Load capability assessment and enhancement for transformers with integration of large-scale renewable energy: A brief review
- School of New Energy and Power Engineering, Lanzhou Jiaotong University, Lanzhou, China
With the rapid development of large-scale distributed renewable energy in China, the load factor of distribution transformer increases year by year, this directly become a new challenge to the load capacity of the transformer. Once subjected to this condition for a long time will have an impact on the hotspot temperature and consequently, the useful life of the transformer. In this regard, load capacity assessment of the distribution transformer becomes an essential work for unit upgrading or even replacement. This article systematically summarized the evaluation methods of transformer load capacity, as well as the load capacity prediction and dynamic capacity enhancement strategies of transformer. Discussions for the future work regarding this topic are also carried out.
1 Introduction
To fulfill the goal of carbon neutral, the Chinese power utilities are advancing the development of a new power system dominated by new energy, especially for large-scale integrated wind and solar power generations and the distributed renewable energy resources, as shown in Figure 1. This directly leads to the increase of the load factor of transformers year by year. On the distribution side, the power flow is no longer one direction, i.e., flowing from the distribution transformer to users, but becomes a bi-direction mode as distributed renewable energy resources integrated to the network (Feng et al., 2018; Feng et al., 2022; Jiang et al., 2022). Figure 1 shows a schematic diagram of a distribution network dominated by new energy generation, demonstrating the load changes from the traditional single distribution load curve to a composite load combining distribution load and new energy online load.
The introduction of large-scale distributed renewable energy sources poses a great threat to the safe operation of the power grid. If the load capacity assessment and dynamic capacity enhancement of the transformer can be realized, the load potential can be fully exploited and the threat posed to the grid by the composite load can be controlled. This enables real-time regulation of the transformer load, so intelligent transformers (Ma et al., 2015) with information sensing technology become a new trend. To improve the intelligence of transformer, the condition assessment model, overload model, insulation aging model, heat balance model, cooling model, etc. have been established to assess and predict the condition of the transformer. This greatly advances the condition-based maintenance of the transformer.
Therefore, the study of the load capacity of transformers is essentially a matter of ensuring that the actual maximum load that a transformer can withstand and the duration it can last without the hotspot temperature (HST) exceeding the limit (Tang et al., 2017). In the standards of IEC 60076-7, GB/T 1094.7 and IEEE Std C57.91-2011, the limits for overload operation of oil-immersed power transformers are specified. The HST of the transformer winding insulation system is the main determinant of the load capacity, and when the HST exceeds the limit, it will lead to insulation damage or loss of life (Zhong et al., 2021).
This paper briefly summarizes the current load capacity assessment methods and expounds the load prediction methods, as well as the necessity of dynamic capacity enhancement. The problems of current load capacity assessment methods are discussed and suggestions for the future study are proposed.
2 Influence factor of transformer load capacity
The load capacity of a transformer is determined by factors such as material selection and process control during its manufacturing phase, which reflects the inherent level of transformer load capacity. The load capacity of a transformer in operation is not only determined by its inherent level, but is also influenced by the operating factors such as the HST, ambient temperature, and cooling method. This section will detail such factors one by one.
2.1 Hot spot temperature
The core and winding of the transformer are the main source of the heat generation, where the temperature of the hottest spot in the winding is called the HST. Currently, there are three kinds of method to obtain the HST of transformers, including the direct method, the numerical analysis method, and the thermoelectric analogous model. The HST temperature limit for transformers is specified in GB/T 1094.7, which can be expressed as Eq. 1. The load condition of the transformer can have an effect on the HST.
where, θa is the ambient temperature, ∆θor is the standard oil temperature rise, ∆θhr is the oil temperature gradient, R is the loss ratio, K is the ratio of actual load to rated load, x is top oil index, H is HST coefficient, gr is the temperature difference between the average temperature of the winding and oil temperature measured by the test, y winding index, f1(t), f2(t) are in the exponential form, indicating the slow process of internal temperature rise or fall.
2.1.1 Direct detection method
The direct detection methods incorporate two categories: invasive measurements (Qian et al., 2003; Lobo Ribeiro et al., 2008; Liu et al., 2020) and non-invasive measurements (Ruan et al., 2020).
The invasive detection of winding HST is mainly realized by direct measurements. For example, the U. S. Luxtron Corporation applies a stable light body temperature sensor (Qian et al., 2003) to sense the HST of a transformer, and a multipoint fiber optic temperature sensor network system is integrated inside the transformer (Lobo Ribeiro et al., 2008). Some built-in distributed fibers combined with thermocouple are also introduced to for direct measurement (Liu et al., 2020).
