- 1Department of Physical Therapy, University of British Columbia, Vancouver, BC, Canada
- 2Rehabilitation Research Program, GF Strong Rehabilitation Centre, Vancouver, BC, Canada
- 3School of Physical Therapy, Western University, London, ON, Canada
- 4Department of Occupational Therapy and Graduate Institute of Behavioral Sciences, College of Medicine, Chang Gung University, Taoyuan City, Taiwan
- 5Department of Physical Medicine and Rehabilitation, Chang Gung Memorial Hospital, Chiayi, Taiwan
- 6The David Mowafaghian Centre for Brain Health, University of British Columbia, Vancouver, BC, Canada
- 7Centre for Hip Health and Mobility, Vancouver Coastal Health Research Institute, Vancouver, BC, Canada
Background: Walking within the community requires the ability to walk while simultaneously completing other tasks. After a stroke, completing an additional task while walking is significantly impaired, and it is unclear how the functional activity of the brain may impact this.
Methods: Twenty individual in the chronic stage post-stroke participated in this study. Functional near-infrared spectroscopy (fNIRS) was used to measure prefrontal, pre-motor, sensorimotor, and posterior parietal cortices during walking and walking while completing secondary verbal tasks of varying difficulty. Changes in brain activity during these tasks were measured and relationships were accessed between brain activation changes and cognitive or motor abilities.
Results: Significantly larger activations were found for prefrontal, pre-motor, and posterior parietal cortices during dual-task walking. Increasing dual-task walking challenge did not result in an increase in brain activation in these regions. Higher general cognition related to lower increases in activation during the easier dual-task. With the harder dual-task, a trend was also found for higher activation and less motor impairment.
Conclusions: This is the first study to show that executive function, motor preparation/planning, and sensorimotor integration areas are all important for dual-task walking post-stroke. A lack of further brain activation increase with increasing challenge suggests a point at which a trade-off between brain activation and performance occurs. Further research is needed to determine if training would result in further increases in brain activity or improved performance.
Introduction
Successfully walking within the community requires an intricate ability to continue walking while completing various additional tasks such as conversing and avoiding obstacles (i.e., dual-tasking). The addition of these secondary tasks often results in a decline in performance compared to completing the task on its own (i.e., walking only or talking only). After a stroke, the magnitude of decline is typically significantly larger than that observed in their age-matched healthy counterparts (1) and the decline in the overall dual-task performance are related to an individual's functional ambulation category (2) and number of falls (3). In order to improve walking post-stroke, it may be important to understand the mechanisms underlying dual-tasking in this population.
Performance of two tasks simultaneously may require additional or alternative neural resources compared to completing each task on its own (4). Several mechanistic hypotheses have been proposed to help explain performance during dual-task walking. Depending on the neuronal requirements of each task, there can be a bottleneck (5), lack of resources (6), or capacity limit (7) that impact the performance of each task when completed together. In brief, the Bottleneck Theory posits that certain components of each task are able to undergo parallel processing, however, if the two tasks require similar processes at the same time [e.g., response selection (8)], a bottleneck occurs and only one task will be completed at a time (5). Therefore, when asked to simultaneously complete two tasks (e.g., walking and arithmetic), if one task requires longer processing (e.g., arithmetic), the completion of the second task will be delayed (e.g., decreased gait speed). The Multiple Resource Theory suggests that there is a limit on the number of resources available at a given time. If two tasks require more resources than what is available, especially similar resources, a deterioration of one or both tasks may be observed (9). Theoretically, simultaneous completion of two motor tasks would have greater interference than completing a cognitive and motor task simultaneously. Finally, the Capacity Limit Theory states that parallel processing can occur when two tasks are presented, however, functional resources will be shared between the two, resulting in an increased completion time for both tasks (7). For a single task, in comparison to healthy adults, individuals with stroke generally show greater brain activity (10). With this already elevated brain activation, a limit may be met sooner in individuals post-stroke. Several studies have shown this capacity limit in older adults where neural activation no longer increases or sometimes even decreases with dual-tasks [review: (11)].
Evidence of this across multiple cortical regions in the stroke population is limited. After stroke, we have shown that faster walking is related to an increased magnitude of brain activation in prefrontal cortex (PFC), sensorimotor cortex (SMC), and posterior parietal cortex (PPC) (12). When walking complexity increases, increased activation has primarily been observed in PFC and only a few studies showed activation in pre-motor cortex (PMC) and SMC [review: (13, 14)]. While the PFC plays an important role in dual-task walking, the literature has also identified other cortical areas that are involved in dual-tasking [review: (4)]. These areas include the PMC (15, 16), SMC (17, 18), and PPC (19, 20). The functional role of these additional areas can provide some insight on how resources are being allocated during different walking complexities. For example, increases in PMC activity would suggest a need for more motor planning and preparation, SMC increases would suggest an impact on motor output or sensory input, and PPC activity would suggest heightened need for sensorimotor integration. Additionally, the relationship between brain activation and clinical measures is unclear; one study showed that higher cognitive and motor status predicted greater brain activation during complex walking (21) but another study showed a negative relationship with motor status and dual-task brain activation (22). Thus, further work is needed to characterize brain activation during dual-task walking after stroke and to determine the relationship between the activation levels and clinical measures.
The overall purpose of this study is to investigate how brain activation changes with dual-task walking after stroke and how it relates to an individual's cognitive and motor status. Specifically, we first look at how the addition of a secondary cognitive task to an existing motor task will increase overall brain activation and if the addition of a more difficult secondary cognitive task will further increase activation. Secondly, we aim to determine if the magnitude of regional brain activity changes relate to an individual's cognitive or motor status.
Methods
Participants
Recruitment
Participants were recruited by purposive sampling through posters at local rehabilitation centers, private clinics, and online platforms. Study details were also disseminated through phone or mail to previous participants who have agreed to be contacted for future studies. The study was approved by the university clinical research ethics board and all participants provided written and informed consent.
Screening
Interested individuals were first screened for eligibility via telephone. Inclusion criteria included an age ≥18, telephone Mini-Mental Status Exam >21/26 (23, 24) indicating mild cognitive impairment at most, stroke incident >6 months previous (i.e., chronic stroke), one-sided hemiparesis, able to walk independently (gait aids allowed) for 1-min bouts, able to understand and follow directions in English, and able to clearly communicate verbally. Exclusion criteria included orthopedic injury impairing current walking, neurological injury other than stroke, and multiple known strokes.
Demographic Data
Age, sex, and gait aid used were collected. When available, stroke characteristics were obtained through medical charts. When charts were not available, details on time post-stroke, and stroke type (ischemic/hemorrhagic) were collected through verbal reports by the participants. Lesion location was determined through structural MRI obtained through medical records or collected for this study when eligible.
