- 1Department of Epidemiology and Biostatistics, School of Public Health, Tianjin Medical University, Tianjin, China
- 2Tianjin Key Laboratory of Environment, Nutrition and Public Health, Tianjin Medical University, Tianjin, China
- 3Tianjin Center for International Collaborative Research on Environment, Nutrition and Public Health, Tianjin Medical University, Tianjin, China
- 4Project Office, Tianjin Women and Children’s Health Center, Tianjin, China
- 5School of Public Health, Shanxi Medical University, Shanxi, China
- 6Population Cancer Research Program and Department of Pediatrics, Dalhousie University, Halifax, NS, Canada
- 7Chronic Disease Epidemiology Laboratory, Pennington Biomedical Research Center, Baton Rouge, LA, United States
- 8Department of Medicine and Therapeutics and Li Ka Shing Institute of Health Sciences, Prince of Wales Hospital, The Chinese University of Hong Kong, Hong Kong, Hong Kong SAR, China
- 9Department of Toxicology and Sanitary Chemistry, School of Public Health, Tianjin Medical University, Tianjin, China
- 10Scientific Research Platform of the Second School of Clinical Medicine & Key Laboratory of 3D Printing Technology in Stomatology, Guangdong Medical University, Dongguan, China
Aims: The study aimed to explore additive interactions of CDKAL1 rs7747752 and GUDCA/DCA for GDM risk and whether the interactive effects on the risk of GDM was mediated via increasing lysophosphatidylcholines (LPC) 18:0 and/or saturated fatty acid (SFA) 16:0.
Methods: A 1:1 age-matched study nested in a prospective cohort of pregnant women (207 pairs) was organized in Tianjin, China. Additive interactions were used to test interaction effects while mediation analyses and Sobel tests were used to test mediation effects of LPC18:0 and SFA16:0 between copresence of rs7747752 and low GUDCA/DCA, and GDM risk.
Results: The CDKAL1 rs7747752 was associated with GDM (P<0.05). The rs7747752 C polymorphism markedly enhanced ORs of low GUDCA from 4.04 (0.72-22.8) to 9.02 (1.63-49.7) and low DCA from 1.67 (0.68-4.11) to 4.24 (1.84-9.76), both with significant additive interactions. Further adjustment for LPC18:0 attenuated the interactive effects of rs7747752 and low DCA, with a significant mediation effect (P=0.003). High SFA16:0 did not mediate the interactive effects of rs7747752 and low DCA/GUDCA on GDM risk.
Conclusions: The CDKAL1 rs7747752 C carrier status and low GUDCA/DCA had significant additive interactions on the risk of GDM with the effect from interaction with DCA being partially mediated via increasing LPC18:0.
Introduction
The prevalence of gestational diabetes mellitus (GDM) has been rapidly increasing worldwide (1). GDM is not only associated with perinatal adverse outcomes but also predispose women to increased risk of diabetes and cardiovascular disease in later life, and their offspring to increased risk of childhood obesity (2, 3). On the other hand, intensive management of GDM does not have a detectable effect on the risk of postpartum diabetes in the mothers (4) and childhood obesity in their offspring (5). How to prevent GDM has become increasingly important to reduce the burden of GDM. However, our meta-analysis of 29 randomized controlled trials shows that lifestyle interventions within 15 weeks of pregnancy can only achieve as low as 20% reduction in risk of GDM while later lifestyle interventions are ineffective in reducing the risk (6). Indeed, a better understanding of the pathophysiology of GDM and identification of its novel biomarkers are critically important for the prediction and prevention of GDM in early pregnancy.
GDM is a complex disease determined by a constellation of factors, including genetic, metabolic, and other environmental factors (7). Among genetic factors, single nucleotide polymorphisms (SNP) within the cyclin-dependent kinase 5 regulatory subunit-associated protein1-like 1 (CDKAL1) locus was found to be strongly associated with GDM by genome-wide association analysis (GWAS) and dozens of replication studies in different populations (8–10). CDKAL1 gene is located on the short arm of human chromosome 6 and encodes a protein of 579 amino acids, which is indeed a member of the methylthiotransferase that specifically modifies transfer ribonucleic acid (tRNA) Lys in mammals (11). The CDKAL1 gene plays a role in the cell cycle control of beta cells by inhibiting the activity of cyclin-dependent kinase 5 (CDK5) and acting as a tRNA-modifying enzyme (12). The association between CDKAL1 rs7747752 polymorphism and GDM was also reported in Chinese pregnant women (8).
In addition to genetic origin and environmental factors, various metabolic factors, such as bile acids (BAs) and saturated fatty acids (SFAs), can act as risk factors (13, 14). In this connection, our group found that serum glycoursodeoxycholic acid (GUDCA) ≤ 0.07 nmol/mL and deoxycholic acid (DCA) ≤ 0.28 nmol/mL in early pregnancy were independently associated with markedly increased risk of GDM in Chinese pregnant women (15). Interestingly, adjustment for LPC18:0 attenuated the risk associations of both low DCA and low GUDCA with GDM while low DCA and low GUDCA enhanced the risk association between LPC18:0 and GDM (16). The interaction between genetic disposition and metabolic factors plays a critical role in the development of GDM (17). Indeed, the CDKAL1 gene was related to defects in the conversion of proinsulin (18) and insulin response under glucose stimulation (19, 20). It is known that defection of insulin response reduces its ability to inhibit lipolysis in adipose tissue, further resulting in increased flow of SFAs into the circulation (21). In line with these findings from mechanistic investigations, our group reported that high SFA16:0 played a vital role in the risk of GDM, which had a significant interactive effect with CDKAL1 rs7747752 on the risk of GDM (14). In addition, an animal study showed that defection of CDKAL1 gene led to impaired insulin secretion and longitudinal fluctuations in insulin sensitivity during high-fat feeding in mice (22). Notably, BAs, as cholesterol-derived metabolites, have a well-established role in the digestion and absorption of dietary fats (23); furthermore, BAs also affect insulin secretion (24, 25). This observation suggests that BAs and CDKAL1 variants may have a synergistic effect on the risk of GDM, possibly being mediated by LPC18:0 or SFA16:0. Indeed, it is worthwhile to explore whether abnormal BAs have any interactions with CDKAL1 gene variants towards increased risk of GDM. It is also quite interesting to understand potential interrelationships among CDKAL1, BAs, SFAs, and LPCs and their roles in the etiology of GDM.
