. This position-specific value will therefore differ between variants within the same sample and for the same variant across different samples depending on the sequencing coverage (Invitae 95MDAF technical note APM059.A).
● Alternate observation (AO)=Total number of reads that support the alternate allele.
● Unique alternate observation (UAO)=Total number of unique start sites represented by all the alternate reads that intersect the variant.
● Allele fraction (AF)= Reads that support the alternative allele (AO/DP).
● Depth (DP)= The total high quality unique molecule depth covering the variant.
● gnomAD AF =The frequency of the allele called at this locus, from gnomAD global population (60) (https://gnomad.broadinstitute.org).
● 50 Minimal detectable allele fraction (50MDAF)= The AF at which we would consider a variant significant (i.e., above the background noise) given the provided Normal Data Set and taking all consensus reads into account. If the true AF in the sample is at least this, and this identical experiment were run multiple times, 50% of the time there would be sufficient signal to capture this variant.
● 95 Minimal detectable allele fraction (95MDAF) = The AF at which we would consider a variant significant (i.e., above the background noise) given the provided Normal Data Set and taking all consensus reads into account. If the true AF in the sample is at least this, and this identical experiment were run multiple times, 95% of the time there would be sufficient signal to capture this variant.
● Read or unique fragment = Deduplicated consensus read (i.e. molecular bin) having the same unique molecular barcode.
ddPCR Analysis
The copy number of ctDNA per μl and MAF was determined using the QX200 ddPCR (Bio-Rad, Hercules, CA, USA) as previously described (7). The amount of input DNA template varied for plasma cfDNA while for melanoma cell lines 5 ng of DNA was used for ddPCR. Cancer-associated BRAF V600E and NRAS Q61K/L/R mutations were detected using ddPCR mutation detection assays (Bio-Rad, Hercules, CA, USA). TERT promoter mutations -124 C>T and -146 C>T were identified using ddPCR expert design assays dHsaEXD20945488 (TERT C228T_88) and dHsaEXD85215261 (TERT C250T_88) (61) (Bio-Rad, Hercules, CA, USA), according to the manufacturer’s instructions. The TERT promoter assays were optimized by inclusion of 200 μM 7-deaza-dGTP (New England Biolabs, Ipswich, MA, USA), as previously described (25, 62). The DNA copy number/μl for mutant and wild-type circulating DNA species was determined with QuantaSoft software version 1.7.4 (Bio-Rad, Hercules, CA, USA) using a manual threshold setting. The minimum number of positive droplets for calling a mutation was set at two.
Statistical Analysis
Pearson correlation coefficient analysis and generation of violin plots was performed using Graphpad Prism version 9.1.2.
Results
Cohort and Sample Characteristics
A total of 19 cutaneous melanoma patients and three healthy controls were recruited between August 2015 and May 2021. Of the 19 melanoma patients, 2 had blood samples collected at 2 timepoints making a total of 21 melanoma samples for NGS analysis. Of the melanoma patients, 4/19 (21%) had stage III melanoma with a median age of 73 years (63–83) and 15/19 (79%) had stage IV melanoma with a median age of 65 years (30–88) (Table 1). The median age of the healthy control cohort was 39 years (range 29-54) and consisted of 2 females and one male.
Tissue mutation analysis was available for all patients: 8/19 (42%) had a BRAF V600 mutation, 1/19 (5%) had a BRAF non-V600 mutation, 6/19 (32%) had an NRAS Q61 mutation and 4/19 (21%) patients were BRAF/NRAS wild type (Table 1).
Evaluation of the Performance of the Custom Melanoma ctDNA Panel
Based on the 15 gene targets (Table S1) our custom melanoma ctDNA panel was predicted to cover 66% of cutaneous melanoma patients’ mutations [skin cutaneous melanoma TCGA dataset (45, 46)], 88% of uveal melanoma patients’ mutations [uveal melanoma TCGA dataset (45, 46)] and 24% of acral melanoma patients’ mutations [acral melanoma TCGA dataset (45, 46)]. For cutaneous melanoma the combination of BRAF V600 and NRAS Q61 mutation targets in the custom panel was predicted to cover 60% of patients [skin cutaneous melanoma TCGA dataset (45, 46)]. Addition of TERT promoter mutation targets in the custom panel was predicted to further increase coverage by ~15% in those cutaneous melanoma patients that are NRAS or BRAF WT (33, 38). In contrast for uveal melanoma TERT promoter mutations are extremely rare (63, 84). For this reason, we focused only on cutaneous melanoma when testing the panel. The custom panel design included several gene targets that were not included in our previous Thermofisher custom panel design (25) and several gene targets not included in the commercial Guardant360 pan-cancer panel (Table S1).
The performance of the custom melanoma ctDNA panel was initially evaluated based on unique amplification fragments generated from each forward (+) and reverse (-) gene specific primer (with the universal primer) for each of the 34 target regions (Figure 1A). Overall, for the 24 samples analyzed, made up of 21 melanoma patient samples and 3 healthy controls, the mean coverage (based on unique fragments) was similar for the majority of fragments (range 423-1831 unique fragments across 34 target regions). The lowest mean unique fragment count was observed for both fragments covering the STK19 gene target (STK19- and STK19+ 423 and 478 fragments, respectively), and the TERT promoter primers produced 1250 and 768 unique fragments for the TERT+ and TERT- primers, respectively (Figure 1A).
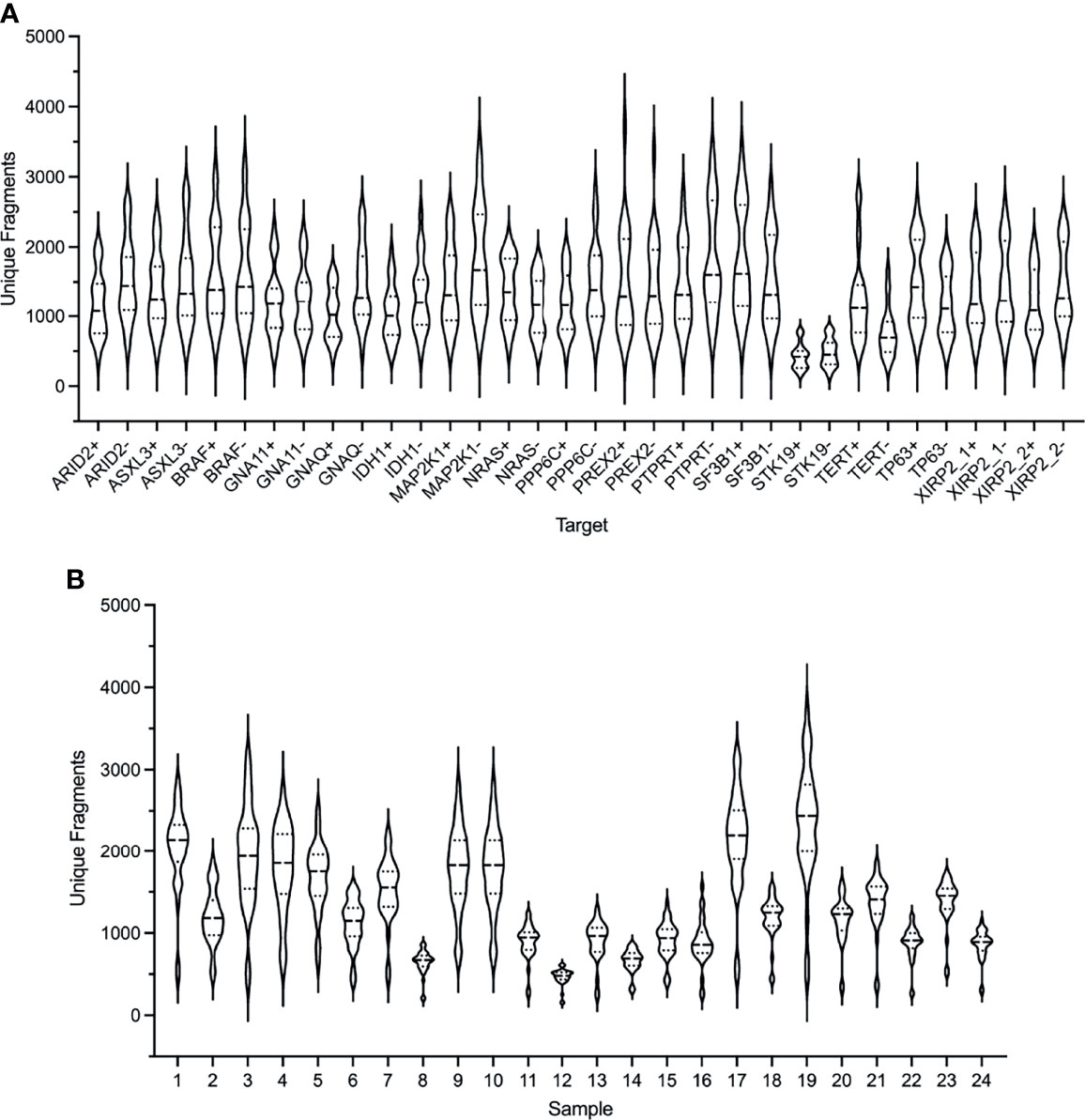
Figure 1 Performance of the ArcherDX custom melanoma ctDNA panel. (A) Distribution of unique fragments for each gene specific target in the custom melanoma panel based on sequencing of 24 samples. For each gene target (see Table S1 for targets) both + and – DNA strand generated fragments are shown. (B) Distribution of unique fragments obtained for each sample based on the 34 targets shown in (A). Samples 1-21 are melanoma patients and 22-24 are healthy controls. Next generation sequencing (NGS) libraries were generated using an ArcherDX Liquidplex NGS workflow followed by Illumina MiSeq sequencing. Unique fragments were defined as deduplicated consensus reads having the same unique molecular barcode. Violin plots show median and SD.