Task Procedure
The walking tasks were completed in a 50-m hallway. Participants performed three walking conditions: walking only (ST-Walk), walking while saying a word repeatedly (DT-Easy), and walking while completing a verbal fluency task (DT-Hard). All participants first completed the ST-Walk condition then either the DT-Easy or DT-Hard condition (randomized) (Figure 1A). For the ST-Walk condition, participants were asked to walk at their comfortable-pace. During the DT-Easy condition, participants were told to walk while continuously and audibly saying one of the following words: ma, pa, da, ba, ta. Finally, for the DT-Hard condition, participants were told to walk while saying as many words as possible that started with one of the following letters: B, R, D, C, H. Repeat words, proper names, or words with similar prefixes but a different suffix were not accepted. These specific letters were classified as easy-moderate difficulty (25) and has approximately the same number of words within the English Oxford dictionary.
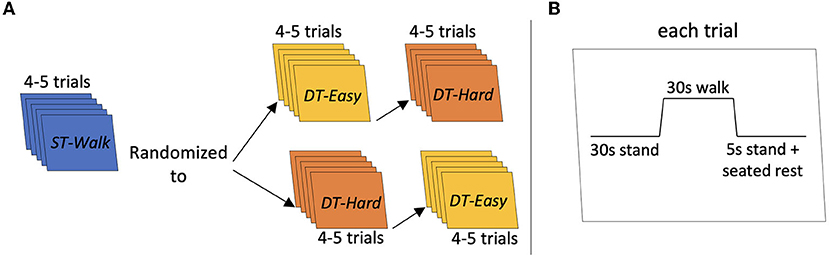
Figure 1. Schematic of task procedure. (A) Indicates the procedure for each trial. (B) Indicates the order of the conditions. Each participant started with 4–5 trials of ST-Walk first then they were randomized to either DT-Easy or DT-Hard walking conditions next.
Participants were first provided with 1–2 familiarization trials of each walking condition. Each trial started with the participant standing at either end of the hallway. The starting side was randomly determined by the researcher. After a minimum 30 s quiet stance, a verbal “go” from the researcher indicated the start of the walking trial and a verbal “stop” indicated the end of the trial. All participants were given instructions to keep their head position consistent and avoid talking throughout the walking only trials. Each walking trial was 30 s long and was performed 4–5 times. For all trials, a manual wheelchair and a spotter were positioned behind the participant for safety. At the end of each walking trial, participants stood for 5 s; they were then asked to sit in the wheelchair and then pushed to the end of the hallway to start the next trial. All trials had at least 30 s of quiet standing immediately prior to the start of the trial (Figure 1B)—this allowed for the functional near-infrared spectroscopy signals to return to baseline and a portion of this period was used for baseline comparisons. The PychoPy3.0 program was used for randomizing the conditions and triggering/timing the trials (26).
Measures
Functional Brain Activation
Functional brain activity was measured using functional near-infrared spectroscopy (fNIRS). A wireless and portable fNIRS device (NIRSport2, NIRx Medical Technology, Germany) was used. Emitters released near-infrared light at 760 and 850 nm which enabled measurement of both HbO and HbR. The optodes were wired to the fNIRS collection device that was worn as a backpack by the participants. fNIRS data were continuously sampled at 4.36 Hz through Aurora 1.4 (NIRx Medical Technologies, Berlin, Germany). The probe configuration for this experiment arranged the emitter and detector pairs to result in 48 long separation channels (~30–35 mm apart) and 8 short separation channels (7 mm apart). The fNIRS cap was set up by locating the Cz position (27) at the midpoint between the nasion and inion and the periauricular points and visually inspected for alignment along the midsagittal plane (Figure 2). Precise localization of each fNIRS channel was determined using a 3D digitizer (Polhemus Patriot, USA) with a custom interface for accurate placement of the digitization stylus in the optode holder. Channels were then grouped into regions of interest using the digitized points that were converted to Montreal Neurological Institute using AtlasViewer (28) and then Brodmann labels using the Allen Human Brain Atlas (29) and the Yale BioImage Suite Package web application (30). Regions of interest included the PFC, PMC, SMC, and PPC. For breakdown on the number of channels that contributed to each region for each participant see Supplementary Table 3. Channels over cortical stroke lesions were identified using individual MRIs and removed from further analysis.
Cognitive Status
The Montreal Cognitive Assessment (MoCA) (31) was used to assess global cognition. It assesses a range of cognitive constructs such as: visuoconstructional skills, memory, attention and concentration, executive function, language, conceptual thinking, calculations, and orientation. It has excellent inter-rater reliability (32).
Motor Status
The lower extremity portion of the Fugl-Meyer Assessment (FMLE) (33) was used to evaluate motor impairment after stroke. It has excellent inter- (34) and intra-rater (35) reliability, and is a recommended outcome measure for individuals living after stroke (36).
Both MoCA and FMLE were conducted by a trained physiotherapist either on the same day of or within 7 days of fNIRS testing.
Gait Speed
Gait speeds were first calculated for every trial by determining the distance walked during the 30 s trials. An average gait speed was calculated for each condition. A percent change in gait speed between the single- and dual-task conditions was calculated using the following formula:
With this equation, a positive percent shows an increase in gait speed during the dual-task conditions (i.e., DT-Easy and DT-Hard) compared to the single task (i.e., ST-Walk). Whereas a negative percentage indicates a decrease in gait speed during dual- compared to single-task.
Analysis
To assess the impact of secondary tasks on walking performance, paired t-tests were conducted between ST-Walk, DT-Easy, and DT-Hard. A corrected p ≤ 0.017 was used to adjust for multiple comparisons.
Preprocessing of fNIRS data was completed using an open source software, HomER2 (37). All HomER2 functions and corresponding parameters are indicated within square brackets. Noisy channels were first removed [enPruneChannels: SNRtresh = 2, dRange = 5e-4 to 1e + 00, SDrange:0–45] and compared with the calibration from the Aurora software. Data were then converted into optical density [hmrIntensity2OD]. Motion artifacts were then identified by using 0.5 s time windows to determine if the signal exceeded either 20 standard deviations above the mean signal for each channel or showed a change >100 times in amplitude [hmrMotionArtifactByChannel: tMotion = 0.5, tMask = 1.0, STDEVthresh = 20.0, AMPthresh = 100.00]. The number of removed channels for each participant can be found in Supplementary Table 4. Motion correction was then applied using a wavelet transformation with a 1.5 interquartile range [hmrMotionCorrectWavelet: iqr = 1.5] (38, 39). A lowpass filter of 0.15 Hz was then applied to the data [hmrBandpassFilt: lpf = 0.15] and converted to hemoglobin concentration using the modified Beer-Lambert equation [hmrOD2Conc: ppf = 6.0] (40, 41). The hemodynamic response was estimated using a general linear model with an ordinary least squares approach (42, 43) and a 0.5 s width and 0.5 s step consecutive gaussian basis function (44). Superficial contributions to the signal were also removed by regressing out the data from the short separation channel that has the highest correlation to each channel (43–47). Any drift within the signal was corrected using a 3rd order polynomial correction (43) [hmrDeconvHRF_DriftSS: trange = −20.0 35.0, glmSolveMethod = 1, idxBasis = 1, paramsBasis = 0.5 0.5, rhoSD_ssThresh = 15.0, flagSSmethod = 1, driftOrder = 3, flagMotionCorrect = 0].
