- 1Division of Radiation Oncology, Department of Oncology, McGill University, Montreal, QC, Canada
- 2Department of Pathology, McGill University, Montreal, QC, Canada
- 3Research Institute of the McGill University Health Centre, Glen Site, Montreal, QC, Canada
- 4Department of Medicine, McGill University, Montreal, QC, Canada
Radiomics analysis has had remarkable progress along with advances in medical imaging, most notability in central nervous system malignancies. Radiomics refers to the extraction of a large number of quantitative features that describe the intensity, texture and geometrical characteristics attributed to the tumor radiographic data. These features have been used to build predictive models for diagnosis, prognosis, and therapeutic response. Such models are being combined with clinical, biological, genetics and proteomic features to enhance reproducibility. Broadly, the four steps necessary for radiomic analysis are: (1) image acquisition, (2) segmentation or labeling, (3) feature extraction, and (4) statistical analysis. Major methodological challenges remain prior to clinical implementation. Essential steps include: adoption of an optimized standard imaging process, establishing a common criterion for performing segmentation, fully automated extraction of radiomic features without redundancy, and robust statistical modeling validated in the prospective setting. This review walks through these steps in detail, as it pertains to high grade gliomas. The impact on precision medicine will be discussed, as well as the challenges facing clinical implementation of radiomic in the current management of glioblastoma.
Introduction
Glioblastoma (GBM) is the most common astrocytic primary brain malignancy, with an annual incidence of 2–3 cases per 100,000 adults in North America and Europe (1, 2). The standard of care for newly diagnosed GBM combines maximum safe resection followed by chemo-radiation and adjuvant courses of temozolomide (TMZ) (3). The median overall survival is poor at 14.6 months and 5-year survival rates are under 10% following standard of care treatment. If patients tolerate the chemoradiotherapy without progression, they may be considered for tumor-treatment fields. Even in this setting, the survival is still limited at a median of 20.9 months (4). Given these poor outcomes, there is hope that up-and-coming therapies will show benefit in the randomized setting (5, 6). It will be essential to ascertain which patients can benefit from these therapies, highlighting the need for efficacious tools to offer personalized medicine.
Magnetic resonance imaging (MRI) is the preferred imaging modality for both the diagnosis and monitoring of central nervous system (CNS) malignancies (7). It provides a massive amount of information to clinicians. Unfortunately, clinicians are typically restricted to qualitative descriptors or subjective quantitative assessments to articulate changes in imaging. The resulting clinical evaluations have a significant potential for bias.
Clinicians immensely value non-invasive approaches that can direct patients to the correct therapeutic approach in an objective fashion. This begins at diagnosis, where various molecular factors differentiate the diagnosis between low-grade glioma, high-grade glioma, or GBM (8). Such factors may also be predict the efficacy of a systemic agent (9, 10). This information requires tissue, introducing patient morbidity, an additional procedure, and a variety of expensive molecular assessments.
Radiomics has demonstrated remarkable progress in demonstrating that it may be a tool that can derive this information. Radiomics is a field of biomedical imaging using advanced non-invasive assessments of complex imaging characteristics within the MRI images that are too complex for a human to appreciate (11–14). These characteristics are known as features. Imaging features have been associated with a CNS tumor's histological features (14), progression (15) grade (16), or even overall survival (17–21). Radiomics analysis thus hosts a major role in producing novel non-invasive biomarkers acquired from a test—MRI—that is already routinely acquired from patients as part of the standard of care.
Radiomics Methodology
A standard pipeline of radiomic analysis has been described by several studies in the past (Figure 1) as mentioned previously by several studies (12, 13, 19, 21–23). This review discusses recent studies in the development of MRI-based radiomics analysis in relationship to this pipeline. For CNS malignancies, the literature discusses the most significant cause of diagnostic and management dilemmas—low and high-grade glioma. To facilitate an understanding of the process, there are sections on the: (1) preprocessing and image acquisition for developing a radiomic model; (2) segmentation/labeling of the cancer; (3) identification of relevant features types that may relate to the molecular properties of the tumor (14, 24) and (4) statistical modeling to describe a radiomic profile's relationship with a clinical outcomes. Given the number of variables at each step, collaboration is essential. Radiologists and oncologists must ensure that the appropriate regions are being assessed and the right questions are being asked. Molecular scientists must communicate the relevant genetic and proteomic characteristics that will influence a patient's clinical course. Engineering teams must determine what information can be reliably extracted from the images and then adapt the machine learning to fashion a reliable model. Consultation with statisticians will allow for a methodological approach allows for a potentially statistically significant solution.
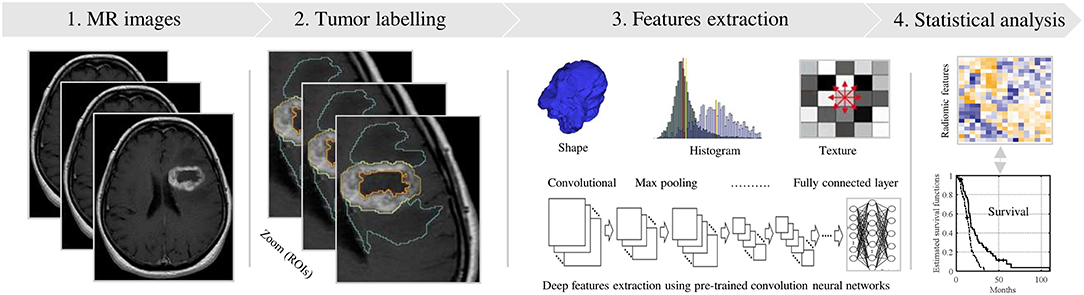
Figure 1. Standard pipeline of the radiomics analysis. (1) MR Image acquisition with a standardization. (2) Tumor labeling viewing in 3D (e.g., red, yellow and cyan contours). (3) Radiomic features extraction using shape, texture and convolution neural network techniques. (4) Statistical analyses, based significance test and classifier models, to identify relevant features for predicting the clinical outcome.