In comparative, the non-invasive measurement of HST is mainly realized by infrared techniques with interpreting the streamline heat dissipation model of the internal heat source of the equipment (Ruan et al., 2020). The mapping relationship between the shell characteristic temperature measurement point and the internal HST of the transformer is established.
2.1.2 Numerical analysis method
The numerical analysis methods predict transformer temperature rise based on multi-physics field coupling calculations. The establishment of the solution model and the determination of the boundary conditions are complicated. Experts use the fluid-solid coupling method (Xie et al., 2016) to calculate the HST of the transformer, or use magnetic-thermal coupling (Liao et al., 2021) to solve the temperature distribution to improve the accuracy of the internal temperature calculation results. For the simulation model, the shortcoming lies that it is difficult to reflect the real oil flow and heat transfer inside the transformer and the final temperature rise distribution cannot reflect the temperature rise in the full domain. For load capacity analysis, the numerical analysis is often realized by Ansys Fluent and COMSOL, and the results are used as complementary to experimental testing verification to justify and support the overall analysis.
2.1.3 Thermoelectric analogy model
Considering the heat flow is similar to the current flow in circuit, the hot circuit model of transformer conduction is constructed by thermoelectric analogy (Swift et al., 2001). In load capacity assessment, researchers (Susa and Nordman, 2009) considered the change in oil viscosity and winding loss with temperature and established a simplified model of HST based on the top oil temperature. Such models greatly reduce the demand of input parameters. Some experts also use a thermoelectric analogous model to estimate the HST, thermal resistance and thermal capacity to improve the dynamic response of the model, and propose a load capacity assessment model in consequence (Li et al., 2018). Besides, the influence of HST on the load capacity of traction transformers is also studied and a collector heat path model is established to simplify the nonlinear thermal resistance to real-time thermal capacity evaluation (Zhou et al., 2021).
Since the results of transformer HST directly affect the accuracy of transformer load capacity, a large number of scholars have conducted research in HST calculation. At present, the HST is mainly obtained by direct measurements, analyzed and demonstrated by applying numerical analysis method, and estimated by the idea of thermoelectric analogy.
2.2 Dissipation mode
The dissipation theory of the transformer assumes part of the heat generated by the core and coil can be dissipated by transformer oil and dissipated by dissipaters. The process of heat dissipation is actually the process of reducing the internal temperature and increasing the load factor. It is suggested to introduce dissipation mode as a factor of load capability assessment to provide better guidance for transformer optimization (Li et al., 2018). If damage to the fan occurs when the transformer has to withstand an overload situation, it can lead to an impact on the overload capacity.
2.3 Ambient temperature
As one of the important parameters affecting the load capacity of transformer, ambient temperature plays a key role. In order to establish a more accurate load prediction model, the highest as well as the lowest temperature of the day needs to be taken into account, and the ambient temperature will be fuzzy discretized according to the standard GB/T 1094.7-2008. Moreover, under different initial loads, the change of allowable overload time has similar characteristics: it decreases rapidly with the increase of ambient temperature. Therefore, the accuracy of ambient temperature has a great influence on the calculation of transformer overload capacity.
3 Progress on load capacity assessment
With the integration of large-scale new energy, load capacity assessment becomes a critical task for the new energy power systems. In this regards, it is necessary to pay more attention to load capacity prediction as well as the dynamic capacity enhancement strategy of transformer.
3.1 Load capacity prediction
According to the prediction requirement in temporal dimension, load capacity prediction can be classified as ultra-short-term prediction (in hours and minutes), short-term prediction (in days), medium-term prediction (in months) and long-term prediction (in years). The accuracy of load capacity prediction requires both the reliability of data and the applicability of the prediction algorithm. Load capacity prediction mainly focuses on two types: one is prediction based on actual transformer measurement data, such as top oil temperature and HST, and the other is simulation results prediction based on computational model.
3.1.1 Measured data-based prediction
The top oil temperature prediction based on Kalman filtering combines current data with historical loads to predict the trend of transformer load capacity (Zhou et al., 2020). For such method, an evaluation model would be constructed with multiple data inputs, as shown in Figure 2A, but it has not been applied in practices.
