Microbial Diversity of Reconstituted, Degraded, and Agricultural Soils Assessed by 16S rDNA Multi-Amplicon Sequencing
- 1Department of Agronomy, Food, Natural Resources, Animals and Environment (DAFNAE), University of Padova, Legnaro, Italy
- 2Crop Production and Biostimulation Laboratory (CPBL), Université Libre De Bruxelles, Bruxelles, Belgium
- 3MCM Ecosistemi S. r. l., Piacenza, Italy
The microbial diversity is, among soil key factors, responsible for soil fertility and nutrient biogeochemical cycles, and can be modified upon changes in main soil physicochemical properties and soil pollution. Over the years, many restoration techniques have been applied to restore degraded soils. However, the effect of these approaches on soil microbial diversity is less understood and thus requires more investigation. In this study, we analyzed the impact, on soil microbial diversity of a patented novel technology, used to restore degraded soils. Soil samples were collected from three nearby sites located in Borgotrebbia, Piacenza, Italy, and categorized as reconstituted, degraded, and agricultural soils. After total soil DNA extraction, 16S rDNA multi-amplicon sequencing was carried out using an Ion GeneStudio S5 System to compare soils’ bacterial community profiles. Sequenced reads were processed to assign taxonomy and then key microbial community differences were identified across the sampling sites. Species diversity featured significant abatement at all rank levels in the degraded soil when compared to the agricultural control. The 5 year restoration technique showed full recovery of this index at the genus level but not at the phylum level, displaying a rank-dependent gradient of restored richness. In parallel, the abundance of genes involved in the nitrogen (N) biogeochemical cycle was assessed using quantitative Real-Time PCR (qPCR). Total DNA content was significantly higher (p < 0.05) in degraded (μ = 12.69 ± 2.58 μg g−1) and reconstituted (μ = 11.73 ± 1.65 μg g−1) soil samples when compared to the agricultural soil samples (μ = 2.39 ± 0.50 μg g−1). The taxonomic diversity of each soil site was significantly different, with some instances unique of the agricultural soil even at the phylum level. The analysis of N functional genes showed that the relative abundance of bacterial amoA (p < 0.05) and nosZ (p < 0.01) genes were significantly lower in the agricultural than in the reconstituted and degraded soils. We concluded that the application of the soil reconstitution technique appears to enhance the active microbial community, with distinct diversity and functionality towards genes involved in N biogeochemical cycle, as compared to both the degraded and the agricultural soil.
Introduction
Soil originates from the weathering of parent materials under the combined action of climate, living organisms, and in function of the watershed relief and time (Jenny, 1946; Hartemink, 2016). During pedogenesis soils form complex assemblages of clay minerals (hydr-)oxides and organic matter, that result in their ultimate structure. Soil structure is responsible for soil’s physical and chemical functions in the environment such as water movement and retention, and mobility and bioavailability of nutrients and pollutants (Jastrow and Miller, 1991; Gregorich et al., 1997; Robinson et al., 2009; Bünemann et al., 2018). The biotic compartment of soil, composed of interrelated communities of fungi, bacteria, archaea, viruses, protists, and other microbial eukaryotes, is also defined as the soil microbiome (Jansson and Hofmockel, 2020).
It is estimated that soil microbiome controls up to 90% of soil processes, thus it plays a fundamental role in ecosystem functioning (Gregorich et al., 1997; Nannipieri et al., 2003; Young and Crawford, 2004). Moreover, the soil microbiome influences the biogeochemical cycles of nutrients, for example, acting as source or sink of gases, it contributes to nitrogen (N) and carbon (C) rates of fixation and oxidation, and it can degrade organic pollutants (Fierer, 2017). Therefore, though only a minor portion of the available soil space is colonized by the microbial communities (Young and Crawford, 2004), the stability and the resilience of the soil system are determined by the combination of soil physical structure, nutrient availability, microbial diversity and activity (Meuer et al., 2020). The soil microbiome is impacted by human activities like agriculture, soil sealing and industrial emissions that cause environmental pollution (Roose-Amsaleg et al., 2001; Maron et al., 2011) due to the changes that these activities induce in the soil structure. Since anthropogenic activities have decreased biodiversity in soils, the assessment of the soil microbiome can be a crucial indicator of soil quality (Lehmann et al., 2020; Vieira et al., 2022).
Soil is a non-renewable natural resource, and owing to the recent increased attention to its conservation, restoration of soil quality has become a key topic in science (Qilu et al., 2017; Yan et al., 2018; Xu et al., 2019). Several techniques have been used to form a porous structure in massive non-structured soils, and those based on the amendment with organic matter, revegetation or landfarming are among the most used (Sims and Sims, 2003). However, these techniques are primarily based on the mixing of soil with organic matter that improves their texture, mineralogy, pH value and cation exchange capacity, whereas the formation of a complex structure is slow and mainly due to the action of plant roots and soil microbes over relatively longer time periods. Techniques involving physical, chemical, and biological approaches have been also used to remove or transform harmful pollutants. Among these techniques, remediation using microbial consortia is well-established and widely used due to the lack of secondary pollution, potentially rapid degradation rates, and low cost (Agamuthu et al., 2013; Hesnawi and Mogdami, 2013). However, little is known about the effect of these restoration techniques on soil microbial diversity in degraded soils that have undergone microbial biomass loss. While microbial activity can be significantly increased by soil restoration, a steady increase of diversity of mirobial communities in restored soils is more difficult to achieve, thus such techniques require a deeper investigation.
The study of soil microbiome has been constrained for a long time because only a minority of microorganisms can be cultivated using standard techniques (Robe et al., 2003; Vester et al., 2015). Amann et al. (1995) observed that the culturability of bacteria from environmental samples ranged between 0.001 and 0.3% depending on the characteristics of the matrix. High-throughput culture-unrelated techniques, like Next Generation Sequencing (NGS), have been established over time to bypass the underestimation of soil microbial diversity problem (Chiodi et al., 2020). 16S rDNA multi-amplicon metabarcoding, sequencing at the same time several hypervariable regions, can generate a substantial amount of sequences, providing crucial information for a deep characterization of the microbiome even of extremely complex natural matrices such as soils (Young et al., 2017).
In this study, combining 16S rDNA metabarcoding and qPCR analyses, we investigated soil microbiome and individual genes coding for the enzymes involved in the N biogeochemical cycle, on soils treated with a novel technology, termed soil reconstruction and patented by MCM Ecosistemi S. r. l, which aims at creating a de novo soil structure from the original soil solid phase.
Such in a way we aimed at filling what we perceived as a gap of knowledge. The degree of novelty of the present report being the combination of the patented novel technique and the multi-amplicon sequencing assessment of its effects on soil microbial communities.
Materials and Methods
Site Location and Soil Sampling
Soil samples were collected from three sites located in Borgotrebbia, Piacenza, Italy (4503′58″ N 0939′06″ E, Figure 1). Vegetation types were mainly annual terophytes, dominated by Scotch thistle (Onopordum acanthium L.) (Giupponi et al., 2013, Giupponi et al., 2015).
