- 1Department of Chemotherapy, The Second Hospital of Nanjing, Nanjing University of Chinese Medicine, Nanjing, China
- 2Department of General Surgery, The Second Hospital of Nanjing, Nanjing University of Chinese Medicine, Nanjing, China
Background: The immune system plays a crucial role in rectal adenocarcinoma (READ). Immune-related genes may help predict READ prognoses.
Methods: The Cancer Genome Atlas dataset and GSE56699 were used as the training and validation datasets, respectively, and differentially expressed genes (DEGs) were identified. The optimal DEG combination was determined, and the prognostic risk model was constructed. The correlation between optimal DEGs and immune infiltrating cells was evaluated.
Results: Nine DEGs were selected for analysis. Moreover, ADAMDEC1 showed a positive correlation with six immune infiltrates, most notably with B cells and dendritic cells. F13A1 was also positively correlated with six immune infiltrates, particularly macrophage and dendritic cells, whereas LGALS9C was negatively correlated with all immune infiltrates except B cells. Additionally, the prognostic risk model was strongly correlated with the actual situation. We retained only three prognosis risk factors: age, pathologic stage, and prognostic risk model. The stratified analysis revealed that lower ages and pathologic stages have a better prognosis with READ. Age and mRNA prognostic factors were the most important factors in determining the possibility of 3- and 5-year survival.
Conclusion: In summary, we identified a nine-gene prognosis risk model that is applicable to the treatment of READ. Altogether, characteristics such as the gene signature and age have a strong predictive value for prognosis risk.
Introduction
Rectal adenocarcinoma (READ) is a rare form of colorectal cancer with a high mortality rate worldwide. Patients with early READ who undergo radical surgery have a better prognosis. However, the prognosis for advanced READ is poor, which can endanger the patient’s life and result in death (Burton et al., 2006). Within 2 years, more than 80% of READ patients who experienced local recurrence underwent total mesorectal excision (Chen et al., 2017). Furthermore, after 75 months, no local recurrence of READ necessitating total mesorectal excision was observed (Chen et al., 2017). On average, the 5-year survival rate is described as 66.5% (Fazeli and Keramati 2015). Lymph node and pulmonary metastasis are common in READ, and both contribute to an unfavorable prognosis. Due to the lack of specific characteristics of early READ, early identification has become a considerable challenge. In clinical practice, approximately two-thirds of the READ patients are diagnosed at an advanced stage (Merchea et al., 2018). Typically, noticeable symptoms manifest when the tumor is typically in the middle or late stages. The occurrence of READ is widely believed to be a multistage and multigene process, and tumor occurrence and development of tumors are regulated by several genes (Chen et al., 2017).
Radiation therapy and chemotherapy are frequently used in conjunction with surgery to treat READ patients (Perez et al., 2017). However, these therapies can exacerbate patients’ immune problems. In recent years, immunotherapy as PD-1/PD-L1 immune checkpoints has demonstrated remarkable efficacy in various cancers, including READ (Hecht et al., 2016; Vareki et al., 2017). Tumor immunotherapy aimed to circumvent the tumor immune escape mechanism and awakens the immune cells that are capable of eradicating cancer cells. The immune cells and related genes may play a significant role in the infiltration process. Furthermore, this process has been detected in most human solid tumors, where READ lymphocytic infiltration conferred a survival advantage (Caputo et al., 2016). However, the microenvironment that can predict prognosis in READ remains unknown in terms of molecular events and tumor cell-immunocyte interaction.
Reliable biomarkers can be used to predict prognosis and overall survival. These biomarkers can be clinical variables, physiological or biochemical indicators, or molecular factors (Zhang Z. et al., 2018; Zhang et al., 2019). In recent years, researchers have examined the effect of gene expression levels on predicting survival prognosis for READ patients (Beer et al., 2002). However, most studies were limited by small sample sizes, insufficient evidence, or excessive data. With the development of cancer-specific databases, open and accessible databases such as The Cancer Genome Atlas (TCGA) (Zhu et al., 2018) and ImmPort (Bhattacharya et al., 2018; Sun et al., 2020) provide tremendous and valuable data for mining.
Thus, the gene expression level can be used to deduce specific molecular biological mechanisms underlying tumor occurrence and development. Investigating active and effective tumor markers at the genetic level opens up new treatment options for tumors. We analyzed the transcriptome expression level characteristics of READ samples from The Cancer Genome Atlas database and screened for immune-related genes in READ in this study. In addition, a model for READ disease survival prognostic risk prediction was developed using prognostic-related immune genes.
Materials and Methods
Data Source
RNA-seq data from READ patients at the fragments per kilobase million gene level with clinical information and produced by Illumina HiSeq 2000 RNA sequencing platform were downloaded as training datasets from TCGA websites using the genomic data commons data transfer tool (https://gdc-portal.nci.nih.gov/) before 19 October 2021. DEGs were detected using 158 READ tissues and nine normal controls. Supplementary File 1 contains the sample name obtained from TCGA. Simultaneously, the validation data set for GSE56699 was downloaded from the GEO database (http://www.ncbi.nlm.nih.gov/geo/) using the Illumina HumanHT-12 WG-DASL V4.0 R2 expression bead chip. We included the samples with genome-wide expression profile data and clinical prognostic information. Overall, a total of 61 samples were included.
Identification of Differentially Expressed Genes
The flowchart of this study is depicted in Supplementary Figure S1. The DEGs in the TCGA training data set were identified using the limma package version (v) 3.34.7 of R v3.6.1 (https://bioconductor.org/packages/release/bioc/html/limma.html) with a false discovery rate (FDR) of 0.05 and |log2 (fold change) | > 1. Then, a two-way hierarchical clustering analysis was performed using heatmap v1.0.8 in R v3.6.1 (https://cran.r-project.org/web/packages/pheatmap/index.html) on the DEG expression levels obtained in the training data set.
Functional Enrichment Analysis of Immune-Related Differentially Expressed Genes
Immune-related DEGs were downloaded for further analysis from the AmiGO 2 (http://amigo.geneontology.org/amigo) and KEGG databases (https://www.kegg.jp/). The function of these DEGs was then determined using the GO biology process and KEGG signal pathway enrichment using DAVID v6.8 (https://david.ncifcrf.gov/), with an FDR threshold of <0.05.
Construction and Evaluation of the Prognostic Risk Model
Univariate and multivariate Cox regression analyses were used to identify independent DEGs associated with overall survival (OS) using the survival package v2.41-1 of R v3.6.1 (http://bioconductor.org/packages/survivalr/). Significant DEGs were identified using a log-rank p-value threshold of <0.05.