Using an age-matched case-control study nested in a large population-based cohort of pregnant women in Tianjin, China, this analysis aimed to explore 1) additive interactions between CDKAL1 rs7747752 polymorphism and low GUDCA/DCA for the risk of GDM; and 2) whether the additive interactive effect if any between rs7747752 polymorphism and low GUDCA/DCA on the risk of GDM was mediated via LPC18:0 and/or SFA16:0.
Materials and Methods
Research Design and Population
The design and method of this study have been described previously (26). To be brief, we set up a prospective cohort study of 22 302 pregnant women at their first antenatal care with median 10th gestational weeks in Tianjin, China, from October 2010 to August 2012. The ethics of the study protocol was approved by the Ethics Committee of Tianjin Women and Children’s Health Center (TWCHC), and written informed consent was obtained before data collection.
A two-step screening procedure was used to identify GDM. First, a 1-h 50-g glucose challenge test (GCT) was performed on pregnant women at 24-28th gestational weeks in the primary hospital. Second, a 2-hour 75-g oral glucose tolerance test (OGTT) was performed on pregnant women with GCT ≥ 7.8 mmol/L at the GDM clinic in TWCHC. The diagnosis of GDM is based on the cutoff points of the International Association of Diabetes and Pregnancy Study Group (27). Of the 22 302 participants, 2 991 pregnant women donated fasting blood samples. We finally included 207 GDM cases and 207 non-GDM controls who were matched by maternal age (± 1 year) (15). Data from the 207 pairs of women with high-quality SNP (28) were used to explore the research questions of this study. The flowchart of the study participants was available elsewhere (14).
Data Collection Procedures
Data were collected during the first antenatal care visit, GCT, OGTT, and the postpartum period, which has been described in detail (26). Demographic, clinical and lifestyle information included age, weight, height, systolic/diastolic blood pressure (SBP/DBP), ethnicity, education level, parity, family history of diabetes, in first degree relatives, smoking and drinking habits before and during pregnancy.
Measurement of Serum BAs, LPCs and SFAs
Liquid chromatography-tandem mass spectrometry (LC–MS/MS) was used to assay the concentrations of serum BAs, LPCs, and SFAs. The detailed measurement methods of serum BAs, LPCs, and SFAs were available elsewhere (14–16).
Genotyping
Genotyping was conducted using the Illumina Infinium® Global Screening Array and genotype data was imputed using minimal 3 with the 1000 Genomes Project phase 3 v 5 as reference panel. Genotyping data from specific candidate SNP (rs7747752) were extracted from the genome-wide genotyping data. The overall genotype call rate was 99.4%.
Statistical Analysis
Power and sample size analysis was performed using PASS 15 (NCSS, LLC. Kaysville, Utah, USA). In the 1:1 age-matched case-control study, we assumed that the probability of exposure among sampled control patients is 10% and the correlation coefficient for exposure between matched case and control patents is 0.2, and type I error is set at 0.05. The sample size required to achieve 85% power for an odds ratio (OR) of 2.0 is 256 (128 GDM and 128 control). Therefore, the current sample size of our study (n=414) had more than 85% power to detect the assumed risk association.
All other statistical analyses were performed using Statistical Analysis System (SAS) release 9.4 (SAS Institute, Cary, NC). Quantitative data were compared between the GDM group and the non-GDM group, using the paired Student’s t-test or Wilcoxon signed-rank test. The categorical data were compared using the McNemar test or Fisher’s exact test. In addition, Spearman correlation analysis was used to calculate the correlation coefficients of these serum BAs and rs7747752 with neonatal birth weight. In addition, Kruskal-Wallis test was used to test the differences of serum levels of BAs between different rs7747752 genotypes. In this analysis, a P-value < 0.05 was regarded to be statistically significant.
In our previous analysis, we detected that GUDCA ≤ 0.07 nmol/mL, DCA ≤ 0.28 nmol/mL, LPC18:0 ≥ 18.0 nmol/mL and SFA16:0 ≥ 17.1 nmol/mL were independently associated with markedly increased risk of GDM in restricted cubic spline analysis (14–16). In this analysis, we used the same cutoff points of GUDCA, DCA, LPC18:0 and SFA16:0 to define low GUDCA, low DCA, high LPC18:0 and high SFA16:0. Conditional logistic regressions were used to obtain the ORs and their 95% confidence intervals (CIs) of rs7747752 and GUDCA/DCA for the risk of GDM in unadjusted models and adjusted models. The adjusted models considered confounding effects of traditional risk factors of GDM, including pre-pregnancy body mass index (BMI), family history of diabetes in first degree relatives, SBP, current smoker before pregnancy, and weight gain to the time of GCT. Next, the combined effects of rs7747752 genotypes (CC vs. CG vs. GG) and low serum levels of GUDCA/DCA on GDM susceptibility were examined to explore whether the rs7747752 and low GUDCA/DCA have potential interactive effects on the risk of GDM. Then, based on the results of combined effects of rs7747752 genotypes (CC vs. CG vs. GG) and low GUDCA/DCA on GDM, we further tested the additive interactions between rs7747752 C risk allele and low GUDCA/DCA for GDM. Three measures, i.e., relative excess risk due to the interaction (RERI), attributable proportion due to the interaction (AP), and synergy index (SI) were used to judge additive interactions (29). Any of RERI > 0, AP > 0 or SI > 1 indicates a significant additive interaction.
In order to shed light on the potential mechanisms of the additive interactions between CDKAL1 rs7747752 C allele and low GUDCA/DCA on the risk of GDM, we further conducted mediation analyses to examine whether high LPC18:0 and/or SFA16:0 can account for the association between copresence of CDKAL1 rs7747752 C allele carrier status and low GUDCA/DCA, and the increased risk of GDM. First, we estimated the ORs of copresence of rs7747752 and low GUDCA/DCA for high LPC18:0 and high SFA16:0, and the ORs of high LPC18:0 and high SFA16:0 for GDM. Then, we calculated the ORs of copresence of rs7747752 and low GUDCA/DCA for GDM after adjusting for high LPC18:0 and high SFA16:0. At last, Sobel test was used to assess the mediation effects of LPC18:0 and SFA16:0 (30).