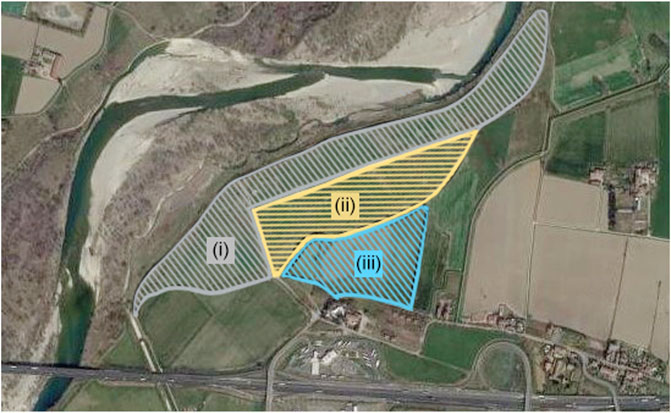
FIGURE 1. Aerial photograph of the studied area located along the hydrographic right bank of the Trebbia river. Geographical coordinates: 45°03′58″ N 09°39′06″ E. (i) Degraded sampling site, (ii) Reconstituted sampling site, (iii) Agricultural sampling site.
The degraded sampling site was a closed landfill made of municipal solid wastes. The landfill, which was active from 1972 to 1985 and that was covered with a 50 cm thick layer of backfill soil, covers a 20 ha area. The reconstituted sampling site corresponded to half of the landfill that underwent a reconstruction process, becoming a technosol, operated by MCM Ecosistemi S. r. l. with a patented novel technology (Manfredi et al., 2019). The agricultural sampling site was an adjacent agricultural field under conventional maize cultivation. Sampling was carried out with the linear transect technique (Brown, 1993). From each sampling site, 12 sub-samples were collected at a 20 cm depth using a manual auger. Sub-samples triplets were mixed to obtain four main samples for each site, referred to as: Reconstituted Soil (RS) (RS1, RS2, RS3, RS4), Degraded Soil (DS) (DS1, DS2, DS3, DS4), Agricultural Soil (AS) (AS1, AS2, AS3, AS4). Composite soil samples were air-dried at room temperature for 48 h, crushed, and sieved (Ø 0.5 mm) before the analysis.
Soil Chemical Analyses
Soil pH was measured potentiometrically in ultra-pure water (ratio soil/water 1:2.5 w/v) for each of the analyzed samples. Total carbon (C) and total nitrogen (N) content was determined by dry combustion using a CNS Vario Macro elemental analyzer (Elementar, Hanau, Germany), based on the Dumas combustion method (Dumas, 1831). The calibration curve was created using a certified sulphanilamide standard. The organic carbon content of each sample was tested using the Walkley-Black method (Walkley and Black 1934), while the extractable phosphorus (P) was evaluated using the Olsen method (Olsen et al., 1954).
Total Soil DNA Extraction, Multi-Amplicon 16S rDNA Sequencing, Analysis of Functional Genes of the N Biogeochemical Cycle
Total soil DNA was extracted from 250 mg of air-dried soil using the DNeasy PowerSoil Pro Kit (Qiagen, Germany), according to the manufacturer’s instructions. Nucleic acid quantification was performed using the Qubit 3.0 fluorometer (Thermo Fisher Scientific, Carlsbad, CA) with Qubit DNA High Sensitivity Assay Kit (Thermo Fisher Scientific).
Library preparation was carried out using the 16S Ion Metagenomics Kit (Thermo Fisher Scientific) that contains two pools of primers targeting seven different hypervariable regions (V2-V4-V8 primer pool and V3-V6-V7-V9 primer pool). 16S rRNA multi-amplicon sequencing was performed using an Ion GeneStudio S5 System (Thermo Fisher Scientific).
Raw reads were trimmed for 20 nucleotides on both ends to remove primers using the cutadapt utility and analyzed using Quantitative Insights Into Microbial Ecology 2 (QIIME2) v2020.08 (Bolyen et al., 2019) microbiome pipeline. Imported reads were first denoised and dereplicated using the “qiime dada2” plugin followed by taxonomic classification of Amplicon Sequence Variants (ASVs) by a “classify-consensus-blast” plugin using SILVA SSU v138.1 (Quast et al., 2012) as reference database. To check the quality of the achieved sequencing depth, alpha diversity rarefaction analysis was done using the “qiime alpha-diversity” plugin. The taxonomy abundance table at different taxonomic levels was further processed using the Calypso online suite (Zakrzewski et al., 2016) to Total Sum Scaling (TSS) normalized for library size differences. The resultant normalized table was filtered out by omitting taxa with less than the average of 10 reads across samples, and used for further diversity analysis and group comparison at different taxonomic levels. Principal component analysis was performed in Calypso using Bray-Curtis distances and the Shannon diversity index and Taxonomic Richness and community evenness were used for diversity comparisons.
The abundance of amoA (eubacterial, AOB), nifH, nirK, and nosZ bacterial genes was analyzed by quantitative Real-Time PCR (qPCR) using a QuantStudio 12K-Flex apparatus (Thermo Fisher Scientific). The 5 μL reaction mix was composed of 2.5 µL PowerUp SYBR Green Master Mix (Thermo Fisher Scientific), 0.15 µL each of forward and reverse primer (Table 1), 1.2 µL PCR-grade water, and 1 µL template DNA. A standard curve using known amounts of the target genes cloned in plasmids of known length (Chiodi et al., 2020) was obtained and data were used to calculate the copy number of the gene targets based on the Ct value.
Data analysis of gene abundance was performed using SPSS Statistics v28.0.0.0 (190) (IBM, Armonk, NY). Significant differences among the mean values were evaluated with a one-way ANOVA followed by Duncan’s post hoc test. Data were expressed as mean ± standard error of the mean. A Principal Component Analysis (PCA) based on Bray-Curtis distances was performed to display the core microbiome of the three soils.
Overall data analysis, including soil chemistry, was performed using SPSS Statistics v28.0.0.0 (190) (IBM, Armonk, NY). Significant differences among the mean values were evaluated with a one-way analysis of variance (one-way ANOVA) followed by post hoc analysis (S-N-K test). Data are expressed as mean ± standard error of the mean.
Results
Soil chemical analyses results are summarized in Table 2.

TABLE 2. Results of the chemical analyses on the soil samples at the beginning of the experiment. Means with the same letter in the vertical comparison among the sampling sites are not significantly different at S-N-K test. *Significance level p < 0.05.
The pH value of the RS was significantly lower (p < 0.05) than that of the DS and AS. The total C content of the AS was significantly lower (p < 0.05) than those of the RS and DS, whereas the organic C content was significantly higher (p < 0.05) in the RS when compared to DS and AS. Total N content was significantly higher (p < 0.05) in the following ranking order: RS > DS > AS. Extractable Olsen P was significantly lower (p < 0.05) in AS but no differences between DS and RS were observed.
Quantification of the total soil DNA showed a significantly (p < 0.05) higher amount of DNA in DS (μ = 12.69 ± 2.58 μg g−1) and RS (μ = 11.73 ± 1.65 μg g−1) soils compared to the AS soil (μ = 2.39 ± 0.50 μg g−1).
Bacterial 16S rDNA metabarcoding on the 12 soil samples provided a total number of 6,926,539 single-end reads, with an average length of 234 nucleotides. A total amount of 9,348 ASVs were identified and finally classified into 717 taxa. The alpha diversity rarefaction plot, corresponding to the number of observed features within samples, showed the highest number of detected sequences in AS samples compared to DS and RS samples (Figure 2).