Image Acquisition
MRI radiomics has repeatedly shown the ability to differentiate low and high-grade glioma, which have different management strategies (https://www.nccn.org/) and a remarkably different prognosis (25–28). One reason this data can be more rapidly generated is that there is a wealth of clinical information available—glioma patients have regular MRIs throughout their lives. However, reproducibility is a significant issue at different stages of the radiomics pipeline. The issues begin at image acquisition. Different academic groups acquire their MRI images to different settings at the first step of the pipeline. This is one reason that radiomic analysis collaboration has been limited between research groups. Standardization offers a rational solution to overcome this barrier.
Standardization
Potential variations in images are often secondary to the MRI scanner model, including image resolution (i.e., pixel size and slice spacing), image contrast, slice thickness, patient position, and further variations introduced by different reconstruction algorithms. When generating or applying a radiomics model, standardization must occur so the data can be assumed that it was extracted from similar settings. To accomplish this, volume datasets are usually re-sampled to a common voxel resolution of 1 mm3 and an image size of 2563 (or 5123) voxels.
A common further step is normalizing the intensities within each volume image to the [0,1] or [0,255] range. Less commonly adopted normalization approaches have included gaussian and Z-score normalization. For example in Ellingson et al. (29), Gaussian normalization was the best normalization technique for image intensity correction. The need for standardization would be reduced if radiomic analysis could be performed with data acquired at a single geographical site. However, a single site would only provide a limited dataset. Thus, several studies have augmented their datasets through the use of multiple sites and an imputation technique to facilitate standardization (30).
The lack of standardization is a recognized problem. The Quantitative Imaging Biomarker Alliance offers an expert consensus after reviewing the available data. This group offers insightful guidelines for standardization that should be heavily considered in present and future studies. Such guidelines will be dynamic. Radiomic features may change from site to site or have new ways to be extracted or MRI image acquisition may change. Standardization in either of these contexts will a challenge in the future. Ongoing communication between institutions and robust reporting of new methodological approaches will be essential to groups studying radiomics.
Segmentation of Brain Tumors
Accurate labeling of brain tumors in the images is required for radiomic analysis. It first involves defining the tumor volume, known as the region of interest (ROI), so it can then have its radiomic features extracted. The act of employing clinical, pathological and imaging features to mark out the ROI on the two-dimensional MRI images is called either the segmentation or labeling process. Segmentation is performed by clinicians—typically a radiologist or oncologist. The process is subject to inter-rater variability, as the ROI definition will inevitably differ between clinicians. An approach to overcome this variation is different clinicians each generating their own ROI. The geographical regions common between the different ROIs is considered the true tumor mask. This tumor mask is then matched with the corresponding brain images to then extract the imaging features (i.e., radiomic features).
Since MRI generates several image sequences, the registration step involves matching the mask to the relevant MR series (ex. T1 weighted, T1-post contrast, T2 weighted, FLAIR), a well-described process (20, 31). Many tools used to delineate the ROI, such as the publicly accessible 3D Slicer (32), require slice by slice labeling on each series to ensure accuracy and precision (21). For efficiency and to minimize both inter- and intra-user variability, several studies have explored segmentation to all relevant MRI sequences without registration across the sequences (33). Registration distortions between MRI series may limit this approach (34). Distortion could cause incorrect localization of the ROI, directing the radiomic analyses to the incorrect MRI-defined anatomy. More investigation is required to allow for the trans-sequence application of user-generated segmentation data.
To overcome user variability in registration, (semi-)automated segmentation has been explored in various studies (35–38). Strong signals for a successful model, a promising Dice Similarity Coefficient (DSC) of 80%, have been reported with fully automated segmentation based on an adaptive algorithm with multi-level of thresholding (38). When deep learning radiomics (DLR) was applied to multiple tumor regions, the ability to label the tumor subregions achieved a DSC of 90% (35). DLR has become a success story for machine learning integral to limiting user variability. The use of DLR's convolutional neural networks (CNNs) to the various steps of the radiomics pipeline is elegantly described elsewhere (39). As to fully automated segmentation, further validation is required. Success here could enable the rapid integration of radiomics into personalized medicine.
Radiomic Features Extraction
Extracting radiomic features is the first step in analyzing the segmented image. The features themselves are measures of the heterogeneity within the ROI (40). The degree to which these different features are present is a radiomics feature cluster, perhaps better conceptualized as an ROI's radiomic signature. There are different types of features, the most common and presently relevant are outlined in the Table 1.
Feature-Analyses
Once the features have been extracted, statistical modeling can highlight relationships between the extent a given feature is present and a clinical characteristic. There are various methodologies to analyze this, including minimizing the number of features likely to contribute to the statistical analysis. Feature selection methods (60) or reducing dimensionality in another fashion can accomplish this minimization. This has included sorting features by their minimum redundancy maximum relevance, mutual information, principal component analysis feature rank or the importance of features in other classifier models (31, 61–65). Once the features that are potentially relevant for analysis are determined, they are typically subject to assessments of their significance (e.g., Wilcoxon test, Kruskal-Wallis, log-rank, etc.) and correlation (e.g., Spearman rank, Pearson). These forms of univariate analysis determine if a feature is a significant predictor for the selected clinical outcome, with significance typically being defined as either a p < 0.01 or 0.05. The p-values should be corrected by the Bonferroni or Holm-Bonferroni procedure to limit the influence of random chance, including the false discovery rate (21, 60, 66).
Multivariate Analysis and Model Building
Multivariate analysis fills an essential role in separating seemingly relevant features on univariate analysis from those that are likely independent predictors for the clinical outcome being assessed for. This is a critical step, limiting non-contributory features from influencing our eventual final statistical model (67). Once these features are selected from multi-variate analysis, the radiomics team must determine how many of their finite number of clinical cases will be used to produce/train their model and how many need to be reserved to validate the model.
Increasing the size of the training cohort will increase the model's accuracy. Thus, typically 70–80% of the dataset is used for the training stage. Alternatively, if an external dataset is available, then all the datasets can train the model. This is the preferred scenario, allowing for a demonstration of external validity. If the datasets are limited in size, k-folds cross-validation can mitigate some of the statistical concerns (31, 68, 69).