Although each sample NGS library passed QC (as described in material and methods) and was sequenced at the same final concentration, there was variation in the median unique fragment count across the 34 amplified target regions for each sample (Figure 1B). This did not predict the ability to detect ctDNA mutations as the 7 mutation negative samples (7, 8, 12, 15, 17, 18, 20 and 21) had a median unique fragment count ranging from low to high (Figure 1A: median unique fragment count values of 479-2194). Further, this variation in the median unique fragment count was not reflected in the raw paired end reads obtained for each sample which were generally similar (median 654,127 reads) with the exception of sample 7 which had 1,0297,547 raw paired end reads (Figure S1). Although sample 7 had the highest paired end reads (Figure S1), it did not have the highest mean unique fragment count (Figure 1B). Variation in sample performance was not due to the quantity of cfDNA template. For 20 of 24 samples the input was 20 ng while for the remaining samples 10, 20, 22 and 24 the input range was 11.9-15.9 ng. Those samples with the lowest cfDNA input did not yield consistently low median unique fragment counts (Figure 1B). Variations in the size distribution of ctDNA may account for variation in sequencing performance. It has been shown that enrichment of ctDNA in the size range 90-150 bp from patients with melanoma improves ctDNA detection by sequencing (64).
Identification of Melanoma BRAF and NRAS Mutations Using the Custom Melanoma ctDNA Panel
The ArcherDX custom melanoma ctDNA panel detected 13/17 (76%) patients with BRAF- or NRAS-mutant melanoma. This included 12/14 (86%) stage IV and 1/3 (33%) stage III melanoma patients (Figure 2). Liquid biopsies revealed an additional NRAS Q61R-driver mutation in the BRAF V600-mutant samples 4 and 59 from the same patient, and both NRAS mutations were at less than 1% allele frequency compared to the ~30% BRAF mutation frequency (Tables S2, S3). Initially, only tissue immunohistochemistry was used to detect BRAF V600E (65) in these samples. Subsequently, ddPCR analysis of a patient derived melanoma cell line (corresponding to a timepoint 12 months after liquid biopsy samples 4 and 9) confirmed the BRAF V600E mutation but not the NRAS Q61R mutation (ddPCR data not shown). The negative NRAS Q61R signal is likely due to the time point difference from liquid biopsy samples and the fact this was a selected cell population highlighting the subclonal nature of the NRAS mutation. Although, oncogenic BRAF and NRAS mutations are usually mutually exclusive (66), they co-occur in approximately 7% of untreated melanoma and NRAS mutations confer resistance in 27% of BRAFV600-mutant melanoma patients who progress on combination BRAF and MEK inhibitor therapy (67). It is worth noting that this patient (corresponding to samples 4 and 9) had prior combination dabrafenib and trametinib (combi-DT) treatment. The fact that two liquid biopsy samples collected from the same patient at different times displayed identical BRAF/NRAS mutation profiles provides confidence that these mutation data are accurate.
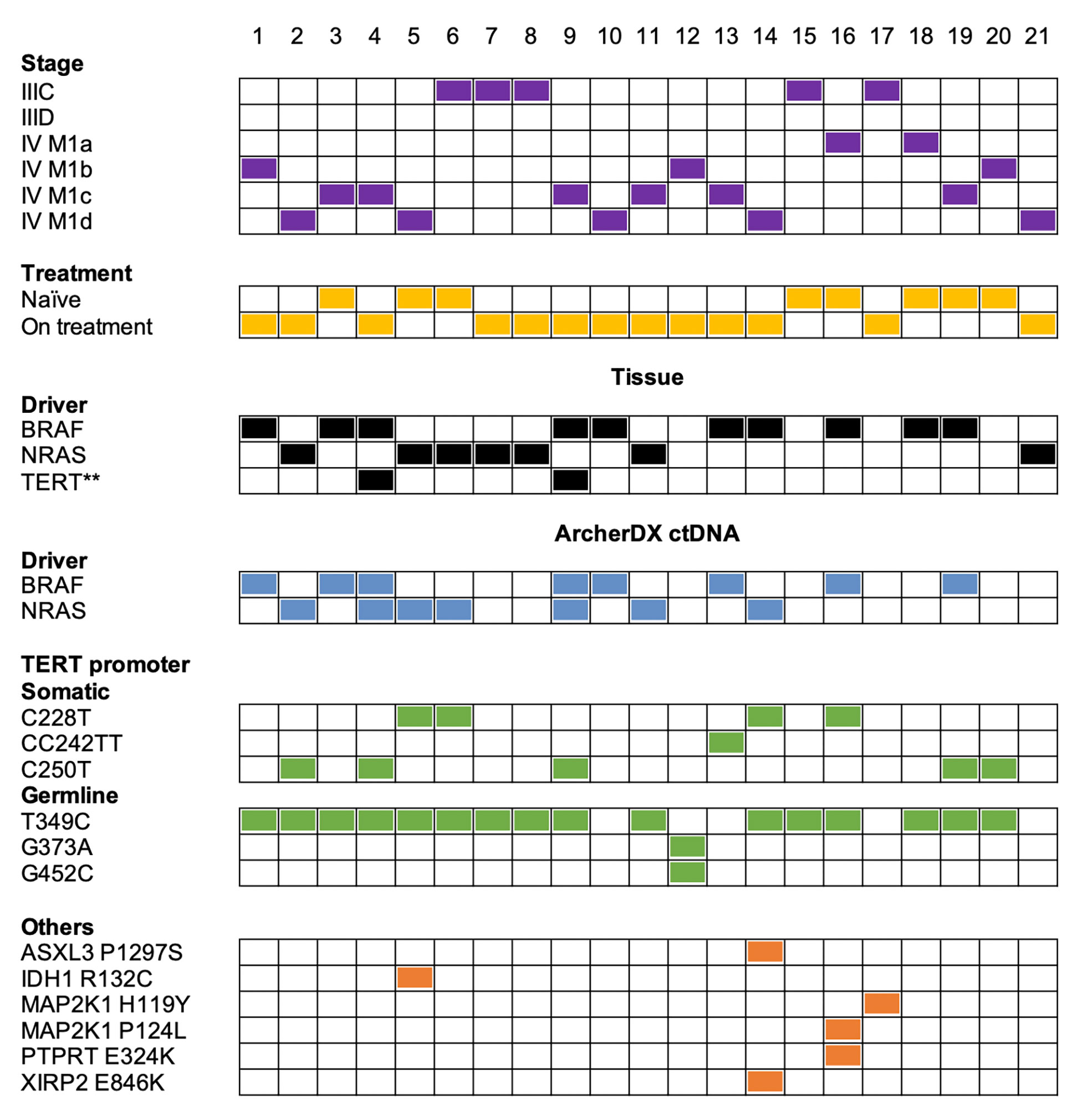
Figure 2 Summary of the melanoma mutation profile identified across the 21 melanoma patient samples from a cohort of 19 melanoma patients. Comparison of melanoma stage (purple boxes), treatment (yellow boxes), tissue driver mutations (black boxes) versus mutations detected with the ArcherDX custom melanoma ctDNA panel including driver mutations (blue boxes), TERT promoter somatic mutations or germline variations (green boxes) and other cancer-associated mutations (orange boxes). Numbers represent sample number. For further details on specific gene variations identified and patient details refer to Table S2. Samples 4 and 9 are derived from a single patient at two time points. Samples 7 and 8 are derived from a single patient at two time points. **Mutation data from a cell line derived from patient tissue collected 12 months after liquid biopsy samples.