The optimal DEGs combination was then determined using the LASSO Cox regression model in R v3.6.1 of penalized package v0.9.50 (https://cran.r-project.org/web/packages/penalized/index.html). The screening model’s optimal parameter “lambda” is obtained through 1,000 cross-validation likelihood algorithm calculation cycles. The following prognostic risk model was constructed using the prognostic coefficients from the LASSO Cox regression model and the DEG expression level:
Prognostic risk score = ∑βDEGs × ExpDEGs
Here, βDEGs denote the DEGs coefficient derived from the LASSO Cox regression model, whereas ExpDEGs denote the target DEGs expression level in the training dataset.
Evaluation of the Prognostic Risk Model
Each sample in the TCGA training and GSE56699 validation datasets was analyzed and assigned a prognostic risk score. The median value was used to classify the samples as high or low risk. In the TCGA training and GSE56699 validation datasets, the correlation between actual survival prognosis and that predicted by the prognostic risk model was evaluated using the Kaplan–Meier curve method in R v3.6.1 with the survival package v2.41-1GSE56699.
Correlation Analysis Between Prognostic-Related Differentially Expressed Genes and Different Immune Infiltrating Cells
The gene modules of the Tumor Immune Estimation Resource (TIMER; cistrome. shinyapps.io/timer/) were used to investigate the correlation between the expression of prognostic DEGs, and the abundance of six immune infiltrates (B cell, CD4+ T cell, CD8+ T cell, neutrophil, macrophage, and dendritic cell). A heatmap was generated using the partial correlation index for each DEG in each immune infiltrate and several scatterplots.
Screening of Independent Clinical Factors
Independent clinical factors such as age (years), gender, pathologic M (M0/M1/-), pathologic N (N0/N1/N2/-), pathologic T (T1/T2/T3/T4/-), pathologic stage (I/II/III/IV/-), history of colon polyps, lymphatic invasion, radiotherapy, prognostic model, death, and OS time (months) screened patients with READ in the TCGA training data set using univariate and multivariate Cox regression analyses with log-rank p-value <0.05 as the threshold. The analysis was stratified by age (>65 and ≤65 years of age) and pathologic stage (N0, N1, and N2).
Model Comparison
In order to evaluate the prognostic risk prediction model, stratified analysis was performed on the samples that were divided into different sample comparing groups. The nomogram displaying 3-year and 5-year OS was constructed to further reveal the correlation between independent factors and actual prognosis using the rms package v5.1-2 (https://cran.r-project.org/web/packages/rms/index.html) in R v3.6.1. survcomp version 1.34.0 (http://www.bioconductor.org/packages/release/bioc/html/survcomp.html) was used to calculate the C-index in R v3.6.1.
Results
Identification of Immune-Related Differentially Expressed Genes
There were 1,772 DEGs identified, with 768 upregulated and 1,004 downregulated across all genes (Figure 1A). From the heatmap, we observed the clustering of tumor and control samples clustered separately, ensuring the reliability of the original data (Figure 1B).
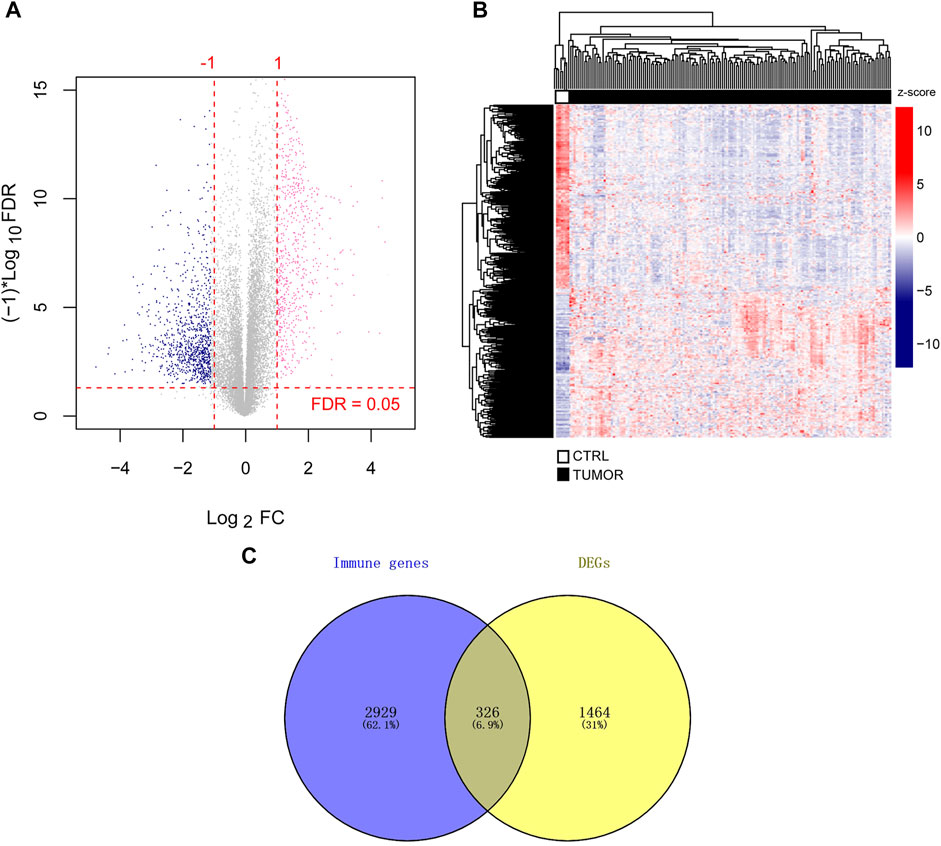
FIGURE 1. Identification of immune-related DEGs. (A) DEGs volcano map. The horizontal axis depicted the effect size (log2 FC), while the vertical axis depicted -log10 (FDR). The pink and blue dots represented DEGs that have been upregulated or downregulated, respectively. FDR <0.05 is indicated above the horizontal dashed line, and |log2 FC|>1 is indicated outside the two vertical dashed lines. (B) Heatmap of DEGs. (C) Immune-related genes and DEGs Venn diagram. FDR stands for false discovery rate and DEGs stand for differentially expressed genes. Fold change, FC.
Simultaneously, we downloaded 3,020 and 817 unique immune-related genes from the AmiGO two and KEGG databases, respectively, leaving 3,255 union immune-related genes. When TCGA DEGs were compared to immune-related genes, 326 immune-related DEGs were retained for further investigation (Figure 1C). We provided detailed information on 326 DEGs (log2 FC, p-value, and FDR) in Supplementary File 2.
Functional Enrichment Analysis of Immune-Related Differentially Expressed Genes
Additionally, we examined the functions of 326 DEGs using GO and KEGG analyses, identifying 36 BP and 22 KEGG under a <0.05 p-value (Figure 2; Table 1). The DEGs were mainly enriched in the GO term of the immune response, inflammatory response, chemokine-mediated signaling pathway, chemotaxis, innate immune response, positive regulation of the ERK1 and ERK2 cascades, response to lipopolysaccharide, adaptive immune response, positive regulation of transcription from the RNA polymerase II promoter, and positive regulation of cell proliferation (Figure 2A). Additionally, KEGG pathways also involved cytokine–cytokine receptor interactions; the chemokine signaling, Ras signaling, complement, coagulation cascades, cancer, Fc epsilon RI signaling pathways; natural killer cell-mediated cytotoxicity; leukocyte transendothelial migration; B cell receptor signaling pathway, and serotonergic synapse (Figure 2B).