Results
Characteristics of the Research Participants
The clinical and biochemical information of the study participants was shown in Table 1. The mean age of participants at the first antenatal care visit was 29.24 ± 3.04 standard deviation (SD) years. There were no differences in height, ethnicity, education level, parity, weight gain to GCT, smoking and drinking habits before and during pregnancy among the controls and GDM groups (P > 0.05). Women with GDM had significantly higher weight, BMI, SBP, DBP and GCT glucose levels than the controls (P < 0.05). Women with GDM also had a higher proportion of family history of diabetes in first degree relatives than the non-GDM group. Women with GDM had a lower serum level of GUDCA and DCA while had a high serum level of LPC18:0 and SFA16:0, compared with non-GDM women. The frequencies of the heterozygote (CG) and homozygous (CC) of the CDKAL1 rs7747752 were found to be significantly higher in women with GDM than in the controls (non-GDM) (P < 0.05). Whereas, we failed to find any significant differences of serum levels of GUDCA and DCA between rs7747752 genotypes (Table S1).
In addition, Spearman correlation analysis was used to calculate the correlation coefficients of these serum BAs and rs7747752 with neonatal birth weight. The correlation coefficients of DCA, GUDCA and rs7747752 with birth weight were 0.063 (P = 0.199), -0.022 (P = 0.658) and -0.017 (P = 0.728), respectively, all being not significant.
Associations of CDKAL1 rs7747752 and Low Serum Levels of GUDCA/DCA With GDM
The GUDCA ≤ 0.07 nmol/mL was associated with increased risk of GDM (OR: 5.84, 95% CI: 2.13-16.0) after adjustment for traditional risk factors, including pre-pregnancy BMI, family history of diabetes in first degree relatives, SBP, current smoker before pregnancy and weight gain to the time of GCT. Similarly, after adjustment for traditional risk factors, DCA ≤ 0.28 nmol/mL was also associated with increased risk of GDM (OR: 2.28, 95% CI: 1.43-3.64). In the cohort, the C allele frequency for rs7747752 was 49.89%. The CDKAL1 rs7747752 C allele was significantly associated with GDM in the unadjusted model (OR: 1.48, 95% CI: 1.12-1.96) and adjusted model (OR: 1.76, 95% CI: 1.26-2.44). After adjustment for traditional risk factors, heterozygote (CG) and homozygote (CC) genotypes of CDKAL1 rs7747752 were all associated with increased risk of GDM with ORs being 1.95 (95% CI: 1.15-3.31) and 3.07 (95% CI: 1.59-5.95), respectively. In dominant and recessive models, the ORs of the genotype distribution of rs7747752 in the adjusted model were 2.19 (95% CI: 1.31-3.65) and 1.88 (95% CI: 1.13-3.15), respectively (Table 2).
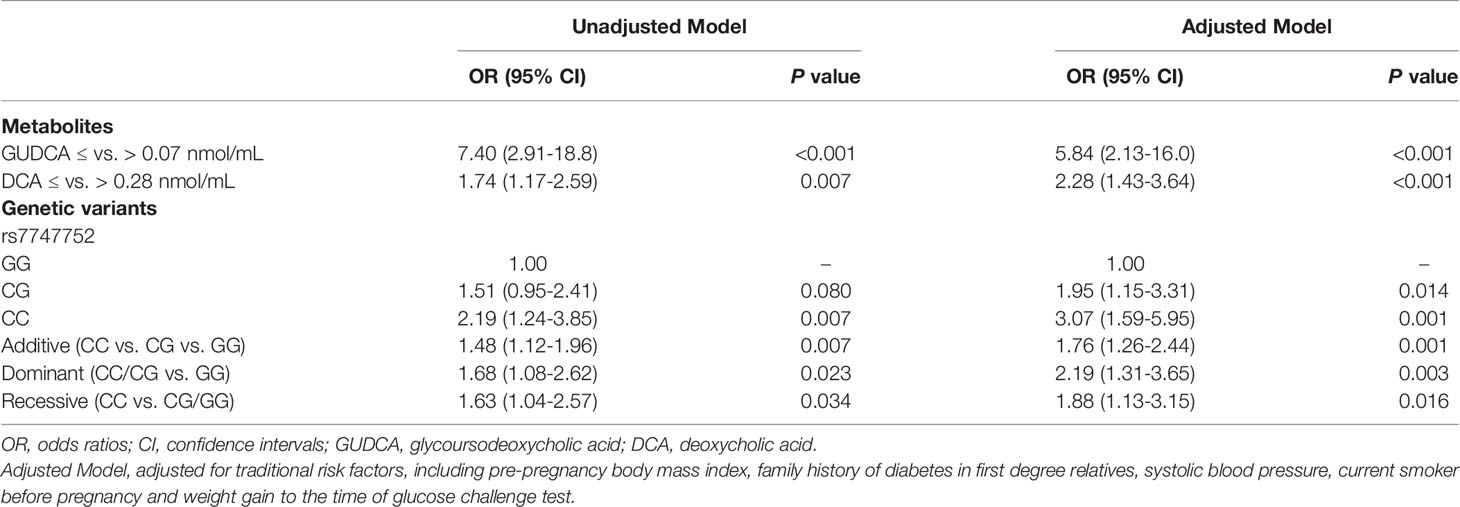
Table 2 Odds ratios of the CDKAL1 rs7747752 and bile acid metabolisms for increased risk of gestational diabetes mellitus.
Combined Effects With rs7747752 Genotypes (CC vs. CG vs. GG) and Low Serum Levels of GUDCA/DCA for the Risk of GDM
Using the GG genotype of rs7747752 and serum GUDCA > 0.07 nmol/mL as the reference, GUDCA ≤ 0.07 nmol/mL combined with CG and CC were associated with significantly increased risk of GDM after adjustment for traditional GDM risk factors, and the ORs were 9.58 (95% CI: 1.60-57.5) and 16.5 (95% CI: 2.59-106), respectively. In the same vein, using the GG genotype of rs7747752 and DCA > 0.28 nmol/mL as the reference, the combinations of rs7747752 CG or CC genotypes and DCA ≤ 0.28 nmol/mL were associated with markedly increased risk of GDM and ORs were 3.51 (95% CI: 1.50-8.24) and 8.06 (95% CI: 2.85-22.8), respectively (Table 3).