Preprocessed data were exported to a custom Matlab script for baseline corrections (−5–0 s before walking onset). Brain activations during the task were then determine by averaging hemoglobin response amplitudes during the first 20 s of walking and subtracting this average by the average response during the 5 s prior to walking.
To look at the effects of increasing walking complexity on brain activation, the conditions (ST-Walk, DT-Easy, DT-Hard) were included as fixed effects within a linear mixed model. Hemisphere (ipsilesional, contralesional) was also included as fixed effects and Participants were added as random effects. Four separate linear mixed models were created for each region of interest (PFC, PMC, SMC, PPC). Both HbO and HbR were assessed. As HbO is more reproducible and stable over time (48), has the highest correlation to fMRI BOLD measures (49), and has shown more changes with walking after a stroke [(12) current issue], results will focus on HbO findings and HbR results will only be presented as tables in the Supplementary Material. The statistical package “lme4” within the R Studio software was used to model the data. Interaction effects between fixed variables were assessed and only included if it significantly contributed to the model.
To assess the relationship between changes in brain activation and cognitive or motor status, Pearson's correlations were computed. If data was not normally distributed or contained outliers, Spearman's rank-order correlations were computed instead. Relationships between changes in brain activation (DT-Easy minus ST-Walk and DT-Hard minus ST-Walk) and MoCA and FMLE scores were assessed.
All relevant assumptions and diagnostics were checked for each statistical test and appropriate modifications were made and reported when necessary. Given the relatively small sample size with exploratory objectives, we present the data results with a standard alpha of 0.05 in order not to miss potential relationships. We also show the data with a Bonferroni corrected p ≤ 0.0125 (0.05/4 models) and Benjamini–Hochberg corrected correlation findings demonstrating the results if we reduce the risk of false positives (type 1 error) with multiple comparison.
To facilitate future discussion and investigations on diversity within neuroscientific findings (50), we conducted exploratory subgroup analyses to explore any possible differences with sex. All brain data across each condition were averaged across sex and effect sizes and confidence intervals of the difference between the two sexes are reported.
Results
All 20 participants completed the ST-Walk and DT-Hard condition. One participant was too fatigued to continue and did not complete the DT-Easy condition. Participant average demographic and performance data are presented in Table 1. Detailed individual data are presented in Supplementary Table 1.
Performance Results
The mean gait speed from the ST-Walk to the DT-Easy was similar. Individual data showed that ten participants increased their speed [8.70 (5.071)%] while eight participants decreased their speed [−6.40 (5.107)%]; one participant showed no change in gait speed. For DT-Hard, there was a significant reduction of about 10% in speed; three participants increased their gait speed [7.25 (1.328)%] while 17 participants decreased their gait speed [−12.86 (6.366)%]. Group averages of gait speed for each condition are shown in Table 1 and individual performance data are shown in Supplementary Table 1.
Significant effects of walking condition were observed with a significant increase in activation in PFC and PMC during both DT-Easy and DT-Hard conditions compared to ST-Walk (Table 2, Figure 3). A significant increase in PPC activation was found for DT-Easy compared to ST-Walk. There was an increase in SMC activation for DT-Easy with the standard alpha of 0.05 but it did not reach significance with a Bonferroni correction. There was a significant effect of Hemisphere for PFC with the ipsilesional hemisphere overall showing greater activation compared to the contralesional hemisphere. There was also an effect of Hemisphere for PPC with the contralesional hemisphere showing greater activation with the standard alpha, but it did not remain significant with the Bonferroni correction. The inclusion of an interaction term (Hemisphere*Condition) did not significantly add to the model and thus was not included in the final model.
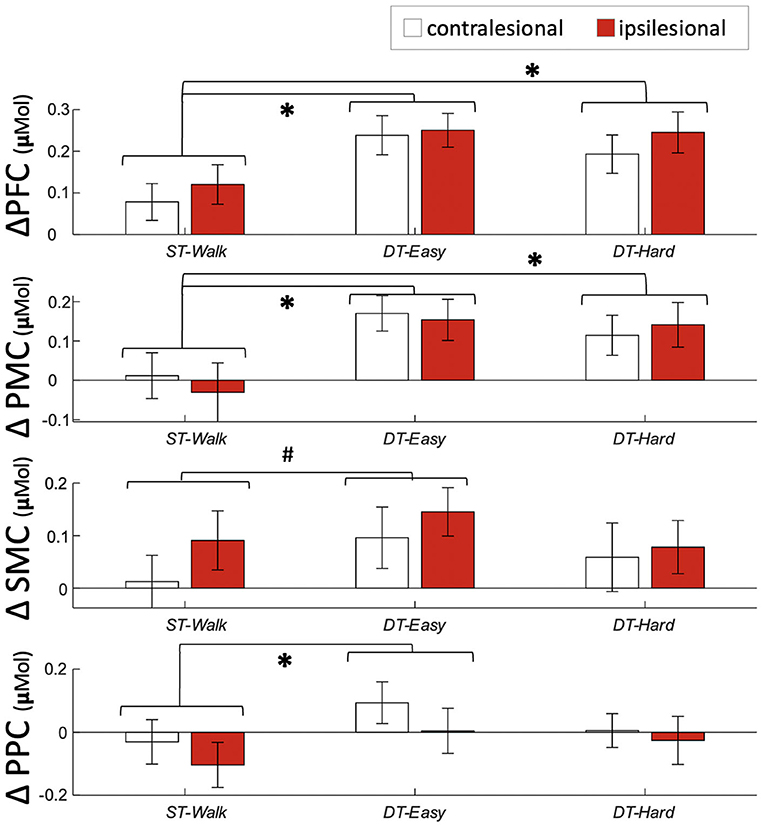
Figure 3. Average and standard error activation during each walking condition. #Indicates a significant difference between conditions with p <0.05 *indicates a significant difference between conditions with p <0.0125. PFC, prefrontal cortex; PMC, pre-motor cortex; SMC, sensorimotor cortex; PPC, posterior parietal cortex.
MoCA scores were not normally distributed, and Spearman's rank-order correlations were conducted to account for this. Individual MoCA scores related to brain activation changes during DT-Easy and DT-Hard. This relationship was only observed in ipsilesional PMC when looking at changes with the easy dual-task. Specifically, higher MoCA scores (i.e., higher cognitive status) related to less increase in brain activation during the dual-task walking conditions compared to walking only (Table 3, Figure 4).