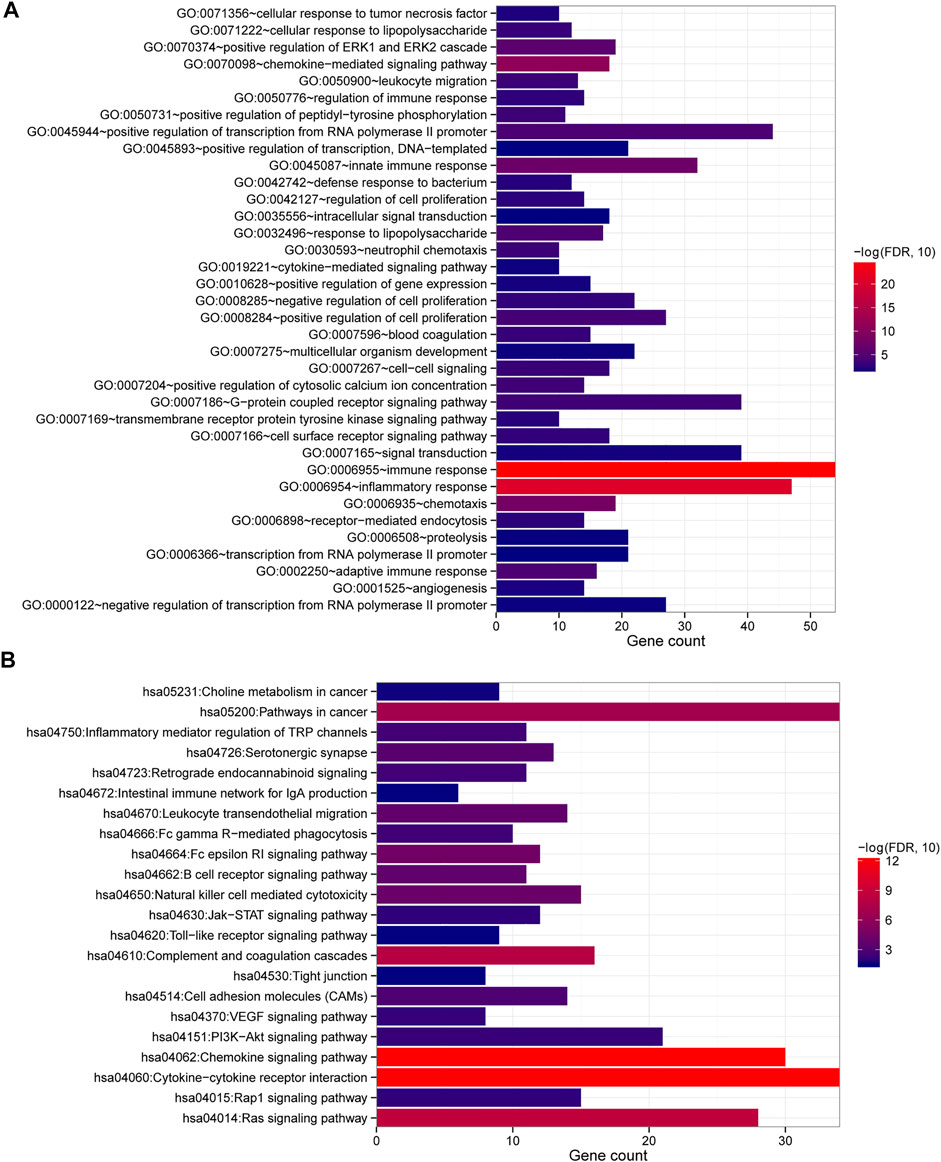
FIGURE 2. GO and KEGG analysis of DEGs. (A) Enriched GO terms with p values <0.05. (B) KEGG pathways were enriched with a p value of <0.05. The number of DEGs was represented by the horizontal axis, and the GO or KEGG items were represented by the vertical axis: the greater the significance, the closer the column color is to red.
Construction and Evaluation of the Prognostic Risk Model
The overall 326 DEGs were subjected to univariate Cox regression analysis, and 41 were identified as prognostic-related DEGs. Following the multivariate Cox regression analysis, a total of 22 DEGs remained. After that, nine optimal DEG combinations related to immunity were identified, including galectin 9C [LGALS9C; hazard ratio (HR) = 0.930, 95% confidence interval (CI) = 0.850–0.973], coagulation factor XIII A chain (F13A1; HR = 1.012, 95% CI = 1.004–1.554), ADAM-like decysin 1 (ADAMDEC1; HR = 0.987, 95% CI = 0.854–0.992), macrophage receptor with collagenous structure (MARCO; HR = 1.008, 95% CI = 1.002–1.504), L3MBTL histone methyl-lysine binding protein 1 (L3MBTL1; HR = 0.883, 95% CI = 0.803–0.970), solute carrier family 7 member 11 (SLC7A11; HR = 0.881, 95% CI = 0.814–0.954), UL16 binding protein 3 (ULBP3; HR = 0.884, 95% CI = 0.800–0.976), complement component 4 binding protein alpha (C4BPA; HR = 1.019, 95% CI = 1.001–1.458), and Cbp/p300 interacting transactivator with Glu/Asp rich carboxy-terminal domain 1 (CITED1; HR = 1.065, 95% CI = 1.008–1.125) (Table 2). The prognostic risk model was constructed based on LGALS9C, F13A1, ADAMDEC1, MARCO, L3MBTL1, SLC7A11, ULBP3, C4BPA, and CITED1 expression levels. Then, using the constructed model, the prognostic risk score of each sample was calculated as: prognostic risk score = (−0.2332) × ExpLGALS9C + 0.1177 × ExpF13A1 + (−0.1821) × ExpADAMDEC1 + 0.1287 × ExpMARCO+ (−0.4846) × ExpL3MBTL1+ (−0.6165) × ExpSLC7A11+ (−0.5122) × ExpULBP3+ 0.0929 × ExpC4BPA+ 0.3338 × ExpCITED1.