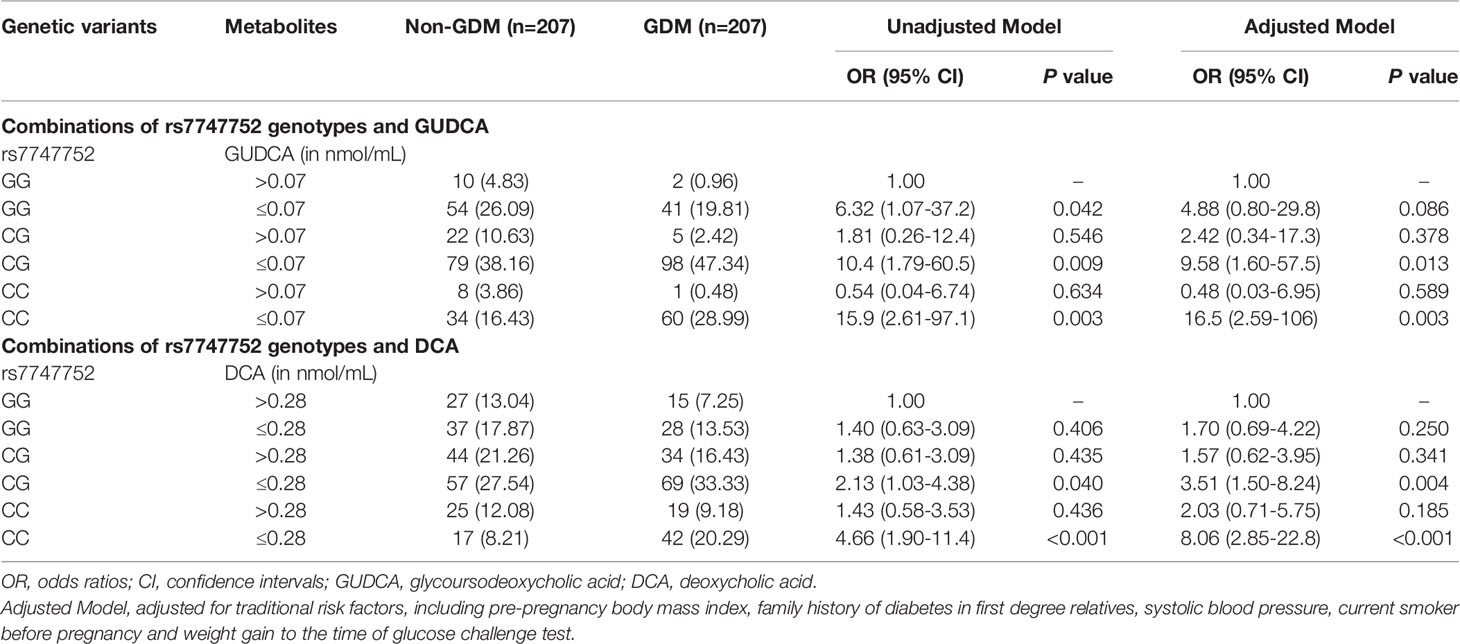
Table 3 Risk associations of combinations of rs7747752 genotypes (CC vs. CG vs. GG) and low serum levels of GUDCA/DCA with gestational diabetes mellitus.
Additive Interactions Between rs7747752 C Allele (C vs. G) and Low Serum Levels of GUDCA/DCA for the Risk of GDM
Using rs7747752 G allele and GUDCA > 0.07 nmol/mL as the reference, the presence of rs7747752 C allele markedly increased the OR of GUDCA ≤ 0.07 nmol/mL for GDM in adjusted model from 4.04 (95% CI: 0.72-22.8) for GUDCA ≤ 0.07 nmol/mL alone to 9.02 (95% CI: 1.63-49.7) for the presence of both. There was a significant additive interaction between CDKAL1 rs7747752 C allele and GUDCA ≤ 0.07 nmol/L for GDM (AP: 0.50, 95% CI: 0.17-0.83).
Similarly, CDKAL1 rs7747752 C allele also markedly increased OR of DCA ≤ 0.28 nmol/mL for GDM in adjusted model from 1.67 (95% CI: 0.68-4.11) for DCA ≤ 0.28 nmol/mL alone to 4.24 (95% CI: 1.84-9.76) for copresence of both. The additive interaction was also significant (AP: 0.46, 95%CI: 0.09-0.83) (Table 4).