Mutations detected in plasma and tissue were concordant in 16/21 patients (76%) and included 12 BRAF/NRAS mutation positive and 4 BRAF/NRAS mutation negative patients (Figure 2). Five samples (samples 7, 8, 14, 18, 21) had a detectable driver mutation in the tissue that was not identified in the liquid biopsy (Table S2). We confirmed in samples 7, 8 and 18 that the driver mutation could not be detected in ctDNA using single molecule ddPCR (Table S3). Analysis of an NRAS Q61R-mutant NM47 melanoma cell line (68) confirmed that the Q61R ddPCR signal for patient-matched stage III samples 7 and 8 consists of low droplet counts with low intensity (Figure S3). Thus, we cannot confidently detect the NRAS Q61R mutation in these two samples by ddPCR or sequencing. ddPCR was unable to detect a BRAF V600E mutation in sample 18 (Table S3). Sample 14 had a BRAF G469E mutation which was not covered by our custom ArcherDX panel (Table S1). BRAF G469E is a deactivating BRAF mutation that promotes melanomagenesis through oncogenic NRAS (45, 46, 69). This patient has the less common NRAS G60V detected in the liquid biopsy (Table S2). This NRAS mutation [TCGA dataset (45, 46)] is predicted to be a driver mutation (COSM4606360). For sample 21 no remaining ctDNA or plasma was available for ddPCR.
Identification of Melanoma TERT Promoter Mutations Using the Custom Melanoma ctDNA Panel
In contrast to our previous NGS study using a Thermofisher custom melanoma ctDNA panel (25), the ArcherDX custom melanoma ctDNA panel detected TERT promoter mutations in ctDNA. Cancer-associated TERT promoter mutations (C228T, CC242TT and C250T) (36, 59) were identified in 10/21 (48%) of melanoma samples (Figure 2). Of these 5/10 (50%) were C250T, 4/10 (40%) were C228T and 1/10 (10%) was CC242TT. For patient ID 4, corresponding to samples 4 and 9, we did confirm TERT promoter mutation C250T in a cell line derived from tissue of the same patient collected 12 months after liquid biopsy using ddPCR (Figure 2; ddPCR data not shown). This distribution of TERT promoter mutations aligns with previous studies on melanoma (33, 38). Co-occurrence of TERT promoter mutations with either BRAF or NRAS mutations was found in 9/10 (90%) of the samples. The detection of a TERT C250T mutation in sample 20 (Figure 2), which was BRAF and NRAS mutation negative based on both the tissue panel and custom panel (Table S2), increased the overall detection rate for the custom panel from 13/21 (62%) to 14/21 (67%) (based on BRAF/NRAS/TERT promoter).
Although unique fragment coverage for TERT promoter centered around cancer-associated TERT promoter mutations, the nature of anchored multiplex PCR did result in coverage beyond the target region albeit with lower read depth (Figure S2). Therefore we were able to detect a number of previously described germline TERT promoter variations (70) including T349C (rs2853669), G373A (rs35226131) and G452C (rs35161420) at MAFs ranging from 38-99% (Figure 2 and Table S2). None of these variations were present in the 3 healthy controls (data not shown). Of these previously described germline variations, T349C was found in 14/21 (67%) patients while G373A and G452C co-occurred in 1/21 (5%) patients (Figure 2). A previous study found TERT T349C to be present in 52% of melanoma cell lines (43). The high frequency of T349C also aligns with previous studies on other cancers (70–72). All the TERT promoter mutations C228T and C250T and 14/17 (82%) BRAF/NRAS mutations co-occurred with the germline TERT promoter variant T349C (Figure 2). There was no clear relationship between either melanoma stage or BRAF/NRAS mutation status and the presence of TERT promoter variant T349C (Figure 2).
Identification of Other Melanoma Cancer-Associated Mutations Using the Custom Melanoma ctDNA Panel
Several other cancer-associated mutations were identified in 4/21 (19%) patients using the ArcherDX custom melanoma ctDNA panel (Figure 2). Of the BRAF and NRAS mutation negative samples, the custom panel was able to detect a MAP2K1 H119Y mutation in sample 17 (Figure 2). The patient, who was BRAF V600 WT, had been treated for 1 week with pembrolizumab and subsequently maintained persistently detectable disease. This mutation has been recently proposed to be a non-hotspot MAP2K1 mutation which activates the ERK pathway (73) and therefore could be targeted with an ERK inhibitor (74). The remaining mutations did not increase detection coverage of the custom panel as they all co-occurred with either BRAF or NRAS driver mutations (Figure 2). Of note, PTPRT E324K, along with MAP2K1 P124L, was detected in treatment naïve sample 16 which also harbored a BRAF V600K mutation (Figure 2). In melanoma, mutations in PTPRT, such as E324K, which create neoepitopes, may be associated with better outcomes for patients on immunotherapy (75). This may have been an option for this patient who subsequently had a partial response to combination BRAF/MEK inhibition with the presence of MAP2K1 P124L presumably contributing to resistance (76, 77). For sample 14, ASXL3 P1297S and XIRP2 E846K were detected in an NRAS G60V background which was also BRAF G469E (based on tissue biopsy) (Figure 2 and Table S2). Given the MAF of ASXL3 P1297S was 55.88% and this mutation is not a previously identified melanoma-associated mutation (45, 46), suggests that it may be a germline polymorphism or a result of clonal hematopoiesis, which has been noted for mutations in the related protein ASXL1 (78). Sample 5 had an NRAS Q61 and IDH1 R132 mutation in the liquid biopsy and the co-occurrence of these mutations (Figure 2) is significant (p<0.001) based on the skin cutaneous melanoma TCGA database analysis of mutual exclusivity (45, 46).
Sensitivity of the Custom Melanoma ctDNA Panel
The cutoff for calling a mutation, based on the 95% confidence interval for the normal samples (95MDAF as defined in material and methods), of the custom melanoma ctDNA panel was inversely proportional to read depth but did approach a saturation point beyond which increasing read depth did not lead to a lower 95MDAF value (Figure 3). This 95MDAF value was ~0.3% for BRAF, 0.26% for TERT promoter mutations and 0.2% for NRAS mutations (Figure 3). The custom panel was also able to detect a MAP2K1 H119Y mutation with an 95MDAF of 0.18% (Table S2). In addition, several cancer-associated mutations were noted whereby the MAF was <95MDAF but still >50MDAF as defined in material and methods (Table S2). For these mutations further validation would be required. To demonstrate the accuracy of these low MAF cutoff values does ultimately require running a dilution series of samples for each mutation consisting of known MAFs.
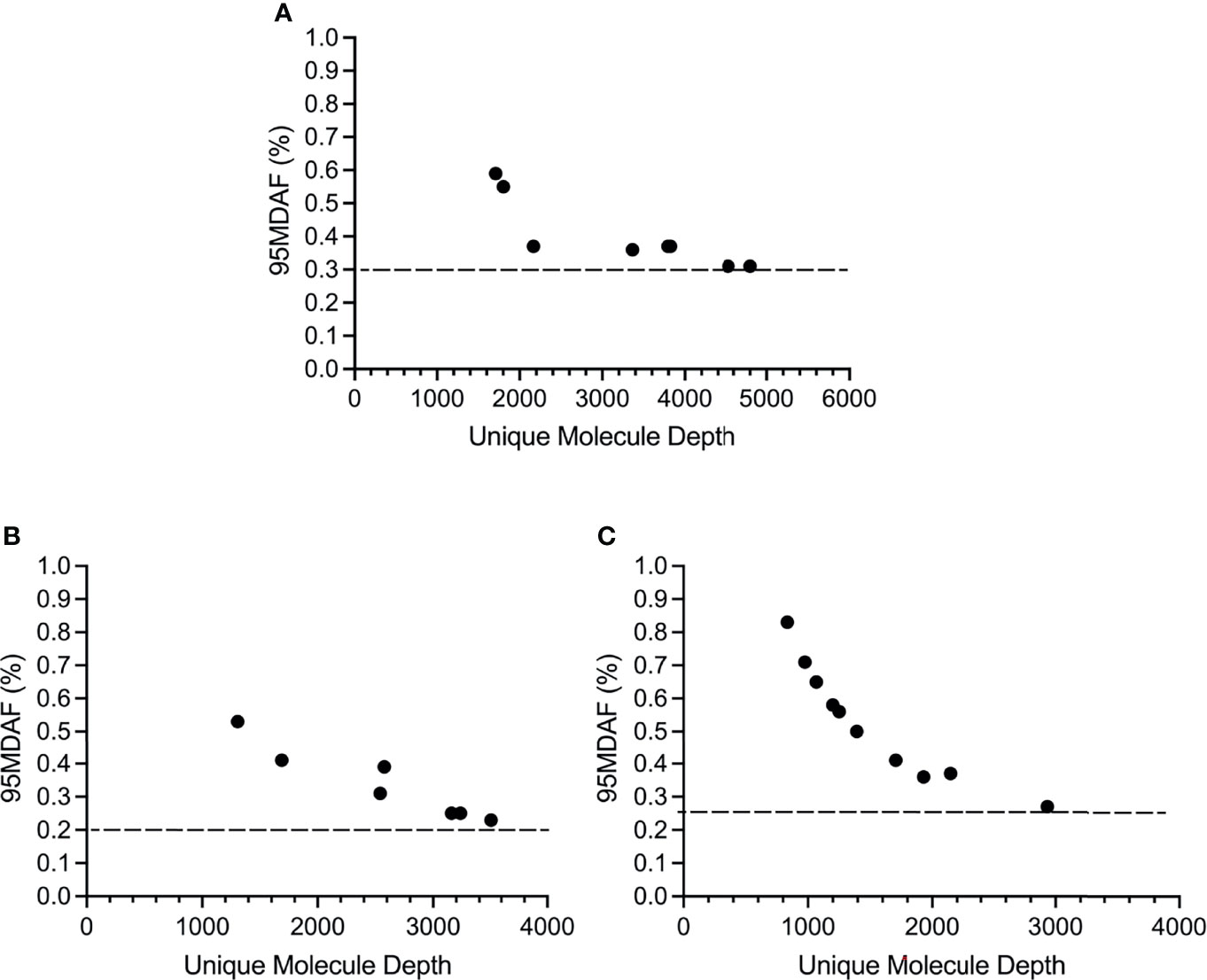
Figure 3 Sensitivity of the custom melanoma ctDNA panel based on BRAF, NRAS or TERT promoter mutations identified across the melanoma cohort. Each circle corresponds to the 95 minimal detectable allele fraction (95MDAF) values and unique molecule depth (unique fragments covering the specified region) for a single sample. (A) BRAF V600 mutations. Data derived from samples 1, 3, 4, 9, 10, 13, 16, 19. (B) NRAS G60/Q61 mutations. Data derived from samples 2, 4, 5, 6, 9, 11, 14. (C) TERT 228-250 promoter mutations. Data derived from samples 2, 4, 5, 6, 9, 13, 14, 16, 19, 20.