Correlation Analysis Between the Expression of Prognostic-Related DEGs and Six Immune Infiltrates
The highly expressed genes in tumor cells typically have positive associations with tumor purity. As such, we examined the association between prognosis DEG expression and six immune infiltrates (Supplementary Figure S2). ADAMDEC1 correlated positively with six immune infiltrates, particularly B cell and dendritic cell infiltrates (partial correlation = 0.421, p = 2.52e-07; Figures 3A,B). The results indicated that increased ADAMDEC1 expression was associated with a higher purity of READ tumor cells in B and dendritic cells. F13A1 also had positive correlation with six immune infiltrates, most notably macrophages (partial correlation = 0.423, p = 2.06e-07; Figures 3A,C) and dendritic cells (partial correlation = 0.598, p = 7.35e-15; Figures 3A,C). Apart from the B cells, LGALS9C had a negative correlation with immune infiltrates (Figure 3A).
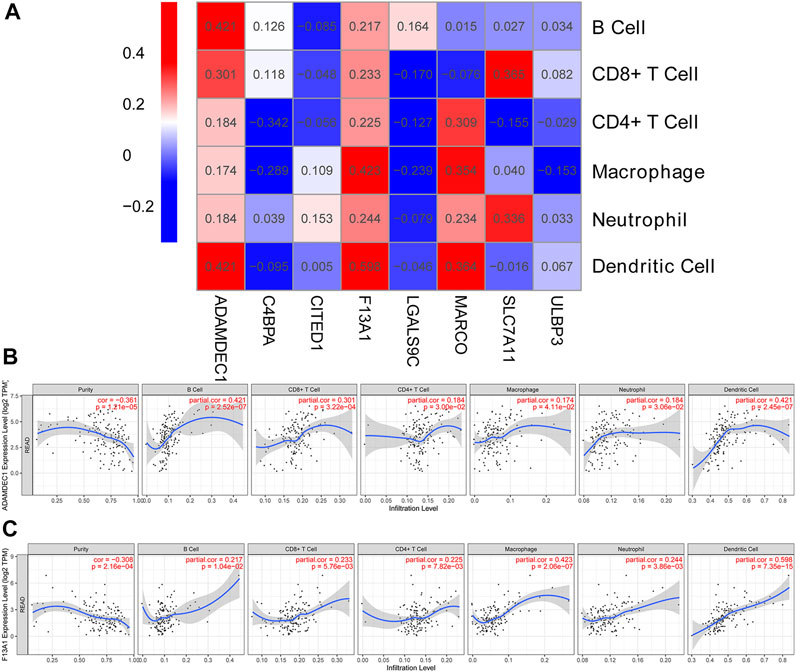
FIGURE 3. Correlation heatmap between DEGs and immune infiltration cells. (A) Heatmap of the correlation between DEGs and immune infiltration cells. (B,C) Scatter plots of the correlation between immune infiltration cells and ADAMDEC1 and F13A1 expression levels, respectively.
Evaluation of the Prognostic Risk Model
The prognostic risk model was evaluated by classifying samples into high- and low-risk groups in the TCGA training and GSE56699 validation datasets. Then, in two data sets, the high- and low-risk groups were compared according to their prognostic risk models to those classified according to their actual status. Both TCGA training [HR = 9.989 (3.382–29.50), p = 3.373e-07; Figure 4A] and GSE56699 validation datasets [HR = 8.428 (1.074–66.12), p = 8.077e-03; Figure 4B] revealed significant differences between high- and low-risk groups. Additionally, the prognostic risk model demonstrated a strong correlation with the actual situation in both the TCGA training [AUC = 0.906 (0.908, 0.893); Figure 4C] and GSE56699 validation datasets [AUC = 0.836 (0.860, 0.727); Figure 4D].
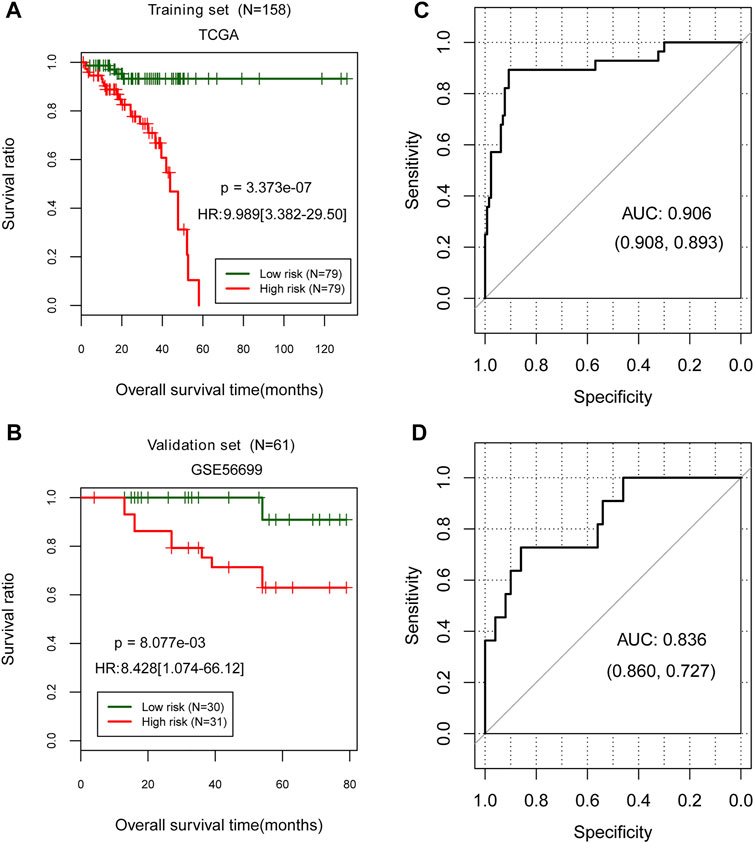
FIGURE 4. Evaluation of the prognostic risk model in TCGA training data set and GSE56699 validation dataset. (A,C) Kaplan–Meier curve method was used to evaluate a prognostic risk model in the TCGA training and GSE56699 validation datasets. The ROC curve of the prognostic risk model prediction results (B,D). Numbers in parentheses in the figure represent the ROC curve’s specificity and sensitivity.
Screening of Independent Clinical Factors
Univariable Cox regression analysis was used to eliminate independent clinical prognosis factors such as age (p = 7.97e-05), pathologic M (p = 2.30e-03), pathologic N (p = 4.61e-03), pathologic T (p = 3.07e-02), pathologic stage (p = 1.03e-03), and prognostic model (p = 3.37e-07) (Table 3). After multivariable Cox regression analysis, only three factors were retained for further investigation: age (p = 1.27e-03), pathologic stage (p = 4.91e-02), and prognostic risk model (p = 4.88e-03; Table 3).
The stratified analysis based on age (>65 and ≤65 years old) revealed that patients aged 65 years and older had a significantly lower survival rate [HR = 3.812 (1.537–9.449), p = 1.409e-03; Figure 5A]. According to the risk score proposed above, the samples in each subgroup were divided into low- and high-risk groups. In patients ≤65 years of age, those with high-risk scores had a significantly shorter OS time than those with low-risk scores [HR = 5.522 (1.926–22.43), p = 2.846e-04; Figure 5B]. In patients over the age of 65, those with a high-risk score had a significantly shorter OS time than those with a low-risk score [HR = 6.190 (2.073–18.49), p = 1.595e-04; Figure 5C].