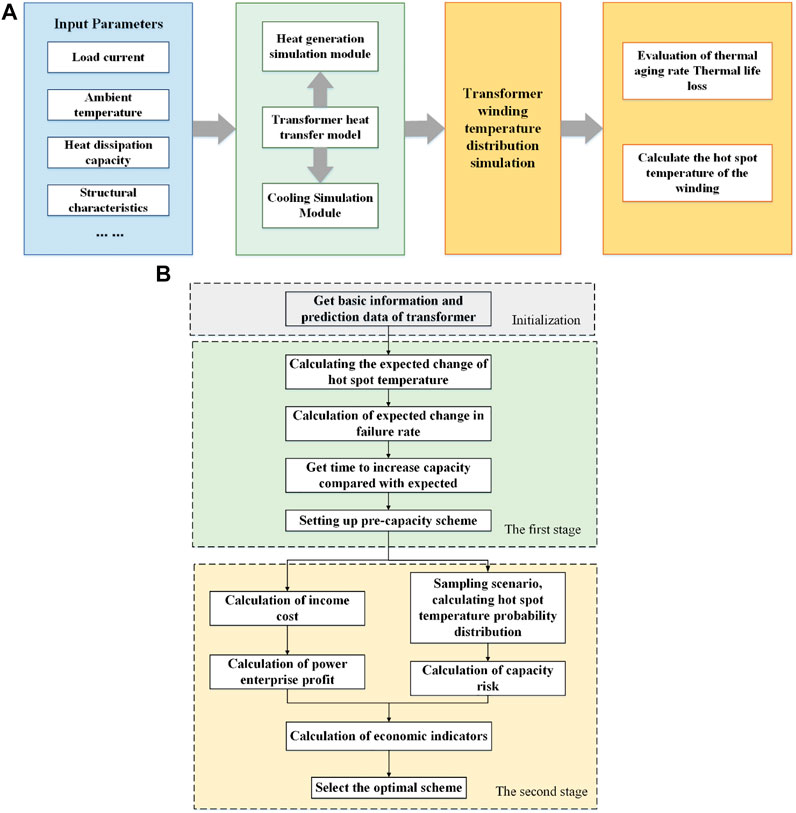
FIGURE 2. Online evaluation model and calculation process for load capacity assessment. (A) Online evaluation model for load capacity of oil-immersed power transformers, (B) calculation process of multi-risk transformer dynamic capacity increase strategy.
3.1.2 Computational model-based prediction
Computational model-based load capacity prediction methods mainly include statistical methods as well as machine learning methods. For statistical methods, the typical algorithms include Kalman filter, linear extrapolation and wavelet fractional solution method (Shen et al., 2016). Since statistical methods require high stability of time series, so it is difficult to reflect the non-linear influence of weather, events and other factors. In comparison, the machine learning algorithms such as fuzzy inference (Wang et al., 2022), support vector machines, artificial neural networks (Fan et al., 2022) and cluster analysis (Shen et al., 2016) have demonstrated their capability of improving the non-linear fitting ability of the models. It is believed that the computational model-based load capacity prediction methods have a good prospect. However, its ability of extracting deep features from actual highly fluctuating time series is still a problem to be solved in the future.
3.2 Dynamic load capacity enhancement
Dynamic load capacity enhancement refers to the operation of power transmission line or transformation equipment with load exceeding rated capacity under the premise of ensuring safety and reliability. The existing studies on dynamic load capacity enhancement of transformer pays more attention to the evaluation of HST and failure rate, and also combines economic indicators (Zhang et al., 2013; Dong et al., 2021). In this regard, the load curve, ambient temperature and relevant operation and maintenance information of the transformer are firstly obtained to determine the scenario and operating conditions of dynamic load. The equipment load scenario, service age and condition monitoring information are then taken to carry out thermal ageing for health state evaluation. Based on that, load constraints and corresponding threshold values can be determined for dynamic load capacity enhancement.
Besides, some researchers also use the Weibull model to calculate the real-time failure rate and risk of transformer when the load capacity increases (Qian et al., 2015). Under constrains like temperature, failure rate and economic investment, dynamical load capacity enhancement approaches are proposed (Zhang et al., 2017). The flow chart for this solution is shown in Figure 2B. The method is carried out in two stages: firstly, the working condition prediction data is used to obtain a pre-capacity enhancement solution under the HST temperature and fault rate constraints, with load reduction if any of the constraints are not met to ensure the safety and reliability of the capacity increase. Afterwards, the economics of each capacity enhancement decision is evaluated and the economically optimal solution is selected. Such method can be taken as a typical solution for dynamic transformer capacity enhancement.
4 Discussion
For a long time, studies on transformer load capability assessment mainly concentrated on remaining useful life of the insulation system as well as the economy investment. For the equipment installed in the new energy power system, the traditional load capacity evaluation approach becomes no longer adaptive due to the following issues:
• Some factors like historical health condition, dissipation mode and ambient parameters are not fully considered, since they can affect the load potential of the transformer.