As regards the taxonomy depth achieved, 85.5% of the annotated sequences were classified at genus rank level, 92.7% at family level, 94.3% at order level, 95.9% at class level and 96.5% at phylum level.
Upon splitting the output of the amplified sequence variants taxonomy table in subsets relative to the five different ranks of phylum, class, order, family, genus, and summing up the numbers of each in pivot tables, the consequent diversity within each level could be examined by calculating three ecological indexes assessing community richness, diversity and evenness and the results are shown in Figure 3.
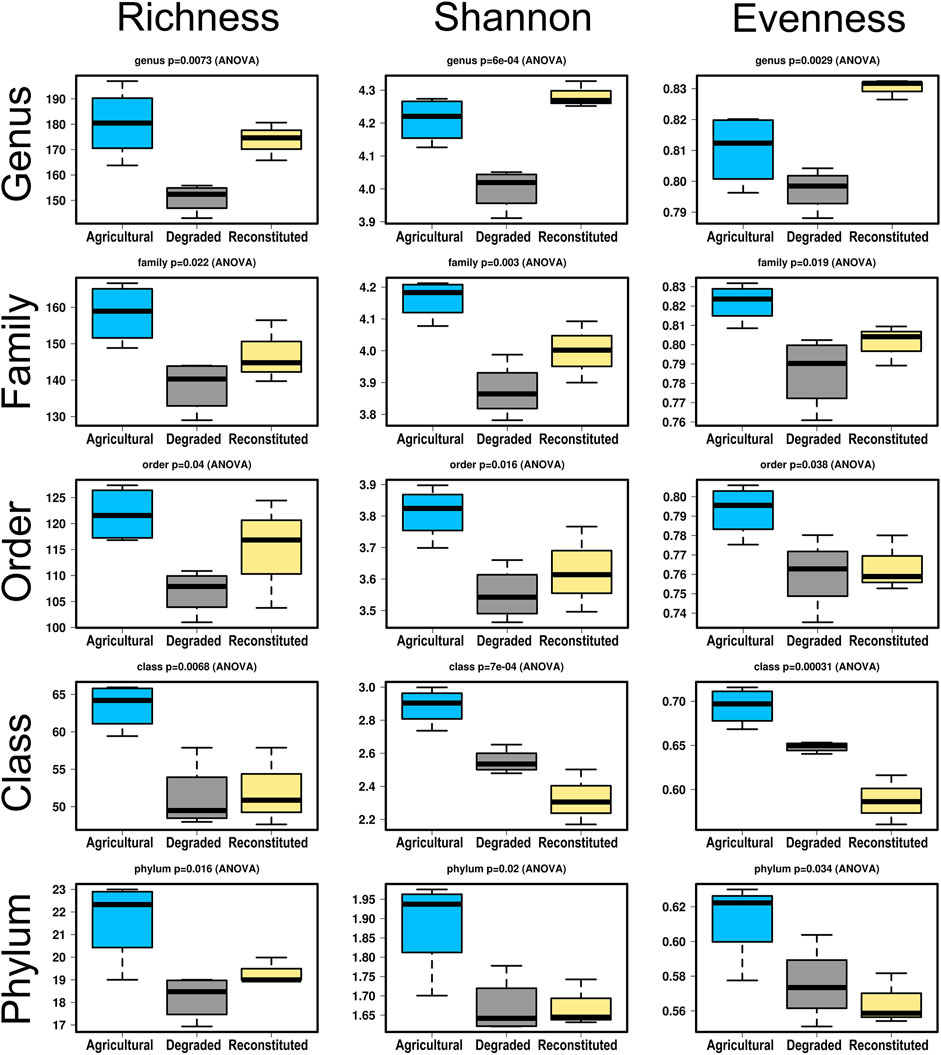
FIGURE 3. Boxplot comparisons of three ecological parameters (Taxa Richness, Shannon Index and Community Evenness) across five level of taxonomical ranks, for the bacterial communities resulting from the 16rDNA sequencing. Significance levels (ANOVA) are reported above each graph.
It can be seen that for all the three parameters, and in particular for those of diversity and richness, the agricultural control sampling site presents in most cases significantly higher values than its compared degraded and reconstituted sampling sites as far as the broader systematics divisions are concerned. However, moving up to finer clades, starting from the order, and culminating in the most distinct level (genus), the rise of the values for the reconstituted sampling site is very evident and eventually yields means that become also higher than those of the agricultural sampling site. On the contrary, the values of the degraded sampling site tend to stay inferior to both other soils in almost all cases, with exceptions mainly at class level for the Shannon index and evenness values.
The relative difference of each community was further analyzed by cluster analysis and the results are shown in Figure 4.
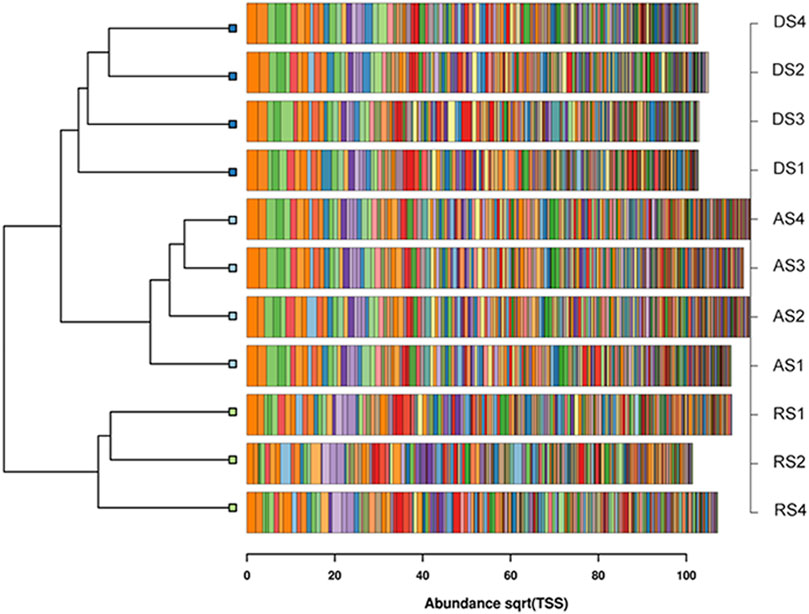
FIGURE 4. Clustered barchart dendrogram based on the Bray-Curtis distances of the first most abundant 250 taxa for each community.
The communities coming from the three soil management types are indeed partitioned accordingly in three clustered groups. The distance between the group of the agricultural soil and that of the degraded soil is shorter than the one that separates both of them from the reconstituted soil. Consistently with its nature of a reconstituted soil, the hosted bacterial communities appear thereby more distinct from those of the other origin.
Multivariate analyses were performed to further inspect the relative ordination of each of the communities and the consistency of the replicates within each group. Principal Coordinates Analysis, Principal Component Analysis and PERMDISP2 were computed and the results are shown in Figure 5.