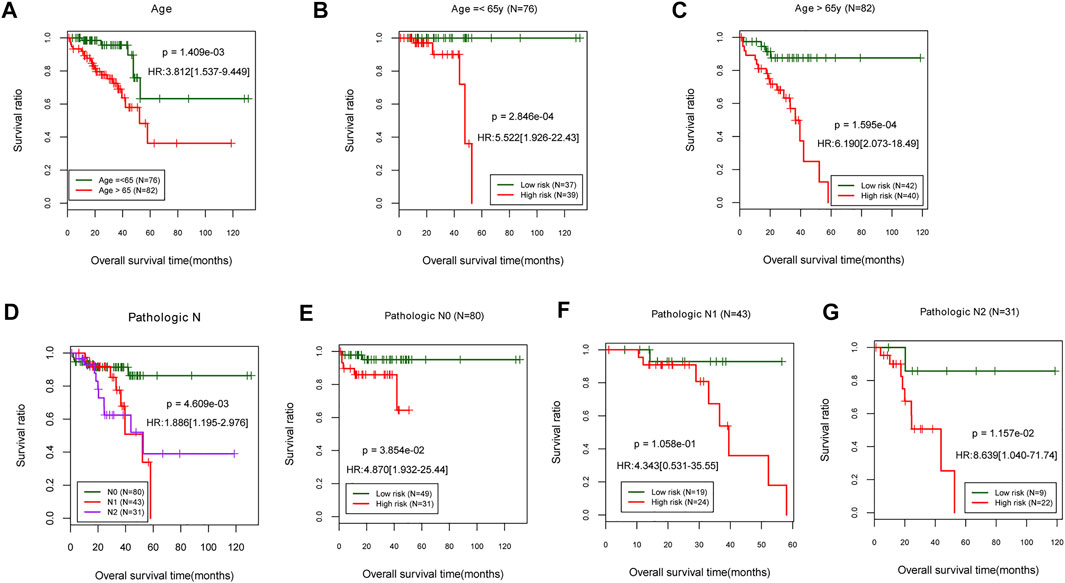
FIGURE 5. Stratified analysis on age and pathologic. (A) Age-related prognostic Kaplan–Meier curve. (B,C) Prognosis-related Kaplan–Meier curves in TCGA samples for patients aged 65 and younger. (D) Prognostic-related Kaplan–Meier curve of pathologic stage. The pathologic stages N0, N1, and N2 are represented in TCGA sample’s prognosis-related Kaplan–Meier curve chart (E–G). TCGA, The Cancer Genome Atlas.
The stratified analysis based on pathologic stage (N0, N1, and N2) revealed that patients with a higher pathologic stage [N1, N2; HR = 1.886 (1.195–2.976), p = 4.609e-03; Figure 5D] had a significantly lower survival rate than those who were (N0). The patients with low-risk scores at pathologic N0 have a significantly shorter OS time than patients with high-risk scores [HR = 4.870 (1.932–25.44), p = 3.854e-02; Figure 5E]. There were no significant differences in OS times between pathologic N1 and N2 patients classified as high- or low-risk (Figures 5F,G). The results indicated that a lower age (≤65 years) and a more advanced pathologic stage are associated with a better prognosis for READ patients, consistent with their actual status.
Model Comparison
The survival nomogram model analysis of TCGA training dataset samples revealed that age and mRNA prognostic factors were the most significant predictors of 3- and 5-year survival (Figure 6A). The 3-year (C-index = 0.759) and 5-year (C-index = 0.724) survival probabilities predicted by the model were generally consistent with actual survival rates (Figure 6B).
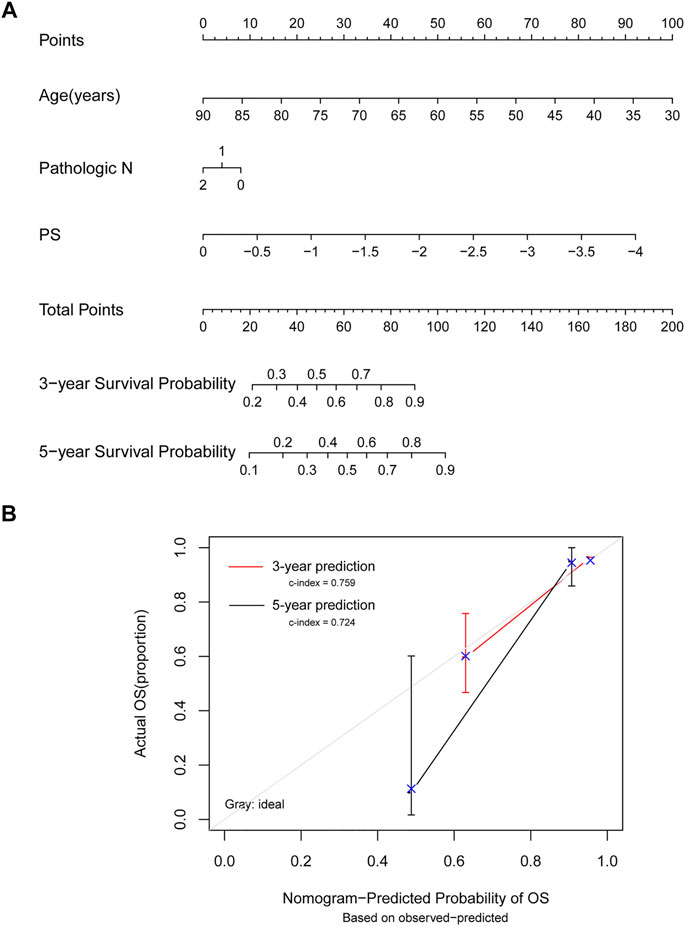
FIGURE 6. Model comparison analyses. (A) Nomogram survival rate prediction model for independent prognostic factors. (B) A 3-year and 5-year survival rate prediction line graph and an actual survival rate consistency line graph. The horizontal axis shows the predicted OS rate, the vertical axis shows the actual OS rate, and the red and black lines show 3- and 5-year predicted line graphs, respectively.
Discussion
Age, gender, and TNM stage are frequently used as prognostic factors in most cancers, including READ. Nevertheless, the high heterogeneity and limited predictive capacity of READ necessitate the inclusion of additional prognosis biomarkers. TCGA has recently provided robust data support for data reanalysis. The use of mRNA signatures can help accelerate the development and application of tumor-specific diagnostic technology, aid in the development of anti-tumor biologics at the genetic level and provide new avenues for tumor treatment. The immune system plays a critical role in the development of all cancers. The local interactions between tumor cells and immune cells, and endothelial and stromal cells, have been shown to have both pro- and anti-tumor effects (Braun et al., 2019). Therefore, we focused on immune-related genes in READ. This study sought to determine the effect of immune-related genes on the prognosis of READ and observed their expression in immune cells.