• Load capability forecasting did not attract enough attention. With large-scale integration of new energy and impulsive loads like electrical vehicles, uncertainties in both source side and load side become a new factor for influence the load capability of the transformer.
• In terms of dynamic load capacity of transformer, the constraints are limited to HST, failure rate and investment. To meet the goal of carbon neutrality, the constraints of load capacity should consider the health status and power loss or carbon emission of transformer itself, residual life and other factors, and increase the constraints of dynamic difference.
In the new power system, the distribution transformer plays a vital role, dynamic capacity enhancement can improve the transformer load capacity, and more accurate load capability assessment model should be constructed to consider different factors. The objective function and the adaptive adjustment scheme of the cooling system should be determined to adapt to minimize transformer loss and maximize its insulation life.
Author contributions
SL proposed the idea and wrote the draft of the manuscript, XL orgnized the references, YK and QG proofread the manuscript.
Conflict of interest
The authors declare that the research was conducted in the absence of any commercial or financial relationships that could be construed as a potential conflict of interest.
Publisher’s note
All claims expressed in this article are solely those of the authors and do not necessarily represent those of their affiliated organizations, or those of the publisher, the editors and the reviewers. Any product that may be evaluated in this article, or claim that may be made by its manufacturer, is not guaranteed or endorsed by the publisher.
References
Dong, X., Zhang, S., Ruan, J., Du, Z., Deng, Y., and Cheng, S. (2021). Research and application of dynamic load assessment technology for oil-immersed power transformers. High. Volt. Technol. 47 (06), 1959–1968. doi:10.13336/j.1003-6520.hve.20210788
Fan, Q., Wen, Q., Lu, Q., Zhang, X., Xiao, N., and Liu, J. (2022). Transformer load forecasting and risk assessment based on main transformer load conditions. Electr. Meas. Instrum. 1-11. Available: http://kns.cnki.net/kcms/detail/23.1202.th.20220527.1737.006.html.
Feng, C., Li, Z., Shahidehpour, M., Wen, F., Liu, W., and Wang, X. (2018). Decentralized short-term voltage control in active power distribution systems. IEEE Trans. Smart Grid 9 (5), 4566–4576. doi:10.1109/tsg.2017.2663432
Feng, C., Liang, B., Li, Z., Liu, W., and Wen, F. (2022). Peer-to-peer energy trading under network constraints based on generalized fast dual ascent. IEEE Trans. Smart Grid 1. doi:10.1109/TSG.2022.3162876
Jiang, Z., Li, S., Ma, X., Li, X., Kang, Y., Li, H., et al. (2022). State estimation of regional power systems with source-load two-terminal uncertainties. Comput. Model. Eng. Sci. 132 (1), 295–317. doi:10.32604/cmes.2022.019996
Li, Y., Liu, N., Liang, J., Xu, S., Lin, D., Mu, H., et al. (2018). Oil-immersed transformer load capacity evaluation model based on temperature rise characteristics. Chin. J. Electr. Eng. 38 (22), 6737–6746. doi:10.13334/j.0258-8013.pcsee.180075
Liao, C., Ruan, J., Liu, C., Wen, W., and Wang, S. (2021). Three-dimensional electromagnetic-fluid-temperature field coupling analysis method for oil-immersed transformers. Power autom. Equip. 35 (9), 50–155.