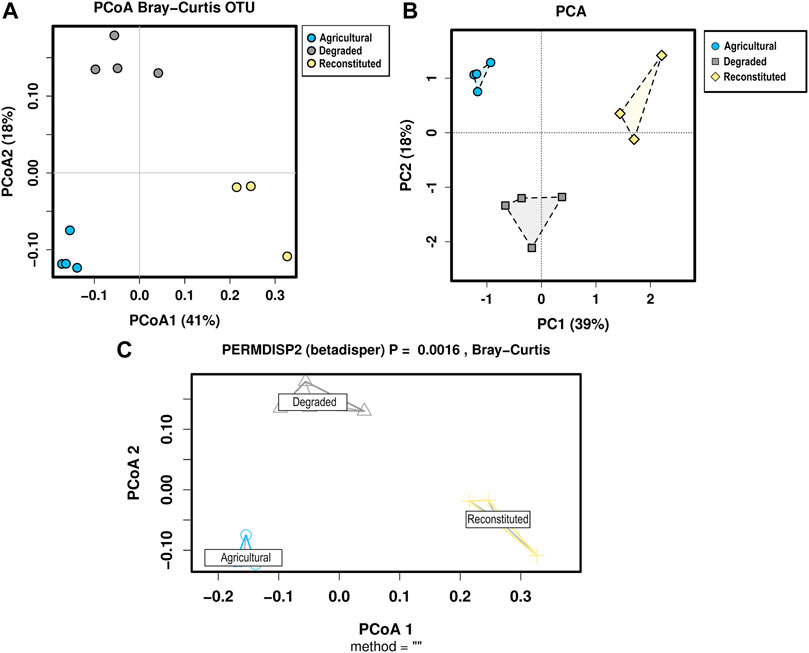
FIGURE 5. Multivariate analyses for the bacterial communities sequencing data. (A) Principal Coordinate Analysis based on the Bray-Curtis distances, (B) Principal Component Analysis, (C) PERMDISP2, which visualizes the distances of each sample to the group centroid in a Principal Coordinate Analysis (PCoA) and provides a p-value for the significance of the treatments.
All approaches coherently separated each community on the basis of the soil management variable. The PCA showed that bacterial taxa of the three soils clustered separately with polygon’s centroids significantly different (PERMANOVA p < 0.05).
Subsequently, on the five different rank-level data subsets, an analysis of the conserved core of shared taxa and of the unique ones was carried out, yielding the results shown in Figure 6.
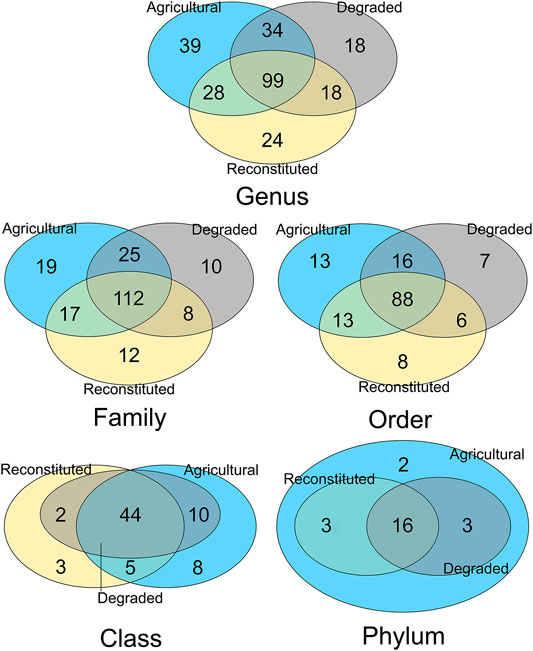
FIGURE 6. Venn diagrams showing the number of shared taxa (overlapping core and partials) and unique ones for each of the three types of soils, computed for each of the five taxonomy resolution layers. The stringency for units individuation abundance cutoff was set with the Relation-in-groups parameter = 0.40.
This analysis of the core versus specific sets of the microbiomes (Shade and Handelsman, 2012) showed the extent of uniqueness of taxa occurring at different ranks in each of the three management types, with the agricultural sampling site retaining the highest degrees of specificity, followed by the reconstituted sampling site and with the least number at all level the degraded sampling site. Additional information about top abundant unique taxa for each of the analysed soils, is reported in Supplementary Table S1 (Supplementary Material S1).
The qPCR analyses results of the nifH, the bacterial amoA, the nosZ, and the nirK genes are compared in Table 3. The RS samples yielded a higher content of the nifH gene copies when compared to the DS samples and to the AS samples. The bacterial amoA gene copies were significantly (p < 0.05) higher in the RS and in the DS samples that in AS samples.

TABLE 3. Gene copy numbers resulting from the qPCR analysis conducted targeting bacterial genes involved in the nitrogen biogeochemical cycle. Means with the same letter in the vertical comparison among the sampling sites are not significantly different at Duncan’s test. *Significance level p < 0.05. **Significance level p < 0.01.
RS samples showed the highest content of nosZ gene copies while DS samples showed a lower abundance and AS samples showed the lowest abundance.
The nirK gene copies showed a significantly lower abundance (p < 0.01) in AS samples than DS and RS samples.
Discussion
Soils comprehend a wide range of variable conditions, including abiotic conditions, for instance, nitrogen availability and circulation, and biotic conditions that can affect the structure and the abundance of microbial communities (Islam et al., 2020). In addition, soil microbial communities are affected by anthropogenic activities like agriculture practices and environmental pollution (Fierer, 2017; Teng and Chen, 2019).
In this study we observed that, despite the lowest quantity of total soil DNA, the AS samples, under conventional management, showed higher α-diversity of the bacterial community when compared to the DS samples and to the RS samples. A lack of correlation between DNA yield and bacterial diversity was previously reported by Sagova-Mareckova et al., 2008. Total soil DNA is more related to soil microbial biomass, and it is generally related to the soil pH value, the clay and organic matter content, and the vegetation cover (Burgmann et al., 2001). Thus, more microbial diversity seems to be related to long term soil activity and it is not easily reproducible with human interventions (Strickland et al., 2009). Abiotic stresses, like the discontinuous availability of nutrients and oxygen, and biotic stresses, like the presence and the abundance of predators, exert evolutionary pressure on soil microbial communities and help to select differences among the species without affecting soil functions (Hovatter et al., 2011; Jackson and Fahrig, 2014). The increase of microbial diversity at deeper taxonomic levels in RS samples when compared to DS samples might depend on the patented reconstruction technique that consists of a chemo-mechanical process. This reconstruction technique seems to implement particle aggregation and soil porosity enhancing the exchange of gasses and liquids (Manfredi et al., 2019), leading to a more suitable environment for soil microorganisms’ proliferation. The PCA plot’s underlying value also confirms that the taxonomic features of each sampling site were significantly different (p < 0.05) to cluster the analyzed soil samples. The same was confirmed by the PCoA and by the Permdisp2 analyses.