The present study identified a nine-gene immune-related mRNA signature biomarker. These genes LGALS9C, F13A1, ADAMDEC1, MARCO, L3MBTL1, SLC7A11, ULBP3, C4BPA, and CITED1 were included in the READ prognosis risk model. As an isoform of LGALS9, LGALS9C is a class of several eosinophil chemoattractants produced by activated T cells (Sato et al., 2002). Notably, these chemoattractants had previously been identified at multiple immune checkpoints (Huang X. et al., 2020). Also, it was discovered that this checkpoint was involved in the prognosis and therapeutic efficacy of READ in the current study. Additionally, unlike the B cells, LGALS9C exhibited a negative correlation with immune infiltrates. High LGALS9 scores were found in every immune subtype, although they were higher in the immune-rich tumors (Alame et al., 2021).
According to Luo et al. (2020), F13A1 alters the immune response and increases the risk of postoperative recurrence in cancers. When combined with BAMBI and LCN2, F13A1 demonstrated superior prognostic properties than when it is used alone (Luo et al., 2020). Additionally, it has been implicated in the development and progression of cancer (Vairaktaris et al., 2007). F13A1 has also been linked to lung cancer (Gao et al., 2019). In this study, F13A1 was identified as an important immune-related gene with a positive correlation to six immune infiltrates, particularly macrophage and dendritic cells. As previously reported, F13A1 inhibits preadipocyte proliferation by downregulating the downstream proliferative signaling pathways and defaulting to hypertrophic adipocyte differentiation profiles as an antagonistic.
ADAMDEC1 is a unique metazinc metalloprotease belonging to the A disintegrin and metalloproteases (ADAMs) family. Furthermore, the studies revealed that ADAMDEC1, which is required for the interaction of dendritic cells and germinal center T-helper cells (Fritsche et al., 2003), was involved in protein metabolism and cell adhesion during preoperative radiotherapy for rectal cancer (Supiot et al., 2013). Additionally, it has been associated with a variety of inflammatory diseases, including atherosclerosis (Papaspyridonos et al., 2006), pulmonary sarcoidosis (Papaspyridonos et al., 2006), osteoarthritis (Papaspyridonos et al., 2006), Crohn’s disease (de Bruyn et al., 2014), gastric adenocarcinoma (Pasini et al., 2014), and colorectal cancer (Macartney-Coxson et al., 2008). Additionally, we discovered a positive correlation between ADAMDEC1 and six immune infiltrates, most notably B and dendritic cells. On binding to PU.1, ADAMDEC1 expression can be regulated in activated dendritic cells and macrophages. The macrophages and B cells express PU.1, which is required for myeloid cell differentiation (Klemsz et al., 1990; Valledor et al., 1998). As reported by (Tong et al., 2021), high ADAMDEC1 expression was significantly correlated with better prognosis. MARCO was also identified as one of six diagnostic and prognostic biomarkers for patients with lung adenocarcinoma (Shang et al., 2017). However, to our knowledge, no previous studies have established MARCO as a READ biomarker. In addition, L3MBTL1 has been identified as a prognosis gene associated with a low risk of recurrence in low-grade, hormone receptor-positive tumors (Wismar et al., 1995). Moreover, we combined L3MBTL1 with hsa-miRNA-595 and lncRNA RP11-909B2.1 to develop a viable biomarker panel for colorectal cancer diagnosis and prognosis.
As shown in our present study, L3MBTL1 functions as both a biomarker for colorectal cancer and READ. Similarly, our previous study demonstrated that overexpressed SLC7A11 was validated as an oncogene in hepatocellular carcinoma (Zhang L. et al., 2018). Additionally, it has been suggested that it may be a prognostic gene in hepatocellular carcinoma (Yue et al., 2019). The previous reports also stated that CITED1 is correlated with lymph node metastasis in CRC patients, suggesting that it may be used to predict the presence of lymph node metastasis (Nasu et al., 2013). Furthermore, CITED1 knockdown can lead to decreased cellular proliferation and modulation of several genes (Rogers et al., 2016). Few studies have examined the effects of ULBP3 or C4BPA on the prognosis of READ. Most of these nine genes were associated with prognosis or cancer, and none were identified as READ biomarkers. Our present study revealed a new perspective on READ, which may play an important role in READ prognoses.
Likewise, Zuo et al. (2019) identified a six-gene signature (EPHA6, TIMP1, IRX6, ART5, HIST3H2BB, and FOXD1) for predicting the prognosis of READ, while (Huang W. et al. (2020) identified a novel mRNA panel for READ prognosis prediction and risk stratification. However, these studies did not examine the relationship between the pathogenesis or progression of READ and the immune system, which may be critical in treatment. Thus, identifying novel and meaningful biomarkers associated with immune-related genes is crucial for the prognosis and treatment of READ patients (Zhang et al., 2015a; Zhang, et al., 2015b). Our present study focused on the immune-related genes and identified significant biomarkers for prognosis prediction.
In summary, we identified an immune-related prognosis risk model that may be useful in the treatment of READ.
Data Availability Statement
The original contributions presented in the study are included in the article/Supplementary Material, further inquiries can be directed to the corresponding author.
Author Contributions
LJ and QW were responsible for drafting the manuscript, as well as the acquisition, analysis, and interpretation of data. PW and MS collected, analyzed, and interpreted the data. LY and QW contributed to the conception and design of the current study. All authors read and approved the final manuscript.
Conflict of Interest
The authors declare that the research was conducted in the absence of any commercial or financial relationships that could be construed as a potential conflict of interest.
Publisher’s Note
All claims expressed in this article are solely those of the authors and do not necessarily represent those of their affiliated organizations, or those of the publisher, the editors, and the reviewers. Any product that may be evaluated in this article, or claim that may be made by its manufacturer, is not guaranteed or endorsed by the publisher.
Supplementary Material
The Supplementary Material for this article can be found online at: https://www.frontiersin.org/articles/10.3389/fgene.2022.880945/full#supplementary-material
Supplementary Figure S1 | Flowchart of this study.
Supplementary Figure S2 | Association between prognosis DEG expression and six immune infiltrates.