Machine learning changes the available options. If unsupervised, the program can utilize different methods (e.g., k-means, nearest neighbors) to partition the features into different groups, then compare the relationships of the different features within their group—not the clinical data. After this is completed, the ability of the different groups to predict the clinical outcome is assessed, even though the clinical data did not contribution to the model's development (70). In comparison, supervised machine learning techniques (e.g., support vector machine, Bayes model, neural network nearest neighbors, random forests) will place varying numbers of the pre-determined relevant features into groups. Then their relative contribution to the model's ability to predict for the clinical outcome is altered until the most reliable combination of weightings is determined. Random forest classifier is a simple model that automatically selects the relevant features. Furthermore, random forest has shown the great ability to predict for survival (71) and endure an imputation technique to account for censored patients (31). Alternatively, the least absolute shrinkage and selection operator (LASSO) Cox regression model has also been reported reliably predict for survival in glioma (72, 73).
A third option is semi-supervised machine learning, wherein some complete clinical data is provided to the program generating the model, but other data is complete. For example, the program would have a range of radiomic features that it knows correspond to high grade glioma and a range of radiomic features that belong to an unknown clinical entity. Thus, all the dataset is used for a training step. The validation step is then a question if the program can correctly identify the unlabeled data. This process has been used to suggest brain tumor histology and prognosis (74).
Progress of Radiomics in GBM
Radiomics has provided key insight into critical features of GBM, as advanced radiomic analysis seek to establish reliable associations between key clinical features and those features derived from images. For example, radiomics has been used to predict for clinical, proteomic (e.g., Ki-67 expression), genomic (e.g., IDH1 status) and transcriptomic characteristics (75–77). This evolution of the radiomics field has been titled multi-omics or radiogenomics, dependent on the source (21, 78–80). This will be part of the future of radiomics, as these details are pertinent to physicians due to their influence on treatment and prognosis (8). In addition, recent advancements have been made in defining radiomic subtypes. By utilizing T1 and FLAIR sequencing, researchers were able to define three distinct imaging subtypes—rim enhancing, irregular and solid. Each subtype represents a distinct phenotype enriched in unique molecular alterations such as MGMT methylation and EGFRvIII mutations (37). Continued advancements in defining tumor heterogeneity using imaging features may offer a complimentary means with which to characterize GBM and provide personalized treatments for patients.
Radiomics analysis has the capacity to answer critical questions facing clinicians such as the discrimination between pseudoprogression and progressive disease in GBM patients. For example, combining the diffusion tensor imaging and dynamic susceptibility contrast MRI features can improve accuracy treatment response and may aid in individualized treatment of patients with GBM (81). Recently, a deep radiomics model used the MR images with clinical features demonstrate the capacity to predict the PsP from progression for patients with GBM (82). While, another study showed that the radiomics analysis is not able to distinguish between true-progression and PsP (83). However, many of these steps exist in an early developmental stage. Combining all such information into an artificial intelligence model would be a promising direction to advance personalized medicine.
Intratumoural Heterogeneity and Radiogenomics
Perhaps the greatest utility of radiomics in the management of gliomas lies in the application of radiogenomics. Radiogenomics implements radiomics analysis to predict specific genetic characteristics. Classically, gliomas have been managed based on their grade—a histopathological characterization made by specialized physicians (neuropathologists) to articulate the likely behavior of the malignancy. Over the past two decades, molecular assessment of the tumor's genome, protein expression, and epigenetic state have become more common as the relevance of these features to outcome and/or therapeutic response is being increasingly understood (84). Given the relative abundance of high quality MRI data which accumulates over time during standard of care for glioma patients (85–87) radiomics offers a potentially efficient and non-invasive method of tumoral evaluation (37, 88, 89). Indeed, recent efforts have generated radiomic signatures to predict the majority of information sought by classical histopathological and modern molecular assessments including: isocitrate dehydrogenase mutations (79, 90–92), 1p/19q codeletion loss of heterozygosity (24, 92, 93), O6-methylguanine-DNA methyltransferase promoter methylation (45, 94) and ATRX mutations (95). This has culminated in recent findings demonstrating a conserved radiomic signature can predict CD8+ T-cell infiltration and response to immunotherapy (96).
However, intratumoral heterogeneity significantly confounds both molecular and histopathological assessments as the entirety of a tumor cannot be assessed by neuropathologists. Disparate clonal populations may be minimally represented in histopathological sampling introducing sampling errors and limiting relevance for informing treatments (97–101). Radiomics offers an opportunity to overcome this limitation as analysis is performed upon the complete tumor enabling spatial mapping of distinct genetic features. In addition, radiomics offers the means to provide quantitative values (e.g., % of tumor mutated) rather than binary designations (e.g., mutant or not) to describe molecular features which may have important implications for predicting response to therapies. Utilizing co-clinical models, researchers are starting to establish radiomic signatures which are closely associated with specific molecular features in an attempt to describe intratumoral heterogeneity (102). Further development of pre-clinical models and correlation with clinical datasets will be essential to drive this field forward toward improving the utility of radiomics for diagnosis in GBM.
Future Radiomics
Radiomics needs massive amounts of biomedical data, so-called “Big data (103),” to validate it's deep-learning approaches and expanding applications. The development of strong public datasets has empowered these approaches, with such initiatives including The Cancer Genome Atlas (TCGA) (85), The Cancer Imaging Archive (86), and The Quantitative Imaging Network (87). However, there is still the barrier of segmentation—such as acquiring clinician input to identify the relevant ROIs. While the clinician will still be sought as the gold standard, deep-learning strategies have the potential to define ROIs without the bias of human segmentation (104). To accomplish this, even larger datasets will be required—further emphasizing the need for reliable Big Data. These strategies have begun in part, but developing validated models to all the clinically relevant questions will simply require more data (105, 106).
The potential applications for radiomics is expanding, with logistical and technical challenges needing to be overcome prior to true clinical deployment. We view these as: (1) expanding what is included in and the access to Big Data, (2) establishing common criteria from image acquisition to feature definitions, (3) agreement on the clinical questions that radiomics must address, and (4) developing a clinically implementable and prospectively validated statistical model to answer those questions.
Conclusions
This review explained how the vast amount of radiological data not used by the clinicians managing CNS malignancies can be used to generate radiological signatures that can predict the characteristics of these brain tumours. In a step-by-step process we outlined how this data can be used to predict for numerous pertinent biological outcomes. With constant progress in deep-learning processes and expanding public access to Big Data, radiomics has the potential to non-invasively address numerous clinical questions or support clinical decision making. There are numerous future directions for radiomics, but a continued focus on ensuring there is public access to large databases of clinical and radiological correlated data will be instrumental to seeing those directions leading to a desirable destination.