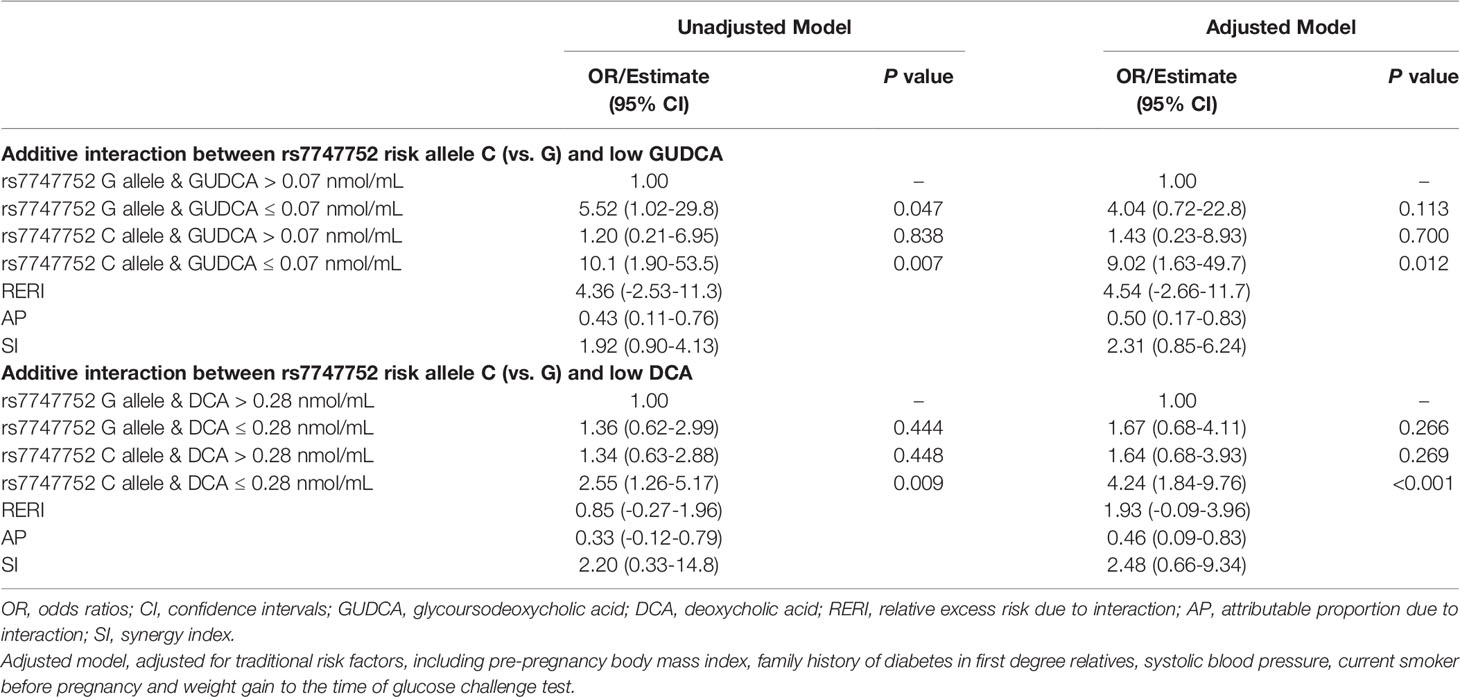
Table 4 Additive interactions between rs7747752 C allele (C vs. G) and low GUDCA/DCA for the risk of gestational diabetes mellitus.
Mediation Effects of LPC18:0 and SFA16:0 for Copresence of rs7747752 C Allele (C vs. G) and Low Serum Levels of GUDCA/DCA to the Risk of GDM
In adjusted model analysis, copresence of rs7747752 C allele and DCA ≤ 0.28 nmol/mL was associated with markedly increased risk of high LPC18:0 (OR: 4.51, 95% CI: 1.79-11.3). Adjustment for LPC18:0 greatly attenuated the OR of copresence of rs7747752 C allele and DCA ≤ 0.28 nmol/mL from 4.24 (1.84-9.76) to 2.67 (0.71-9.99). The mediation effect of LPC18:0 on the risk association of copresence of rs7747752 C risk allele and DCA ≤ 0.28 nmol/mL with GDM was statistically significant (P of Sobel test: 0.003) (Table 5). On the other hand, the mediation effect of LPC18:0 on the risk association of rs7747752 C risk allele and GUDCA ≤ 0.07 nmol/mL with GDM was non-significant (P of Sobel test: 0.114). Whereas, further adjustment for SFA16:0 markedly enhanced the interactive effects of rs7747752 C risk allele and low GUDCA on the risk of GDM up to 7.20 (95% CI: 0.87-59.4), and slightly enhanced the risk association of rs7747752 C risk allele and low DCA with GDM (OR: 4.36, 95% CI: 1.83-10.4), both without any mediation effects (Table S2).
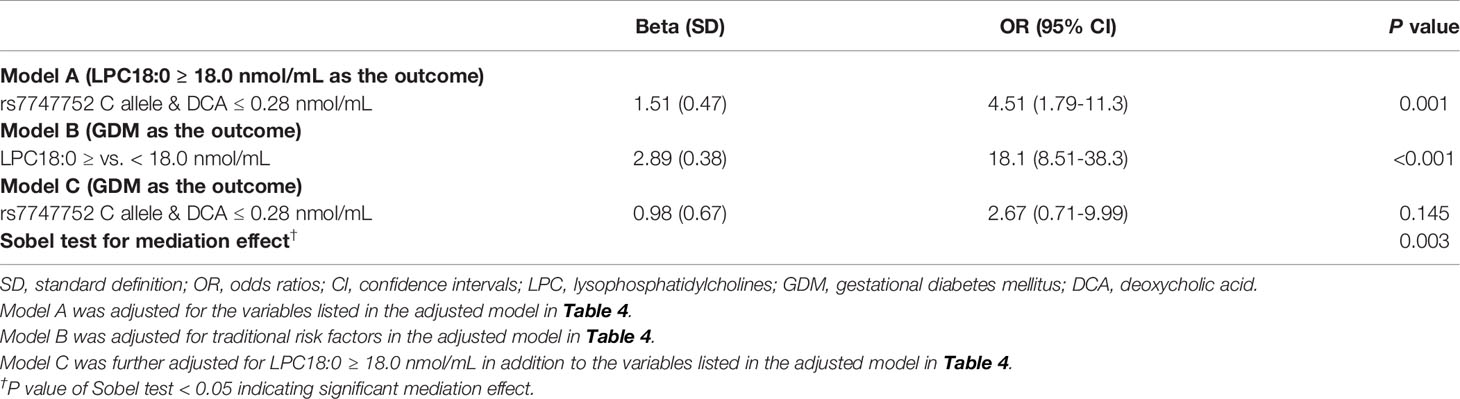
Table 5 Mediation effect of LPC18:0 for interaction between rs7747752 C allele (C vs. G) and low DCA to increased risk of gestational diabetes mellitus.
Discussion
Our study found significant additive interactions between CDKAL1 rs7747752 C allele carrier status and low serum levels of GUDCA and DCA for the markedly increased risk of GDM in Chinese pregnant women. The additive interactive effect of CDKAL1 rs7747752 C allele carrier status and low serum levels of DCA on GDM was partially mediated via high LPC18:0. However, we failed to confirm that the copresence of CDKAL1 rs7747752 C allele carrier status and low GUDCA on the risk of GDM was also mediated via high LPC18:0. In addition, the additive interactions between CDKAL1 rs7747752 C allele carrier status and low serum levels of GUDCA and DCA on GDM risk were independent of high SFA16:0.