Validation of the Custom Melanoma ctDNA Panel
All of BRAF V600E/K or NRAS Q61K/L/R driver mutations identified using the ArcherDX custom melanoma ctDNA panel and tissue biopsy were also identified using either ddPCR or a Thermofisher custom melanoma ctDNA panel (25) (Table S2). Furthermore, there was significant correlation in the MAF for each of these identified mutations when comparing the ArcherDX custom panel to these other liquid biopsy assays (Figure 4A). All of the TERT promoter C228T and C250T mutations identified with the custom panel were confirmed using ddPCR and showed significant correlation based on MAF (Figure 4B).
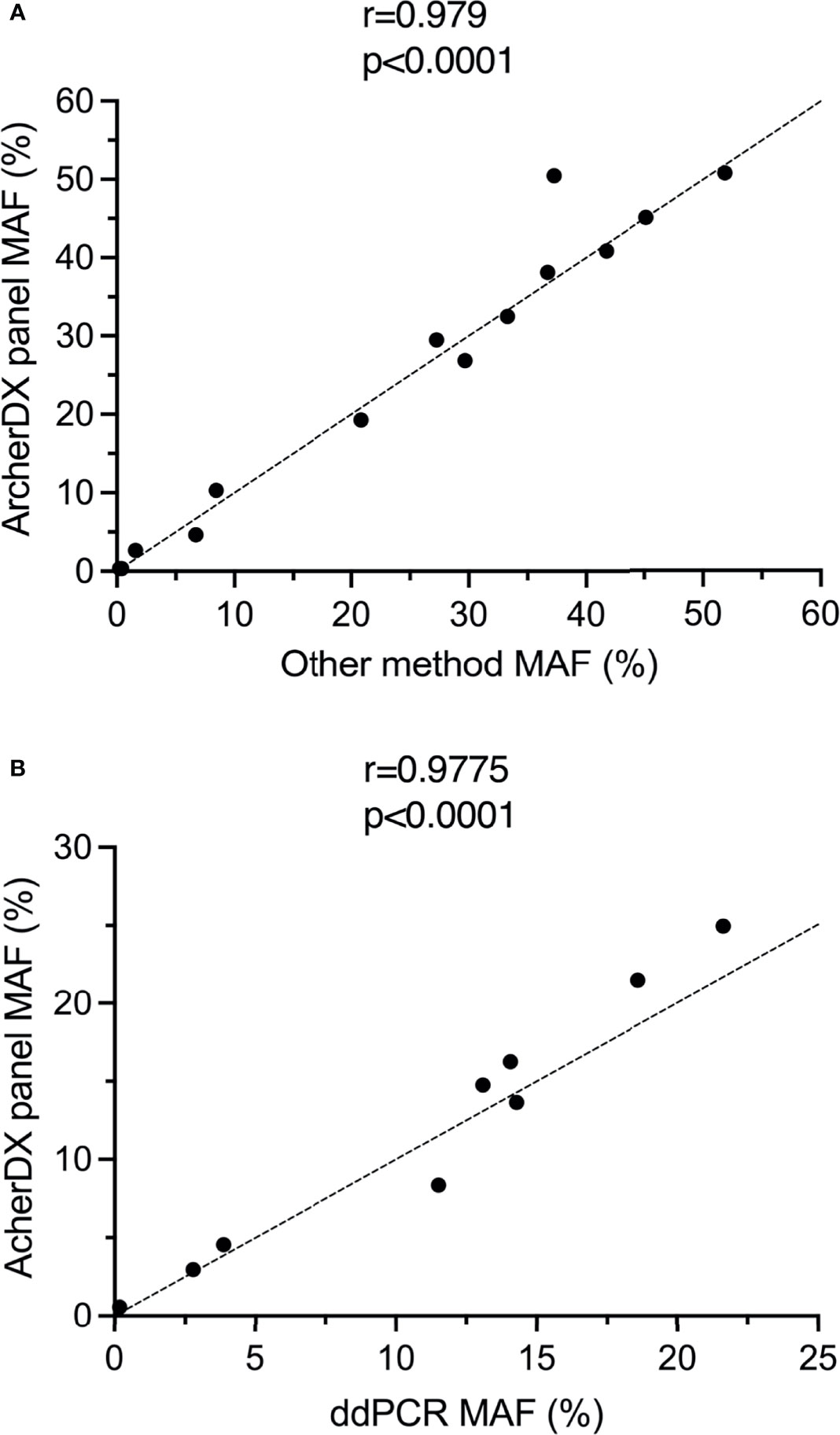
Figure 4 Validation of the ArcherDX custom melanoma ctDNA panel. (A) Correlation of driver mutant allele frequency (MAF) determined by the ArcherDX custom melanoma ctDNA panel versus other liquid biopsy analysis [ddPCR or Thermofisher custom melanoma ctDNA panel (25)]. (B) Correlation of TERT promoter MAF determined by the ArcherDX custom melanoma ctDNA panel versus ddPCR. For ddPCR data see Table S3. Pearson correlation coefficient analysis was performed.
Discussion
In this study, we have developed a custom melanoma NGS panel for detection of ctDNA. This panel was based on multiplex anchored PCR (51) from ArcherDX in contrast to previous studies which used either Thermofisher Ampliseq HD (25, 27), Qiagen QIAseq (24) or Illumina TruSeq Nano (28) workflows. An additional custom melanoma panel based on mass spectrometry detection has also been reported (29). None of these previously reported custom panels have successfully detected TERT promoter mutations, and our previous Thermofisher NGS panel yielded consistently low sequencing depth for the TERT promoter amplicons presumably due to the high GC content (>80%) (25). Given that TERT is one of the most frequently mutated genes in melanoma (42), the primary goal of our NGS panel design was to reliably detect the three common TERT promoter mutations in ctDNA.
The design of the current custom melanoma ctDNA panel was limited to key melanoma-associated gene mutations, but still had a theoretical coverage of ~81% of skin cutaneous melanoma [66% based on skin cutaneous melanoma TCGA database (45, 46) plus (15-60%) triple wild type melanoma with TERT promoter mutations (33, 38–40, 43, 44)]. Mutually exclusive somatic TERT promoter mutations, C250T, C228T and CC242TT, were found in 48% (10/21) of the melanoma cohort and increased the overall ctDNA detection rate from 67%, based on detection of driver (NRAS or BRAF) or cancer-associated mutations, to 71%. The limitation of the current study was that only four triple wild type samples were included. The custom melanoma panel detected only 50% of these four cases (based on detection of a TERT C250T mutation and a MAP2K1 H119Y mutation respectively). Based on a TERT mutation frequency of 15-60% in triple wild type melanoma (33, 38–40, 43, 44) the TERT mutation detection rate of 25% of our wild type melanoma patients using this panel falls within the frequency range. A larger study incorporating many more triple wild-type melanoma samples is needed to accurately determine the detection sensitivity of this pilot ctDNA panel. We expect that analysis of a larger randomly selected or cross-sectional cohort would find more frequent TERT promoter mutations in the absence of BRAF or NRAS mutations, as previously reported (33, 38, 39). It is worth noting, however, that TERT promoter mutations may be subclonal and therefore under-represented in ctDNA relative to other driver mutations (24).
The overall detection rate of 71% in the current study was less than our previous custom ctDNA panel detection rate of 74% primarily due to a larger number of genes covered in our previous study (25). This was the case with lack of detection of BRAF G469E in one patient as this infrequent melanoma mutation (0.1% incidence) was not covered in the custom panel design. On the other hand, the custom panel did detect a rare NRAS G60V mutation in the same patient which was not detected by the tissue panel. This may possibly be due to the fact it is not a listed variant for tissue panels and therefore not called during analysis. A detectability of 20% in the stage III cohort was low although this was only based on five patient samples.