Author Contributions
AC conception and design. AC, PD, and MK drafting the manuscript and review of the literature. MK, PD, BJ-C, TN, SS, and BA critical revision of the manuscript.
Conflict of Interest Statement
Author PD's fellowship was partly funded by a grant from Varian Medical System. The funder had no role in study design, data collection and analysis, or preparation of the manuscript.
The remaining authors declare that the research was conducted in the absence of any commercial or financial relationships that could be construed as a potential conflict of interest.
Acknowledgments
Research supported by grant from Varian Medical System.
References
1. Koca T, Basaran H, Sezen D, Karaca S, Ors Y, Arslan D, Aydin A. Comparison of linear accelerator and helical tomotherapy plans for glioblastoma multiforme patients. Asian Pac J Cancer Prev. (2014) 15:7811–6. doi: 10.7314/APJCP.2014.15.18.7811
2. Ostrom QT, Gittleman H, Truitt G, Boscia A, Kruchko C, Barnholtz-Sloan JS. CBTRUS statistical report: primary brain and other central nervous system tumors diagnosed in the United States in 2011–2015. Neuro Oncol. (2018) 20:iv1–86. doi: 10.1093/neuonc/noy131
3. Stupp R, Hegi ME, Mason WP, van den Bent MJ, Taphoorn MJB, Janzer RC, et al. Effects of radiotherapy with concomitant and adjuvant temozolomide versus radiotherapy alone on survival in glioblastoma in a randomised phase III study: 5-year analysis of the EORTC-NCIC trial. Lancet Oncol. (2009) 10:459–66. doi: 10.1016/S1470-2045(09)70025-7
4. Stupp R, Taillibert S, Kanner A, Read W, Steinberg D, Lhermitte B, et al. Effect of tumor-treating fields plus maintenance temozolomide vs maintenance temozolomide alone on survival in patients with glioblastoma: a randomized clinical trial. JAMA. (2017) 318:2306–16. doi: 10.1001/jama.2017.18718
5. Kong X-T, Nguyen NT, Choi YJ, Zhang G, Nguyen HN, Filka E, et al. Phase 2 Study of bortezomib combined with temozolomide and regional radiation therapy for upfront treatment of patients with newly diagnosed glioblastoma multiforme: safety and efficacy assessment. Int J Radiation Oncol Biol Phys. (2018) 100:1195–203. doi: 10.1016/j.ijrobp.2018.01.001
6. Desjardins A, Gromeier M, Herndon JE, Beaubier N, Bolognesi DP, Friedman AH, et al. Recurrent glioblastoma treated with recombinant poliovirus. N Engl J Med. (2018) 379:150–61. doi: 10.1056/NEJMoa1716435
7. Cao Y, Tseng C-L, Balter JM, Teng F, Parmar HA, Sahgal A. MR-guided radiation therapy: transformative technology and its role in the central nervous system. Neuro Oncol. (2017) 19:ii16–29. doi: 10.1093/neuonc/nox006
8. Louis DN, Perry A, Reifenberger G, Deimling A von, Figarella-Branger D, Cavenee WK, et al. The 2016 World Health Organization classification of tumors of the central nervous system: a summary. Acta Neuropathol. (2016) 131:803–20. doi: 10.1007/s00401-016-1545-1
9. Kaley T, Touat M, Subbiah V, Hollebecque A, Rodon J, Lockhart AC, et al. BRAF Inhibition in BRAFV600-mutant gliomas: results from the VE-BASKET study. JCO. (2018) JCO.2018.78.9990. doi: 10.1200/JCO.2018.78.9990
10. Alexander BM, Trippa L, Gaffey SC, Arrillaga I, Lee EQ, Tanguturi SK, et al. Individualized screening trial of innovative glioblastoma therapy (INSIGhT). Am Soc Clin Oncol. (2017). doi: 10.1200/JCO.2017.35.15_suppl.TPS2079
11. Aerts HJWL. The potential of radiomic-based phenotyping in precision medicine: a review. JAMA Oncol. (2016) 2:1636. doi: 10.1001/jamaoncol.2016.2631
12. Aerts HJ, Velazquez ER, Leijenaar RT, Parmar C, Grossmann P, Cavalho S, et al. Decoding tumour phenotype by noninvasive imaging using a quantitative radiomics approach. Nat Commun. (2014) 5:5644. doi: 10.1038/ncomms5644
13. Chaddad A, Daniel P, Niazi T. Radiomics evaluation of histological heterogeneity using multiscale textures derived from 3D wavelet transformation of multispectral images. Front Oncol. (2018) 8:96. doi: 10.3389/fonc.2018.00096
14. Chaddad A, Desrosiers C, Toews M. GBM heterogeneity characterization by radiomic analysis of phenotype anatomical planes. In: Martin AS, Elsa DA, editors. Medical Imaging 2016 Image Processing. Orlando, FL: International Society for Optics and Photonics. p. 978424.