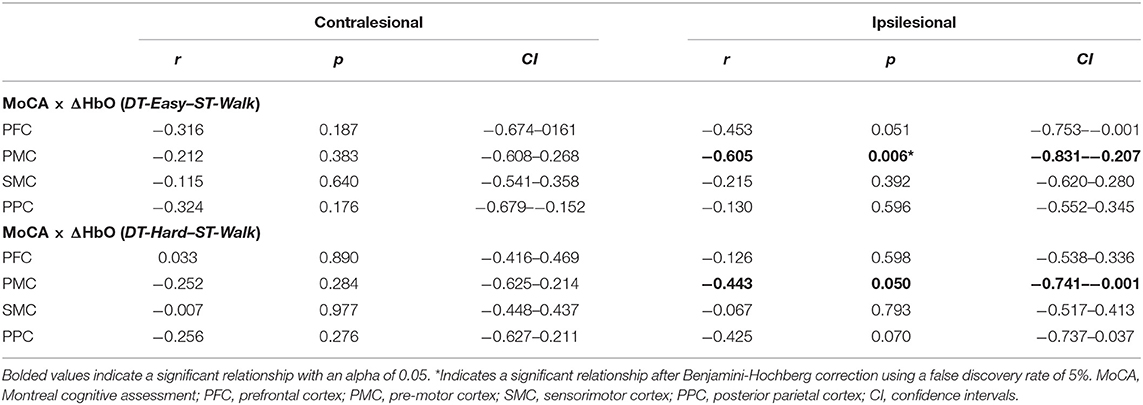
Table 3. Spearman's rank-order correlation results between cognitive status (MoCA) and changes in brain activation (ΔHbO).
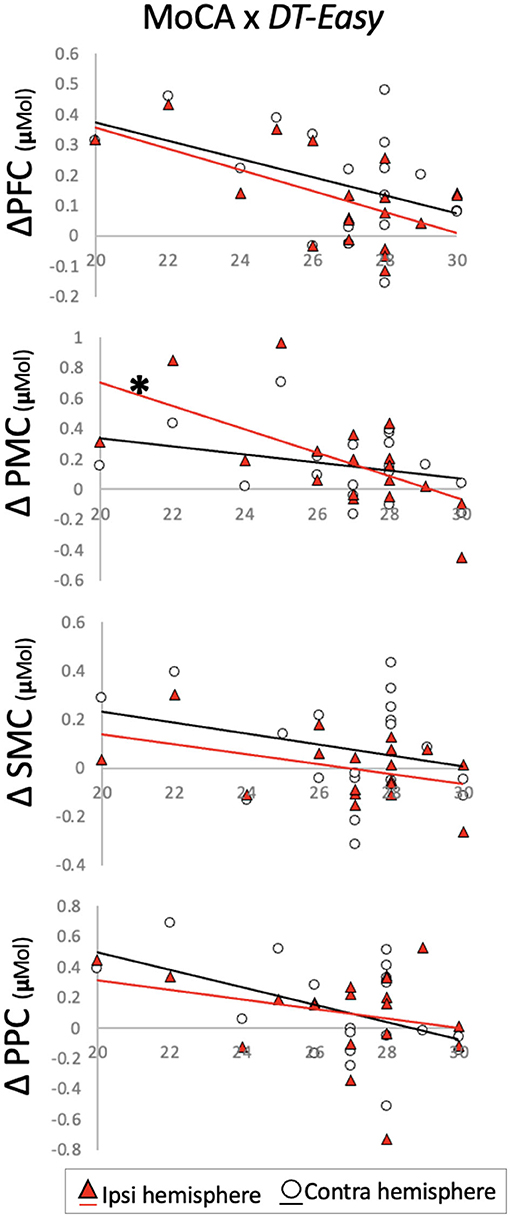
Figure 4. Change in brain activation from (y-axis) ST-Walk to DT-Easy plotted against Montreal Cognitive Assessment (MoCA) scores (x-axis). *indicates significant correlation after Benjamini–Hochberg correction. PFC, prefrontal cortex; PMC, pre-motor cortex; SMC, sensorimotor cortex; PPC, posterior parietal cortex.
Individual FMLE scores related to brain activation changes during the DT-Hard condition only. These relationships were observed in contralesional PMC and PPC and in bilateral SMC. Less impairment (i.e., higher FMLE scores) correlated to a greater increase in brain activation from the -ST-Walk condition. After correction for multiple comparisons, however, no relationships remained significant (Table 4, Figure 5).
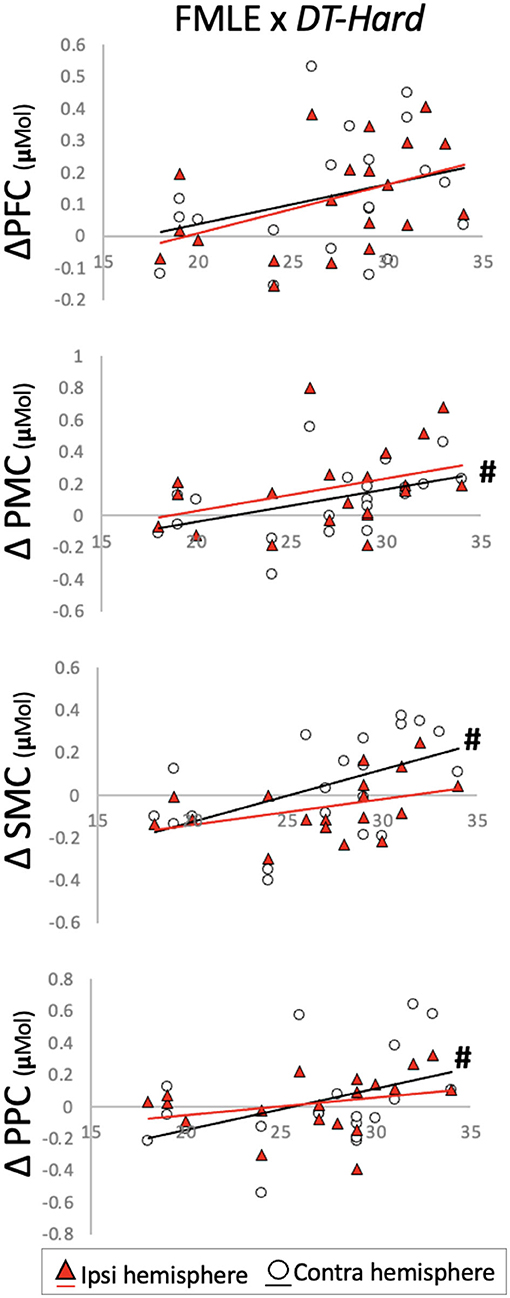
Figure 5. Change in brain activation from (y-axis) ST-Walk to DT-Hard plotted against the Fugl-Meyer Lower Extremity (FMLE) scores (x-axis). #Indicates significant correlation with alpha at 0.05. PFC, prefrontal cortex; PMC, pre-motor cortex; SMC, sensorimotor cortex; PPC, posterior parietal cortex.
