Global value chain embeddedness, digital economy and green innovation—Evidence from provincial-level regions in China
- 1School of International Economics and Trade, Shanghai Lixin University of Accounting and Finance, Shanghai, China
- 2Institute of Digital Economy and Green Development, Chifeng University, Chifeng, China
- 3Department of Information Technology & Decision Sciences, Old Dominion University, Norfolk, VA, United States
- 4Global Value Chain Research Center, Zhejiang Gongshang University, Hangzhou, China
With globalization and digitalization, participating in Global Value Chain (GVC) and developing digital economy have had a profound impact, which transforms China’s economy into a green and innovative one. This paper studies the intrinsic influential mechanism of GVC embeddedness and digital economy on green innovation and proposes some research hypotheses. Based on panel data of 30 Chinese provinces from 2002 to 2016, we constructed some core indicators such as GVC embeddedness, digital economy and green innovation. The ordinary panel model and spatial panel model are used to empirically test the impact of GVC embeddedness and digital economy on China’s green innovation at the provincial level. The research findings are: First, GVC embeddedness and digital economy have significant promotion effects on green innovation. Second, the development of digital economy will not only directly promote green innovation, but also indirectly promote green innovation by effectively promoting the integration of provincial economy into GVC. The results of mediating effect test show that GVC embeddedness has a partial mediating effect in the influential mechanism of digital economy to promote green innovation. Third, GVC embeddedness and green innovation have significant spatial spillover effects. It indicates that Chinese provinces (cities1) have significantly promoted green innovation in neighboring provinces through many possible channels and mechanisms in the process of participating in GVC, and the robustness test shows the stability of the spatial spillover mechanism. The findings provide useful policy implications for China’s deeply participating in GVC, vigorously developing digital economy and promoting green innovation.
1 Introduction
In the era of globalization, China’s economic integration into Global Value Chains (GVC) has enabled high economic growth, which comes at a high price of resources and ecological environment. In fact, some studies have found that after considering the environmental cost, the promotion effect of Chinese enterprises’ participation in GVC on employment, labor income, scientific and technological innovation and comprehensive rate of return was greatly reduced, and even had a negative inhibitory effect(Wang et al., 2019; Wang et al., 2021a; Wang et al., 2022a), which shows the high environmental costs. Now, how to move from the original high pollution and high energy consumption to low-carbon, environmental protection and green development has become the focus of high-quality development. To this end, the central government of China has promoted green development and green innovation to a high level. In 2019, the National Development and Reform Commission and the Ministry of Science and Technology jointly published the “Guidance on Building a Market-Oriented Green Technology Innovation System”. The Two Sessions of the National People’s Congress (NPC) and the Chinese People’s Political Consultative Conference (CPPCC) in 2021 and 2022 both argued for accelerating green development, promoting green science and technology innovation, strengthening green industries, and doing a solid job in carbon peaking and carbon neutralization. Many studies have shown that green innovation helps to improve ecological and environmental efficiency(Beltran-Esteve and Picazo-Tadeo, 2015), industry productivity (Johnstone et al., 2017) and corporate financial performance(Tang et al., 2018; Wang et al., 2018), and plays a key role in solving ecological and environmental problems and high-quality development problems (Carrion-Flores et al., 2013; Wang et al., 2022b). With China’s increasing efforts in environmental protection, achieving carbon peak and carbon neutrality, the key to truly realizing the grand blueprint of green development lies in green innovation.
At present, there is no unified definition of green innovation, but it is generally agreed that green innovation is an innovative behavior that aims to achieve resource conservation and environmental protection based on novelty and value characteristics (Schiederig et al., 2012). Cooke, (2010) and Horbach, (2007) have combined green development with innovation systems, and have conducted green innovation research from various perspectives such as resource conservation and environmental protection. Green innovation has the unique property of “dual externality” of environment and innovation (Rennings, 2000). In a broad sense, if any behavior, which meets the characteristics of innovation, such as new technology and new knowledge, can achieve the goal of reducing environmental pollution or saving natural resources, it can be considered as green innovation. Currently, China is one of the fastest growing countries in terms of GVC participation. Wang et al. (2022) used WIOD data and ADB Multi-Regional Input Output Database (MRIO) to create new GVC participation indices of the top 3 countries of GDP (United States, China and Japan), where China has a higher GVC participation ratio, in terms of both the forward participation index and backward participation index of GVC than United States and Japan. At the same time, China’s digital economy has developed rapidly. According to statistics from China Academy of Information and Communications Technology (CAICT), China’s digital economy will reach 45.5 trillion yuan by 2021, accounting for about 39.8% of GDP. With the impact of the COVID-19 and global economic downturn, however, China’s digital economy still achieves a high growth rate of 9.7% in 2020, and the empowering effect of digital economy is increasingly visible (Hu. et al., 2022a). In this sense, the rapid development of GVC embeddedness and digital economy has become an engine for the development of green innovation in China. Therefore, it needs to study how GVC embeddedness and digital economy affect green innovation.
There are some research studying GVC embeddedness, digital economy, and their impact on green innovation. First, most of the existing literature used KWW method to calculate the GVC embeddedness index (Koopman et al., 2010), and the research found that GVC embeddedness help the formation of GVC (Hu. et al., 2021a; Hu et al., 2022b). Wang et al. (2021b) used WIOD database to study the relationship between developing countries’ participation in GVC, technological progress and environmental pollution. Their study found that if the degree of GVC embeddedness is lower than the threshold in developing countries, technological advances will cause increased pollution; Otherwise, technological progress can reduce emissions. Yang et al. (2022) reached a similar conclusion by using the data of 19 industries in China. Second, Studies have found that the development of the Internet can significantly reduce energy consumption and reduce environmental pollution (Ren et al., 2021; Ren et al., 2022). At the city level or at the firm level, some studies support the role of the digital economy in promoting green innovation(Cao et al., 2021; Zhang et al., 2022; Lin and Ma, 2022; Feng et al., 2022; Gelenbe and Caseau, 2015; Ma and Zhu, 2022; Martin and Rice, 2022; Wang and Chen, 2022). Also, studies have shown that the digital economy has a positive impact on the GVC embeddedness and GVC upgrading (Gonzalez and Jouanjean, 2017; Gopalan et al., 2022). Third, as to spatial spillover, Qian et al. (2022) confirmed the spatial spillover effect of GVC embeddedness on carbon dioxide emissions. Also, Peng et al. (2021), Li and Du, (2021), and Fang et al. (2022) all found the spatial relevance of green innovation from different spatial dimensions. Ren et al. (2022), Wang et al. (2022d), and Wu et al. (2021) found that the digital economy has significant spatial spillover effects on carbon emissions and green development, using provincial data and prefecture-level city data, respectively. Most of the existing studies focus on the relationship either between GVC embeddedness and environmental pollution or between digital economy and environmental pollution. However, there are few research studying the relationship among GVC embeddedness, digital economy, and green innovation. Moreover, the existing studies are mainly conducted at the national level or industry level, and most of the measurement methods of GVC embeddedness adopt the KWW method by Koopman et al. (2010). However, there are few calculations of GVC embeddedness at the regional level, and few studies on GVC embeddedness at a smaller spatial scale.
This paper firstly proposes a theoretical hypothesis on the influence mechanism among GVC embeddedness, digital economy and green innovation. Then, it conducts empirical testing, using the panel data of 30 Chinese provinces (including municipalities directly under the control of the central government) from 2002–20162. It shows that GVC embeddedness and digital economy have a significant contribution to green innovation, and that digital economy has a positive impact on green innovation, with GVC embeddedness as a mediating variable. In addition, our study confirms the existence of spatial spillover effects of GVC embeddedness and digital economy on green innovation. These findings have important policy implications as to how to develop green innovation in China.
The main contributions of this paper are as follows: First, the paper theoretically discusses the internal influence logic of GVC embeddedness and digital economy on green innovation, introduces the mediation effect model, and demonstrates the mediation effect of GVC embeddedness on green innovation. Second, a mixed method of the ordinary panel model and spatial panel model is used to reveal the spatial spillover effect of GVC embeddedness and digital economy on green innovation. It shows the spatial dependence of green innovation, and provides theoretical basis for promoting the coordinated development of green innovation in various regions of China. Third, the paper provides policy guidance for countries involved in GVC, digital transformation, and environmental protection.
The remainder of this paper is organized as follows. Section 2 provides the theoretical mechanism, and proposes research hypothesis; Section 3 describes methodology and data; Section 4 reports empirical results; Section 5 conducts the robustness test; Section 6 concludes the paper and provides policy recommendations.