As regards details from the core vs. specific/unique microbiome analysis, at phylum level two bacterial phyla, Latescibacterota and NB1-j, were uniquely present in AS samples, although at low frequencies (both <1%). Those phyla are reported to be present in several environments although their function is still unknown (Jimenez et al., 2020; Dries et al., 2021; Hamdan et al., 2008; Coelho et al., 2016). At order level Chtonomonadales, a bacterial order capable to utilize different carbohydrate substrates as carbon and energy sources (Wang et al., 2019), were almost unique in AS samples. Lactobacillales, lactic acid bacteria (Baureder and Hederstedt, 2013), and Bacteroidales, a bacterial order present in human and animal faeces (Levantesi et al., 2012), were encountered almost only in DS samples, although not in a dominant fashion. RS samples, instead featured Chlamydiales, a typical soil order reported being found in agricultural soils (Schmalenberger and Tebbe, 2002), but again not as prevailing members. In general however, it can be commented that, at each taxonomy level examined, the truly dominant members across all replicates of the three types of soils were the same, with the Proteobacteria (Gamma- and Alpha-) and Actinobacteria leading at Phylum/Class levels, the Rhizobiales and Burkholderiales at order level, the nitrifying Nitrosomonadaceae at family level. More peculiarities instead emerged at Family and even more at Genus levels. These phenomena, besides the Venn diagram comparisons shown in Figure 6, are also entailed in the rank-related shifts shown for the three ecological parameters in Figure 3. Interestingly, the superior biodiversity values displayed by the AS samples are mostly maintained at high ranks as phylum, order, class etc., but are progressively overcome by the reconstituted restored soil, that appears to “catch up” when examined levels are unclustered in deeper and deeper ranks, culminating at genus level. In the comparison between the degraded and reconstituted soils, it is worth noticing that, while at high ranks, and particularly at class level, the Shannon index and evenness means of the reconstituted soil were lower than those of the degraded one, such is not the case for richness, which is the only index of the three, whose formula is not linked to the number of individuals found. This suggests that in both these soils numbers of individuals have an impacting effect on the ecological outcome, irrespective of the number of taxa, as long as broad categories are considered. On the contrary, when finer taxonomy resolution is the metrics (genus), the reconstituted sampling site prevails and equates the agricultural sampling site. Such sampling site can be considered to be also under a disturbance (being cropped), but with a very long history of adaptation to that predictable and recurring type of perturbation. In practice, Figure 3 shows that the agricultural soil is both phylum-rich and genus-rich, the degraded soil is phylum-poor and genus-poor, and the reconstituted soil is phylum-poor but genus-rich. This trend is consistent with data on really undisturbed controls as climaxing forest soils (Roesch et al., 2007), in those, phylum richness resulted even higher than that of cropped soils, but their established communities had relatively less genera and species, leading to what would be the fourth of these combinations (phylum-rich and genus-poor). In practice, the reconstruction of the degraded soils shows that in a few years, such degraded soil, which is the origin of the restored one, could be rescued up to a level of microbiodiversity that compares with that of the nearest agricultural soil control. Thus, the environmental carrying capacity K for possibly hosted species (Odum, 1953) and its imposed diversity ceiling appear to have been achieved by the soil reconstruction procedure, which can be seen as a-rather relevant ecological goal.
The interpretation of these trends suggests an intriguing picture. The short/unpredictable/non-cyclic type of human perturbation that led to the landfill conversion (the degraded soil DS) abated community structure and led to the loss of high-ranked taxonomical divisions (phyla), whose establishment involves time. The same soil, after 5 years of restoration (the RS soil) shows that, although this is too short a time to allow the return of lost phyla, it is nevertheless sufficient to drive a low-ranked diversification, leading to the recovery of diversity when measured by the genus metric. It can be also hypothesized that in fact the sudden absence of some previously present phyla, could even have left the available niches that would be then filled by the multiplying variants stemming from the remaining phyla. A scenario that, upon scaling-up of larger size and generation time, would comply to the “dinosaur-extinction/mammals radiation” model. The covariation of metabolic rate and body mass is in this sense well-demonstrated by Kleiber’s law (Kleiber, 1947). However, viewing the phenomena observed here as truly micro-evolutionary, would conflict with the notion of the 16S sequence being a molecular clock whose changes should require far longer timescales (Clark et al., 1999). Therefore, the rise of genus-level diversity in the reconstituted sampling site could be interpreted possibly as partly due to a physical recruitment (airborne immigration from other sites) and partly to a ‘technical’ recruitment, consisting in an increased detectability of reads in sequencing libraries as a consequence of the loss of other taxa that were otherwise quenching the counts of rare ones. The former mechanism (immigration) would not be sufficient by itself to explain why cells representative of missing genera should immigrate more easily than those of missing phyla and classes. But, since some phyla can encompass an extremely high diversity, while some other could be represented by even a single known species, the difference can be accounted for.
As regards the qPCR analyses of the N cycle genes, assessing the abundance of bacterial functional genes involved in nitrogen circulation is a useful tool to evaluate soil health and quality. The key steps of the nitrogen biogeochemical cycle are nitrification, the process that converts ammonium firstly in nitrite and secondly in nitrate, and denitrification, the process that reduces nitrate to molecular nitrogen (Tang et al., 2019). qPCR validated the abundance of selected microbial targets by evaluating nifH, bacterial amoA, nosZ, and nirK genes involved in the nitrogen biogeochemical cycle within the sampling sites. The degraded and the reconstituted soil samples showed a higher abundance of all the nitrogen-circulating tested genes compared to the agricultural soil samples. These disparities in gene copy numbers might be related to soils nitrogen content. Anikwe and Nwobodo (2002) reported that nitrogen content in the superficial horizon was 646–750% higher in long-term municipal waste landfill sites compared to agricultural sites. In addition, our chemical analyses results, that are in accordance with previously published results by Manfredi et al., 2019, highlighted that the reconstruction patented technique increases soils’ nitrogen content. Thus, the increased nitrogen inputs could have led to a higher nitrification and denitrification potential of degraded and restored soils. The fact that all these functional genes were found quantitatively in higher copies in the degraded and restored soil can be interpreted also in light of the above discussed result of the averagely six-fold higher content of total extractable soil DNA in both of them when compared to the agricultural cropped AS control soil. In interpreting both that difference and the ones resulting in these N-linked functional genes, it can be commented that the degraded and restored soils, being examples of recent and non-cyclic perturbations, turning over their previous nature, can be envisaged also as the equivalent of active construction worksites, in which the microbial populations would be engaged in multiplication, new nutrient flow interception, and a number of reorganizational responses in the shifted communities, that would explain the observed higher DNA values. Nevertheless, soil total DNA could be also contributed by fungi, protists, and by the remnant material from plants and animal origin. Therefore, the active state of bacteria could not necessarily be involving all of them but more likely, some funcrional groups as the ones we tested by qPCR.
In conclusion, several ecological hints arose from this comparative study. It is not easy to assess whether the differences acquired by the restored soil arose by the new chances open by the perturbation as such, which modified the environmental conditions, or were more specifically due to the restoration technique itself that requires the application of non-sterile sludges coming from specific industrial processes. In addition it is still unclear if the enhanced microbial diversity in RS samples, when compared to that of the DS samples, would be temporary or permanent. It can be also underlined that, while the restoration allowed the recovery of the ecological indexes of diversity, however the resulting community profile moved even farther away from the one of the agricultural soil, as shown in the cluster dendrogram of Figure 4, in which the degraded and agricultural soil bacteria appear closer to each other. The effect was therefore that of a shift to a novel assemblage, whose equilibrium and fate would have to be assessed in time. Of equal importance would be to determine whether the increased gene copies of the nitrogen cycling could entail some novel environmental concerns. In highly fertilized soils, considering that, in those cases, soil microbial activity is not able to metabolize the entire amount of nitrogen (Zilio et al., 2020), leading to nitrogen leaching through the vertical profile that can potentially reach subsurface water bodies. Their N enrichment is in fact one of the main causes of eutrophication. Such environmental syndrome, consisting of nutrients enrichment of water, culminates in the large production of biomasses related to algae proliferation. The degradation of these, once their short life cycle turns them into necrotic masses, results in hypoxia or anoxia situations and, also, in toxic bacterial emissions of methane, carbon dioxide and hydrogen sulphide (Le Moal et al., 2019). On the other hand, however, three reassuring issues can be put forward against these concerns. The first is the fact that a higher content of soil DNA is also reported in literature as a positive proxy for soil equilibrium (Fusaro et al., 2018). The second is that, since the gene copies detected by qPCR increased in all targeted genes, the phenomenon could be framed within that of the overall increase of soil DNA. The third is that, among the four PCR-targeted genetic determinants, the one that increased the most, and that did so in a statistically significant manner also in the comparison between degraded and restored soil, was nifH, i.e. one of the structural subunits of the nitrogenase protein, to which biological nitrogen fixation from either free-living or symbiotic prokaryotes is ultimately due. Being such metabolism the main gateway for nitrogen entrance into terrestrial as well as aquatic food chains, the enhancement of its key enzyme can be described as a positive premise in the pursuit of an improved environmental sustainability.