The role of BAs in the regulation of glucose metabolism is a hot topic of recent diabetes research. Several studies in pregnant women have attempted to address associations between BAs and GDM with inconsistent findings. A nested case-control study of 131 women with GDM and 138 controls in early pregnancy did not find significant differences in DCA, ursodeoxycholic acid (UDCA), and GUDCA between GDM and non-GDM women, but did observe that the primary BAs were down-regulated while some other secondary BAs were up-regulated in GDM versus non-GDM in the univariate analysis (31). Two other studies also did not detect a significant difference in DCA and UDCA between GDM women and their control group at 28th gestational weeks (13, 32). Using the restricted cubic spline technique, our group found that GUDCA and DCA had clear threshold effects on the risk of GDM, with the GUDCA ≤ 0.07 nmol/mL and the DCA ≤ 0.28 nmol/mL being associated with markedly increased risk of GDM (15). Our findings further indicated that women in low serum levels of GUDCA and DCA with rs7747752 C risk alleles had markedly increased the risk of GDM. It is worthwhile to investigate whether population differences in proportions of CDKAL1 rs7747752 C allele carriers contributed to the inconsistent findings regarding the association between BA metabolites and the risk of GDM in different studies.
CDKAL1 gene has a strong association with GDM among the genes identified to date. CDKAL1 genetic variants also have been shown to predict GDM and related glycemic traits (10), suggesting that it might have a synergistic effect with other risk factors. The rs7747752 under study was significantly associated with increased GDM risk. Similar to our findings, another study also found that Chinese women with the rs7747752 polymorphism were predisposed to a high risk of GDM (8). Previous studies have reported significant interaction effects of CDKAL1 with lifestyle interventions, SFAs, and vitamin D on GDM risk (14, 17, 33). In a recent study, our group found that CDKAL1 genetic variants had a significant additive interaction with serum SFAs, resulting in a markedly increased risk of GDM (14). The interaction between both risk factors may suggest that GDM develops when impaired beta-cell function cannot produce enough insulin in response to increased insulin resistance as manifested by high SFA16:0. In this analysis, we further found that the CDKAL1 rs7747752 C allele carrier status had significant additive interactions with low GUDCA and low DCA for the risk of GDM, independent of high SFA16:0. Interestingly, LPC18:0 mediated the interactive effect of CDKAL1 rs7747752 and low DCA on the risk of GDM.
To our knowledge, our study was the first that investigated additive interactions between the CDKAL1 genetic variants (rs7747752) and low serum levels of GUDCA/DCA for the risk of GDM. We also observed that LPC18:0 but not SFA16:0 mediated the interactive effects of rs7747752 and low DCA on the risk of GDM. The functions of CDKAL1 and methylthiotransferase in the regulation of metabolism are not fully understood, so do the mechanisms underlying the additive interactions between CDKAL1 rs7747752 and low GUDCA/DCA. Recent studies have suggested several biological links between BAs and glucose regulation. First, a biological link between decreased DCA/GUDCA and GDM is plausible. Some BAs can activate FXR in the intestine stimulate the synthesis of fibroblast growth factor (FGF) 15/19 (34) and upregulate expression of pancreatic beta cells (35), which exerts pleiotropic effects on hepatic BAs metabolism as well as lipid, protein, and glucose metabolism (34). What’s more, BAs-mediated TGR-5 signaling increases the release of GLP-1, which augments glucose-stimulated insulin secretion from pancreatic beta cells (36). Notably, the CDKAL1 gene is tightly associated with impaired beta-cell function (37) and insulin secretion (38), thus being associated with increased risk of GDM. Hence, it is possible that copresence of low GUDCA/DCA and CDKAL1 genetic variants markedly increased the risk of GDM via impaired beta-cell function. Second, multiple lines of evidence support a pathway from the additive interactions of low DCA/GUDCA and CDKAL1 to increased LPC18:0 could play a role in the etiology of GDM. A mouse study found that increased intestinal taurine-beta-muocholic acid, an antagonist of FXR, reduced high-fat diet-induced increase in LPCs by inhibiting the activity of FXR (39). In this regard, another study showed that the biological link of gut microbiota-GUDCA-FXR was related to glucose intolerance (40). Furthermore, LPCs, the active metabolites of SFA16:0, promoted insulin resistance and cell death in diabetes by activating endoplasmic reticulum (ER) stress (41, 42). In this regard, our study found that high SFA16:0 enhanced the risk association of copresence of low DCA/GUDCA and CDKAL1 genetic variants with the risk of GDM. Thus, it is also possible that impaired beta-cell function cannot produce enough insulin to cope with increased insulin resistance as caused by high LPC18:0, thereby triggering a high risk of GDM. Hence, the markedly increased susceptibility to GDM due to exposure to both CDKAL1 rs7747752 C allele and low GUDCA/DCA is biologically plausible although we are not sure whether impaired insulin secretion or increased insulin resistance plays a dominant role. Indeed, molecular mechanisms underlying the interactions between rs7747752 and low GUDCA/DCA for GDM warrant further investigations.
Our findings have public health implications. GDM begets adverse short- and long-term health risks to the mother, developing fetus, and their offspring. Thus, understanding the pathophysiology of GDM and identifying potentially modifiable risk factors and biomarkers for early diagnosis of GDM is essential. In the contention, our study corroborated the link of the CDKAL1 rs7747752 with GDM in Chinese pregnant women and confirmed that the additive interactions between rs7747752 and low serum levels of GUDCA/DCA for markedly increased risk of GDM, were independent of serum levels of SFA16:0. Notably, the additive interaction effect between rs7747752 and low DCA on the risk of GDM was partly mediated via increasing levels of LPC18:0. These risk genetic and metabolomics markers may be useful in the identification of women at high risk of GDM in early pregnancy.