2 Theoretical mechanism and research hypothesis
2.1 Impact of GVC embeddedness on green innovation
Green innovation focuses on emerging technologies that reduce consumption, reduce pollution, improve ecology, promote the construction of ecological civilization, and realize the harmonious coexistence of human and nature. Therefore, to a certain extent, green innovation is more challenging and difficult to achieve than general innovation. It is an upgraded or advanced version of general innovation, since it focuses more on green technologies or processes, green materials and products, and reuse of resources to achieve both economic benefits and environmental protection benefits.
The existing studies on GVC embeddedness and green innovation mainly focus on the impact of GVC embeddedness on the improvement of production efficiency and that of GVC embeddedness on environmental protection and green development. The influence mechanism of GVC embeddedness on production efficiency is mainly realized through two ways. One is the learning and knowledge spillover mechanism. In the process of participating in GVC, developing countries can achieve technological progress and leap in production efficiency through the path of “digestion-absorption-reinvention” (Hummels et al., 2001; Hu. et al., 2021a; Wang et al., 2021b). In addition, the technology diffusion or knowledge spillover enabled by the linkage of production networks between upstream and downstream enterprises (Hu. et al., 2021b) can enhance the production efficiency of the whole industry or region, thus realizing the advanced industrial structure (Ivarsson and Alvstam, 2010). The other is the competition mechanism. After an industry is embedded in the GVC division of labor system, it means competition and cooperation with upstream and downstream industries and similar industries in the global production network. As the market scale that it faces becomes larger (Kılıçaslan et al., 2021), there will be more competitors and competitive pressure at the same time. For a long time, China’s participation in the GVC division of labor has always been “two sides abroad”3. However, this model has to face the double squeeze of both sellers and buyers. It will eventually force Chinese enterprises to improve production technology and processes, upgrade equipment (Humphrey and Schmitz, 2002), drive industrial upgrading (Wang et al., 2022e), and improve the productivity of regional manufacturing industry. Many empirical studies have confirmed that GVC division of labor can either promote scientific and technological innovation or improve labor productivity (Amiti and Wei, 2009; Kılıçaslan et al., 2021).
The impact of GVC embeddedness on environmental protection and green development has also received attention from many researchers, but no consistent conclusions has been reached yet. Shi et al. (2022) found that participation in GVC generated great ecological benefits to the “Belt and Road” countries; Wang and Song, (2017) found that reverse outsourcing promoted green technology progress from the perspective of global supply chains. Other researchers found that after participating in GVC, pollutants will be transferred around the world through trade activities (Wang et al., 2020a; Zhong et al., 2021). Due to the differences in science and technology, and economic benefits among countries, the impact of GVC embeddedness on the environment is different among participating countries (Wang et al., 2020b; Jin et al. (2022).
According to the 2016 global Environmental Performance Index (EPI) report, China ranked the second from the bottom among 180 countries, becoming the worst affected area of haze. The developed countries are still ahead of China, in terms of environmental protection and green technology. China tends to import the obsoleted production capacity or processes of developed countries in GVC division of labor, and some production segments with high pollution and energy consumption are outsourced to China and other developing countries. As China’s position in the GVC division of labor continues to rise, the products produced in China are gradually moving from labor-intensive and pollution-intensive to technology-intensive and environment-friendly production segments. In other words, they shift from general innovation to green innovation. Based on the relationship between green innovation and general innovation, we argue that the influence mechanism and practical effects of GVC embeddedness on general innovation are also applicable to green innovation. So, we propose the first research hypothesis.
Hypothesis 1. GVC embeddedness helps promote green innovation.
2.2 Influence mechanism of digital economy on green innovation
The impact of digital economy on the environment has been confirmed by existing studies. Digital economy, represented by the Internet, can promote industrial upgrading and economic structural transformation (Bennett et al., 2007), and effectively reduce environmental pollution (Ren et al., 2022). The impact mechanism of digital economy on green innovation can be described in two ways. First, as to direct impact mechanisms, digital economy promotes green innovation through enhancing factor productivity and environmental benefits. On the one hand, digital economy improves economic efficiency. According to Schumpeter’s theory, the so-called innovation is to “establish a new production function”. In the era of digital economy, due to the widespread use of digital technologies, such as the Internet, big data, and cloud computing, digital factors have changed the resource allocation efficiency of traditional factors of production, and changed the production function and cost-benefit relationship of traditional factors (Murthy et al., 2021). It can effectively integrate innovation factors, such as talent, capital, technology, and knowledge in a short period of time, providing strong support for green technology innovation. At the same time, digital economy reduces the marginal cost of green technology innovation, by reducing the time lag of information transmission and solving the problem of information asymmetry. The characteristics of “sharing” and “openness” of data produce strong positive externalities of digital economy. They help break down the information and knowledge barriers among industries, enterprises and employees, and further contribute to the formation of knowledge spillover and green innovation ideas. On the other hand, digital economy promotes green innovation, in terms of environmental protection and resource conservation. From the perspective of data production, data has infinite reproducibility and ease of dissemination. As a result, digital economy tends to be environment-friendly, less dependent on natural resources, and will not destroy the ecological environment on a large scale. The rapid development of digital economy can squeeze traditional high-pollution and high-energy-consuming industries, promote enterprises to conduct green technology research and development, and realize the optimization and upgrading of industrial structure.
Second, as to indirect influence mechanism, digital economy influences GVC embeddedness, and thus enhances green innovation. In other words, GVC embeddedness plays a mediating role between digital economy and green innovation. Studies have found that the application of digital technologies, such as the Internet, enhances the willingness and effectiveness of enterprises to participate in the GVC division of labor (Lanz et al., 2018; Gopalan et al., 2022). Many studies have shown that digital economy contributes to the improvement of GVC position (Ardolino et al., 2018; Oliveira et al., 2021). Its main impact mechanisms are: optimizing the layout of GVC production division of labor through mechanisms, such as reducing resource mismatch, increasing proportion of highly skilled talents, and improving production efficiency (Guan and Guo, 2022); promoting international knowledge and technology spillover, which enhances enterprises’ technological innovation capacity and efficiency of achievement transformation, and accelerates the industrial structure layout to the upstream of GVC (Duraivelu, 2022; Zhang et al., 2022); improving the level of digital service, amplifying the promotion effect of application research investment on GVC’s status, and enhancing the positive feedback effect of basic research investment on GVC (Yang and Yi, 2021); due to the development of digital technology, the search cost of credit information has been greatly reduced (Spence, 2021), and the information asymmetry existing in almost all markets has been reduced to the lowest level. In this sense, the combination of data and human capital can create a higher return rate (Spence, 2021), help enterprises to participate in knowledge intensive and other high value-added production activities, and drive the upgrading of GVC status (Szalavetz, 2022).
Based on the analysis above, we propose the second research hypothesis.
Hypothesis 2. While digital economy has a direct positive impact on driving local green innovation, it will also have a mediating effect through GVC embeddedness, which will indirectly promote green innovation.
2.3 Spatial spillover effects of GVC embeddedness and digital economy on green innovation promotion
From the existing literature and economic practice, GVC embeddedness, digital economy and green innovation, as three types of economic activities, should comply with the “first law of geography”4.
In other words, the economic behavior of a certain region has a certain influence on the neighboring regions, which is the spatial spillover effect of economic activities. In the era of globalization, the embeddedness of regional economy in GVC not only means that the region has established extensive economic cooperation network with foreign countries, but also the spatial interaction among regions is essential, based on the need of factor flow and resource integration. It is a natural result to produce radiation effect on neighboring regions, i.e., the spatial spillover effect of GVC embeddedness, which is mainly reflected as “direct spillover effect of knowledge and technology” (Almeida and Kogut, 1999; Los and Verspagen, 2000), “competition effect” of neighboring regions on industrial upgrading in the region (Xu et al., 2022).
The most important advantages of digital economy are convenience and efficiency. Compared with traditional elements, data elements can overcome spatial and temporal constraints more easily, and combining digital economy with GVC can enhance the ability of economic activities to overcome regional barriers, greatly enhancing the breadth and depth of economic activities of economic agents across regions (Cong et al., 2021). Through Internet of Things, artificial intelligence, cloud computing, and other technologies, digital economy enables services that could not be traded, and unmet needs to be realized in a larger space. It thus promotes the faster flow of innovation elements among regions, and improves the efficiency of resource allocation. Existing literature uses urban panel data and provincial panel data for empirical studies, and the results show that digital economy has significant spatial spillover effects on regional innovation, total factor productivity, and green innovation (Ballestar et al., 2020; Pan et al., 2022; Shen et al., 2022; Ghazy et al., 2022).