Changes in brain activation between ST-walk and DT-Easy for contralesionally PPC were not normally distributed and Spearman's rank-order correlations were conducted when determining relationships with the MoCA or FMLE. One outlier was also found for ipsilesional SMC and PPC and was removed before correlation analysis.
Table 5 shows the brain activation data separated by sex. In general, mean values show a trend toward brain activation levels being greater in male vs. female participants. Medium effect sizes were primarily found between males and females for the ST-Walk condition. A range of small to large effect sizes were calculated for the DT-Easy condition and only small effect sizes were calculated for the DT-Hard condition. Confidence intervals for all brain regions crossed zero, indicating greater sample sizes are needed to find differences between sexes for regions and tasks with medium to large effect sizes.
Discussion
We found increased PFC, PMC, and PPC activation with dual-task walking as we hypothesized; however, the magnitude of activation did not further increase with increasing complexity of dual-task walking. We did not observe any increase of SMC activation with increasing task complexity. We also showed relationships between the amount of activation increase with walking complexity and individuals' cognitive status. Individuals with higher MoCA scores had lower increases in brain activity when walking while completing an easy dual-task compared to walking on its own.
Dual-Task Walking Involves Executive Function, Motor Planning, and Sensorimotor Integration Areas
Increases in executive function and motor planning regions were observed for both dual-task conditions. Increases in the executive function area (i.e., PFC) during dual-task walking are well-known and have been observed in several populations (14, 51–53). Increases in PMC, however, are less investigated and have only been documented once using fNIRS in the stroke population (54). Liu et al. (54) looked at PFC, PMC, and supplementary motor area activation during dual-task walking in individuals >6 months post-stroke. Their participants were on average over 10 years younger (51 vs. 64 years) and had greater walking ability (minimum comfortable walking speed of 0.58 vs. 0.12 m/s, and no gait aids vs. 7 people using gait aids) compared to the current study. Despite these demographic differences, they similarly found increases in bilateral PMC with cognitive dual-task walking compared to walking only. Together, these two studies suggest an important need for ongoing motor planning and preparation during complex walking after stroke.
Greater activity in PPC was observed for the easier dual-task condition only. With an additional task, a greater amount of sensorimotor integration would be needed to perform the task. It is possible that the increased complexity of the harder dual-task required resources to be allocated away from PPC to meet the demands of other regions for task completion. Increases in other regions would then be observed. We, however, did not find any increases in activation from the easier to harder dual-task, which may suggest that resources were being directed to subcortical regions such as the cerebellum (4). Further research is needed to test this hypothesis. Alternatively, the nature of the easier dual-task may explain the activation of PPC. PPC is known to be involved in rhythmic, beat-based timing (55) and for the easier dual-task, participants typically repeated the word in a rhythmic manner. This beat-like vocalization was not specifically documented in this study and further work is needed to understand the differential role of PPC in dual-tasking.
A Brain Activation and Gait Performance Trade-Off
Interestingly, the increase in dual-task complexity did not result in a further increase in activation for the brain regions measured. While previous literature suggests an increase in brain activity with increasing task complexity (56, 57), only one previous study had investigated different difficulties of dual-task walking in individuals post-stroke (58). Hermand et al. assessed PFC activation during walking only compared to walking while completing a 1-back and a 2-back cognitive test. No differences in brain activation were found between any walking condition. Significant decreases in gait speed were seen in both dual-task conditions and the harder dual-task resulted in greater errors on the secondary task. Thus, their lack of PFC increase suggests that a limit was reached, which then came at a detriment to performance. In the current study, we only showed a decrease in gait speed with the harder dual-task and not the easier dual-task despite seeing increases in PFC and PMC activity for both conditions and no differences between the two dual-tasks. Along the same lines of the study by Hermand et al. (58), our results could be explained by a brain activation and performance trade off. For the easier dual-task, increases in brain activity were needed to maintain gait performance, however, with the harder dual-task, a limit may have been reached and this came at a cost to gait performance. This is in line with the Capacity Limit Theory (7).
Brain Activation Changes Relating to Cognitive and Motor Status
For the easier dual-task, it appears that individuals with better general cognitive status (i.e., higher MoCA score) do not activate brain regions as much as those with lower MoCA scores. This negative relationship is observed in the regions involved in executive function (PFC) and motor planning/preparation (PMC), and integration (PPC) areas, with the strongest correlation in the motor planning/preparation region. Along similar lines, previous work has shown that individuals who show greater intelligence also show more efficient brain activity (59, 60). This may suggest that individuals who have a higher cognitive status may be more efficient (i.e., less additional resources required) at performing easy dual-task walking. Alternatively, the easy verbal task may simply not require any additional resources for individuals with higher cognitive status. Thus, combining walking with the easy verbal task does not elevate brain activity more than just walking alone. With a harder dual-task, however, the relationship is not as strong. With an alpha of 0.05, a significant moderate correlation was observed for ipsilesional PMC only. It's possible that the cognitive demand of the harder verbal task requires additional resources from all individuals. In fact, Neubauer and Fink (61) conducted a review to assess the relationship between intelligence and neural efficiency. They concluded that those with higher intelligence show great efficiency with subjectively easy tasks whereas brain activity during harder tasks was not as efficient and possibly even greater in those with higher intelligence.
On the other hand, with an alpha of 0.05, relationships with an individual's Fugl-Meyer score showed that those with higher motor status activated motor regions (PMC, SMC, PPC) to a greater extent. This may be because they have a greater capacity to increase these brain regions and increase it to a greater extent to mitigate the reductions in gait speed during the harder dual-task. To further explore this, we ran a Pearson's correlation between individual's motor status and their percent change in gait speed for the hard dual-task; they were not correlated (r2 = 0.003, p = 0.74). Alternatively, the greater increase may simply be due to their actual gait speeds during the hard dual-task: motor status and dual-task gait speed were correlated (r2 = 0.443, p = 0.001). This suggests that these individuals simply activate motor regions more because they are able to walk faster.
Limitations
The study results are limited to the specific dual-tasks measured. Previous studies have utilized numerous secondary tasks with walking and have found differing results (21, 22, 54, 58, 62, 63). While some have suggested that verbal tasks are not recommended with fNIRS due to the higher potential of motion artifacts created (53), the secondary tasks that were chosen for this study were intentional. Yang et al. (64) assessed the psychometric properties of different secondary tasks with walking and showed the greatest reliability with using a verbal fluency task. Reliability was an important factor to consider for the purposes of future interventional or longitudinal research and the clinical impact of these results. In addition, pilot tests and visual inspection of the data did not show any motion artifacts related to verbal responses, especially after pre-processing.