15. Abrol S, Kotrotsou A, Hassan A, Elshafeey N, Hassan I, Idris T, et al. Radiomic analysis of pseudo-progression compared to true progression in glioblastoma patients: a large-scale multi-institutional study. Am Soc Clin Oncol. (2017). doi: 10.1200/JCO.2017.35.15_suppl.2015
16. Zacharaki EI, Wang S, Chawla S, Soo Yoo D, Wolf R, Melhem ER, et al. Classification of brain tumor type and grade using MRI texture and shape in a machine learning scheme. Magn Reson Med. (2009) 62:1609–18. doi: 10.1002/mrm.22147
17. Grabowski MM, Recinos PF, Nowacki AS, Schroeder JL, Angelov L, Barnett GH, et al. Residual tumor volume versus extent of resection: predictors of survival after surgery for glioblastoma. J Neurosurg. (2014) 121:1115–23. doi: 10.3171/2014.7.JNS132449
18. Cui Y, Ren S, Tha KK, Wu J, Shirato H, Li R. Volume of high-risk intratumoral subregions at multi-parametric MR imaging predicts overall survival and complements molecular analysis of glioblastoma. Eur Radiol. (2017) 27:3583–92. doi: 10.1007/s00330-017-4751-x
19. Chaddad A, Desrosiers C, Hassan L, Tanougast C. A quantitative study of shape descriptors from glioblastoma multiforme phenotypes for predicting survival outcome. Br J Radiol. (2016) 89: 20160575. doi: 10.1259/bjr.20160575
20. Chaddad A, Tanougast C. Extracted magnetic resonance texture features discriminate between phenotypes and are associated with overall survival in glioblastoma multiforme patients. Med Biol Eng Comput. (2016) 54:1707–18. doi: 10.1007/s11517-016-1461-5
21. Chaddad A, Daniel P, Desrosiers C, Toews M, Abdulkarim B. Novel radiomic features based on joint intensity matrices for predicting glioblastoma patient survival time. IEEE J Biomed Health Informat. (2019) 23:795–804. doi: 10.1109/JBHI.2018.2825027
22. Kumar V, Gu Y, Basu S, Berglund A, Eschrich SA, Schabath MB, et al. Radiomics: the process and the challenges. Magn Reson Imaging. (2012) 30:1234–48. doi: 10.1016/j.mri.2012.06.010
23. Lambin P, Leijenaar RTH, Deist TM, Peerlings J, de Jong EEC, van Timmeren J, et al. Radiomics: the bridge between medical imaging and personalized medicine. Nat Rev Clin Oncol. (2017) 14:749–62. doi: 10.1038/nrclinonc.2017.141
24. Lu C-F, Hsu F-T, Hsieh KL-C, Kao Y-CJ, Cheng S-J, Hsu JB-K, et al. Machine learning-based radiomics for molecular subtyping of gliomas. Clin Cancer Res. (2018). 24:4429–36. doi: 10.1158/1078-0432.CCR-17-3445
25. Qin J, Liu Z, Zhang H, Shen C, Wang X, Tan Y, et al. Grading of gliomas by using radiomic features on multiple magnetic resonance imaging (MRI) sequences. Med Sci Monit. (2017) 23:2168–78. doi: 10.12659/MSM.901270
26. Zhang X, Yan L-F, Hu Y-C, Li G, Yang Y, Han Y, et al. Optimizing a machine learning based glioma grading system using multi-parametric MRI histogram and texture features. Oncotarget. (2017) 8:47816–30. doi: 10.18632/oncotarget.18001
27. Chang K, Bai HX, Zhou H, Su C, Bi WL, Agbodza E, et al. Residual convolutional neural network for the determination of IDH status in low-and high-grade gliomas from MR imaging. Clin Cancer Res. (2018) 24:1073–81. doi: 10.1158/1078-0432.CCR-17-2236
28. Cho H, Park H. Classification of low-grade and high-grade glioma using multi-modal image radiomics features. In: Engineering in Medicine and Biology Society (EMBC), 2017 39th Annual International Conference of the IEEE (Jeju Island: IEEE), 3081–4.
29. Ellingson BM, Zaw T, Cloughesy TF, Naeini KM, Lalezari S, Mong S, et al. Comparison between intensity normalization techniques for dynamic susceptibility contrast (DSC)-MRI estimates of cerebral blood volume (CBV) in human gliomas. J Magn Reson Imag. (2012) 35:1472–7. doi: 10.1002/jmri.23600
30. Newman DA. Longitudinal modeling with randomly and systematically missing data: a simulation of ad hoc, maximum likelihood, and multiple imputation techniques, Longitudinal Modeling with Randomly and Systematically Missing Data: a simulation of ad hoc, maximum likelihood, and multiple imputation techniques. Organ Res Methods. (2003) 6:328–362. doi: 10.1177/1094428103254673
31. Chaddad A, Sabri S, Niazi T, Abdulkarim B. Prediction of survival with multi-scale radiomic analysis in glioblastoma patients. Med Biol Eng Comput. (2018) 1–14. doi: 10.1007/s11517-018-1858-4
32. 3D Slicer. Available online at: http://www.slicer.org/ (accessed October 20, 2014).
33. Zhou H, Vallières M, Bai HX, Su C, Tang H, Oldridge D, et al. MRI features predict survival and molecular markers in diffuse lower-grade gliomas. Neuro-oncology. (2017) 19:862–70. doi: 10.1093/neuonc/now256
34. Lin JS, Fuentes DT, Chandler A, Prabhu SS, Weinberg JS, Baladandayuthapani V, et al. Performance assessment for brain MR imaging registration methods. AJNR Am J Neuroradiol. (2017) 38:973–80. doi: 10.3174/ajnr.A5122
35. Kamnitsas K, Ledig C, Newcombe VFJ, Simpson JP, Kane AD, Menon DK, et al. Efficient multi-scale 3D CNN with fully connected CRF for accurate brain lesion segmentation. Med Image Anal. (2017) 36:61–78. doi: 10.1016/j.media.2016.10.004
36. Bakas S, Zeng K, Sotiras A, Rathore S, Akbari H, Gaonkar B, et al. GLISTRboost: combining multimodal MRI segmentation, registration, and biophysical tumor growth modeling with gradient boosting machines for glioma segmentation. In: Brainlesion: Glioma, Multiple Sclerosis, Stroke and Traumatic Brain Injuries Lecture Notes in Computer Science. (Cham: Springer), 144–155.
37. Rathore S, Akbari H, Rozycki M, Abdullah KG, Nasrallah MP, Binder ZA, et al. Radiomic MRI signature reveals three distinct subtypes of glioblastoma with different clinical and molecular characteristics, offering prognostic value beyond IDH1. Sci Rep. (2018) 8:5087. doi: 10.1038/s41598-018-22739-2
38. Chaddad A, Tanougast C. Quantitative evaluation of robust skull stripping and tumor detection applied to axial MR images. Brain Inform. (2016) 3:53–61. doi: 10.1007/s40708-016-0033-7
39. Krizhevsky A, Sutskever I, Hinton GE. Imagenet classification with deep convolutional neural networks. In: Pereira F, Burges CJC, Bottou L, Weinberger KQ, editors. Advances in Neural Information Processing Systems (Lake Tahoe, NV: Curran Associates Inc.), 1097–1105.