The ability to detect somatic TERT promoter mutations co-occurring with BRAF or NRAS mutations is also valuable given that a TERT-mutation positive genetic profile is associated with a worse prognosis for melanoma patients (30, 38, 79). Further reports suggest that TERT promoter mutations may be predictive of improved response to immunotherapy (80) and a poorer response to BRAF/MEK inhibition (35). Given our small cohort size and the fact that the majority of the patients were non-responders to immunotherapy, we were unable to conclude whether detection of TERT promoter mutations does in fact have any prognostic or predictive value.
We also detected the previously described germline single nucleotide polymorphisms (SNPs) in the TERT promoter T349C, G373A, G452C (70) in 81% of our melanoma cohort at a MAF ranging from 38 to 99%. The most frequent TERT SNP (T349C; rs2853669) was found in 76% of our melanoma cohort. This TERT SNP has been identified in a range of other cancers and may act to disrupt a pre-existing ETS binding site in the TERT promoter (81). Nevertheless, its prognostic role remains controversial with contrasting reports on its influence on TERT expression and differing conclusions on its prognostic value (43, 70–72, 82, 83, 85–88). In melanoma, TERT T349C has been reported to modify the effects of somatic TERT promoter mutations leading to increased survival in melanoma (40) and this may be mediated through a lengthening of telomeres (43). We found no obvious association of this variation either with somatic TERT mutation or patient outcomes in our melanoma cohort.
In addition to successful detection of TERT promoter mutations our current custom melanoma panel performed favorably, based on tissue concordance (76%), 95MDAF (0.2%) and significant correlation with ddPCR, when compared to previously published custom panels (24, 25, 27–29). Future studies will incorporate our findings from previous (25) and current panel designs to produce an optimized melanoma custom ctDNA panel with detection rates of at least 90% in stage IV cutaneous melanoma patients. An optimized melanoma ctDNA (50 gene targets), which will increase the theoretical coverage will be useful for monitoring residual disease in stage III patients after resection and therapy and for longitudinal monitoring of progression in stage IV melanoma patients. In both cases ctDNA mutations identified pre-treatment will be monitored longitudinally using targeted approaches such as ddPCR. To improve ctDNA detection sensitivity ctDNA mutation detection may also be complemented with ctDNA methylation analysis. Ultimately establishing if our optimized melanoma panel truly reaches the theoretical coverage will depend on analysis of a larger cohort consisting of 100-200 patients.
Data Availability Statement
The original contributions presented in the study are publicly available. This data can be found here: https://www.ncbi.nlm.nih.gov/, PRJNA798432, https://www.ncbi.nlm.nih.gov/bioproject/PRJNA798432.
Ethics Statement
The studies involving human participants were reviewed and approved by Sydney Local Health District Human Research Ethics Committee, Protocol No. X15–0454 and HREC/11/RPAH/444. Macquarie University Human Research Ethics Committee Protocol No. 52020195621941. The patients/participants provided their written informed consent to participate in this study.
Author Contributions
Conceptualization, RD, JL, and HR. Methodology, RD and HR. Formal analysis, RD, JL, AS, and HR. Investigation, RD, JL, and AS. Resources, AM, MC, GL, RPMS, JS, and RAS. Writing—original draft preparation, RD and HR. Writing—review and editing, RD, JL, AS, AM, MC, GL, RPMS, JS, RAS, and HR. Visualization, RD, JL, and HR. Supervision, HR. Project administration, HR. Funding acquisition, HR. All authors contributed to the article and approved the submitted version.
Funding
RD was supported in part by a donation to Melanoma Institute Australia from the CLEARbridge Foundation. This work was also supported in part by the National Health and Medical Research Council (APP1093017 and APP1128951). HR, RAS, and GL are supported by National Health and Medical Research Council Research Fellowships (APP1104503, APP1141295 and APP1119059). GL is also supported by the Medical Foundation of The University of Sydney and Melanoma Institute Australia. AMM is supported by a Cancer Institute NSW Fellowship, Nicholas and Helen Moore and Melanoma Institute Australia. JS and RPMS are supported by Melanoma Institute Australia. Support from colleagues at Melanoma Institute Australia, Royal Prince Alfred Hospital and Westmead Hospital is also gratefully acknowledged. Support from The Ainsworth Foundation is also gratefully acknowledged.
Conflict of Interest
GL receives consultant service fees from Amgen, BMS, Array, Pierre-Fabre, Novartis, Merck Sharp & Dohme (MSD), Qbiotics, Roche and Sandoz. AM is an advisory board member for BMS, MSD, Novartis, Roche, Pierre Fabre and Qbiotics. MC is an advisory board member for MSD, BMS, Novartis, Pierre-Fabre, Roche and Amgen. RAS has received fees for professional services from Evaxion, Provectus Biopharmaceuticals Australia, Qbiotics Group Limited, Novartis Pharma AG, MSD Sharp & Dohme (Australia), NeraCare, AMGEN Inc., Bristol-Myers Squibb, Novartis Pharmaceuticals Australia Pty Limited, Myriad Genetics GmbH, GlaxoSmithKline Australia. RPMS has received honoraria for advisory board participation from MSD, Novartis and Qbiotics and speaking honoraria from BMS.
The remaining authors declare that the research was conducted in the absence of any commercial or financial relationships that could be construed as a potential conflict of interest.
Publisher’s Note
All claims expressed in this article are solely those of the authors and do not necessarily represent those of their affiliated organizations, or those of the publisher, the editors and the reviewers. Any product that may be evaluated in this article, or claim that may be made by its manufacturer, is not guaranteed or endorsed by the publisher.
Acknowledgments
Biobank and sample collection support from Hazel Burke, Maria Gonzalez, Rebecca Velickovic, and Rebecca Kasalo (Melanoma Institute Australia) as well as Gilles Guillemin and Benjamin Heng (Macquarie University) is much appreciated. ArcherDX workflow and analysis support from Hamilton Fraval, Zena Kassir and Woei Loon is also much appreciated.
Supplementary Material
The Supplementary Material for this article can be found online at: https://www.frontiersin.org/articles/10.3389/fonc.2022.820510/full#supplementary-material
References
1. Thompson MK, Gale D, Brenton JD. Circulating Tumour DNA for Clinicians: Current and Future Clinical Applications. Clin Radiol (2021) 76(10):737–47. doi: 10.1016/j.crad.2021.07.005
2. Cirmena G, Dameri M, Ravera F, Fregatti P, Ballestrero A, Zoppoli G. Assessment of Circulating Nucleic Acids in Cancer: From Current Status to Future Perspectives and Potential Clinical Applications. Cancers (Basel) (2021) 13(14). doi: 10.3390/cancers13143460
3. Cheng ML, Pectasides E, Hanna GJ, Parsons HA, Choudhury AD, Oxnard GR. Circulating Tumor DNA in Advanced Solid Tumors: Clinical Relevance and Future Directions. CA Cancer J Clin (2021) 71(2):176–90. doi: 10.3322/caac.21650