Data Availability Statement
The dataset generated for this study can be found in the European Nucleotide Archive, ID PRJEB48383, https://www.ebi.ac.uk/ena/browser/view/PRJEB48383.
Author Contributions
The first two authors contributed equally to this work. LM, SD, and PS made the conception, design of the study. LM, SD, GC, GR, and PM carried out the sampling. LM, SD, and CC did the sequencing. LM, SD, and SR, performed analyses. LM, SD, and AS conducted statistical analyses. LM, SD, and PS wrote the paper. LM, SD, AS, GR, and PS contributed to critical writing and reviewing of the manuscript. All authors reviewed the manuscript and gave final approval for publication.
Funding
This project was funded by the Veneto Region in the framework of the PSR 2014–2020. Author SR was supported by Cariparo Foundation (Italy).
Conflict of Interest
PM is employed by MCM Ecosistemi S. r. l.
The remaining authors declare that the research was conducted in the absence of any commercial or financial relationships that could be construed as a potential conflict of interest.
Publisher’s Note
All claims expressed in this article are solely those of the authors and do not necessarily represent those of their affiliated organizations, or those of the publisher, the editors and the reviewers. Any product that may be evaluated in this article, or claim that may be made by its manufacturer, is not guaranteed or endorsed by the publisher.
Supplementary Material
The Supplementary Material for this article can be found online at: https://www.frontiersin.org/articles/10.3389/fenvs.2021.807889/full#supplementary-material
References
Agamuthu, P., Tan, Y. S., and Fauziah, S. H. (2013). Bioremediation of Hydrocarbon Contaminated Soil Using Selected Organic Wastes. Proced. Environ. Sci. 18, 694–702. doi:10.1016/j.proenv.2013.04.094
Amann, R. I., Ludwig, W., and Schleifer, K. H. (1995). Phylogenetic Identification and In Situ Detection of Individual Microbial Cells without Cultivation. Microbiol. Rev. 59, 143–169. doi:10.1128/mr.59.1.143-169.1995
Anikwe, M., and Nwobodo, K. C. A. (2002). Long Term Effect of Municipal Waste Disposal on Soil Properties and Productivity of Sites Used for Urban Agriculture in Abakaliki, Nigeria. Bioresour. Technol. 83, 241–250. doi:10.1016/S0960-8524(01)00154-7
Baureder, M., and Hederstedt, L. (2013). Heme Proteins in Lactic Acid Bacteria. Adv. Microb. Physiol. 62, 1–43. doi:10.1016/B978-0-12-410515-7.00001-9
Bolyen, E., Rideout, J. R., Dillon, M. R., Bokulich, N. A., Abnet, C. C., Al-Ghalith, G. A., et al. (2019). Reproducible, Interactive, Scalable and Extensible Microbiome Data Science Using QIIME 2. Nat. Biotechnol. 37 (8), 852–857. doi:10.1038/s41587-019-0209-9
Brown, A. (1993). A Review of Soil Sampling for Chemical Analysis. Aust. J. Exp. Agric. 33 (8), 983. doi:10.1071/EA9930983
Bünemann, E. K., Bongiorno, G., Bai, Z., Creamer, R. E., De Deyn, G., De Goede, R., et al. (2018). Soil Quality - A Critical Review. Soil Biol. Biochem. 120, 105–125. doi:10.1016/j.soilbio.2018.01.030
Bürgmann, H., Pesaro, M., Widmer, F., and Zeyer, J. (2001). A Strategy for Optimizing Quality and Quantity of DNA Extracted from Soil. J. Microbiol. Methods 45, 7–20. doi:10.1016/S0167-7012(01)00213-5
Chiodi, C., Moro, M., Squartini, A., Concheri, G., Occhi, F., Fornasier, F., et al. (2020). High-throughput Isolation of Nucleic Acids from Soil. Soil Syst. 4 (1), 3. doi:10.3390/soilsystems4010003
Clark, M. A., Moran, N. A., and Baumann, P. (1999). Sequence Evolution in Bacterial Endosymbionts Having Extreme Base Compositions. Mol. Biol. Evol. 16(11), 1586–1598. doi:10.1093/oxfordjournals.molbev.a026071
Coelho, F. J. R. C., Louvado, A., Domingues, P. M., Cleary, D. F. R., Ferreira, M., Almeida, A., et al. (2016). Integrated Analysis of Bacterial and Microeukaryotic Communities from Differentially Active Mud Volcanoes in the Gulf of Cadiz. Sci. Rep. 6, 35272. doi:10.1038/srep35272
Dries, L., Bussotti, S., Pozzi, C., Kunz, R., Schnell, S., Löhnertz, O., et al. (2021). Rootstocks Shape Their Microbiome-Bacterial Communities in the Rhizosphere of Different Grapevine Rootstocks. Microorganisms 9 (4), 822. doi:10.3390/microorganisms9040822
Fierer, N. (2017). Embracing the Unknown: Disentangling the Complexities of the Soil Microbiome. Nat. Rev. Microbiol. 15, 579–590. doi:10.1038/nrmicro.2017.87
Fusaro, S., Squartini, A., and Paoletti, M. G., (2018). Functional Biodiversity, Environmental Sustainability and Crop Nutritional Properties: A Case Study of Horticultural Crops in north-eastern Italy. Appl. Soil Ecol. 123, 699, 708. doi:10.1016/j.apsoil.2017.06.023
Giupponi, L., Corti, C., Manfredi, P., and Cassinari, C. (2013). Application of the Floristic-Vegetational Indexes System for the Evaluation of the Environmental Quality of a Semi-natural Area of the Po Valley (Piacenza, Italy). Plant Sociol. 50 (2), 47–56. doi:10.7338/pls2013502/03