40. McGarry SD, Hurrell SL, Kaczmarowski AL, Cochran EJ, Connelly J, Rand SD, et al. Magnetic resonance imaging-based radiomic profiles predict patient prognosis in newly diagnosed glioblastoma before therapy. Tomography. (2016) 2:223. doi: 10.18383/j.tom.2016.00250
41. Chaddad A, Tanougast C. High-throughput quantification of phenotype heterogeneity using statistical features. Adv Bioinform. (2015) 2015:e728164. doi: 10.1155/2015/728164
42. Aerts HJWL, Velazquez ER, Leijenaar RTH, Parmar C, Grossmann P, Carvalho S, et al. Decoding tumour phenotype by noninvasive imaging using a quantitative radiomics approach. Nat Commun. (2014) 5:4006. doi: 10.1038/ncomms5006
43. Downey K, Riches SF, Morgan VA, Giles SL, Attygalle AD, Ind TE, et al. Relationship between imaging biomarkers of stage I cervical cancer and poor-prognosis histologic features: quantitative histogram analysis of diffusion-weighted MR images. AJR Am J Roentgenol. (2013) 200:314–20. doi: 10.2214/AJR.12.9545
44. Rosenkrantz AB. Histogram-based apparent diffusion coefficient analysis: an emerging tool for cervical cancer characterization? AJR Am J Roentgenol. (2013) 200:311–3. doi: 10.2214/AJR.12.9926
45. Li Z-C, Bai H, Sun Q, Li Q, Liu L, Zou Y, et al. Multiregional radiomics features from multiparametric MRI for prediction of MGMT methylation status in glioblastoma multiforme: a multicentre study. Eur Radiol. (2018) 28:3640–50. doi: 10.1007/s00330-017-5302-1
46. Chen Y, Li Z, Wu G, Yu J, Wang Y, Lv X, et al. Primary central nervous system lymphoma and glioblastoma differentiation based on conventional magnetic resonance imaging by high-throughput SIFT features. Int J Neurosci. (2018) 128:608–18. doi: 10.1080/00207454.2017.1408613
47. Yang D, Rao G, Martinez J, Veeraraghavan A, Rao A. Evaluation of tumor-derived MRI-texture features for discrimination of molecular subtypes and prediction of 12-month survival status in glioblastoma. Med Phys. (2015) 42:6725–35. doi: 10.1118/1.4934373
48. Haralick RM, Shanmugam K, Dinstein I. Textural features for image classification. IEEE Trans Syst Man Cyber. (1973) SMC-3:610–21. doi: 10.1109/TSMC.1973.4309314
49. Amadasun M, King R. Textural features corresponding to textural properties. IEEE Trans Syst Man Cyber. (1989) 19:1264–74. doi: 10.1109/21.44046
50. Sun C, Wee WG. Neighboring gray level dependence matrix for texture classification. Comp Vision Graphics Image Process. (1983) 23:341–52. doi: 10.1016/0734-189X(83)90032-4
51. Berry JR, Goutsias J. A comparative study of matrix measures for maximum likelihood texture classification. IEEE Trans Syst Man Cyber. (1991) 21:252–61. doi: 10.1109/21.101156
52. Chu A, Sehgal CM, Greenleaf JF. Use of gray value distribution of run lengths for texture analysis. Pattern Recogn Lett. (1990) 11:415–9. doi: 10.1016/0167-8655(90)90112-F
53. Gonzalez RC, Woods RE, Eddins SL. Digital Image Processing Using MATLAB. Pearson Education India (2004).
54. Skogen K, Schulz A, Helseth E, Ganeshan B, Dormagen JB, Server A. Texture analysis on diffusion tensor imaging: discriminating glioblastoma from single brain metastasis. Acta Radiol. (2018) 60:356–66. doi: 10.1177/0284185118780889
55. Chaddad A, Bouridane A, Hassan L, Tanougast C. Wavelet based radiomics for brain tumour phenotypes discrimination. In Proceedings—CIE 45: 2015 International Conference on Computers and Industrial Engineering (Metz: NY Curran Associates Inc.), 1167–74. Available online at: http://nrl.northumbria.ac.uk/26740/ (accessed October 24, 2017).
56. Litjens G, Kooi T, Bejnordi BE, Setio AAA, Ciompi F, Ghafoorian M, van der Laak JAWM, van Ginneken B, Sánchez CI. A survey on deep learning in medical image analysis. Medical Image Analysis. (2017) 42:60–88. doi: 10.1016/j.media.2017.07.005
57. Chaddad A, Desrosiers C, Niazi T. Deep radiomic analysis of MRI related to Alzheimer's disease. IEEE Access. (2018) 6:58213–21. doi: 10.1109/ACCESS.2018.2871977.
58. Chaddad A, Desrosiers C, Toews M. Phenotypic characterization of glioblastoma identified through shape descriptors. In: Proceedings Volume 9785, Medical Imaging 2016: Computer-Aided Diagnosis. San Diego, CA: International Society for Optics and Photonics (2016).
59. Czarnek N, Clark K, Peters KB, Mazurowski MA. Algorithmic three-dimensional analysis of tumor shape in MRI improves prognosis of survival in glioblastoma: a multi-institutional study. J Neurooncol. (2017) 132:55–62. doi: 10.1007/s11060-016-2359-7
60. Yu L, Liu H. Feature selection for high-dimensional data: a fast correlation-based filter solution. In: T. Fawcett, and N. Mishra (Eds.), Proceedings, Twentieth International Conference on Machine Learning. Vol. 2 (2003), pp. 856–863.
61. Xing EP, Jordan MI, Karp RM. Feature selection for high-dimensional genomic microarray data. In: ICML (Citeseer), 601–608.
62. Nguyen HT, Franke K, Petrovic S. Towards a generic feature-selection measure for intrusion detection. In: Pattern Recognition (ICPR), 2010 20th International Conference on (Istanbul: IEEE), 1529–1532.