As a resource-saving and environment-friendly innovation behavior (Schiederig et al., 2012), green innovation helps emission reduction and improvement of environmental quality (Peng et al., 2021). So, green innovation is characterized by the dual externalities of technology and environment. On the one hand, the developers of green technologies cannot benefit from the technologies exclusively, and bring positive externalities to the “free riders”. On the other hand, the environmental improvement effect enabled by the region, where the green technology innovation is developed, will often benefit the surrounding areas, thus bringing positive environmental externalities. Therefore, green innovation has the potential for spatial spillover. Fan and Xiao (2021) used SBM-DDF model, gravity model, and social network analysis model to analyze the spatial correlation network of green innovation in China, and the results showed that there are spatial effects in the correlation network of green innovation in China. Liu et al. (2021) used the data of green patent authorization to find that the green innovation of each province has spatial correlation and spillover effect. Most of the more developed eastern provinces in China, especially the provinces in the Yangtze River Delta, the Pearl River Delta, and the Bohai Rim region, have strong ability to absorb and control green innovation resources.
Based on the discussion above, we propose the third research hypothesis.
Hypothesis 3. GVC embeddedness and digital economy have positive spatial spillover effects on green innovation, and can promote the improvement of green innovation level in neighboring regions.
3 Methodology and data
3.1 Econometric methodology
3.1.1 Benchmark model
Based on the literature review and the above theoretical analysis, this paper first constructs an OLS econometric model to test the impact of GVC embeddedness and digital economy on green innovation. Considering the differences in provincial conditions and development stages among provinces, there may be factors that do not change over time between provinces, so a regional fixed-effect model is used. The econometric model is shown in Eq. 1, where
3.1.2 Influence mechanism: Mediating effect model
Both theoretical studies and empirical tests show that digital economy and GVC embeddedness contribute to the development of green innovation. According to the literature and theoretical hypotheses, among digital economy, GVC embeddedness, and green innovation, digital economy may have a direct effect on green innovation. It may also affect green innovation by influencing GVC embeddedness, which is used as a mediating variable. To verify the possible mediating effect among the three economic activities, the following model is proposed, drawing on the test method of mediating effect by Trivellas et al. (2015) and Zeng et al. (2022).
For Eqs (2–4), the variables are the same as Eq. (1). Eq. (2) focuses on the impact of digital economy on green innovation, and estimates the coefficient of the impact of digital economy on green innovation. Eq. (3) focuses on the impact of digital economy on GVC embeddedness, and examines how GVC embeddedness, as a mediating variable, is affected by digital economy. Eq. (4) is to estimate the impact of the two variables, GVC embeddedness and digital economy, on green innovation. According to the process of mediating effect test (Namazi and Namazi, 2016), Eq. (2) is the basic test model of mediating effect. If
3.1.3 Spatial econometric model
Considering that the green innovation of provincial regions has a certain spatial autocorrelation, we introduce a spatial econometric model to examine how provincial green innovation is influenced by neighboring provinces. Three types of commonly used spatial econometric models are constructed as follows:
In Eqs (5–7),
3.2 Variables and their measurement method
3.2.1 Degree of GVC embeddedness (GVC)
This paper adopts the calculation method of Upward et al. (2013) and Su and Shao (2017) to measure the degree of GVC embeddedness in 30 Chinese provinces (cities), based on customs microdata from 2002–2016. Since the customs database records every import and export transaction information of customs clearance enterprises in detail, it is possible to measure the degree of regional GVC embeddedness. First, the import and export trade data are matched with their production or consumption provinces to identify the production or consumption regions for each import and export trade. The three fields of import from consumption region or export from production region, enterprise address, and zip code are used in turn for identification. If the records are not successfully determined, they are identified based on the enterprise name, by using Baidu map positioning. If the transaction records are still not determined, they are removed. Second, it needs to identify imported intermediate goods. For processing trade, since all imported products need to be used to produce and process export products, the imported products can be regarded as intermediate products. For general trade, imported intermediate products need to be identified with the help of the comparison table of BEC and HS customs codes, and it is assumed that imported intermediate products are used in domestic sales and general trade exports in the same proportion. Finally, it needs to identify the actual import and export activities of each province (city). Due to the existence of intermediate trade agents, provinces (cities) may show excessive import or excessive export. To identify the actual import and export activities of each province (city), the paper adopts the method of Ahn et al. (2011). According to different trade modes, the proportion of import of intermediate products by intermediate trade agents in the total import of intermediate products is used to replace the proportion of intermediate products imported from intermediate trade agents in different trade modes of provinces (cities). Also, the proportion of export of intermediate trade agents in the total export is used to replace the proportion of export of intermediate trade agents in different trade modes of provinces (cities), and the intermediate trade agents shall be eliminated. Considering the trade modes and intermediate trade agents, the formula for calculating the degree of GVC embeddedness of each province (city) is as follows, using the idea of Hummels et al. (2001).
where the subscripts p and o denote processing trade and general trade, respectively.
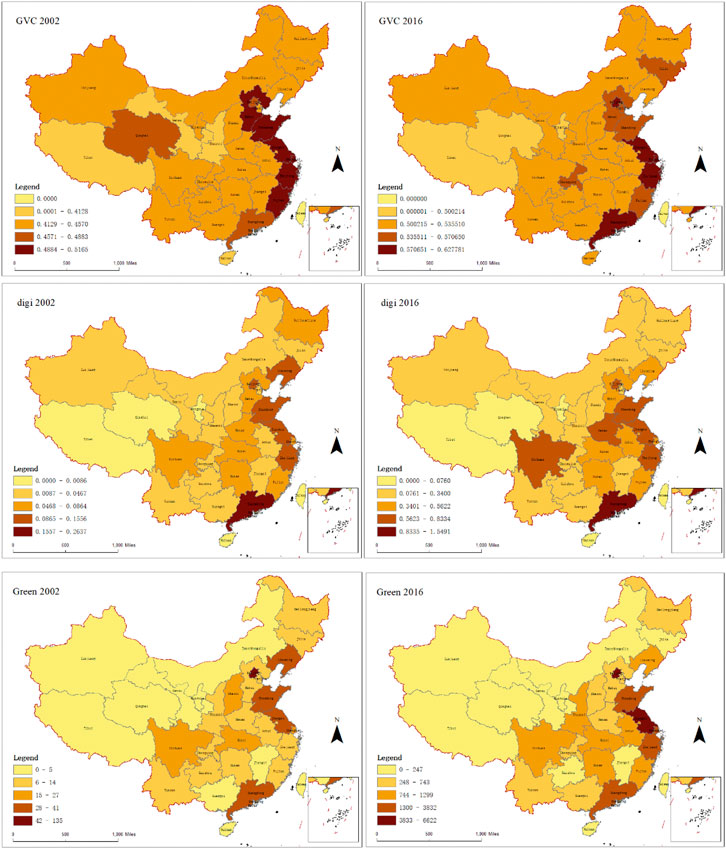
FIGURE 1. Geographic Distribution of GVC embeddedness, Digital Economy and Green Innovation in China in 2002 and 2016. Note: the data of Tibet, Hong Kong, Macao, and Taiwan are 0 due to the lack of data.
3.2.2 Digital economy (digi)
There is no direct data indicator for digital economy, and most of the existing literature uses different segmentation indicators. To measure the development of digital economy as accurately as possible, researchers have adopted different data indicators and statistical methods. Ren et al. (2021), Ren et al., 2022) constructed the evaluation system of digitalization development level from four dimensions of Internet popularization, Internet infrastructure, Internet information resources, and Internet applications. The EU Digital Economy and Society Index (European Commission. DESI, 2015) uses four main indicators: human capital, broadband connectivity, commercial application of digital technologies, and digital public services (Stavytskyy et al., 2019; Borowiecki et al., 2021). OECD measures the degree of digital economy development in terms of smart infrastructure investment, enhancing social dynamics, unleashing innovation and creativity, and promoting growth and employment (OECD, 2014). Wu and Yang, (2022) measured digital economy based on dimensions, such as digital infrastructure, digital innovation, and digital application. Based on the research above, the paper adopts digital infrastructure, digital talents, digital industry, digital services, etc. to calculate digital economy indicators. Considering the availability of data, the data used include five aspects: 1) The number of employees in information transmission, computer services, and software industry; 2) Number of Internet users; 3) Total telecommunication services; 4) Number of mobile phone users at the end of the year; 5) Total amount of postal services. The detailed description is shown in Table 1. The data period is from 2002–2016. Based on the five types of data, the paper uses Principal Component Analysis (PCA) to obtain provincial digital economy indicators. PCA uses the idea of dimension reduction to determine the weight, based on the characteristics of the data, and converts multiple variables into a few comprehensive variables (principal components). It can not only avoid the interference of subjective random factors, but also eliminate the differences in the magnitude of each variable, overcome the problem of missing information of a single variable, and simplify complex statistical data. The PCA calculation process is done with STATA software. The distribution of digital economy in each province is shown in Figure 1.