Our results are also limited to the small sample size and inter-subject variability. To account for this, results were presented with both an alpha of 0.05 and with corrections for multiple comparisons. The large range in comfortable walking speed and functional ambulation also likely contributes to the variability in functional brain activation. With a small sample size, subgroup analyses were not possible. Future work should utilize our results to determine appropriate sample sizes for subgroup analyses.
Conclusions
This is the first study to investigate frontal to parietal brain activations during real-time walking with a secondary task and relate it to cognitive and motor status post-stroke. An increase in walking complexity resulted in an increase in executive function, motor preparation/planning and sensorimotor integration areas. Increasing difficulty of the dual-task walking did not result in further increase in brain activation. In comparison to walking only, individuals with lower cognitive status required larger increases in executive function, motor preparation/planning, and sensorimotor integration areas during the easier dual-task walking. These findings provide further insight on the mechanisms of complex walking post-stroke. Future work should aim to assess longitudinal changes in brain activations during complex walking after stroke and determine if training can optimize dual-task performance and change functional brain activations.
Data Availability Statement
The original contributions presented in the study are included in the article/Supplementary Material, further inquiries can be directed to the corresponding author.
Ethics Statement
The studies involving human participants were reviewed and approved by Clinical Research Ethics Board, University of British Columbia. The patients/participants provided their written informed consent to participate in this study.
Author Contributions
SL and JE conceived the questions. SL, SP, and C-lY collected the data. SL analyzed the data and prepared the first draft of the manuscript. All authors contributed to the study design and edited the manuscript. All authors contributed to the article and approved the submitted version.
Funding
The authors would like to acknowledge the funding agencies that help supported this research: University of British Columbia 4-Year Fellowship (SL), Canadian Institute of Health Research (Fellowship for SL and SP; Foundation Grant FND143340 to JE), the Michael Smith Foundation for Health Research (SP), the Canada Research Chairs Program (JE, TL-A, and LB), and the Heart and Stroke Foundation Partnership for Stroke Recovery (Post-doc award to Cl-Y). The funders had no role in study design, data collection, analysis, or preparation of the manuscript.
Conflict of Interest
The authors declare that the research was conducted in the absence of any commercial or financial relationships that could be construed as a potential conflict of interest.
Publisher's Note
All claims expressed in this article are solely those of the authors and do not necessarily represent those of their affiliated organizations, or those of the publisher, the editors and the reviewers. Any product that may be evaluated in this article, or claim that may be made by its manufacturer, is not guaranteed or endorsed by the publisher.
Acknowledgments
This study was previously published within SL doctoral thesis from the University of British Columbia (2021).
Supplementary Material
The Supplementary Material for this article can be found online at: https://www.frontiersin.org/articles/10.3389/fneur.2022.904145/full#supplementary-material
References
1. Yang L, Lam F, Huang M, He C, Pang M. Dual-task mobility among individuals with chronic stroke: changes in cognitive-motor interference patterns and relationship to difficulty level of mobility and cognitive tasks. Euro J Phys Rehabil Med. (2017) 54:526–35. doi: 10.23736/S1973-9087.17.04773-6
2. Yang YR, Chen YC, Lee CS, Cheng SJ, Wang RY. Dual-task-related gait changes in individuals with stroke. Gait Posture. (2007) 25:185–90. doi: 10.1016/j.gaitpost.2006.03.007
3. Tsang CSL, Pang MYC. Association of subsequent falls with evidence of dual-task interference while walking in community-dwelling individuals after stroke. Clin Rehabil. (2020) 34:971–80. doi: 10.1177/0269215520923700
4. Leone C, Feys P, Moumdjian L, D'Amico E, Zappia M, Patti F. Cognitive-motor dual-task interference: a systematic review of neural correlates. Neurosci Biobehav Rev. (2017) 75:348–60. doi: 10.1016/j.neubiorev.2017.01.010
5. Ruthruff E, Pashler HE, Klaassen A. Processing bottlenecks in dual-task performance: structural limitation or strategic postponement? Psychon Bull Rev. (2001) 8:73–80. doi: 10.3758/BF03196141
6. Wickens CD. Multiple resources and mental workload. Hum Fact. (2008) 50:449–55. doi: 10.1518/001872008X288394
7. Tombu M, Jolicœur P. A central capacity sharing model of dual-task performance. J Exp Psychol Hum Percept Perform. (2003) 29:3–18. doi: 10.1037/0096-1523.29.1.3
8. Pashler H. Dual-task interference in simple tasks: data and theory. Psychol Bull. (1994) 116:220–44. doi: 10.1037/0033-2909.116.2.220
9. Mcleod P. A dual task response modality effect: support for multiprocessor models of attention. Q J Exp Psychol. (1977) 29:651–67. doi: 10.1080/14640747708400639
10. Lim SB, Eng JJ. Increased sensorimotor cortex activation with decreased motor performance during functional upper extremity tasks poststroke. J Neurol Phys Ther. (2019) 43:141–50. doi: 10.1097/NPT.0000000000000277
11. Udina C, Avtzi S, Durduran T, Holtzer R, Rosso AL, Castellano-Tejedor C, et al. Functional near-infrared spectroscopy to study cerebral hemodynamics in older adults during cognitive and motor tasks: a review. Front Aging Neurosci. (2020) 11:367. doi: 10.3389/fnagi.2019.00367
12. Lim SB. Shedding light on the brain-characterizing functional brain activation during simple and complex walking after stroke (Ph. D. thesis). University of British Columbia, Vancouver, BC, Canada (2021).