4. Sacco A, Forgione L, Carotenuto M, Luca A, Ascierto PA, Botti G, et al. Circulating Tumor DNA Testing Opens New Perspectives in Melanoma Management. Cancers (Basel) (2020) 12(10). doi: 10.3390/cancers12102914
5. Gandini S, Zanna I, De Angelis SP, Cocorocchio E, Queirolo P, Lee JH, et al. Circulating Tumour DNA and Melanoma Survival: A Systematic Literature Review and Meta-Analysis. Crit Rev Oncol Hematol (2021) 157:103187. doi: 10.1016/j.critrevonc.2020.103187
6. Gray ES, Rizos H, Reid AL, Boyd SC, Pereira MR, Lo J, et al. Circulating Tumor DNA to Monitor Treatment Response and Detect Acquired Resistance in Patients With Metastatic Melanoma. Oncotarget (2015) 6(39):42008–18. doi: 10.18632/oncotarget.5788
7. Lee JH, Long GV, Boyd S, Lo S, Menzies AM, Tembe V, et al. Circulating Tumour DNA Predicts Response to Anti-PD1 Antibodies in Metastatic Melanoma. Ann Oncol (2017) 28(5):1130–6. doi: 10.1093/annonc/mdx026
8. Santiago-Walker A, Gagnon R, Mazumdar J, Casey M, Long GV, Schadendorf D, et al. Correlation of BRAF Mutation Status in Circulating-Free DNA and Tumor and Association With Clinical Outcome Across Four BRAFi and MEKi Clinical Trials. Clin Cancer Res (2016) 22(3):567–74. doi: 10.1158/1078-0432.CCR-15-0321
9. Valpione S, Gremel G, Mundra P, Middlehurst P, Galvani E, Girotti MR, et al. Plasma Total Cell-Free DNA (cfDNA) is a Surrogate Biomarker for Tumour Burden and a Prognostic Biomarker for Survival in Metastatic Melanoma Patients. Eur J Cancer (2018) 88:1–9. doi: 10.1016/j.ejca.2017.10.029
10. Seremet T, Jansen Y, Planken S, Njimi H, Delaunoy M, El Housni H, et al. Undetectable Circulating Tumor DNA (ctDNA) Levels Correlate With Favorable Outcome in Metastatic Melanoma Patients Treated With Anti-PD1 Therapy. J Transl Med (2019) 17(1):303. doi: 10.1186/s12967-019-2051-8
11. Syeda MM, Wiggins JM, Corless BC, Long GV, Flaherty KT, Schadendorf D, et al. Circulating Tumour DNA in Patients With Advanced Melanoma Treated With Dabrafenib or Dabrafenib Plus Trametinib: A Clinical Validation Study. Lancet Oncol (2021) 22(3):370–80. doi: 10.1016/S1470-2045(20)30726-9
12. Awada G, Jansen Y, Schwarze JK, Tijtgat J, Hellinckx L, Gondry O, et al. A Comprehensive Analysis of Baseline Clinical Characteristics and Biomarkers Associated With Outcome in Advanced Melanoma Patients Treated With Pembrolizumab. Cancers (Basel) (2021) 13(2). doi: 10.3390/cancers13020168
13. Marsavela G, Johansson PA, Pereira MR, McEvoy AC, Reid AL, Robinson C, et al. The Prognostic Impact of Circulating Tumour DNA in Melanoma Patients Treated With Systemic Therapies-Beyond BRAF Mutant Detection. Cancers (Basel) (2020) 12(12). doi: 10.3390/cancers12123793
14. Marsavela G, Lee J, Calapre L, Wong SQ, Pereira MR, McEvoy AC, et al. Circulating Tumor DNA Predicts Outcome From First-, But Not Second-Line Treatment and Identifies Melanoma Patients Who May Benefit From Combination Immunotherapy. Clin Cancer Res (2020) 26(22):5926–33. doi: 10.1158/1078-0432.CCR-20-2251
15. Lee JH, Saw RP, Thompson JF, Lo S, Spillane AJ, Shannon KF, et al. Pre-Operative ctDNA Predicts Survival in High-Risk Stage III Cutaneous Melanoma Patients. Ann Oncol (2019) 30(5):815–22. doi: 10.1093/annonc/mdz075
16. Lee RJ, Gremel G, Marshall A, Myers KA, Fisher N, Dunn JA, et al. Circulating Tumor DNA Predicts Survival in Patients With Resected High-Risk Stage II/III Melanoma. Ann Oncol (2018) 29(2):490–6. doi: 10.1093/annonc/mdx717
17. McEvoy AC, Pereira MR, Reid A, Pearce R, Cowell L, Al-Ogaili Z, et al. Monitoring Melanoma Recurrence With Circulating Tumor DNA: A Proof of Concept From Three Case Studies. Oncotarget (2019) 10(2):113–22. doi: 10.18632/oncotarget.26451
18. Tan L, Sandhu S, Lee RJ, Li J, Callahan J, Ftouni S, et al. Prediction and Monitoring of Relapse in Stage III Melanoma Using Circulating Tumor DNA. Ann Oncol (2019) 30(5):804–14. doi: 10.1093/annonc/mdz048
19. Diefenbach RJ, Lee JH, Strbenac D, Yang JYH, Menzies AM, Carlino MS, et al. Analysis of the Whole-Exome Sequencing of Tumor and Circulating Tumor DNA in Metastatic Melanoma. Cancers (Basel) (2019) 11(12). doi: 10.3390/cancers11121905
20. McEvoy AC, Warburton L, Al-Ogaili Z, Celliers L, Calapre L, Pereira MR, et al. Correlation Between Circulating Tumour DNA and Metabolic Tumour Burden in Metastatic Melanoma Patients. BMC Cancer (2018) 18(1):726. doi: 10.1186/s12885-018-4637-6
21. Warburton L, Meniawy TM, Calapre L, Pereira M, McEvoy A, Ziman M, et al. Stopping Targeted Therapy for Complete Responders in Advanced BRAF Mutant Melanoma. Sci Rep (2020) 10(1):18878. doi: 10.1038/s41598-020-75837-5
22. Warburton L, Calapre L, Pereira MR, Reid A, Robinson C, Amanuel B, et al. Circulating Tumour DNA in Advanced Melanoma Patients Ceasing PD1 Inhibition in the Absence of Disease Progression. Cancers (Basel) (2020) 12(11). doi: 10.3390/cancers12113486
23. Lee JH, Long GV, Menzies AM, Lo S, Guminski A, Whitbourne K, et al. Association Between Circulating Tumor DNA and Pseudoprogression in Patients With Metastatic Melanoma Treated With Anti-Programmed Cell Death 1 Antibodies. JAMA Oncol (2018) 4(5):717–21. doi: 10.1001/jamaoncol.2017.5332
24. Calapre L, Giardina T, Robinson C, Reid AL, Al-Ogaili Z, Pereira MR, et al. Locus-Specific Concordance of Genomic Alterations Between Tissue and Plasma Circulating Tumor DNA in Metastatic Melanoma. Mol Oncol (2019) 13(2):171–84. doi: 10.1002/1878-0261.12391
25. Diefenbach RJ, Lee JH, Menzies AM, Carlino MS, Long GV, Saw RPM, et al. Design and Testing of a Custom Melanoma Next Generation Sequencing Panel for Analysis of Circulating Tumor DNA. Cancers (Basel) (2020) 12(8). doi: 10.3390/cancers12082228
26. Park JJ, Diefenbach RJ, Byrne N, Long GV, Scolyer RA, Gray ES, et al. Circulating Tumor DNA Reflects Uveal Melanoma Responses to Protein Kinase C Inhibition. Cancers (Basel) (2021) 13(7). doi: 10.3390/cancers13071740
27. Berger M, Thueringer A, Franz D, Dandachi N, Talakic E, Richtig G, et al. Circulating Tumor DNA as a Marker for Treatment Response in Metastatic Melanoma Patients Using Next-Generation Sequencing-A Prospective Feasibility Study. Cancers (Basel) (2021) 13(12). doi: 10.3390/cancers13123101
28. Gangadhar TC, Savitch SL, Yee SS, Xu W, Huang AC, Harmon S, et al. Feasibility of Monitoring Advanced Melanoma Patients Using Cell-Free DNA From Plasma. Pigment Cell Melanoma Res (2018) 31(1):73–81. doi: 10.1111/pcmr.12623
29. Gray ES, Witkowski T, Pereira M, Calapre L, Herron K, Irwin D, et al. Genomic Analysis of Circulating Tumor DNA Using a Melanoma-Specific UltraSEEK Oncogene Panel. J Mol Diagn (2019) 21(3):418–26. doi: 10.1016/j.jmoldx.2018.12.001
30. Sheen YS, Chu CY. Co-Occurrence of TERT Promotor Mutations With BRAF or NRAS Alterations Correlates With Worse Prognosis in Melanoma. Br J Dermatol (2021) 184(3):390–1. doi: 10.1111/bjd.19416
31. Huang FW, Hodis E, Xu MJ, Kryukov GV, Chin L, Garraway LA. Highly Recurrent TERT Promoter Mutations in Human Melanoma. Science (2013) 339(6122):957–9. doi: 10.1126/science.1229259
32. Ekedahl H, Lauss M, Olsson H, Griewank KG, Schadendorf D, Ingvar C, et al. High TERT Promoter Mutation Frequency in Non-Acral Cutaneous Metastatic Melanoma. Pigment Cell Melanoma Res (2016) 29(5):598–600. doi: 10.1111/pcmr.12500