3.2.3 Green innovation (green)
The number of patent applications reflects the degree of expectation and protection consciousness of patent applicants to own patent assets, and can dynamically reflect the active degree of innovation. Patents need to pass rigorous examinations before they can be granted, so the number of patents granted can reflect the level of technological innovation in a region. So, the paper uses the number of patents granted for green technology inventions to characterize green innovation in each province (city). First, the International Classification of Green Patents (IPC) codes listed in the IPC GREEN INVENTORY5 provided by the World Intellectual Property Organization (WIPO) are determined. Second, using the green professional international classification codes, we obtain green patent data at both the city levels from the Chinese patent database developed by the State Intellectual Property Office, and at the provincial level by comparing patent types, IPC green classification codes, addresses of patent applicants, etc. The city-level data are summed up to obtain provincial-level green patent data. The green science and innovation database inside CCER economic and financial database and China’s annual science and technology statistical yearbook are employed to verify the data. The interpolation method is used to fill in some missing data. In fact, green invention patents are substantive innovations with higher innovation quality, whereas utility model patents are strategic innovations (Prud’homme, 2016). Therefore, the paper first uses the number of green invention patents granted for empirical research, and then uses the number of green utility model patents for robustness analysis. The distribution of green invention patents by provinces (cities) in China is shown in Figure 1.
3.2.4 Other control variables
According to existing literature, increasing R&D will help technological innovation and alleviate environmental pollution (Bai et al., 2019; Song et al., 2020). Marketization level (Zeng et al., 2021), foreign direct investment (Luo et al., 2021; Song and Han, 2022), environmental protection policy (Song et al., 2020; Hu. et al., 2021b), industrial structure (Du et al., 2021), and other variables have a promoting effect on green innovation. In addition to the core variables introduced above, the paper also includes other control variables that affect green innovation to reduce the endogenous problems that may be caused by missing variables. The main control variables include: 1) R&D investment (lnrd): measured by the logarithm of R&D expenditure in each province (city); 2) marketization level (market): a composite index of marketization extracted from Wang Xiaolu and Fan Gang’s China Marketization Index Report by Province (2018); 3) foreign direct investment (lnfdi): measured by the logarithm of the actual amount of foreign direct investment in the region; 4) environmental protection policy (ENV): expressed as the proportion of investment in environmental pollution control to GDP in each province; (5) industrial structure (struc), expressed as the proportion of tertiary industry output value to total output value.
3.3 Data sources and statistical description
The data sample of the paper is selected from 2002–20166, and includes 30 provinces and municipalities directly under the control of the central government of China, excluding Tibet, Hong Kong, Macao, and Taiwan, where data are not available. The data on the degree of GVC embeddedness comes from Import and Export Database of Chinese Customs Enterprises. Green innovation comes from China patent database developed by China National Intellectual Property Office. Digital economy, R&D investment, foreign direct investment, environmental protection policy, industrial structure, and other data come from EPS database of China National Bureau of Statistics. The per capita GDP data of each province used in the economic distance spatial weight matrix are mainly from the statistical yearbook of China and the statistical yearbook of each province (municipality). The spatial weight matrix of geographic distance is based on the geographic distance data of the provincial capitals. The data is presented by the Geoda software, using the 1:4 million electronic map, provided by the National Geographic Information System website. Table 2 shows the descriptive statistics results of the main variables. The results show that the mean value of green innovation (lnGreen) is 4.559, the maximum value is 8.798, the minimum value is 0, and the standard deviation is 1.732, indicating that the development of green innovation varies greatly among different provinces (cities) The degree of GVC embeddedness fluctuates between 0.339 and 0.628, with a mean of 0.478. The development gap of digital economy among provinces (cities) is large, showing the characteristics of “small mean value and large fluctuation”. The above descriptive statistical results indicate that the data are applicable to the empirical model.
4 Empirical results and discussion
4.1 Regression results of benchmark model
To alleviate the problem of heteroscedasticity and autocorrelation, we conducted heteroscedasticity and autocorrelation tests on the OLS model, and adopted the “heteroscedasticity and autocorrelation” robust standard error tests. The regression results of the benchmark OLS model are shown in Table 3. In models (1–6), the regression coefficients of GVC embeddedness and the development level of digital economy on the provincial green innovation have passed the significance test at the level of 1%. It indicates that the continuous deepening of the integration of Chinese provinces (cities) into GVC and the rapid development of digital economy have played a positive role in promoting green innovation. Hu et al. (2022c) divided green innovation into green product innovation and green process innovation, and the empirical study found that GVC embeddedness has a significant contribution to both. The findings of this paper are generally consistent with them. The findings of the above empirical study support research Hypothesis 1.
4.2 Analysis on the results of mediating effect test
The regression results of the mediating effect model are shown in Table 4. The regression coefficients
4.3 Spatial spillover effect test
4.3.1 Spatial autocorrelation test
Before analyzing the spatial spillover effect, this paper adopts the Exploratory Spatial Data Analysis (ESDA) method to test the global spatial autocorrelation of provincial green innovation (
In Eq. (9), N denotes the number of spatial units (provinces),
4.3.2 Results of SAR model and discussions
According to the screening process of Anselin spatial econometric model, through LM test, LR test, Wald test, and Hausman test, and by comparing the regression results of time fixed effect, spatial fixed effect, and spatiotemporal fixed effect, the paper adopts the spatial fixed effect and Spatial Autoregressive Model (SAR) for empirical analysis.
Table 6 reports the spatial econometric regression results of GVC embeddedness and digital economy on green innovation. The results in Table 6 show that the spatial autoregressive coefficient (rho) of green innovation in SAR model is significantly positive, indicating that the green innovation of each province (city) has a significant spatial spillover effect. It means that the green innovation of each province (city) is affected not only by the independent variables of each province (city) itself, but also by the green innovation of neighboring provinces (cities). The regression coefficients of the core variables GVC embeddedness and digital economy are significantly positive. Among the control variables, R&D investment, marketization level, and FDI have passed the significance test. Comparing the results in Table 6 with Table 3, it can be found that the regression results of the ordinary panel OLS model generally overestimate the impact of independent variables on green innovation. In Table 3, the impact coefficient of GVC embeddedness variable is 2.29–8.02, and after considering the spatial autocorrelation factor, the impact coefficient of GVC embeddedness on green innovation decreases to 1.39–1.48 (as shown in Table 6). The impact coefficient of digital economy decreases from 1.02–5.37 in Table 3 to 0.93–0.95 in Table 6. The impact coefficients of the control variables R&D investment, marketization level, industrial structure, etc. also show the decline, indicating that the impact coefficients of the independent variables are somewhat overestimated in the OLS panel model. The main reason for the phenomenon is that the ordinary panel model ignores the spatial spillover effect and considers each province (city) as an independent regional entity, which does not follow the theory and reality. In the real world, cross regional flows of commodities and factors among provinces are very common, and economic actions and government policies will have an impact on adjacent regions, which has contributed to the spatial spillover of green innovation among regions. So, the introduction of the spatial autoregressive model can provide a more accurate estimation of the influencing factors of green innovation.
According to the spatial econometric theory of Elhorst, (2014), when there is spatial spillover effect, the independent variable not only has an impact on green innovation in the province (city), but also has an impact on green innovation in the surrounding or neighboring regions, which enables a chain reaction through a circular feedback mechanism. Therefore, the influence of the independent variable on green innovation can be decomposed into direct effect and indirect effect. Among them, the total effect of the independent variable on the green innovation of a given province (city) is the direct effect, which includes two components. First, the effect of the independent variable on the green innovation of the province (city). Second, the independent variables have an impact on the green innovation of the surrounding or neighboring provinces (cities), and the green innovation of the neighboring regions in turn has an impact on the green innovation of the province (cities). This process is also called the spatial feedback effect. The impact of the independent variable on green innovation in the surrounding or neighboring provinces (cities) is the indirect effect, which is also referred to as the spatial spillover effect. As shown in Table 6, the spatial econometric results give the decomposition results of the direct and indirect effects of model (3). Overall, the main explanatory variables GVC embeddedness, digital economy, and control variables R&D investment and marketization level all pass the significance test, and their direct and indirect effects are positive. Thus, it can be seen that to develop green innovation, a province (city) should increase its own openness, continuously integrate into GVC, vigorously develop digital economy, increase R&D investment, and continuously improve the level of marketization. Also, it should focus on regional linkage, utilize the spatial spillover effect, and take advantage of the green innovation of neighboring provinces (cities). The above analysis and discussion further validate Hypotheses 1 and 3.