Our research has several limitations. First, our study was a case-control study nested in a population-based prospective cohort of pregnant women in Tianjin, China. Our findings need to be replicated in other Chinese and non-Chinese cohorts of pregnant women. Second, due to busy clinical settings and a limited budget, lifestyle factors such as dietary habits were not collected. Third, we used a two-step GDM screening procedure to identify GDM and some GDM cases might have been missed. Fourth, universal OGTT in early pregnancy was not conducted due to tight budget and we cannot exclude the possibility that some women with GDM may have had prepregnancy impaired glucose tolerance.
In conclusion, we found that CDKAL1 rs7747752 and serum GUDCA ≤ 0.07 nmol/mL and DCA ≤ 0.28 nmol/mL had significant interactive effects on the risk of GDM in Chinese pregnant women, independent of serum levels of SFA16:0. The additive interaction effect between CDKAL1 rs7747752 and low DCA on GDM was partially mediated via increasing levels of LPC18:0. The discovery of the interactions between CDKAL1 rs7747752 C risk allele and low DCA/GUDCA is an important step towards precision prevention of GDM in early pregnancy. The additive interaction has potential values in the prediction of GDM in early pregnancy that may benefit from specific intervention if validation studies can confirm our findings in other cohorts of pregnant women populations. It is also warranted to investigate the molecular roles of CDKAL1 gene and methylthiotransferase in the regulation of metabolic and signaling pathways of BAs, SFAs, and LPCs for increased risk of GDM for better understanding of the etiology of GDM.
Data Availability Statement
The data and code used during the current study are available from the corresponding author on reasonable request.
Ethics Statement
The studies involving human participants were reviewed and approved by Ethics Committee of Tianjin Women and Children’s Health Center (TWCHC). The patients/participants provided their written informed consent to participate in this study.
Author Contributions
XYang, YW and ZF conceived the idea and designed the study. JLeng and WL collected the clinical data. ZF measured the metabolomics and integrated metabolomics data. YW measured the genetics and integrated the genetic data. HW and JLi analyzed the data and wrote the first draft. All the authors gave critical comments and edited the manuscript. XYang and HW took full responsibility for the work, including the study design, access to the data, and decision to submit. All authors contributed to the article and approved the submitted version.
Funding
This work was supported by the National Natural Science Foundation of China (Grant No: 81870549), the National Key Research and Development Program of China (Grant No: 2019YFA0802300); the Engaged Talents of Guangdong Medical University in 2017 (Grant No: 2XB17028) and the Sailing Plan of Guangdong Province (Grant No: 4YF16001G).
Conflict of Interest
The authors declare that the research was conducted in the absence of any commercial or financial relationships that could be construed as a potential conflict of interest.
Publisher’s Note
All claims expressed in this article are solely those of the authors and do not necessarily represent those of their affiliated organizations, or those of the publisher, the editors and the reviewers. Any product that may be evaluated in this article, or claim that may be made by its manufacturer, is not guaranteed or endorsed by the publisher.
Acknowledgments
The authors thank all the health professionals of Tianjin Antenatal Network for their involvement and contribution to the study. XYang was the guarantor of this manuscript.
Supplementary Material
The Supplementary Material for this article can be found online at: https://www.frontiersin.org/articles/10.3389/fendo.2022.808956/full#supplementary-material
References
1. Wang H, Li N, Chivese T, Werfalli M, Sun H, Yuen L, et al. IDF Diabetes Atlas: Estimation of Global and Regional Gestational Diabetes Mellitus Prevalence for 2021 by International Association of Diabetes in Pregnancy Study Group’s Criteria. Diabetes Res Clin Pract (2022) 183:109050. doi: 10.1016/j.diabres.2021.109050
2. Song C, Lyu Y, Li C, Liu P, Li J, Ma RC, et al. Long-Term Risk of Diabetes in Women at Varying Durations After Gestational Diabetes: A Systematic Review and Meta-Analysis With More Than 2 Million Women. Obes Rev (2018) 19(3):421–9. doi: 10.1111/obr.12645
3. Gao M, Cao S, Li N, Liu J, Lyu Y, Li J, et al. Risks of Overweight in the Offspring of Women With Gestational Diabetes at Different Developmental Stages: A Meta-Analysis With More Than Half a Million Offspring. Obes Rev (2022) 23(3):e13395. doi: 10.1111/obr.13395
4. Li N, Yang Y, Cui D, Li C, Ma RCW, Li J, et al. Effects of Lifestyle Intervention on Long-Term Risk of Diabetes in Women With Prior Gestational Diabetes: A Systematic Review and Meta-Analysis of Randomized Controlled Trials. Obes Rev (2021) 22(1):e13122. doi: 10.1111/obr.13122
5. Li J, Liu J, Zhang C, Liu G, Leng J, Wang L, et al. Effects of Lifestyle Intervention of Maternal Gestational Diabetes Mellitus on Offspring Growth Pattern Before Two Years of Age. Diabetes Care (2021) 44(3):e42–4. doi: 10.2337/dc20-2750
6. Song C, Li J, Leng J, Ma RC, Yang X. Lifestyle Intervention Can Reduce the Risk of Gestational Diabetes: A Meta-Analysis of Randomized Controlled Trials. Obes Rev (2016) 17(10):960–9. doi: 10.1111/obr.12442