13. Kahya M, Moon S, Ranchet M, Vukas RR, Lyons KE, Pahwa R, et al. Brain activity during dual task gait and balance in aging and age-related neurodegenerative conditions: a systematic review. Exp Gerontol. (2019) 128:110756. doi: 10.1016/j.exger.2019.110756
14. Lim SB, Louie DR, Peters S, Liu-Ambrose T, Boyd LA, Eng JJ. Brain activity during real-time walking and with walking interventions after stroke: a systematic review. J Neuroeng Rehabil. (2021) 18:1–19. doi: 10.1186/s12984-020-00797-w
15. Blumen HM, Holtzer R, Brown LL, Gazes Y, Verghese J. Behavioral and neural correlates of imagined walking and walking-while-talking in the elderly. Hum Brain Mapp. (2014) 35:4090–104. doi: 10.1002/hbm.22461
16. Van Impe A, Coxon JP, Goble DJ, Wenderoth N, Swinnen SP. Age-related changes in brain activation underlying single- and dual-task performance: visuomanual drawing and mental arithmetic. Neuropsychologia. (2011) 49:2400–9. doi: 10.1016/j.neuropsychologia.2011.04.016
17. Gazes Y, Rakitin BC, Steffener J, Habeck C, Butterfield B, Ghez C, et al. Performance degradation and altered cerebral activation during dual performance: evidence for a bottom-up attentional system. Behav Brain Res. (2010) 210:229–39. doi: 10.1016/j.bbr.2010.02.036
18. Johnson AN, Shinohara M. Corticomuscular coherence with and without additional task in the elderly. J Appl Physiol. (2012) 112:970–81. doi: 10.1152/japplphysiol.01079.2011
19. Collette F, Olivier L, Van Der Linden M, Laureys S, Delfiore G, Luxen A, et al. Involvement of both prefrontal and inferior parietal cortex in dual-task performance. Cogn Brain Res. (2005) 24:237–51. doi: 10.1016/j.cogbrainres.2005.01.023
20. Rémy F, Wenderoth N, Lipkens K, Swinnen SP. Dual-task interference during initial learning of a new motor task results from competition for the same brain areas. Neuropsychologia. (2010) 48:2517–27. doi: 10.1016/j.neuropsychologia.2010.04.026
21. Chatterjee SA, Fox EJ, Daly JJ, Rose DK, Wu SS, Christou EA, et al. Interpreting prefrontal recruitment during walking after stroke: influence of individual differences in mobility and cognitive function. Front Hum Neurosci. (2019) 13:194. doi: 10.3389/fnhum.2019.00194
22. Hawkins KA, Fox EJ, Daly JJ, Rose DK, Christou EA, McGuirk TE, et al. Prefrontal over-activation during walking in people with mobility deficits: interpretation and functional implications. Hum Mov Sci. (2018) 59:46–55. doi: 10.1016/j.humov.2018.03.010
23. Newkirk LA, Kim JM, Thompson JM, Tinklenberg JR, Yesavage JA, Taylor JL. Validation of a 26-point telephone version of the mini-mental state examination. J Geriatr Psychiatry Neurol. (2004) 17:81–7. doi: 10.1177/0891988704264534
24. Folstein MF, Folstein SE, McHugh PR. “Mini-mental state”. A practical method for grading the cognitive state of patients for the clinician. J Psychiatr Res. (1975) 12:189–98. doi: 10.1016/0022-3956(75)90026-6
25. Borkowski JG, Benton AL, Spreen O. Word fluency and brain damage. Neuropsychologia. (1967) 5:135–40. doi: 10.1016/0028-3932(67)90015-2
26. Peirce J, Gray JR, Simpson S, Macaskill M, Höchenberger R, Sogo H, et al. PsychoPy2 : experiments in behavior made easy. Behav Res Methods. (2019) 51:195–203. doi: 10.3758/s13428-018-01193-y
27. Jurcak V, Tsuzuki D, Dan I. 10/20, 10/10, and 10/5 systems revisited: their validity as relative head-surface-based positioning systems. Neuroimage. (2007) 34:1600–11. doi: 10.1016/j.neuroimage.2006.09.024
28. Aasted CM, Yücel MA, Cooper RJ, Dubb J, Tsuzuki D, Becerra L, et al. Anatomical guidance for functional near-infrared spectroscopy: AtlasViewer tutorial. Neurophotonics. (2015) 2:020801. doi: 10.1117/1.NPh.2.2.020801
29. Hawrylycz MJ, Lein ES, Guillozet-bongaarts AL, Shen EH, Ng L, Miller JA, et al. An anatomically comprehensive atlas of the adult human brain transcriptome. Nature. (2012) 489:391–9. doi: 10.1038/nature11405
30. Lacadie C, Fulbright RK, Arora J, Constable R, Papademetris X. Brodmann areas defined in MNI space using a new tracing tool in BioImage suite. In: 14th Annual Meeting of the Organization for Human Brain Mapping (Melbourne, VIC). (2008).
31. Nasreddine Z, Phillips N, Bedirian V, Charbonneau S, Whitehead V, Collin I, et al. The montreal cognitive assessment, MoCA: a brief screening tool for mild cognitive impairment. J Am Geriatr Soc. (2005) 53:695–9. doi: 10.1111/j.1532-5415.2005.53221.x
32. Cumming TB, Lowe D, Linden T, Bernhardt J. The AVERT MoCA data: scoring reliability in a large multicenter trial. Assessment. (2020) 27:976–81. doi: 10.1177/1073191118771516
33. Fugl-Meyer AR, Jääsk, ö L, Leyman I, Olsson S, Steglind S. The post-stroke hemiplegic patient. 1. A method for evaluation of physical performance. Scand J Rehabil Med. (1975) 7:13–31.
34. Duncan P, Propst M, Nelson S. Reliability of the Fugl-Meyer assessment of sensorimotor recovery following cerebrovascular accident. Phys Ther. (1983) 63:1606–10. doi: 10.1093/ptj/63.10.1606
35. Sullivan KJ, Tilson JK, Cen SY, Rose DK, Hershberg J, Correa A, et al. Fugl-Meyer assessment of sensorimotor function standardized training procedure for clinical practice and clinical trials. Stroke. (2011) 42:427–32. doi: 10.1161/STROKEAHA.110.592766
36. Kwakkel G, Lannin NA, Borschmann K, English C, Ali M, Churilov L, et al. Standardized measurement of sensorimotor recovery in stroke trials: consensus-based core recommendations from the stroke recovery and rehabilitation roundtable. Int J Stroke. (2017) 12:451–61. doi: 10.1177/1747493017711813
37. Huppert TJ, Diamond SG, Franceschini MA, Boas DA. HomER: a review of time-series analysis methods for near-infrared spectroscopy of the brain. Appl Opt. (2009) 48:D280–98. doi: 10.1364/AO.48.00D280
38. Molavi B, Dumont GA. Wavelet-based motion artifact removal for functional near-infrared spectroscopy. Physiol Meas. (2012) 33:259–70. doi: 10.1088/0967-3334/33/2/259
39. Hocke LM, Oni IK, Duszynski CC, Corrigan AV, Frederick BD, Dunn JF. Automated processing of fNIRS data-A visual guide to the pitfalls and consequences. Algorithms. (2018) 11:67. doi: 10.3390/a11050067
40. Kocsis L, Herman P, Eke A. The modified Beer-Lambert law revisited. Phys Med Biol. (2006) 51:N91–8. doi: 10.1088/0031-9155/51/5/N02