Giupponi, L., Corti, C., and Manfredi, P. (2015). The Vegetation of the Borgotrebbia Landfill (Piacenza, Italy): Phytosociological and Ecological Characteristics. Plant Biosyst. - Int. J. Dealing all Aspects Plant Biol. 149, 865–874. doi:10.1080/11263504.2014.945507
Gregorich, E. G., Carter, M. R., Doran, J. W., Pankhurst, C. E., and Dwyer, L. M. (1997). “Chapter 4 Biological Attributes of Soil Quality,” in Developments in Soil Science. Editors E. G. Gregorich, and M. R. Carter (Amsterdam, Netherlands: Elsevier), 81–113. doi:10.1016/s0166-2481(97)80031-1
Hamdan, L. J., Gillevet, P. M., Sikaroodi, M., Pohlman, J. W., Plummer, R. E., and Coffin, R. B. (2008). Geomicrobial Characterization of Gas Hydrate-Bearing Sediments along the Mid-Chilean Margin. FEMS Microbiol. Ecol. 65, 15–30. doi:10.1111/j.1574-6941.2008.00507.x
Hartemink, A. E. (2016). The Definition of Soil since the Early 1800s. Adv. Agron., 137, 73–126. doi:10.1016/bs.agron.2015.12.001
Henry, S., Baudoin, E., López-Gutiérrez, J. C., Martin-Laurent, F., Brauman, A., and Philippot, L. (2004). Quantification of Denitrifying Bacteria in Soils by nirK Gene Targeted Real-Time PCR. J. Microbiol. Methods 59 (3), 327–335. doi:10.1016/j.mimet.2004.07.002
Hesnawi, R. M., and Mogadami, F. S. (2013). Bioremediation of Libyan Crude Oil-Contaminated Soil under Mesophilic and Thermophilic Conditions. APCBEE Proced. 5, 82–87. doi:10.1016/j.apcbee.2013.05.015
Hovatter, S. R., Dejelo, C., Case, A. L., and Blackwood, C. B. (2011). Metacommunity Organization of Soil Microorganisms Depends on Habitat Defined by Presence of Lobelia Siphilitica Plants. Ecology 92, 57–65. doi:10.1890/10-0332.1
Islam, W., Noman, A., Naveed, H., Huang, Z., and Chen, H. Y. H. (2020). Role of Environmental Factors in Shaping the Soil Microbiome. Environ. Sci. Pollut. Res. 27, 41225–41247. doi:10.1007/s11356-020-10471-2
Jackson, N. D., and Fahrig, L. (2014). Landscape Context Affects Genetic Diversity at a Much Larger Spatial Extent Than Population Abundance. Ecology 95, 871–881. doi:10.1890/13-0388.1
Jansson, J. K., and Hofmockel, K. S. (2020). Soil Microbiomes and Climate Change. Nat. Rev. Microbiol. 18, 35–46. doi:10.1038/s41579-019-0265-7
Jastrow, J. D., and Miller, R. M. (1991). Methods for Assessing the Effects of Biota on Soil Structure. Agric. Ecosyst. Environ. 34, 279–303. doi:10.1016/0167-8809(91)90115-E
Jenny, H. (1946). Arrangement of Soil Series and Types According to Functions of Soil-Forming Factors. Soil Sci. 61 (5), 375–392. doi:10.1097/00010694-194605000-00005
Jiménez, J. A., Novinscak, A., and Filion, M. (2020). Inoculation with the Plant-Growth-Promoting Rhizobacterium Pseudomonas Fluorescens LBUM677 Impacts the Rhizosphere Microbiome of Three Oilseed Crops. Front. Microbiol. 11, 569366. doi:10.3389/fmicb.2020.569366
Kleiber, M. (1947). Body Size and Metabolic Rate. Physiol. Rev. 27, 511–541. doi:10.1152/physrev.1947.27.4.511
Le Moal, M., Gascuel-Odoux, C., Ménesguen, A., Souchon, Y., Étrillard, C., Levain, A., et al. (2019). Eutrophication: A New Wine in an Old Bottle? Sci. Total Environ. 651 (1), 1–11. doi:10.1016/j.scitotenv.2018.09.139
Lehmann, J., Bossio, D. A., Kögel-Knabner, I., and Rillig, M. C. (2020). The Concept and Future Prospects of Soil Health. Nat. Rev. Earth Environ. 1, 544–553. doi:10.1038/s43017-020-0080-8
Levantesi, C., Bonadonna, L., Briancesco, R., Grohmann, E., Toze, S., and Tandoi, V. (2012). Salmonella in Surface and Drinking Water: Occurrence and Water-Mediated Transmission. Food Res. Int. 45 (2), 587–602. doi:10.1016/j.foodres.2011.06.037
Manfredi, P., Cassinari, C., Francaviglia, R., and Trevisan, M. (2019). A New Technology to Restore Soil Fertility: Reconstitution. Agrochimica LXIII (3). 247–260. doi:10.12871/00021857201933
Maron, P.-A., Mougel, C., and Ranjard, L. (2011). Soil Microbial Diversity: Methodological Strategy, Spatial Overview and Functional Interest. Comptes Rendus Biologies 334, 403–411. doi:10.1016/j.crvi.2010.12.003
Meuer, J., Koelbel, J., and Hoffmann, V. H. (2020). On the Nature of Corporate Sustainability. Organ. Environ. 33 (3), 319–341. doi:10.1177/1086026619850180
Nannipieri, P., Ascher, J., Ceccherini, M. T., Landi, L., Pietramellara, G., and Renella, G. (2003). Microbial Diversity and Soil Functions. Eur. J. Soil Sci. 54, 655–670. doi:10.1111/ejss.4_1239810.1046/j.1351-0754.2003.0556.x
Odum, Eugene. P. (1953). “Fundamentals of Ecology,” in The Future of Nature: Documents of Global Change. Editors Libby. Robin, Sverker. Sörlin, and Warde. Paul (New Haven: Yale University Press), 233–244. doi:10.12987/9780300188479-022
Olsen, S. R., Cole, C. V., Watanabe, F. S., and Dean, L. A. (1954). Estimation of Available Phosphorus in Soils by Extraction with Sodium Bicarbonate, 939. Washington D.C: USDA circularU.S. Gov. Print. Office.