Liu, Y., Li, X., Li, H., Yin, J., and Gao, S. (2020). Development of a 35 kV oil-immersed transformer with built-in distributed fiber optic sensing. High. Volt. Technol. 46 (06), 1886–1894. doi:10.13336/j.1003-6520.hve.20200615005
Lobo Ribeiro, A. B., Eira, N. F., Sousa, J. M., Guerreiro, P. T., and Salcedo, J. R. (2008). Multipoint fiber-optic hot-spot sensing network integrated into high power transformer for continuous monitoring. IEEE Sens. J. 8 (7), 1264–1267. doi:10.1109/jsen.2008.926926
Ma, H., Saha, T. K., Ekanayake, C., and Martin, D. (2015). Smart transformer for smart grid—Intelligent framework and techniques for power transformer asset management. IEEE Trans. Smart Grid 6 (2), 1026–1034. doi:10.1109/tsg.2014.2384501
Qian, H., Wang, Q., and Chen, X. (2015). Dynamic capacity increase scheduling of transmission cross sections considering multiple risks to the grid. China Electr. 48 (10), 60–64. doi:10.19595/j.cnki.1000-6753.tces.2014.04.029
Qian, Z., Sun, J., Yuan, K., and Sun, D. (2003). Online monitoring technology for hot spot condition of power transformer windings. High. Volt. Technol. 29 (9), 26–28. doi:10.13336/j.1003-6520.hve.2003.09.012
Ruan, J., Deng, Y., Huang, D., Duan, C., Gong, R., Quan, Y., et al. (2020). HST calculation of a 10 kV oil-immersed transformer with 3D coupled-field method. IET Electr. Power Appl. 14 (5), 921–928. doi:10.1049/iet-epa.2019.0469
Shen, C., Qin, J., Sheng, W., and Fang, H. (2016). Wavelet clustering-based short-term load forecasting method for distribution substations. Grid Technol. 40 (02), 521–526. doi:10.13335/j.1000-3673.pst.2016.02.027
Susa, D., and Nordman, H. (2009). A simple model for calculating transformer hot-spot temperature. IEEE Trans. Power Deliv. 24 (3), 1257–1265. doi:10.1109/tpwrd.2009.2022670
Swift, G., Molinski, T. S., and Lehn, W. (2001). A fundamental approach to transformer thermal modeling. I. Theory and equivalent circuit. IEEE Trans. Power Deliv. 16 (2), 171–175. doi:10.1109/61.915478
Tang, W., Qian, T., Huang, J., Lu, G., Wang, Y., and Luan, L. (2017). Improved thermoelectric analog model for transformer load capacity assessment. J. South China Univ. Technol. Nat. Sci. Ed. 45 (10), 71–77+86. doi:10.3969/j.issn.1000-565X.2017.10.010
Wang, C., Wang, Y., Ding, Z., Zheng, T., Hu, J., and Zhang, K. (2022). A based transformer-based method of multi-energy load forecasting in integrated energy system. IEEE Trans. Smart Grid. doi:10.1109/TSG.2022.3166600
Xie, Y., Lin, L., Song, Y., and Wang, S. (2016). Multi-physics field coupling calculation method for temperature rise of oil-immersed power transformer windings. Chin. J. Electr. Eng. 36 (21), 5957–5965. doi:10.13334/j.0258-8013.pcsee.160806
Zhang, X., Song, Z., and Yang, Z. (2013). An oil-immersed transformer fault rate model based on load factor and equipment detection information. Grid Technol. 37 (4), 1159–1165. doi:10.13335/j.1000-3673.pst.2013.04.019
Zhang, Y., He, D., Xu, Y., Guo, C., Du, X., and Bai, D. (2017). Short-term capacity increase decision method for oil-immersed transformers taking into account comprehensive risks. Power Syst. autom. 41 (13), 86–91+118. doi:10.7500/AEPS20161121007
Zhong, X., Ekanayake, C., Ma, H., and Saha, T. K. (2021). Ageing analysis of solar farm inverter transformers. IEEE Trans. Power Deliv. 36 (6), 3815–3824. doi:10.1109/TPWRD.2021.3049505
Zhou, B., Xu, X., Wing, S., Li, Canbing., Wu, Q., Zhang, C., et al. (2020). Thermodynamic modelling of buried transformer substations for dynamic loading capability assessment considering underground heat accumulative effect. Int. J. Electr. Power & Energy Syst. 121, 106153–106615. doi:10.1016/j.ijepes.2020.106153
Keywords: renewable energy, distribution transformer, hotspot temperature, load capacity, dynamic capacity
Citation: Li S, Li X, Kang Y and Gao Q (2022) Load capability assessment and enhancement for transformers with integration of large-scale renewable energy: A brief review. Front. Energy Res. 10:1002973. doi: 10.3389/fenrg.2022.1002973
Received: 25 July 2022; Accepted: 15 August 2022;
Published: 07 September 2022.
Edited by:
Changsen Feng, Zhejiang University of Technology, ChinaReviewed by:
Zhanhong Wei, Lanzhou University of Technology, ChinaYing Han, Southwest Jiaotong University, China
Copyright © 2022 Li, Li, Kang and Gao. This is an open-access article distributed under the terms of the Creative Commons Attribution License (CC BY). The use, distribution or reproduction in other forums is permitted, provided the original author(s) and the copyright owner(s) are credited and that the original publication in this journal is cited, in accordance with accepted academic practice. No use, distribution or reproduction is permitted which does not comply with these terms.
*Correspondence: Shuaibing Li, lishuaibing1105@163.com