5 Robustness analysis
To test the reliability of the empirical test conclusion, we used the methods of replacing the dependent variable, replacing the spatial weight matrix, and analyzing the spatial heterogeneity in different regions to test the robustness of the OLS panel model and SAR model.
5.1 Substitution of dependent variable
The same method that used to obtain green invention data was used to obtain the number of green utility model patents granted at the provincial level. Green utility model patents (lnGreen02) were used to replace green inventions (lnGreen) to test the impact of GVC embeddedness and digital economy on green innovation through OLS panel model. The test results are shown in Table 7. GVC and digi in model (1) and model (2) passed the significance test at the level of 1%. Compared with Table 3, the regression coefficient is decreased slightly, indicating that GVC embeddedness and digital economy have a significant role in promoting green innovation, which is highly consistent with previous research conclusions. After the green utility model patent (lnGreen02) was used to replace the green invention (lnGreen), model (1) and model (2) in Table 4 become model (1) and model (2) in Table 7. The regression results passed the mediating effect test. It confirms that GVC embeddedness has partial mediating effect, and the mediating effect model has strong robustness. The rho values have passed the significance test in models (3–7), which verifies that green innovation has a significant spatial spillover effect.
5.2 Replacement of spatial weight matrix
In addition to the spatial weight matrix of economic distance used in the paper, spatial contiguity weight matrix and spatial distance weight matrix are also common spatial weight matrices used in spatial econometric analysis. In the paper, the spatial contiguity weight matrix uses the Rook contiguity rule, where
In addition to the SAR model test results based on the economic distance weight matrix reported in Table 6, the empirical results of spatial autoregressive models under various spatial weight matrices are reported in models (3–7) in Table 7. The rho values all pass the significance test at the 1% level, showing the spatial autocorrelation characteristics of green innovation. GVC embeddedness, digital economy all pass the significance test under all three types of spatial weight matrices, showing a significant positive correlation with provincial green innovation. Each unit increase in provincial GVC embeddedness will increase the level of green innovation by 47%–135%. Each unit increase in the level of digital economy will increase green innovation by 48%-96%. The above empirical results are consistent with the results in Table 6. These findings, validate the robustness of the previous findings, and confirm the importance of integrating GVC, developing digital economy, regional linkage of green innovation, and other policies to promote provincial green innovation.
5.3 Analysis of regional spatial heterogeneity
There are differences in geographical location, economic development, and policies among provinces in China. So, the role of participation in GVC and digital economy development in different regions is not completely consistent. To test the heterogeneity among regions, the paper uses the classification method of Liu et al. (2021), and classifies the country into three regions: the eastern, the central, and the western. The eastern region includes 11 provinces, i.e., Guangdong, Fujian, Zhejiang, Jiangsu, Shandong, Shanghai, Beijing, Tianjin, Hebei, Liaoning and Hainan. The central region includes 8 provinces, i.e., Hubei, Hunan, Henan, Anhui, Jiangxi, Shanxi, Jilin, and Heilongjiang. The western region includes 11 provinces, i.e., Xinjiang, Qinghai, Gansu, Ningxia, Yunnan, Guizhou, Sichuan, Shaanxi, Chongqing, Guangxi, and Inner Mongolia. Based on the above three groups of sample data, the SAR model and the economic distance weight matrix are used to determine the impact of GVC embeddedness and digital economy development on green innovation in three regions.
The regression results of SAR models about the three regions are reported in Table 8. The rho values in the three regions have passed the significance test, which indicates that green innovation in the three regions has significant spatial autocorrelation. As to the significance of GVC embeddedness, the significance gradually increases from the eastern, the central to the western. The regression coefficient gradually increases, which shows that participation in GVC has an increasing impact on green innovation from the eastern, central to western regions. As to digital economy, the three regions have passed the significance test at the level of 1%–10%, and the regression coefficient shows the reverse order, compared to GVC embeddedness. The above results show that there is spatial heterogeneity among the three regions. The reason is that there exist ladder differences in GVC embeddedness, digital economy development, and the overall level of economic development among the three regions.
Due to geographical location and other reasons, the level and openness of economic development of the eastern region are higher than those of the central and western regions. The eastern region was embedded in GVC earlier and more deeply than the central and western regions. With the rising cost of labor and other factors in the eastern region, the manufacturing industry continues to shift from the eastern to the central and western regions, and some low value-added industries also gradually shift to the central and western regions. The eastern region focuses on high value-added, green and high-tech industries. Therefore, the eastern region has gradually changed from copy-cat innovation through GVC to original innovation. In contrast, the central and western regions are in the stage of undertaking the eastern industrial transfer and embedding GVC to achieve low-level innovation. In this sense, for the central and western regions, embedding GVC to drive the development of some industries is still an important way to upgrade industries and promote green innovation. As to digital economy, the eastern region is also leading the central and western regions (see Figure 1). Compared with the central and western regions, the eastern region has more advantages in urban agglomeration (Ma and Zhu, 2022), high-tech talents (Wu and Yang, 2022), digital infrastructure, and other factors. The digital dividend that it enjoys may play an important role in promoting green innovation. It means that the central and western regions should increase efforts to integrate into GVC, develop digital economy, fill the digital gap between the eastern region, and promote the development of green innovation.
6 Conclusion and policy implications
6.1 Research conclusions
Through combing the existing literature, this paper theoretically studies the intrinsic influence mechanism of GVC embeddedness and digital economy on green innovation, and proposes three research hypotheses. Core indicators such as GVC regional embeddedness, digital economy, and green innovation are constructed by using China Customs micro database, China patent database developed by the China National Intellectual Property Administration, and EPS database from 2002 to 2016. Based on the ordinary panel model, and the Exploratory Spatial Data Analysis method (ESDA), a spatial panel model based on multiple spatial weight matrices is constructed to empirically test the impact of GVC embeddedness and digital economy on China’s green innovation at the provincial level. The research findings are as follows: First, GVC embeddedness and digital economy at the provincial level have significant promoting effects on green innovation. Second, the development of digital economy will not only directly promote green innovation, but also indirectly promote green innovation by effectively promoting the integration of provincial economy into GVC. The results of the mediating effect test indicate that there is a partial mediating effect of GVC embeddedness in the influential mechanism of the digital economy to promote green innovation. Third, green innovation and GVC embeddedness have significant spatial spillover effects. It indicates that in the process of participating in GVC, Chinese provinces (cities) have significantly promoted green innovation in surrounding provinces through many channels and mechanisms, such as product and factor flow, upstream and downstream industry linkages, competition effect or imitation effect, and technology spillover. The robustness test shows the stability of the spatial spillover mechanism.
6.2 Practical implications
From a global perspective, the “global supply chain”, “global industrial chain” and “global value chain” based on the division of labor of global value chain (GVC) have formed a “one body, three chains” system, which has become the basic support for economic interdependence and common development of countries in the global economic system for a long time, from now and in the future. Moreover, digital technologies continue to facilitate the formation and development of “global innovation chains”, making innovation activities cross national borders on a large scale and become a global system (Ambos et al., 2021). It can be said that integrating into GVC and accelerating the development of digital economy have become key drivers for the development of green innovation in China. Based on these findings, there are three important policy implications as follows.
First, increase the determination and strength to integrate into GVC, combine participation in GVC with industrial upgrading, and promote the development of green innovation. Under the current situation of counter-globalization and the COVID-19, the government should further strengthen its opening-up, rely on the “Belt and Road” initiative, speed up the transformation from policy based opening to institutional opening, especially in the central and western regions, further improve the business environment, improve the level of trade facilitation, gradually change from cost advantage to other competitive advantages, and strive to integrate into GVC at a high level. In the process of participating in GVC, the government should create a combination of industrial policies, environmental policies, and financial policies to promote the transformation of business entities to green, technology-intensive, and high value-added ones.
Second, improve data standards, facilitate data flow and sharing, and promote digital economy and GVC embeddedness to form a joint force. Further improve the standardization of data in collection, storage, security, and application, and establish and improve the digital standard systems in line with international standards. Improve the legislation of digital economy, clarify data ownership, use right and management right, remove the institutional and regulatory barriers when data flows, promote the openness and sharing of data within and across provinces, and create a good policy environment for the development of digital economy. Considering the development gap of provinces (cities), formulate and implement differentiated digital transformation policies, and solve the unequal application of digital technology among enterprises, industries and regions. Make full use of the platform of “Free Trade Zone” of all provinces (cities), create new regulatory rules about digital trade, and make digital technology an important driving force to promote provinces (cities) to integrate into GVC.