Qilu, C., Xueling, W., ligen, X., Hui, L., Yuhua, Z., and Qifa, Z. (2017). High-quality, Ecologically Sound Remediation of Acidic Soil Using Bicarbonate-Rich Swine Wastewater. Sci. Rep. 7, 11911. doi:10.1038/s41598-017-12373-9
Quast, C., Pruesse, E., Yilmaz, P., Gerken, J., Schweer, T., Yarza, P., et al. (2012). The SILVA Ribosomal RNA Gene Database Project: Improved Data Processing and Web-Based Tools. Nucleic Acids Res. 41 (D1), D590–D596. doi:10.1093/nar/gks1219
Robe, P., Nalin, R., Capellano, C., Vogel, T. M., and Simonet, P. (2003). Extraction of DNA from Soil. Eur. J. Soil Biol. 39, 183–190. doi:10.1016/S1164-5563(03)00033-5
Robinson, D. A., Lebron, I., and Vereecken, H. (2009). On the Definition of the Natural Capital of Soils: A Framework for Description, Evaluation, and Monitoring. Soil Sci. Soc. Am. J. 73, 1904–1911. doi:10.2136/sssaj2008.0332
Roesch, L. F. W., Fulthorpe, R. R., Riva, A., Casella, G., Hadwin, A. K. M., Kent, A. D., et al. (2007). Pyrosequencing Enumerates and Contrasts Soil Microbial Diversity. ISME J. 1 (4), 283–290. doi:10.1038/ismej.2007.53
Roose-Amsaleg, C. L., Garnier-Sillam, E., and Harry, M. (2001). Extraction and Purification of Microbial DNA from Soil and Sediment Samples. Appl. Soil Ecol. 18, 47–60. doi:10.1016/S0929-1393(01)00149-4
Rotthauwe, J. H., Witzel, K. P., and Liesack, W. (1997). The Ammonia Monooxygenase Structural Gene amoA as a Functional Marker: Molecular fine-scale Analysis of Natural Ammonia-Oxidizing Populations. Appl. Environ. Microbiol. 63 (12), 4704–4712. doi:10.1128/aem.63.12.4704-4712.1997
Rösch, C., Mergel, A., and Bothe, H. (2002). Biodiversity of Denitrifying and Dinitrogen-Fixing Bacteria in an Acid Forest Soil. Appl. Environ. Microbiol. 68 (8), 3818–3829. doi:10.1128/AEM.68.8.3818-3829.2002
Sagova-Mareckova, M., Cermak, L., Novotna, J., Plhackova, K., Forstova, J., and Kopecky, J. (2008). Innovative Methods for Soil DNA Purification Tested in Soils with Widely Differing Characteristics. Appl. Environ. Microbiol. 74 (9), 2902–2907. doi:10.1128/AEM.02161-07
Schmalenberger, A., and Tebbe, C. C. (2002). Bacterial Community Composition in the Rhizosphere of a Transgenic, Herbicide-Resistant maize (Zea mays) and Comparison to its Non-transgenic Cultivar Bosphore. FEMS Microbiol. Ecol. 40 (1), 29–37. doi:10.1111/j.1574-6941.2002.tb00933.x
Shade, A., and Handelsman, J. (2012). Beyond the Venn Diagram: the hunt for a Core Microbiome. Environ. Microbiol. 14 (1), 4–12. doi:10.1111/j.1462-2920.2011.02585.x
Sims, R. C., and Sims, J. L. (2003). “Landfarming Framework for Sustainable Soil Bioremediation,” in Utilization Bioremediation Reduce Soil Contamination: Probl. Solutions. NATO Sci. Ser. IV Earth Environ. Sci. Editors V. Šašek, J. A. Glaser, and Ph. Baveye (Dordrecht, Netherlands: Springer), 19, 319–334. doi:10.1007/978-94-010-0131-1_27
Strickland, M. S., Osburn, E., Lauber, C., Fierer, N., and Bradford, M. A. (2009). Litter Quality Is in the Eye of the Beholder: Initial Decomposition Rates as a Function of Inoculum Characteristics. Funct. Ecol. 23, 627–636. doi:10.1111/j.1365-2435.2008.01515.x
Tang, J., Zhang, J., Ren, L., Zhou, Y., Gao, J., Luo, L., et al. (2019). Diagnosis of Soil Contamination Using Microbiological Indices: A Review on Heavy Metal Pollution. J. Environ. Manage. 242, 121–130. doi:10.1016/j.jenvman.2019.04.061
Teng, Y., and Chen, W. (2019). Soil Microbiomes-A Promising Strategy for Contaminated Soil Remediation: A Review. Pedosphere 29 (3), 283–297. doi:10.1016/S1002-0160(18)60061-X
Vester, J. K., Glaring, M. A., and Stougaard, P. (2015). Improved Cultivation and Metagenomics as New Tools for Bioprospecting in Cold Environments. Extremophiles 19, 17–29. doi:10.1007/s00792-014-0704-3
Vieira, C. K., dos Anjos Borges, L. G., Bortolini, J. G., Soares, C. R. F. S., Giongo, A., and Stürmer, S. L. (2022). Does a Decrease in Microbial Biomass Alter Mycorrhizal Attributes and Soil Quality Indicators in Coal Mining Areas under Revegetation Process? Sci. Total Environ. 802, 149843. doi:10.1016/j.scitotenv.2021.149843
Walkley, A., and Black, I. A. (1934). An Examination of the Degtjareff Method for Determining Soil Organic Matter, and a Proposed Modification of the Chromic Acid Titration Method. Soil Sci. 37 (1), 29–38. doi:10.1097/00010694-193401000-00003
Wang, B., Wang, Y., Cui, X., Zhang, Y., and Yu, Z. (2019). Bioconversion of Coal to Methane by Microbial Communities from Soil and from an Opencast Mine in the Xilingol Grassland of Northeast China. Biotechnol. Biofuels 12, 236. doi:10.1186/s13068-019-1572-y
Xu, J., Liu, C., Hsu, P.-C., Zhao, J., Wu, T., Tang, J., et al. (2019). Remediation of Heavy Metal Contaminated Soil by Asymmetrical Alternating Current Electrochemistry. Nat. Commun. 10, 2440. doi:10.1038/s41467-019-10472-x
Yan, Y., Xue, F., Muhammad, F., Yu, L., Xu, F., Jiao, B., et al. (2018). Application of Iron-Loaded Activated Carbon Electrodes for Electrokinetic Remediation of Chromium-Contaminated Soil in a Three-Dimensional Electrode System. Sci. Rep. 8, 5753. doi:10.1038/s41598-018-24138-z
Young, I. M., and Crawford, J. W. (2004). Interactions and Self-Organization in the Soil-Microbe Complex. Science 304, 1634–1637. doi:10.1126/science.1097394
Young, J. M., Austin, J. J., and Weyrich, L. S. (2017). Soil DNA Metabarcoding and High-Throughput Sequencing as a Forensic Tool: Considerations, Potential Limitations and Recommendations. FEMS Microbiol. Ecol. 93 (2), fiw207. doi:10.1093/femsec/fiw207,
Zakrzewski, M., Proietti, C., Ellis, J. J., Hasan, S., Brion, M.-J., Berger, B., et al. (2016). Calypso: A User-Friendly Web-Server for Mining and Visualizing Microbiome-Environment Interactions. Bioinformatics 33 (5), btw725–783. doi:10.1093/bioinformatics/btw725
Zilio, M., Motta, S., Tambone, F., Scaglia, B., Boccasile, G., Squartini, A., et al. (2020). The Distribution of Functional N-Cycle Related Genes and Ammonia and Nitrate Nitrogen in Soil Profiles Fertilized with mineral and Organic N Fertilizer. PLoS ONE 15 (6), e0228364. doi:10.1371/journal.pone.0228364
Keywords: microbial diversity, next-generation sequencing, soil microbial activity, soil remediation, qPCR, soil microbial profile, 16S rdna multi-amplicon
Citation: Maretto L, Deb S, Ravi S, Chiodi C, Manfredi P, Squartini A, Concheri G, Renella G and Stevanato P (2022) Microbial Diversity of Reconstituted, Degraded, and Agricultural Soils Assessed by 16S rDNA Multi-Amplicon Sequencing. Front. Environ. Sci. 9:807889. doi: 10.3389/fenvs.2021.807889
Received: 02 November 2021; Accepted: 13 December 2021;
Published: 07 January 2022.
Edited by:
Thomas Keller, Swedish University of Agricultural Sciences, SwedenReviewed by:
Anna Gałązka, Institute of Soil Science and Plant Cultivation, PolandMagdalena Frac, Institute of Agrophysics (PAN), Poland
Copyright © 2022 Maretto, Deb, Ravi, Chiodi, Manfredi, Squartini, Concheri, Renella and Stevanato. This is an open-access article distributed under the terms of the Creative Commons Attribution License (CC BY). The use, distribution or reproduction in other forums is permitted, provided the original author(s) and the copyright owner(s) are credited and that the original publication in this journal is cited, in accordance with accepted academic practice. No use, distribution or reproduction is permitted which does not comply with these terms.
*Correspondence: Piergiorgio Stevanato, stevanato@unipd.it
†These authors have contributed equally to this work and share first authorship