33. Nagore E, Heidenreich B, Rachakonda S, Garcia-Casado Z, Requena C, Soriano V, et al. TERT Promoter Mutations in Melanoma Survival. Int J Cancer (2016) 139(1):75–84. doi: 10.1002/ijc.30042
34. Vicente A, Crovador CS, Macedo G, Scapulatempo-Neto C, Reis RM, Vazquez VL. Mutational Profile of Driver Genes in Brazilian Melanomas. J Glob Oncol (2019) 5:1–14. doi: 10.1200/JGO.19.00169
35. Del Bianco P, Stagni C, Giunco S, Fabozzi A, Elefanti L, Pellegrini S, et al. TERT Promoter Mutations Differently Correlate With the Clinical Outcome of MAPK Inhibitor-Treated Melanoma Patients. Cancers (Basel) (2020) 12(4). doi: 10.3390/cancers12040946
36. Horn S, Figl A, Rachakonda PS, Fischer C, Sucker A, Gast A, et al. TERT Promoter Mutations in Familial and Sporadic Melanoma. Science (2013) 339(6122):959–61. doi: 10.1126/science.1230062
37. Andres-Lencina JJ, Rachakonda S, Garcia-Casado Z, Srinivas N, Skorokhod A, Requena C, et al. TERT Promoter Mutation Subtypes and Survival in Stage I and II Melanoma Patients. Int J Cancer (2019) 144(5):1027–36. doi: 10.1002/ijc.31780
38. Manrique-Silva E, Rachakonda S, Millan-Esteban D, Garcia-Casado Z, Requena C, Traves V, et al. Clinical, Environmental and Histological Distribution of BRAF, NRAS and TERT Promoter Mutations Among Patients With Cutaneous Melanoma: A Retrospective Study of 563 Patients. Br J Dermatol (2021) 184(3):504–13. doi: 10.1111/bjd.19297
39. Griewank KG, Murali R, Puig-Butille JA, Schilling B, Livingstone E, Potrony M, et al. TERT Promoter Mutation Status as an Independent Prognostic Factor in Cutaneous Melanoma. J Natl Cancer Inst (2014) 106(9). doi: 10.1093/jnci/dju246
40. Nagore E, Heidenreich B, Requena C, Garcia-Casado Z, Martorell-Calatayud A, Pont-Sanjuan V, et al. TERT Promoter Mutations Associate With Fast-Growing Melanoma. Pigment Cell Melanoma Res (2016) 29(2):236–8. doi: 10.1111/pcmr.12441
41. Populo H, Boaventura P, Vinagre J, Batista R, Mendes A, Caldas R, et al. TERT Promoter Mutations in Skin Cancer: The Effects of Sun Exposure and X-Irradiation. J Invest Dermatol (2014) 134(8):2251–7. doi: 10.1038/jid.2014.163
42. Cancer Genome Atlas N. Genomic Classification of Cutaneous Melanoma. Cell (2015) 161(7):1681–96. doi: 10.1016/j.cell.2015.05.044
43. Shaughnessy M, Njauw CN, Artomov M, Tsao H. Classifying Melanoma by TERT Promoter Mutational Status. J Invest Dermatol (2020) 140(2):390–394 e1. doi: 10.1016/j.jid.2019.06.149
44. Cheng DT, Mitchell TN, Zehir A, Shah RH, Benayed R, Syed A, et al. Memorial Sloan Kettering-Integrated Mutation Profiling of Actionable Cancer Targets (MSK-IMPACT): A Hybridization Capture-Based Next-Generation Sequencing Clinical Assay for Solid Tumor Molecular Oncology. J Mol Diagn (2015) 17(3):251–64. doi: 10.1016/j.jmoldx.2014.12.006
45. Cerami E, Gao J, Dogrusoz U, Gross BE, Sumer SO, Aksoy BA, et al. The Cbio Cancer Genomics Portal: An Open Platform for Exploring Multidimensional Cancer Genomics Data. Cancer Discov (2012) 2(5):401–4. doi: 10.1158/2159-8290.CD-12-0095
46. Gao J, Aksoy BA, Dogrusoz U, Dresdner G, Gross B, Sumer SO, et al. Integrative Analysis of Complex Cancer Genomics and Clinical Profiles Using the Cbioportal. Sci Signal (2013) 6(269):pl1. doi: 10.1126/scisignal.2004088
47. Barata PC, Koshkin VS, Funchain P, Sohal D, Pritchard A, Klek S, et al. Next-Generation Sequencing (NGS) of Cell-Free Circulating Tumor DNA and Tumor Tissue in Patients With Advanced Urothelial Cancer: A Pilot Assessment of Concordance. Ann Oncol (2017) 28(10):2458–63. doi: 10.1093/annonc/mdx405
48. Shaib WL, Zakka K, Staley C 3rd, Roberts A, Akce M, Wu C, et al. Blood-Based Next-Generation Sequencing Analysis of Appendiceal Cancers. Oncologist (2019) 25:414–21. doi: 10.1634/theoncologist.2019-0558
49. Fujii Y, Ono A, Hayes CN, Aikata H, Yamauchi M, Uchikawa S, et al. Identification and Monitoring of Mutations in Circulating Cell-Free Tumor DNA in Hepatocellular Carcinoma Treated With Lenvatinib. J Exp Clin Cancer Res (2021) 40(1):215. doi: 10.1186/s13046-021-02016-3
50. Mody K, Kasi PM, Yang JD, Surapaneni PK, Ritter A, Roberts A, et al. Feasibility of Circulating Tumor DNA Testing in Hepatocellular Carcinoma. J Gastrointest Oncol (2019) 10(4):745–50. doi: 10.21037/jgo.2019.02.10
51. Zheng Z, Liebers M, Zhelyazkova B, Cao Y, Panditi D, Lynch KD, et al. Anchored Multiplex PCR for Targeted Next-Generation Sequencing. Nat Med (2014) 20(12):1479–84. doi: 10.1038/nm.3729
52. Kriegsmann M, Arens N, Endris V, Weichert W, Kriegsmann J. Detection of KRAS, NRAS and BRAF by Mass Spectrometry - a Sensitive, Reliable, Fast and Cost-Effective Technique. Diagn Pathol (2015) 10:132. doi: 10.1186/s13000-015-0364-3
53. Giannini R, Lupi C, Sensi E, Ali G, Proietti A, Boldrini L, et al. EGFR and KRAS Mutational Analysis in a Large Series of Italian Non-Small Cell Lung Cancer Patients: 2,387 Cases From a Single Center. Oncol Rep (2016) 36(2):1166–72. doi: 10.3892/or.2016.4874
54. Kakavand H, Walker E, Lum T, Wilmott JS, Selinger CI, Smith E, et al. BRAF(V600E) and NRAS(Q61L/Q61R) Mutation Analysis in Metastatic Melanoma Using Immunohistochemistry: A Study of 754 Cases Highlighting Potential Pitfalls and Guidelines for Interpretation and Reporting. Histopathology (2016) 69(4):680–6. doi: 10.1111/his.12992
55. Robertson AG, Shih J, Yau C, Gibb EA, Oba J, Mungall KL, et al. Integrative Analysis Identifies Four Molecular and Clinical Subsets in Uveal Melanoma. Cancer Cell (2017) 32(2):204–220 e15. doi: 10.1016/j.ccell.2017.07.003
56. Hodis E, Watson IR, Kryukov GV, Arold ST, Imielinski M, Theurillat JP, et al. A Landscape of Driver Mutations in Melanoma. Cell (2012) 150(2):251–63. doi: 10.1016/j.cell.2012.06.024
57. Bailey MH, Tokheim C, Porta-Pardo E, Sengupta S, Bertrand D, Weerasinghe A, et al. Comprehensive Characterization of Cancer Driver Genes and Mutations. Cell (2018) 173(2):371–85.e18. doi: 10.1016/j.cell.2018.02.060
58. Hayward NK, Wilmott JS, Waddell N, Johansson PA, Field MA, Nones K, et al. Whole-Genome Landscapes of Major Melanoma Subtypes. Nature (2017) 545(7653):175–80. doi: 10.1038/nature22071
59. Heidenreich B, Nagore E, Rachakonda PS, Garcia-Casado Z, Requena C, Traves V, et al. Telomerase Reverse Transcriptase Promoter Mutations in Primary Cutaneous Melanoma. Nat Commun (2014) 5:3401. doi: 10.1038/ncomms4401
60. Karczewski KJ, Francioli LC, Tiao G, Cummings BB, Alfoldi J, Wang Q, et al. The Mutational Constraint Spectrum Quantified From Variation in 141,456 Humans. Nature (2020) 581(7809):434–43. doi: 10.1038/s41586-020-2308-7
61. Corless BC, Chang GA, Cooper S, Syeda MM, Shao Y, Osman I, et al. Development of Novel Mutation-Specific Droplet Digital PCR Assays Detecting TERT Promoter Mutations in Tumor and Plasma Samples. J Mol Diagn (2019) 21(2):274–85. doi: 10.1016/j.jmoldx.2018.09.003
62. Colebatch AJ, Witkowski T, Waring PM, McArthur GA, Wong SQ, Dobrovic A. Optimizing Amplification of the GC-Rich TERT Promoter Region Using 7-deaza-dGTP for Droplet Digital PCR Quantification of TERT Promoter Mutations. Clin Chem (2018) 64(4):745–7. doi: 10.1373/clinchem.2017.284257
63. Koopmans AE, Ober K, Dubbink HJ, Paridaens D, Naus NC, Belunek S, et al. Prevalence and Implications of TERT Promoter Mutation in Uveal and Conjunctival Melanoma and in Benign and Premalignant Conjunctival Melanocytic Lesions. Invest Ophthalmol Vis Sci (2014) 55(9):6024–30. doi: 10.1167/iovs.14-14901