Third, the regional coordination and linkage of GVC embeddedness and digital economy development in each province (city) should be increased to fully tap its spatial spillover effect on green innovation development. In view of the spatial autocorrelation of GVC embeddedness and digital economy, we should break all kinds of visible and invisible barriers among regions, establish and improve the national market, promote the free flow of resources and factors, strengthen the economic linkage, policy linkage, and strategic linkage among provinces and regions. In terms of environmental protection policies and industrial access standards, all regions should do comprehensive planning, establish reasonable steps of environmental standards and green standards, exploit the diffusion effect and demonstration effect of green scientific and technological innovation among enterprises and industries in regions, and realize the whole upgrading of green innovation across the country.
6.3 Limitations and future work
The paper studies the impact mechanism of GVC embeddedness and digital economy on green innovation, and uses the data at the provincial level of China for empirical testing. However, the paper has some limitations that need to be addressed in future. First, as to the design of impact mechanism, the paper focuses on the impact mechanism of GVC embeddedness and digital economy on green innovation. In fact, there may be a two-way relationship between these variables. For example, green innovation may have an impact on GVC embeddedness and the development of digital economy. In addition, we did not consider the threshold effect of the panel model and the dynamic effect of the spatial econometric model in the empirical study. Second, the measurement of GVC embeddedness, digital economy, and other indicators needs to be further expanded. As to the measurement of GVC embeddedness at the provincial level, the paper adopts Import and Export Database of Chinese Customs Enterprises to calculate it. In future research, KWW method (Koopman et al., 2010) can be used to measure GVC indicators at the regional level. As to digital economy measurement, the data dimensions adopted in the paper are limited. In future research, we can take more data into account to further improve the rationality and accuracy of digital economy indicators. Third, the paper uses provincial level data, which is not fine-grained enough, leading to some variables at city level can’t be taken into account. In addition, due to the availability of data, the data used in this paper is limited to 2002–2016, and the data are limited and not new enough. In the future, city level data will be used for in-depth research.
Data availability statement
The raw data supporting the conclusion of this article will be made available by the authors, without undue reservation.
Author contributions
All the authors participated in the formulating, writing, and revising of this paper. HP and JY created and wrote the first manuscript. HZ and FH conceptualized and supervised the study. HP and JY collected the data and conducted the data analysis. HP, XZ, FH, and HZ revised and edited the manuscript. All authors read and approved the final manuscript.
Funding
This study is supported by the Major Program of the National Social Science Foundation of China (Grant Number 20&ZD124), National Social Science Foundation of China (Grant Number 22BJY017), the National Natural Science Foundation of China (Grant Number 71773115), and the Humanity and Social Science Foundation of Ministry of Education of China (Grant Number 21YJA790043).
Conflict of interest
The authors declare that the research was conducted in the absence of any commercial or financial relationships that could be construed as a potential conflict of interest.
Publisher’s note
All claims expressed in this article are solely those of the authors and do not necessarily represent those of their affiliated organizations, or those of the publisher, the editors and the reviewers. Any product that may be evaluated in this article, or claim that may be made by its manufacturer, is not guaranteed or endorsed by the publisher.
Footnotes
1(city) or (cities) in the paper refers to municipalities directly under the central government, i.e., Beijing, Shanghai, Tianjin, and Chongqing.
2Due to the lack of data on Tibet, Hong Kong, Macao, and Taiwan, this paper only uses data for 30 provinces and cities.
3Raw materials or semi-finished products are purchased from abroad, then manufactured into finished products, which are sold abroad again.
4W. Tobler (1970) proposed the “first law of geography”, that is, the correlation between features is related to the distance and height. Generally speaking, the closer the distance is, the greater the correlation between features is; The farther the distance, the greater the difference between the features.
5See website: http://www.wipo.int/classifications/ipc/en/est.
6The provincial GVC embeddedness data is calculated based on the customs micro database, which is updated to 2016. Some data of digital economy were only available from 2002, and earlier data were not available.
References
Ahn, J., Amit, K. K., and Wei, S. (2011). The role of intermediaries in facilitating trade. J. Int. Econ. 84 (1), 73–85. doi:10.1016/j.jinteco.2010.12.003
Almeida, P., and Kogut, B. (1999). Localization of knowledge and the mobility of engineers in regional networks. Manag. Sci. 45 (7), 905–917. doi:10.1287/mnsc.45.7.905
Ambos, B., Brandl, K., Perri, A., Scalera, V. G., and Assche, A. V. (2021). The nature of innovation in global value chains. J. World Bus. 56 (4), 101221. doi:10.1016/j.jwb.2021.101221
Amiti, M., and Wei, S. J. (2009). Service offshoring and productivity: Evidence from the U.S. World Econ. 32 (2), 203–220. doi:10.1111/j.1467-9701.2008.01149.x
Ardolino, M., Rapaccini, M., Saccani, N., Gaiardelli, P., Crespi, G., and Ruggeri, C. (2018). The role of digital technologies for the service transformation of industrial companies. Int. J. Prod. Res. 56 (6), 2116–2132. doi:10.1080/00207543.2017.1324224
Bai, Y., Song, S., Jiao, J., and Yang, R. (2019). The impacts of government R&D subsidies on green innovation: Evidence from Chinese energy-intensive firms. J. Clean. Prod. 233, 819–829. doi:10.1016/j.jclepro.2019.06.107
Ballestar, M. T., Díaz-Chao, Á., Sainz, J., and Torrent-Sellens, J. (2020). Knowledge, robots and productivity in SMEs: Explaining the second digital wave. J. Bus. Res. 108, 119–131. doi:10.1016/j.jbusres.2019.11.017
Beltran-Esteve, M., and Picazo-Tadeo, A. J. (2015). Assessing environmental performance trends in the transport industry: Eco-innovation or catching-up? Energy Econ. 51 (9), 570–580. doi:10.1016/j.eneco.2015.08.018
Bennett, D. S., Padgham, G. L., McCarty, C. S., and Carter, M. S. (2007). Teaching principles of economics: Internet vs. traditional classroom instruction. J. Econ. Econ. Educ. Res. 8 (1), 21.
Borowiecki, R., Borowiecki, B., Siuta-Tokarska, J., Suder, M., Thier, A., and Zmija, K. (2021). Developing digital economy and society in the light of the issue of digital convergence of the markets in the European Union countries. Energies 14 (9), 2717. doi:10.3390/en14092717
Cao, S., Nie, L., Sun, H., Sun, W., and Taghizadeh-Hesary, F. (2021). Digital finance, green technological innovation and energy-environmental performance: Evidence from China's regional economies. J. Clean. Prod. 327, 129458. doi:10.1016/j.jclepro.2021.129458
Carrion-Flores, C. E., Innes, R., and Sam, A. G. (2013). Do voluntary pollution reduction programs spur or deter environmental innovation? Evidence from 33/50. J. Environ. Econ. Manag. 66 (3), 444–459. doi:10.1016/j.jeem.2013.05.002
Cong, L. W., Xie, D., and Zhang, L. (2021). Knowledge accumulation, privacy, and growth in a data economy. Manag. Sci. 67 (10), 6480–6492. doi:10.1287/mnsc.2021.3986
Cooke, P. (2010). Regional innovation systems: Development opportunities from the ‘green turn. Technol. Analysis Strategic Manag. 22 (7), 831–844. doi:10.1080/09537325.2010.511156
Du, K., Cheng, Y., and Yao, X. (2021). Environmental regulation, green technology innovation, and industrial structure upgrading: The road to the green transformation of Chinese cities. Energy Econ. 98, 105247. doi:10.1016/j.eneco.2021.105247
Duraivelu, K. (2022). Digital transformation in manufacturing industry – a comprehensive insight. Mater. Today Proc. doi:10.1016/j.matpr.2022.07.409
Elhorst, J. P. (2014). Spatial econometrics: From cross-sectional data to spatial panels[M]. Berlin: Springer.