63. Ramírez-Gallego S, Lastra I, Martínez-Rego D, Bolón-Canedo V, Benítez JM, Herrera F, et al. Fast-mRMR: fast minimum redundancy maximum relevance algorithm for high-dimensional big data. Int J Intelligent Syst. 32:134–52. doi: 10.1002/int.21833
64. Chaddad A. Automated feature extraction in brain tumor by magnetic resonance imaging using gaussian mixture models. Int J Biomed Imag. (2015) 11:868031. doi: 10.1155/2015/868031
65. Song F, Guo Z, Mei D. Feature selection using principal component analysis. In: Engineering Design and Manufacturing Informatization 2010 International Conference on System Science (Yichang).p. 27–30.
67. Witten IH, Frank E. Data Mining: Practical Machine Learning Tools and Techniques, Second Edition. San Francisco, CA: Morgan Kaufmann (2005).
68. Arlot S, Celisse A. A survey of cross-validation procedures for model selection. Statist Surv. (2010) 4:40–79. doi: 10.1214/09-SS054
69. Krstajic D, Buturovic LJ, Leahy DE, Thomas S. Cross-validation pitfalls when selecting and assessing regression and classification models. J Cheminform. (2014) 6:10. doi: 10.1186/1758-2946-6-10
70. Guan X, Vengoechea J, Zheng S, Sloan AE, Chen Y, Brat DJ, et al. Molecular subtypes of glioblastoma are relevant to lower grade glioma. PLoS ONE. (2014) 9:e91216. doi: 10.1371/journal.pone.0091216
71. Parmar C, Grossmann P, Bussink J, Lambin P, Aerts HJWL. Machine learning methods for quantitative radiomic biomarkers. Sci Rep. (2015) 5:13087. doi: 10.1038/srep13087
72. Lao J, Chen Y, Li Z-C, Li Q, Zhang J, Liu J, et al. A deep learning-based radiomics model for prediction of survival in glioblastoma multiforme. Sci Rep. (2017) 7:10353. doi: 10.1038/s41598-017-10649-8
73. Kim H, Bredel M. Feature selection and survival modeling in The Cancer Genome Atlas. Int J Nanomed. (2013) 8:57–62. doi: 10.2147/IJN.S40733
74. Cruz-Barbosa R, Vellido A. Semi-supervised analysis of human brain tumours from partially labeled MRS information, using manifold learning models. Int J Neural Syst. (2011) 21:17–29. doi: 10.1142/S0129065711002626
75. Kong D-S, Kim J, Ryu G, You H-J, Sung JK, Han YH, et al. Quantitative radiomic profiling of glioblastoma represents transcriptomic expression. Oncotarget. (2018) 9:6336–45. doi: 10.18632/oncotarget.23975
76. Kickingereder P, Burth S, Wick A, Götz M, Eidel O, Schlemmer H-P, et al. Radiomic profiling of glioblastoma: identifying an imaging predictor of patient survival with improved performance over established clinical and radiologic risk models. Radiology. (2016) 280:880–9. doi: 10.1148/radiol.2016160845
77. Zhang B, Tian J, Dong D, Gu D, Dong Y, Zhang L, et al. Radiomics features of multiparametric MRI as novel prognostic factors in advanced nasopharyngeal carcinoma. Clin Cancer Res. (2017) 23:4259–69. doi: 10.1158/1078-0432.CCR-16-2910
78. Beig N, Patel J, Prasanna P, Partovi S, Varadhan V, Madabhushi A, et al. Radiogenomic analysis of hypoxia pathway reveals computerized MRI descriptors predictive of overall survival in Glioblastoma. In: SPIE Medical Imaging (International Society for Optics and Photonics), p. 101341U
79. Mazurowski MA, Clark K, Czarnek NM, Shamsesfandabadi P, Peters KB, et al. Radiogenomics of lower-grade glioma: algorithmically-assessed tumor shape is associated with tumor genomic subtypes and patient outcomes in a multi-institutional study with The Cancer Genome Atlas data. J Neuro-Oncol. (2017) 133:27–35. doi: 10.1007/s11060-017-2420-1
80. Kickingereder P, Bonekamp D, Nowosielski M, Kratz A, Sill M, Burth S, et al. Radiogenomics of glioblastoma: machine learning–based classification of molecular characteristics by using multiparametric and multiregional MR imaging features. Radiology. (2016) 281:907–18. doi: 10.1148/radiol.2016161382
81. Wang S, Martinez-Lage M, Sakai Y, Chawla S, Kim SG, Alonso-Basanta M, et al. Differentiating tumor progression from pseudoprogression in patients with glioblastomas using diffusion tensor imaging and dynamic susceptibility contrast MRI. Am J Neuroradiol. (2016) 37:28–36. doi: 10.3174/ajnr.A4474
82. Jang B-S, Jeon SH, Kim IH, Kim IA. Prediction of Pseudoprogression versus progression using machine learning algorithm in glioblastoma. Sci Rep. (2018) 8:12516. doi: 10.1038/s41598-018-31007-2
83. Rowe LS, Butman JA, Mackey M, Shih JH, Cooley-Zgela T, Ning H, et al. Differentiating pseudoprogression from true progression: analysis of radiographic, biologic, and clinical clues in GBM. J Neurooncol. (2018) 139:145–52. doi: 10.1007/s11060-018-2855-z
84. van den Bent MJ, Dubbink HJ, Marie Y, Brandes AA, Taphoorn MJB, Wesseling P, et al. IDH1 and IDH2 mutations are prognostic but not predictive for outcome in anaplastic oligodendroglial tumors: a report of the European Organization for Research and Treatment of Cancer Brain Tumor Group. Clin Cancer Res. (2010) 16:1597–604. doi: 10.1158/1078-0432.CCR-09-2902
85. Cancer Genome Atlas Research Network. Comprehensive genomic characterization defines human glioblastoma genes and core pathways. Nature. (2008) 455:1061–8. doi: 10.1038/nature07385
86. Prior FW, Clark K, Commean P, Freymann J, Jaffe C, Kirby J, et al. TCIA: an information resource to enable open science. Conf Proc IEEE Eng Med Biol Soc. (2013) 2013:1282–5. doi: 10.1109/EMBC.2013.6609742