64. Mouliere F, Chandrananda D, Piskorz AM, Moore EK, Morris J, Ahlborn LB, et al. Enhanced Detection of Circulating Tumor DNA by Fragment Size Analysis. Sci Transl Med (2018) 10(466). doi: 10.1126/scitranslmed.aat4921
65. Long GV, Wilmott JS, Capper D, Preusser M, Zhang YE, Thompson JF, et al. Immunohistochemistry is Highly Sensitive and Specific for the Detection of V600E BRAF Mutation in Melanoma. Am J Surg Pathol (2013) 37(1):61–5. doi: 10.1097/PAS.0b013e31826485c0
66. Raaijmakers MI, Widmer DS, Narechania A, Eichhoff O, Freiberger SN, Wenzina J, et al. Co-Existence of BRAF and NRAS Driver Mutations in the Same Melanoma Cells Results in Heterogeneity of Targeted Therapy Resistance. Oncotarget (2016) 7(47):77163–74. doi: 10.18632/oncotarget.12848
67. Long GV, Fung C, Menzies AM, Pupo GM, Carlino MS, Hyman J, et al. Increased MAPK Reactivation in Early Resistance to Dabrafenib/Trametinib Combination Therapy of BRAF-Mutant Metastatic Melanoma. Nat Commun (2014) 5:5694. doi: 10.1038/ncomms6694
68. Rizos H, Darmanian AP, Indsto JO, Shannon JA, Kefford RF, Mann GJ. Multiple Abnormalities of the P16ink4a-pRb Regulatory Pathway in Cultured Melanoma Cells. Melanoma Res (1999) 9(1):10–9. doi: 10.1097/00008390-199902000-00003
69. Heidorn SJ, Milagre C, Whittaker S, Nourry A, Niculescu-Duvas I, Dhomen N, et al. Kinase-Dead BRAF and Oncogenic RAS Cooperate to Drive Tumor Progression Through CRAF. Cell (2010) 140(2):209–21. doi: 10.1016/j.cell.2009.12.040
70. Zhao Y, Gao Y, Chen Z, Hu X, Zhou F, He J. Low Frequency of TERT Promoter Somatic Mutation in 313 Sporadic Esophageal Squamous Cell Carcinomas. Int J Cancer (2014) 134(2):493–4. doi: 10.1002/ijc.28360
71. Gay-Bellile M, Veronese L, Combes P, Eymard-Pierre E, Kwiatkowski F, Dauplat MM, et al. TERT Promoter Status and Gene Copy Number Gains: Effect on TERT Expression and Association With Prognosis in Breast Cancer. Oncotarget (2017) 8(44):77540–51. doi: 10.18632/oncotarget.20560
72. Vinothkumar V, Arun K, Arunkumar G, Revathidevi S, Ramani R, Bhaskar L, et al. Association Between Functional TERT Promoter Polymorphism Rs2853669 and Cervical Cancer Risk in South Indian Women. Mol Clin Oncol (2020) 12(5):485–94. doi: 10.3892/mco.2020.2003
73. Hanrahan AJ, Sylvester BE, Chang MT, Elzein A, Gao J, Han W, et al. Leveraging Systematic Functional Analysis to Benchmark an In Silico Framework Distinguishes Driver From Passenger MEK Mutants in Cancer. Cancer Res (2020) 80(19):4233–43. doi: 10.1158/0008-5472.CAN-20-0865
74. Bhagwat SV, McMillen WT, Cai S, Zhao B, Whitesell M, Shen W, et al. ERK Inhibitor LY3214996 Targets ERK Pathway-Driven Cancers: A Therapeutic Approach Toward Precision Medicine. Mol Cancer Ther (2020) 19(2):325–36. doi: 10.1158/1535-7163.MCT-19-0183
75. He Z, Li A, Lin D, Gu Y, Chen Y, Ou Q, et al. Association of Immune Checkpoint Inhibitor With Survival in Patients With Cancers With Protein Tyrosine Phosphatase Receptor T Mutation. Clin Transl Med (2020) 10(6):e214. doi: 10.1002/ctm2.214
76. Kakadia S, Yarlagadda N, Awad R, Kundranda M, Niu J, Naraev B, et al. Mechanisms of Resistance to BRAF and MEK Inhibitors and Clinical Update of US Food and Drug Administration-Approved Targeted Therapy in Advanced Melanoma. Onco Targets Ther (2018) 11:7095–107. doi: 10.2147/OTT.S182721
77. Carlino MS, Fung C, Shahheydari H, Todd JR, Boyd SC, Irvine M, et al. Preexisting MEK1P124 Mutations Diminish Response to BRAF Inhibitors in Metastatic Melanoma Patients. Clin Cancer Res (2015) 21(1):98–105. doi: 10.1158/1078-0432.CCR-14-0759
78. Fujino T, Kitamura T. ASXL1 Mutation in Clonal Hematopoiesis. Exp Hematol (2020) 83:74–84. doi: 10.1016/j.exphem.2020.01.002
79. Gandini S, Zanna I, De Angelis S, Palli D, Raimondi S, Ribero S, et al. TERT Promoter Mutations and Melanoma Survival: A Comprehensive Literature Review and Meta-Analysis. Crit Rev Oncol Hematol (2021) 160:103288. doi: 10.1016/j.critrevonc.2021.103288
80. Li J, Li Z, Zhang C, Zhang C, Wang H. Male Patients With TERT Mutation may be More Likely to Benefit From Immunotherapy, Especially for Melanoma. Aging (Albany NY) (2020) 12(17):17288–94. doi: 10.18632/aging.103684
81. Hsu CP, Hsu NY, Lee LW, Ko JL. Ets2 Binding Site Single Nucleotide Polymorphism at the hTERT Gene Promoter–Effect on Telomerase Expression and Telomere Length Maintenance in Non-Small Cell Lung Cancer. Eur J Cancer (2006) 42(10):1466–74. doi: 10.1016/j.ejca.2006.02.014
82. Park CK, Lee SH, Kim JY, Kim JE, Kim TM, Lee ST, et al. Expression Level of hTERT is Regulated by Somatic Mutation and Common Single Nucleotide Polymorphism at Promoter Region in Glioblastoma. Oncotarget (2014) 5(10):3399–407. doi: 10.18632/oncotarget.1975
83. Labussiere M, Di Stefano AL, Gleize V, Boisselier B, Giry M, Mangesius S, et al. TERT Promoter Mutations in Gliomas, Genetic Associations and Clinico-Pathological Correlations. Br J Cancer (2014) 111(10):2024–32. doi: 10.1038/bjc.2014.538
84. Griewank KG, Murali R, Schilling B, Scholz S, Sucker A, Song M, et al. TERT Promoter Mutations in Ocular Melanoma Distinguish Between Conjunctival and Uveal Tumours. Br J Cancer (2013) 109(2):497–501. doi: 10.1038/bjc.2013.312
85. Rachakonda PS, Hosen I, de Verdier PJ, Fallah M, Heidenreich B, Ryk C, et al. TERT Promoter Mutations in Bladder Cancer Affect Patient Survival and Disease Recurrence Through Modification by a Common Polymorphism. Proc Natl Acad Sci USA (2013) 110(43):17426–31. doi: 10.1073/pnas.1310522110
86. Ko E, Seo HW, Jung ES, Kim BH, Jung G. The TERT Promoter SNP Rs2853669 Decreases E2F1 Transcription Factor Binding and Increases Mortality and Recurrence Risks in Liver Cancer. Oncotarget (2016) 7(1):684–99. doi: 10.18632/oncotarget.6331
87. Hirokawa T, Arimasu Y, Chiba T, Fujiwara M, Kamma H. Clinicopathological Significance of the Single Nucleotide Polymorphism, Rs2853669 Within the TERT Promoter in Papillary Thyroid Carcinoma. Pathol Int (2020) 70(4):217–23. doi: 10.1111/pin.12900
88. Liu Z, Wang T, Wu Z, Zhang K, Li W, Yang J, et al. Association Between TERT Rs2853669 Polymorphism and Cancer Risk: A Meta-Analysis of 9,157 Cases and 11,073 Controls. PloS One (2018) 13(3):e0191560. doi: 10.1371/journal.pone.0191560
Keywords: anchored multiplex PCR, melanoma, circulating tumor DNA, targeted sequencing, custom panel, TERT promoter
Citation: Diefenbach RJ, Lee JH, Stewart A, Menzies AM, Carlino MS, Saw RPM, Stretch JR, Long GV, Scolyer RA and Rizos H (2022) Anchored Multiplex PCR Custom Melanoma Next Generation Sequencing Panel for Analysis of Circulating Tumor DNA. Front. Oncol. 12:820510. doi: 10.3389/fonc.2022.820510
Received: 23 November 2021; Accepted: 18 March 2022;
Published: 12 April 2022.
Edited by:
Reza Safaralizadeh, University of Tabriz, IranCopyright © 2022 Diefenbach, Lee, Stewart, Menzies, Carlino, Saw, Stretch, Long, Scolyer and Rizos. This is an open-access article distributed under the terms of the Creative Commons Attribution License (CC BY). The use, distribution or reproduction in other forums is permitted, provided the original author(s) and the copyright owner(s) are credited and that the original publication in this journal is cited, in accordance with accepted academic practice. No use, distribution or reproduction is permitted which does not comply with these terms.
*Correspondence: Helen Rizos, helen.rizos@mq.edu.au