Fan, J., and Xiao, Z. (2021). Analysis of spatial correlation network of China’s green innovation. J. Clean. Prod. 299, 126815. doi:10.1016/j.jclepro.2021.126815
Fang, Z., Razzaq, A., Mohsin, M., and Irfan, M. (2022). Spatial spillovers and threshold effects of internet development and entrepreneurship on green innovation efficiency in China. Technol. Soc. 68, 101844. doi:10.1016/j.techsoc.2021.101844
Feng, S., Zhang, R., and Li, G. (2022). Environmental decentralization, digital finance and green technology innovation. Struct. Change Econ. Dyn. 61, 70–83. doi:10.1016/j.strueco.2022.02.008
Gelenbe, E., and Caseau, Y. (2015). The impact of information technology on energy consumption and carbon emissions. Ubiquity 2015, 1–15. doi:10.1145/2755977
Ghazy, N., Ghoneim, H., and Lang, G. (2022). Entrepreneurship, productivity and digitalization: Evidence from the EU. Technol. Soc. 70, 102052. doi:10.1016/j.techsoc.2022.102052
Gonzalez, J. L., and Jouanjean, M. A. (2017). Digital trade: Developing a framework for analysis[R]. OECD Trade Policy Pap. 5, 26. doi:10.1787/524c8c83-en
Gopalan, S., Reddy, K., and Sasidharan, S. (2022). Does digitalization spur global value chain participation? Firm-level evidence from emerging markets. Inf. Econ. Policy 59, 100972. doi:10.1016/j.infoecopol.2022.100972
Guan, H., and Guo, B. (2022). Digital economy and demand structure of skilled talents — analysis based on the perspective of vertical technological innovation. Telematics Inf. Rep. 7, 100010. doi:10.1016/j.teler.2022.100010
Horbach, J. (2007). Determinants of environmental innovation—new evidence from German panel data sources. Res. Policy 37 (1), 163–173. doi:10.1016/j.respol.2007.08.006
Hu, D., Jiao, J., Chen, C., Xiao, R., and Tang, Y. (2022b). Does global value chain embeddedness matter for the green innovation value chain? Front. Environ. Sci. doi:10.3389/fenvs.2022.779617
Hu, D., Jiao, J., Tang, Y., Han, X., and Sun, H. (2021a). The effect of global value chain position on green technology innovation efficiency: From the perspective of environmental regulation. Ecol. Indic. 121, 107195. doi:10.1016/j.ecolind.2020.107195
Hu, D., Jiao, J., Tang, Y., Xu, Y., and Zha, J. (2022c). How global value chain participation affects green technology innovation processes: A moderated mediation model. Technol. Soc. 68, 101916. doi:10.1016/j.techsoc.2022.101916
Hu, F., Qiu, L., Xi, X., Zhou, H., Hu, T., Su, N., et al. (2022a). Has COVID-19 changed China's digital trade? implications for health economics. Front. Public Health 10, 831549. doi:10.3389/FPUBH.2022.831549
Hu, F., Xi, X., and Zhang, Y. (2021b). Influencing mechanism of reverse knowledge spillover on investment enterprises’ technological progress: An empirical examination of Chinese firms. Technol. Forecast. Soc. Change 169, 120797. doi:10.1016/J.TECHFORE.2021.120797
Hummels, D., Ishii, J., and Yi, K. (2001). The nature and growth of vertical specialization in world trade. J. Int. Econ. 54 (1), 75–96. doi:10.1016/S0022-1996(00)00093-3
Humphrey, J., and Schmitz, H. (2002). How does insertion in global value chains affect upgrading in industrial clusters? Reg. Stud. 36 (9), 1017–1027. doi:10.1080/0034340022000022198
Ivarsson, I., and Alvstam, C. G. (2010). Supplier upgrading in the home-furnishing value chain: An empirical study of ikea’s sourcing in China and south east asia. World Dev. 38 (11), 1575–1587. doi:10.1016/j.worlddev.2010.04.007
Jin, Z., Duan, H., Wang, J., Yang, M., Guo, Y., and Cui, X. (2022). Heterogeneous impacts of GVCs participation on CO2 intensity: Evidence from developed and developing countries/regions. Adv. Clim. Change Res. 13 (2), 187–195. doi:10.1016/j.accre.2022.01.002
Johnstone, N., Managi, S., Rodriguez, M. C., Hascic, I., Fujii, H., and Souchier, M. (2017). Environmental policy design, innovation and efficiency gains in electricity generation. Energy Econ. 63 (3), 106–115. doi:10.1016/j.eneco.2017.01.014)
Kılıçaslan, Y., Aytun, U., and Meçik, O. (2021). Global value chain integration and productivity: The case of Turkish manufacturing firms. Middle East Dev. J. 13 (1), 150–171. doi:10.1080/17938120.2021.1898189
Koopman, R., Powers, W., Wang, Z., and Wei, S. (2010). Give credit where credit is due: Tracing value added in global production chains. NBER Work. Pap. doi:10.3386/w16426
Lanz, R., Lundquist, K., and Mansio, G. (2018). E-Commerce and developing country-SME participation in global value chains. WTO Staff Work. Pap. doi:10.30875/ec5f0f21-en
Li, J., and Du, Y. (2021). Spatial effect of environmental regulation on green innovation efficiency: Evidence from prefectural-level cities in China. J. Clean. Prod. 286, 125032. doi:10.1016/j.jclepro.2020.125032
Lin, B., and Ma, R. (2022). How does digital finance influence green technology innovation in China? Evidence from the financing constraints perspective. J. Environ. Manag. 320, 115833. doi:10.1016/j.jenvman.2022.115833
Liu, Y., Shao, X., Tang, M., and Lan, H. (2021). Spatio-temporal evolution of green innovation network and its multidimensional proximity analysis: Empirical evidence from China. J. Clean. Prod. 283, 124649. doi:10.1016/j.jclepro.2020.124649
Los, B., and Verspagen, B. (2000). R&D spillovers and productivity: Evidence from U.S. Manufacturing microdata. Empir. Econ. 25 (3), 127–148. doi:10.1007/s001810050007
Luo, Y., Salman, M., and Lu, Z. (2021). Heterogeneous impacts of environmental regulations and foreign direct investment on green innovation across different regions in China. Sci. Total Environ. 759, 143744. doi:10.1016/j.scitotenv.2020.143744
Ma, D., and Zhu, Q. (2022). Innovation in emerging economies: Research on the digital economy driving high-quality green development. J. Bus. Res. 145, 801–813. doi:10.1016/j.jbusres.2022.03.041
Martin, N., and Rice, J. (2014). Rebalancing climate change debate and policy: An analysis of online discussions. Env. Pol. Gov. 24 (5), 338–350. doi:10.1002/eet.1654
Murthy, K. V., Kalsie, A., and Shankar, R. (2021). Digital economy in a global perspective: Is there a digital divide? Transnatl. Corp. Rev. 13 (1), 1–15. doi:10.1080/19186444.2020.1871257
Namazi, M., and Namazi, N. R. (2016). Conceptual analysis of moderator and mediator variables in business research. Procedia Econ. Finance 36, 540–554. doi:10.1016/S2212-5671(16)30064-8
Oliveira, L., Fleury, A., and Fleury, M. T. (2021). Digital power: Value chain upgrading in an age of digitization. Int. Bus. Rev. 30 (6), 101850. doi:10.1016/j.ibusrev.2021.101850
Pan, W., Xie, T., Wang, Z., and Ma, L. (2022). Digital economy: An innovation driver for total factor productivity. J. Bus. Res. 139, 303–311. doi:10.1016/j.jbusres.2021.09.061
Peng, W., Yin, Y., Kuang, C., Wen, Z., and Kuang, J. (2021). Spatial spillover effect of green innovation on economic development quality in China: Evidence from a panel data of 270 prefecture-level and above cities. Sustain. Cities Soc. 69, 102863. doi:10.1016/j.scs.2021.102863
Prud'homme, D. (2016). Utility model patent regime “strength” and technological development: Experiences of China and other east asian latecomers. China Econ. Rev. 42, 50–73. doi:10.1016/j.chieco.2016.11.007
Qian, Z., Zhao, Y., Shi, Q., Zheng, L., Wang, S., and Zhu, J. (2022). Global value chains participation and CO2 emissions in RCEP countries. J. Clean. Prod. 332, 130070. doi:10.1016/j.jclepro.2021.130070
Ren, S., Hao, Y., and Wu, H. (2022). Digitalization and environment governance: Does internet development reduce environmental pollution? J. Environ. Plan. Manag. 1, 30. doi:10.1080/09640568.2022.2033959
Ren, S., Hao, Y., Xu, L., Wu, H., and Ba, N. (2021). Digitalization and energy: How does internet development affect China's energy consumption? Energy Econ. 98, 105220. doi:10.1016/j.eneco.2021.105220
Rennings, K. (2000). Redefining innovation -- eco-innovation research and the contribution from ecological economics. Ecol. Econ. 32 (2), 319–332. doi:10.1016/S0921-8009(99)00112-3