References
Alame, M., Cornillot, E., Cacheux, V., Rigau, V., Costes-Martineau, V., Lacheretz-Szablewski, V., et al. (2021). The Immune Contexture of Primary central Nervous System Diffuse Large B Cell Lymphoma Associates with Patient Survival and Specific Cell Signaling. Theranostics 11 (8), 3565–3579. doi:10.7150/thno.54343
Beer, D. G., Kardia, S. L. R., Huang, C.-C., Giordano, T. J., Levin, A. M., Misek, D. E., et al. (2002). Gene-expression Profiles Predict Survival of Patients with Lung Adenocarcinoma. Nat. Med. 8 (8), 816–824. doi:10.1038/nm733
Bhattacharya, S., Dunn, P., Thomas, C. G., Smith, B., Schaefer, H., Chen, J., et al. (2018). ImmPort, toward Repurposing of Open Access Immunological Assay Data for Translational and Clinical Research. Sci. Data 5 (1), 180015–180019. doi:10.1038/sdata.2018.15
Braun, L. H., Baumann, D., Zwirner, K., Eipper, E., Hauth, F., Peter, A., et al. (2019). Neutrophil-to-Lymphocyte Ratio in Rectal Cancer-Novel Biomarker of Tumor Immunogenicity during Radiotherapy or Confounding Variable? Ijms 20 (10), 2448. doi:10.3390/ijms20102448
Burton, S., Norman, A. R., Brown, G., Abulafi, A. M., and Swift, R. I. (2006). Predictive Poor Prognostic Factors in Colonic Carcinoma. Surg. Oncol. 15 (2), 71–78. doi:10.1016/j.suronc.2006.08.003
Caputo, D., Caricato, M., Coppola, A., La Vaccara, V., Fiore, M., and Coppola, R. (2016). Neutrophil to Lymphocyte Ratio (NLR) and Derived Neutrophil to Lymphocyte Ratio (D-NLR) Predict Non-responders and Postoperative Complications in Patients Undergoing Radical Surgery after Neo-Adjuvant Radio-Chemotherapy for Rectal Adenocarcinoma. Cancer Invest. 34 (9), 440–451. doi:10.1080/07357907.2016.1229332
Chen, C.-H., Hsieh, M.-C., Hsiao, P.-K., Lin, E.-K., Lu, Y.-J., and Wu, S.-Y. (2017). A Critical Reappraisal for the Value of Tumor Size as a Prognostic Variable in Rectal Adenocarcinoma. J. Cancer 8 (10), 1927–1934. doi:10.7150/jca.17930
de Bruyn, M., Machiels, K., Vandooren, J., Lemmens, B., Van Lommel, L., Breynaert, C., et al. (2014). Infliximab Restores the Dysfunctional Matrix Remodeling Protein and Growth Factor Gene Expression in Patients with Inflammatory Bowel Disease. Inflamm. Bowel Dis. 20 (2), 339–352. doi:10.1097/01.mib.0000438430.15553.90
Fazeli, M. S., and Keramati, M. R. (2015). Rectal Cancer: a Review. Med. J. Islam Repub Iran 29, 171.
Fritsche, J., Muller, A., Hausmann, M., Rogler, G., Andreesen, R., and Kreutz, M. (2003). Inverse Regulation of the ADAM-Family Members, Decysin and MADDAM/ADAM19 during Monocyte Differentiation. Immunology 110 (4), 450–457. doi:10.1111/j.1365-2567.2003.01754.x
Gao, B., Zhao, Y., Li, Y., Liu, J., Wang, L., Li, G., et al. (2019). Prediction of Driver Modules via Balancing Exclusive Coverages of Mutations in Cancer Samples. Adv. Sci. 6 (4), 1801384. doi:10.1002/advs.201801384
Hecht, M., Büttner-Herold, M., Erlenbach-Wünsch, K., Haderlein, M., Croner, R., Grützmann, R., et al. (2016). PD-L1 Is Upregulated by Radiochemotherapy in Rectal Adenocarcinoma Patients and Associated with a Favourable Prognosis. Eur. J. Cancer 65, 52–60. doi:10.1016/j.ejca.2016.06.015
Huang, W., Li, G., Wang, Z., Zhou, L., Yin, X., Yang, T., et al. (2020). "A Ten-N6-Methyladenosine (m6A)-Modified Gene Signature Based on a Risk Score System Predicts Patient Prognosis in Rectum Adenocarcinoma." 10.
Huang, X., Tang, T., Wang, X., Bai, X., and Liang, T. (2020). Calreticulin Couples with Immune Checkpoints in Pancreatic Cancer. Clin. Translational Med. 10 (1), 36–44. doi:10.1002/ctm2.10
Klemsz, M. J., McKercher, S. R., Celada, A., Van Beveren, C., and Maki, R. A. (1990). The Macrophage and B Cell-specific Transcription Factor PU.1 Is Related to the Ets Oncogene. Cell 61 (1), 113–124. doi:10.1016/0092-8674(90)90219-5
Luo, Z., Chen, X., Zhang, Y., Huang, Z., Zhao, H., Zhao, J., et al. (2020). Development of a Metastasis-Related Immune Prognostic Model of Metastatic Colorectal Cancer and its Usefulness to Immunotherapy. Front. Cel. Dev. Biol. 8, 577125. doi:10.3389/fcell.2020.577125:
Macartney-Coxson, D. P., Hood, K. A., Shi, H. J., Ward, T., Wiles, A., O'Connor, R., et al. (2008). Metastatic Susceptibility Locus, an 8p Hot-Spot for Tumour Progression Disrupted in Colorectal Liver Metastases: 13 Candidate Genes Examined at the DNA, mRNA and Protein Level. BMC cancer 8 (1), 1–11. doi:10.1186/1471-2407-8-187
Maleki Vareki, S., Garrigós, C., and Duran, I. (2017). Biomarkers of Response to PD-1/pd-L1 Inhibition. Crit. Rev. oncology/hematology 116, 116–124. doi:10.1016/j.critrevonc.2017.06.001
Merchea, A., Ali, S. M., Kelley, S. R., Duchalais, E., Alabbad, J. Y., Dozois, E. J., et al. (2018). Long-term Oncologic Outcomes of Minimally Invasive Proctectomy for Rectal Adenocarcinoma. J. Gastrointest. Surg. 22 (8), 1412–1417. doi:10.1007/s11605-018-3751-8
Nasu, T., Oku, Y., Takifuji, K., Hotta, T., Yokoyama, S., Matsuda, K., et al. (2013). Predicting Lymph Node Metastasis in Early Colorectal Cancer Using the CITED1 Expression. J. Surg. Res. 185 (1), 136–142. doi:10.1016/j.jss.2013.05.041
Papaspyridonos, M., Smith, A., Burnand, K. G., Taylor, P., Padayachee, S., Suckling, K. E., et al. (2006). Novel Candidate Genes in Unstable Areas of Human Atherosclerotic Plaques. Atvb 26 (8), 1837–1844. doi:10.1161/01.atv.0000229695.68416.76