41. Sassaroli A, Fantini S. Comment on the modified Beer-Lambert law for scattering media. Phys Med Biol. (2004) 49:N255–7. doi: 10.1088/0031-9155/49/14/N07
42. Ye JC, Tak S, Jang KE, Jung J, Jang J. NIRS-SPM: statistical parametric mapping for near-infrared spectroscopy. NeuroImage. (2009) 44:428–47. doi: 10.1016/j.neuroimage.2008.08.036
43. Aasted CM, Yücel MA, Steele SC, Peng K, Boas DA, Becerra L, et al. Frontal lobe hemodynamic responses to painful stimulation: A potential brain marker of nociception. PLoS ONE (2016) 11:e0165226. doi: 10.1371/journal.pone.0165226
44. Yücel MA, Selb J, Aasted CM, Lin PY, Borsook D, Becerra L, et al. Mayer waves reduce the accuracy of estimated hemodynamic response functions in functional near-infrared spectroscopy. Biomed Opt Express. (2016) 7:3078–88. doi: 10.1364/BOE.7.003078
45. Gagnon L, Cooper RJ, Yücel MA, Perdue KL, Greve DN, Boas DA. Short separation channel location impacts the performance of short channel regression in NIRS. Neuroimage. (2012) 59:2518–28. doi: 10.1016/j.neuroimage.2011.08.095
46. Yücel MA, Aasted CM, Petkov MP, Borsook D, Boas DA, Becerra L. Specificity of hemodynamic brain responses to painful stimuli: a functional near-infrared spectroscopy study. Sci Rep. (2015) 5:9469. doi: 10.1038/srep09469
47. Yücel MA, Selb J, Aasted CM, Petkov MP, Becerra L, Borsook D, et al. Short separation regression improves statistical significance and better localizes the hemodynamic response obtained by near-infrared spectroscopy for tasks with differing autonomic responses. Neurophotonics. (2015) 2:35005. doi: 10.1117/1.NPh.2.3.035005
48. Plichta MM, Herrmann MJ, Baehne CG, Ehlis A, Richter MM, Pauli P, et al. Event-related functional near-infrared spectroscopy (fNIRS): are the measurements reliable? Neuroimage. (2006) 31:116–24. doi: 10.1016/j.neuroimage.2005.12.008
49. Strangman G, Culver JP, Thompson JH, Boas DA. A quantitative comparison of simultaneous BOLD fMRI and NIRS recordings during functional brain activation. Neuroimage. (2002) 17:719–31. doi: 10.1006/nimg.2002.1227
50. Dotson VM, Duarte A. The importance of diversity in cognitive neuroscience. Ann N Y Acad Sci. (2020) 1464:181–91. doi: 10.1111/nyas.14268
51. Pelicioni PHS, Tijsma M, Lord SR, Menant J. Prefrontal cortical activation measured by fNIRS during walking: effects of age, disease and secondary task. PeerJ. (2019) 7:e6833. doi: 10.7717/peerj.6833
52. Stuart S, Vitorio R, Morris R, Martini DN, Fino PC, Mancini M. Cortical activity during walking and balance tasks in older adults and in people with Parkinson's disease: a structured review. Maturitas. (2018) 113:53–72. doi: 10.1016/j.maturitas.2018.04.011
53. Vitorio R, Stuart S, Rochester L, Alcock L, Pantall A. fNIRS response during walking — artefact or cortical activity? A systematic review. Neurosci Biobehav Rev. (2017) 83:160–72. doi: 10.1016/j.neubiorev.2017.10.002
54. Liu YC, Yang YR, Tsai YA, Wang RY, Lu CF. Brain activation and gait alteration during cognitive and motor dual task walking in stroke-A functional near-infrared spectroscopy study. IEEE Trans Neural Syst Rehabil Eng. (2018) 26:2416–23. doi: 10.1109/TNSRE.2018.2878045
55. Ross JM, Iversen JR, Balasubramaniam R. The role of posterior parietal cortex in beat-based timing perception: a continuous theta burst stimuation study. J Cogn Neurosci. (2018) 30:634–43. doi: 10.1162/jocn_a_01237
56. Holper L, Biallas M, Wolf M. Task complexity relates to activation of cortical motor areas during uni- and bimanual performance: A FUNCTIONAL NIRS study. Neuroimage. (2009) 46:1105–13. doi: 10.1016/j.neuroimage.2009.03.027
57. Lopes TM, Yasuda CL, Campos BM, de Balthazar MLF, Binder JR, Cendes F. Effects of task complexity on activation of language areas in a semantic decision fMRI protocol. Neuropsychologia. (2016) 81:140–8. doi: 10.1016/j.neuropsychologia.2015.12.020
58. Hermand E, Tapie B, Dupuy O, Fraser S, Compagnat M, Salle JY, et al. Prefrontal cortex activation during dual task with increasing cognitive load in subacute stroke patients: a pilot study. Front Aging Neurosci. (2019) 11:160. doi: 10.3389/fnagi.2019.00160
59. Ramchandran K, Zeien E, Andreasen NC. Distributed neural efficiency: intelligence and age modulate adaptive allocation of resources in the brain. Trends Neurosci Educ. (2019) 15:48–61. doi: 10.1016/j.tine.2019.02.006
60. Schultz DH, Cole MW. Higher intelligence is associated with less task-related brain network reconfiguration. J Neurosci. (2016) 36:8551–61. doi: 10.1523/JNEUROSCI.0358-16.2016
61. Neubauer AC, Fink A. Intelligence and neural efficiency. Neurosci Biobehav Rev. (2009) 33:1004–23. doi: 10.1016/j.neubiorev.2009.04.001
62. Al-Yahya E, Johansen-Berg H, Kischka U, Zarei M, Cockburn J, Dawes H. Prefrontal cortex activation while walking under dual-task conditions in stroke: a multimodal imaging study. Neurorehabil Neural Repair. (2016) 30:591–9. doi: 10.1177/1545968315613864
63. Mori T, Takeuchi N, Izumi SI. Prefrontal cortex activation during a dual task in patients with stroke. Gait Posture. (2018) 59:193–8. doi: 10.1016/j.gaitpost.2017.09.032
Keywords: functional near-infrared spectroscopy, stroke, gait, dual-task, posterior parietal cortex (PPC), sensorimotor cortex (SMC), pre-motor cortex (PMC), prefrontal cortex (PFC)
Citation: Lim SB, Peters S, Yang C-l, Boyd LA, Liu-Ambrose T and Eng JJ (2022) Frontal, Sensorimotor, and Posterior Parietal Regions Are Involved in Dual-Task Walking After Stroke. Front. Neurol. 13:904145. doi: 10.3389/fneur.2022.904145
Received: 25 March 2022; Accepted: 24 May 2022;
Published: 22 June 2022.
Edited by:
Samuel Stuart, Northumbria University, United KingdomReviewed by:
Michael K. Yeung, Hong Kong Polytechnic University, Hong Kong SAR, ChinaSoha Saleh, Kessler Foundation, United States
Copyright © 2022 Lim, Peters, Yang, Boyd, Liu-Ambrose and Eng. This is an open-access article distributed under the terms of the Creative Commons Attribution License (CC BY). The use, distribution or reproduction in other forums is permitted, provided the original author(s) and the copyright owner(s) are credited and that the original publication in this journal is cited, in accordance with accepted academic practice. No use, distribution or reproduction is permitted which does not comply with these terms.
*Correspondence: Janice J. Eng, Janice.eng@ubc.ca