Schiederig, T., Tietze, F., and Herstatt, C. (2012). Green innovation in technology and innovation management: An exploratory literature review. R&D Manage. 42 (2), 180–192. doi:10.1111/j.1467-9310.2011.00672.x
Shen, Z., Wang, S., Boussemart, J. P., and Hao, Y. (2022). Digital transition and green growth in Chinese agriculture. Technol. Forecast. Soc. Change 181, 121742. doi:10.1016/j.techfore.2022.121742
Shi, Q., Shan, Y., Zhong, C., Cao, Y., and Xue, R. (2022). How would GVCs participation affect carbon intensity in the “Belt and road initiative” countries? Energy Econ. 111, 106075. doi:10.1016/j.eneco.2022.106075
Song, M., Wang, S., and Zhang, H. (2020). Could environmental regulation and R&D tax incentives affect green product innovation? J. Clean. Prod. 258, 120849. doi:10.1016/j.jclepro.2020.120849
Song, W., and Han, X. (2022). The bilateral effects of foreign direct investment on green innovation efficiency: Evidence from 30 Chinese provinces. Energy 261, 125332. doi:10.1016/j.energy.2022.125332
Spence, M. (2021). Government and economics in the digital economy. J. Gov. Econ. 3, 100020. doi:10.1016/j.jge.2021.100020
Stavytskyy, A., Kharlamova, G., and Stoica, E. A. (2019). The analysis of the digital economy and society index in the EU. TalTech J. Eur. Stud. 9 (3), 245–261. doi:10.1515/bjes-2019-0032
Su, D., and Shao, C. (2017). Global value chain, regional economic growth and spatial spillover. J. Int. Trade 11, 48–59. doi:10.13510/j.cnki.jit.2017.11.005
Szalavetz, A. (2022). The digitalisation of manufacturing and blurring industry boundaries. CIRP J. Manuf. Sci. Technol. 37, 332–343. doi:10.1016/j.cirpj.2022.02.015
Tang, M., Walsh, G., Lerner, D., Fitza, M. A., and Li, Q. (2018). Green innovation, managerial concern and firm performance: An empirical study. Bus. Strategy Environ. 27 (1), 39–51. doi:10.1002/bse.1981
Trivellas, P., Kakkos, N., Blanas, N., and Santouridis, I. (2015). The impact of career satisfaction on job performance in accounting firms—the mediating effect of general competencies. Procedia Econ. Finance 33, 468–476. doi:10.1016/S2212-5671(15)01730-X
Upward, R., Wang, Z., and Zheng, J. (2013). Weighing China’s export basket: The domestic content and technology intensity of Chinese exports. J. Comp. Econ. 41, 527–543. doi:10.1016/j.jce.2012.07.004
Wang, J., Luo, X., and Zhu, J. (2022d). Does the digital economy contribute to carbon emissions reduction? A city-level spatial analysis in China. Chin. J. Popul. Resour. Environ. 20 (2), 105–114. doi:10.1016/j.cjpre.2022.06.001
Wang, J., Rickman, D. S., and Yu, Y. (2022b). Dynamics between global value chain participation, CO2 emissions, and economic growth: Evidence from a panel vector autoregression model. Energy Econ. 109, 105965. doi:10.1016/j.eneco.2022.105965
Wang, S., and Chen, H. (2022). Could Chinese enterprises real benefit from embedding in global value chains? Environ. Dev. Sustain. doi:10.1007/s10668-022-02348-w
Wang, S., Chen, H., and Yin, K. (2021a). The employment effect of Chinese industrial enterprises embedded in environmental cost-adjusted global value chains. Environ. Sci. Pollut. Res. 29, 18160–18176. doi:10.1007/s11356-021-17118-w
Wang, S., Chen, M., and Song, M. (2018). Energy constraints, green technological progress and business profit ratios: Evidence from big data of Chinese enterprises. Int. J. Prod. Res. 56 (8), 2963–2974. doi:10.1080/00207543.2018.1454613
Wang, S., He, Y., and Song, M. (2021b). Global value chains, technological progress, and environmental pollution: Inequality towards developing countries. J. Environ. Manag. 277, 110999. doi:10.1016/j.jenvman.2020.110999
Wang, S., Li, Z., and Song, M. (2019). How embodied carbon in trade affects labor income in developing countries. Sci. Total Environ. 672, 71–80. doi:10.1016/j.scitotenv.2019.03.456
Wang, S., and Song, M. (2017). Influences of reverse outsourcing on green technological progress from the perspective of a global supply chain. Sci. Total Environ. 595, 201–208. doi:10.1016/j.scitotenv.2017.03.243
Wang, S., Tang, Y., Du, Z., and Song, M. (2020b). Export trade, embodied carbon emissions, and environmental pollution: An empirical analysis of China's high- and new-technology industries. J. Environ. Manag. 276, 111371. doi:10.1016/j.jenvman.2020.111371
Wang, S., Wang, X., and Chen, S. (2022e). Global value chains and carbon emission reduction in developing countries: Does industrial upgrading matter? Environ. Impact Assess. Rev. 97, 106895. doi:10.1016/j.eiar.2022.106895
Wang, S., Wang, X., and Lu, B. (2022a). Is resource abundance a curse for green economic growth? Evidence from developing countries. Resour. Policy 75, 102533. doi:10.1016/j.resourpol.2021.102533
Wang, S., Wang, X., and Tang, Y. (2020a). Drivers of carbon emission transfer in China—an analysis of international trade from 2004 to 2011. Sci. Total Environ. 709, 135924. doi:10.1016/j.scitotenv.2019.135924
Wang, Z., Wei, S., Yu, X., and Zhu, K. (2022c). Global value chains over business cycles. J. Int. Money Finance 126, 102643. doi:10.1016/j.jimonfin.2022.102643
Wu, B., and Yang, W. (2022). Empirical test of the impact of the digital economy on China's employment structure. Finance Res. Lett. 49, 103047. doi:10.1016/j.frl.2022.103047
Wu, H., Hao, Y., Ren, S., Yang, X., and Xie, G. (2021). Does internet development improve green total factor energy efficiency? Evidence from China. Energy Policy 153, 112247. doi:10.1016/j.enpol.2021.112247
Xu, Y., Li, X., Tao, C., and Zhou, X. (2022). Connected knowledge spillovers, technological cluster innovation and efficient industrial structure. J. Innovation Knowl. 7 (3), 100195. doi:10.1016/j.jik.2022.100195
Yang, B., Liu, B., Peng, J., and Liu, X. (2022). The impact of the embedded global value chain position on energy-biased technology progress: Evidence from China’s manufacturing. Technol. Soc. 71, 102065. doi:10.1016/j.techsoc.2022.102065
Yang, S., and Yi, Y. (2021). Effect of technological innovation inputs on global value chains status. J. Glob. Inf. Manag. 29 (5), 37–54. doi:10.4018/JGIM.20210901.oa3
Zeng, J., Ren, J., and Ning, Z. (2022). Mediating effect of corporate social responsibility-based entrepreneurship on environmental improvement: Evidence from 475 heavy-polluting enterprises. J. Bus. Res. 149, 506–517. doi:10.1016/j.jbusres.2022.05.014
Zeng, W., Li, L., and Huang, Y. (2021). Industrial collaborative agglomeration, marketization, and green innovation: Evidence from China’s provincial panel data. J. Clean. Prod. 279, 123598. doi:10.1016/j.jclepro.2020.123598
Zhang, J., Lyu, Y., Li, Y., and Geng, Y. (2022). Digital economy: An innovation driving factor for low-carbon development. Environ. Impact Assess. Rev. 96, 106821. doi:10.1016/j.eiar.2022.106821
Keywords: GVC embeddedness, digital economy, green innovation, mediating effect, spatial spillover effect
Citation: Pan H, Yang J, Zhou H, Zheng X and Hu F (2022) Global value chain embeddedness, digital economy and green innovation—Evidence from provincial-level regions in China. Front. Environ. Sci. 10:1027130. doi: 10.3389/fenvs.2022.1027130
Received: 24 August 2022; Accepted: 30 September 2022;
Published: 14 October 2022.
Edited by:
Shuhong Wang, Shandong University of Finance and Economics, ChinaReviewed by:
Yuanxiang Zhou, Anhui University of Finance and Economics, ChinaDianxi Hu, Anhui University of Finance and Economics, China
Siyu Ren, Nankai University, China
Copyright © 2022 Pan, Yang, Zhou, Zheng and Hu. This is an open-access article distributed under the terms of the Creative Commons Attribution License (CC BY). The use, distribution or reproduction in other forums is permitted, provided the original author(s) and the copyright owner(s) are credited and that the original publication in this journal is cited, in accordance with accepted academic practice. No use, distribution or reproduction is permitted which does not comply with these terms.
*Correspondence: Ji Yang, slancer@126.com
†These authors have contributed equally to this work and share first authorship.