87. Kalpathy-Cramer J, Freymann JB, Kirby JS, Kinahan PE, Prior FW. Quantitative imaging network: data sharing and competitive algorithmvalidation leveraging the cancer imaging archive. Transl Oncol. (2014) 7:147–52. doi: 10.1593/tlo.13862
88. Liu Y, Xu X, Yin L, Zhang X, Li L, Lu H. Relationship between glioblastoma heterogeneity and survival time: an MR imaging texture analysis. Am J Neuroradiol. (2017) 38:1695–701. doi: 10.3174/ajnr.A5279
89. Chow D, Chang P, Weinberg BD, Bota DA, Grinband J, Filippi CG. Imaging genetic heterogeneity in glioblastoma and other glial tumors: review of current methods and future directions. Am J Roentgenol. (2017) 210:30–38. doi: 10.2214/AJR.17.18754
90. Liang S, Zhang R, Liang D, Song T, Ai T, Xia C, et al. Multimodal 3D DenseNet for IDH genotype prediction in gliomas. Genes. (2018) 9:9080382. doi: 10.3390/genes9080382
91. Eichinger P, Alberts E, Delbridge C, Trebeschi S, Valentinitsch A, Bette S, et al. Diffusion tensor image features predict IDH genotype in newly diagnosed WHO grade II/III gliomas. Sci Rep. (2017) 7:13396. doi: 10.1038/s41598-017-13679-4
92. Delfanti RL, Piccioni DE, Handwerker J, Bahrami N, Krishnan A, Karunamuni R, et al. Imaging correlates for the 2016 update on WHO classification of grade II/III gliomas: implications for IDH, 1p/19q and ATRX status. J Neurooncol. (2017) 135:601–9. doi: 10.1007/s11060-017-2613-7
93. Han Y, Xie Z, Zang Y, Zhang S, Gu D, Zhou M, et al. Non-invasive genotype prediction of chromosome 1p/19q co-deletion by development and validation of an MRI-based radiomics signature in lower-grade gliomas. J Neurooncol. (2018) 140:297–306. doi: 10.1007/s11060-018-2953-y
94. Wei J, Yang G, Hao X, Gu D, Tan Y, Wang X, et al. A multi-sequence and habitat-based MRI radiomics signature for preoperative prediction of MGMT promoter methylation in astrocytomas with prognostic implication. Eur Radiol. (2019) 29:877–88. doi: 10.1007/s00330-018-5575-z
95. Hong EK, Choi SH, Shin DJ, Jo SW, Yoo R-E, Kang KM, et al. Radiogenomics correlation between MR imaging features and major genetic profiles in glioblastoma. Eur Radiol. (2018) 28:4350–61. doi: 10.1007/s00330-018-5400-8
96. Sun R, Limkin EJ, Vakalopoulou M, Dercle L, Champiat S, Han SR, et al. A radiomics approach to assess tumour-infiltrating CD8 cells and response to anti-PD-1 or anti-PD-L1 immunotherapy: an imaging biomarker, retrospective multicohort study. Lancet Oncol. (2018) 19:1180–91. doi: 10.1016/S1470-2045(18)30413-3
98. Kros JM, van Run PR, Alers JC, Avezaat CJ, Luider TM, van Dekken H. Spatial variability of genomic aberrations in a large glioblastoma resection specimen. Acta Neuropathol. (2001) 102:103–9. https://www.ncbi.nlm.nih.gov/pubmed/11547944
99. Woodworth GF, McGirt MJ, Samdani A, Garonzik I, Olivi A, Weingart JD. Frameless image-guided stereotactic brain biopsy procedure: diagnostic yield, surgical morbidity, and comparison with the frame-based technique. J Neurosurg. (2006) 104:233–7. doi: 10.3171/jns.2006.104.2.233
100. Steinmetz MP, Barnett GH, Kim BS, Chidel MA, Suh JH. Metastatic seeding of the stereotactic biopsy tract in glioblastoma multiforme: case report and review of the literature. J Neurooncol. (2001) 55:167–71. doi: 10.1023/A:1013873431159
101. Perrin RG, Bernstein M. Iatrogenic seeding of anaplastic astrocytoma following stereotactic biopsy. J Neurooncol. (1998) 36:243–6. doi: 10.1023/A:1005823805767
102. Zinn PO, Singh SK, Kotrotsou A, Hassan I, Thomas G, Luedi MM, et al. A coclinical radiogenomic validation study: conserved magnetic resonance radiomic appearance of periostin-expressing glioblastoma in patients and xenograft models. Clin Cancer Res. (2018) 24:6288–99. doi: 10.1158/1078-0432.CCR-17-3420
103. McNutt TR, Benedict SH, Low DA, Moore K, Shpitser I, Jiang W, et al. Using big data analytics to advance precision radiation oncology. Int J Radiation Oncol Biol Phys. (2018) 101:285–91. doi: 10.1016/j.ijrobp.2018.02.028
104. LeCun Y, Bengio Y, Hinton G. Deep learning. Nature. (2015) 521:436–44. doi: 10.1038/nature14539
105. Djuric U, Zadeh G, Aldape K, Diamandis P. Precision histology: how deep learning is poised to revitalize histomorphology for personalized cancer care. Precision Oncol. (2017) 1:22. doi: 10.1038/s41698-017-0022-1
Keywords: brain, cancer, diagnosis, glioma, MRI, radiomics, glioblastoma
Citation: Chaddad A, Kucharczyk MJ, Daniel P, Sabri S, Jean-Claude BJ, Niazi T and Abdulkarim B (2019) Radiomics in Glioblastoma: Current Status and Challenges Facing Clinical Implementation. Front. Oncol. 9:374. doi: 10.3389/fonc.2019.00374
Received: 26 November 2018; Accepted: 23 April 2019;
Published: 21 May 2019.
Edited by:
Marcos Vinicius Calfat Maldaun, Hospital Sírio-Libanês, BrazilReviewed by:
Sunit Das, St. Michael's Hospital, CanadaRiccardo Soffietti, University of Turin, Italy
Copyright © 2019 Chaddad, Kucharczyk, Daniel, Sabri, Jean-Claude, Niazi and Abdulkarim. This is an open-access article distributed under the terms of the Creative Commons Attribution License (CC BY). The use, distribution or reproduction in other forums is permitted, provided the original author(s) and the copyright owner(s) are credited and that the original publication in this journal is cited, in accordance with accepted academic practice. No use, distribution or reproduction is permitted which does not comply with these terms.
*Correspondence: Bassam Abdulkarim, bassam.abdulkarim@mcgill.ca