Pasini, F. S., Zilberstein, B., Snitcovsky, I., Roela, R. A., Mangone, F. R. R., Ribeiro, U., et al. (2014). A Gene Expression Profile Related to Immune Dampening in the Tumor Microenvironment Is Associated with Poor Prognosis in Gastric Adenocarcinoma. J. Gastroenterol. 49 (11), 1453–1466. doi:10.1007/s00535-013-0904-0
Perez, K., Safran, H., and Sikov, W. (2017). Complete Neoadjuvant Treatment for Rectal Cancer. Am. J. Clin. Oncol. 40 (3), 283–287. doi:10.1097/COC.0000000000000149
Rogers, M. A., Kalter, V., Marcias, G., Zapatka, M., Barbus, S., and Lichter, P. (2016). CITED4 Gene Silencing in Colorectal Cancer Cells Modulates Adherens/tight junction Gene Expression and Reduces Cell Proliferation. J. Cancer Res. Clin. Oncol. 142 (1), 225–237. doi:10.1007/s00432-015-2011-5
Sato, M., Nishi, N., Shoji, H., Seki, M., Hashidate, T., Hirabayashi, J., et al. (2002). Functional Analysis of the Carbohydrate Recognition Domains and a Linker Peptide of Galectin-9 as to Eosinophil Chemoattractant Activity. Glycobiology 12 (3), 191–197. doi:10.1093/glycob/12.3.191
Shang, J., Song, Q., Yang, Z., Li, D., Chen, W., Luo, L., et al. (2017). Identification of Lung Adenocarcinoma Specific Dysregulated Genes with Diagnostic and Prognostic Value across 27 TCGA Cancer Types. Oncotarget 8 (50), 87292–87306. doi:10.18632/oncotarget.19823
Sun, Y.-L., Zhang, Y., Guo, Y.-C., Yang, Z.-H., and Xu, Y.-C. (2020). A Prognostic Model Based on the Immune-Related Genes in Colon Adenocarcinoma. Int. J. Med. Sci. 17 (13), 1879–1896. doi:10.7150/ijms.45813
Supiot, S., Gouraud, W., Campion, L., Jezéquel, P., Buecher, B., Charrier, J., et al. (2013). Early Dynamic Transcriptomic Changes during Preoperative Radiotherapy in Patients with Rectal Cancer: a Feasibility Study. Wjg 19 (21), 3249. doi:10.3748/wjg.v19.i21.3249
Tong, X., Qu, X., and Wang, M. (2021). A Four-Gene-Based Prognostic Model Predicts Overall Survival in Patients with Cutaneous Melanoma. Front. Oncol. 11, 400. doi:10.3389/fonc.2021.639874
Vairaktaris, E., Vassiliou, S., Yapijakis, C., Spyridonidou, S., Vylliotis, A., Derka, S., et al. (2007). Increased Risk for Oral Cancer Is Associated with Coagulation Factor XIII but Not with Factor XII. Oncol. Rep. 18 (6), 1537–1543. doi:10.3892/or.18.6.1537
Valledor, A. F., Borràs, F. E., Cullell-Young, M., and Celada, A. (1998). Transcription Factors that Regulate Monocyte/macrophage Differentiation. J. Leukoc. Biol. 63 (4), 405–417. doi:10.1002/jlb.63.4.405
Wismar, J., Löffler, T., Habtemichael, N., Vef, O., Geißen, M., Zirwes, R., et al. (1995). The Drosophila melanogaster Tumor Suppressor Gene Lethal(3)malignant Brain Tumor Encodes a Proline-Rich Protein with a Novel Zinc finger. Mech. Dev. 53 (1), 141–154. doi:10.1016/0925-4773(95)00431-9
Yue, C., Ren, Y., Ge, H., Liang, C., Xu, Y., Li, G., et al. (2019). Comprehensive Analysis of Potential Prognostic Genes for the Construction of a Competing Endogenous RNA Regulatory Network in Hepatocellular Carcinoma. Ott Vol. 12, 561–576. doi:10.2147/ott.s188913
Zhang, B., Cheng, B., Li, F.-S., Ding, J.-H., Feng, Y.-Y., Zhuo, G.-Z., et al. (2015a). High Expression of CD39/ENTPD1 in Malignant Epithelial Cells of Human Rectal Adenocarcinoma. Tumor Biol. 36 (12), 9411–9419. doi:10.1007/s13277-015-3683-9
Zhang, B., Song, B., Wang, X., Chang, X.-S., Pang, T., Zhang, X., et al. (2015b). The Expression and Clinical Significance of CD73 Molecule in Human Rectal Adenocarcinoma. Tumor Biol. 36 (7), 5459–5466. doi:10.1007/s13277-015-3212-x
Zhang, L., Huang, Y., Ling, J., Zhuo, W., Yu, Z., Luo, Y., et al. (2018). Overexpression of SLC7A11: a Novel Oncogene and an Indicator of Unfavorable Prognosis for Liver Carcinoma. Future Oncol. 14 (10), 927–936. doi:10.2217/fon-2017-0540
Zhang, X., Sun, X.-F., Shen, B., and Zhang, H. (2019). Potential Applications of DNA, RNA and Protein Biomarkers in Diagnosis, Therapy and Prognosis for Colorectal Cancer: a Study from Databases to AI-Assisted Verification. Cancers 11 (2), 172. doi:10.3390/cancers11020172
Zhang, Z., Wang, S., Ji, D., Qian, W., Wang, Q., Li, J., et al. (2018). Construction of a ceRNA Network Reveals Potential lncRNA Biomarkers in Rectal Adenocarcinoma. Oncol. Rep. 39 (5), 2101–2113. doi:10.3892/or.2018.6296
Zhu, X. F., Zhu, B. S., Wu, F. M., and Hu, H. B. (2018). DNA Methylation Biomarkers for the Occurrence of Lung Adenocarcinoma from TCGA Data Mining. J. Cel Physiol 233 (10), 6777–6784. doi:10.1002/jcp.26531
Keywords: rectum adenocarcinoma, mRNA signature, immune, immune infiltrate, prognosis
Citation: Jiang L, Wang P, Su M, Yang L and Wang Q (2022) Identification of mRNA Signature for Predicting Prognosis Risk of Rectal Adenocarcinoma. Front. Genet. 13:880945. doi: 10.3389/fgene.2022.880945
Received: 22 February 2022; Accepted: 14 April 2022;
Published: 18 May 2022.
Edited by:
Shibiao Wan, St. Jude Children’s Research Hospital, United StatesReviewed by:
Xing Niu, China Medical University, ChinaJingting Yu, Salk Institute for Biological Studies, United States
Copyright © 2022 Jiang, Wang, Su, Yang and Wang. This is an open-access article distributed under the terms of the Creative Commons Attribution License (CC BY). The use, distribution or reproduction in other forums is permitted, provided the original author(s) and the copyright owner(s) are credited and that the original publication in this journal is cited, in accordance with accepted academic practice. No use, distribution or reproduction is permitted which does not comply with these terms.
*Correspondence: Qingbo Wang, wangqingbo2021@163.com