7. Saxena R, Voight BF, Lyssenko V, Burtt NP, de Bakker PI, Chen H, et al. Genome-Wide Association Analysis Identifies Loci for Type 2 Diabetes and Triglyceride Levels. Sci (New York NY) (2007) 316(5829):1331–6. doi: 10.1126/science.1142358
8. Wang K, Chen Q, Feng Y, Yang H, Wu W, Zhang P, et al. Single Nucleotide Polymorphisms in CDKAL1 Gene Are Associated With Risk of Gestational Diabetes Mellitus in Chinese Population. J Diabetes Res (2019) 2019:3618103. doi: 10.1155/2019/3618103
9. Tarnowski M, Malinowski D, Pawlak K, Dziedziejko V, Safranow K, Pawlik A. GCK, GCKR, FADS1, DGKB/TMEM195 and CDKAL1 Gene Polymorphisms in Women With Gestational Diabetes. Can J Diabetes (2017) 41(4):372–9. doi: 10.1016/j.jcjd.2016.11.009
10. Powe CE, Kwak SH. Genetic Studies of Gestational Diabetes and Glucose Metabolism in Pregnancy. Curr Diabetes Rep (2020) 20(12):69. doi: 10.1007/s11892-020-01355-3
11. Pierrel F, Douki T, Fontecave M, Atta M. MiaB Protein is a Bifunctional Radical-S-Adenosylmethionine Enzyme Involved in Thiolation and Methylation of tRNA. J Biol Chem (2004) 279(46):47555–63. doi: 10.1074/jbc.M408562200
12. Liu NJ, Xiong Q, Wu HH, Li YL, Yang Z, Tao XM, et al. The Association Analysis Polymorphism of CDKAL1 and Diabetic Retinopathy in Chinese Han Population. Int J Ophthalmol (2016) 9(5):707–12. doi: 10.18240/ijo.2016.05.12
13. Gao J, Xu B, Zhang X, Cui Y, Deng L, Shi Z, et al. Association Between Serum Bile Acid Profiles and Gestational Diabetes Mellitus: A Targeted Metabolomics Study. Clin Chim Acta (2016) 459:63–72. doi: 10.1016/j.cca.2016.05.026
14. Wang H, Yang W, Liu J, Leng J, Li W, Yu Z, et al. Serum Concentrations of SFAs and CDKAL1 Single-Nucleotide Polymorphism Rs7747752 are Related to an Increased Risk of Gestational Diabetes Mellitus. Am J Clin Nutr (2021) 114(5):1698–707. doi: 10.1093/ajcn/nqab225
15. Li J, Huo X, Cao YF, Li SN, Du Z, Shao P, et al. Bile Acid Metabolites in Early Pregnancy and Risk of Gestational Diabetes in Chinese Women: A Nested Case-Control Study. EBioMedicine (2018) 35:317–24. doi: 10.1016/j.ebiom.2018.08.015
16. Liu J, Li J, Li S, Leng J, Li W, Yang W, et al. Circulating Lysophosphatidylcholines in Early Pregnancy and Risk of Gestational Diabetes in Chinese Women. J Clin Endocrinol Metab (2020) 105(4):e982–e93. doi: 10.1210/clinem/dgaa058
17. Mo M, Shao B, Xin X, Luo W, Si S, Jiang W, et al. The Association of Gene Variants in the Vitamin D Metabolic Pathway and Its Interaction With Vitamin D on Gestational Diabetes Mellitus: A Prospective Cohort Study. Nutrients (2021) 13(12):4220. doi: 10.3390/nu13124220
18. Kirchhoff K, Machicao F, Haupt A, Schäfer SA, Tschritter O, Staiger H, et al. Polymorphisms in the TCF7L2, CDKAL1 and SLC30A8 Genes are Associated With Impaired Proinsulin Conversion. Diabetologia (2008) 51(4):597–601. doi: 10.1007/s00125-008-0926-y
19. Stancáková A, Pihlajamäki J, Kuusisto J, Stefan N, Fritsche A, Häring H, et al. Single-Nucleotide Polymorphism Rs7754840 of CDKAL1 is Associated With Impaired Insulin Secretion in Nondiabetic Offspring of Type 2 Diabetic Subjects and in a Large Sample of Men With Normal Glucose Tolerance. J Clin Endocrinol Metab (2008) 93(5):1924–30. doi: 10.1210/jc.2007-2218
20. Cauchi S, Proença C, Choquet H, Gaget S, De Graeve F, Marre M, et al. Analysis of Novel Risk Loci for Type 2 Diabetes in a General French Population: The D.E.S.I.R. Study. J Mol Med (Berl) (2008) 86(3):341–8. doi: 10.1007/s00109-007-0295-x
21. Biswas SK, Mohtarin S, Mudi SR, Anwar T, Banu LA, Alam SM, et al. Relationship of Soluble RAGE With Insulin Resistance and Beta Cell Function During Development of Type 2 Diabetes Mellitus. J Diabetes Res (2015) 2015:150325. doi: 10.1155/2015/150325
22. Okamura T, Yanobu-Takanashi R, Takeuchi F, Isono M, Akiyama K, Shimizu Y, et al. Deletion of CDKAL1 Affects High-Fat Diet-Induced Fat Accumulation and Glucose-Stimulated Insulin Secretion in Mice, Indicating Relevance to Diabetes. PloS One (2012) 7(11):e49055. doi: 10.1371/journal.pone.0049055
23. Xie C, Huang W, Young RL, Jones KL, Horowitz M, Rayner CK, et al. Role of Bile Acids in the Regulation of Food Intake, and Their Dysregulation in Metabolic Disease. Nutrients (2021) 13(4):1104. doi: 10.3390/nu13041104
24. Li T, Chiang JY. Bile Acids as Metabolic Regulators. Curr Opin Gastroenterol (2015) 31(2):159–65. doi: 10.1097/MOG.0000000000000156
25. Staels B, Fonseca VA. Bile Acids and Metabolic Regulation: Mechanisms and Clinical Responses to Bile Acid Sequestration. Diabetes Care (2009) 32(Suppl 2):S237–45. doi: 10.2337/dc09-S355
26. Leng J, Shao P, Zhang C, Tian H, Zhang F, Zhang S, et al. Prevalence of Gestational Diabetes Mellitus and its Risk Factors in Chinese Pregnant Women: A Prospective Population-Based Study in Tianjin, China. PloS One (2015) 10(3):e0121029. doi: 10.1371/journal.pone.0121029
27. Metzger BE, Gabbe SG, Persson B, Buchanan TA, Catalano PA, Damm P, et al. International Association of Diabetes and Pregnancy Study Groups Recommendations on the Diagnosis and Classification of Hyperglycemia in Pregnancy. Diabetes Care (2010) 33(3):676–82. doi: 10.2337/dc09-1848
28. Anderson CA, Pettersson FH, Clarke GM, Cardon LR, Morris AP, Zondervan KT. Data Quality Control in Genetic Case-Control Association Studies. Nat Protoc (2010) 5(9):1564–73. doi: 10.1038/nprot.2010.116
29. Andersson T, Alfredsson L, Kallberg H, Zdravkovic S, Ahlbom A. Calculating Measures of Biological Interaction. Eur J Epidemiol (2005) 20(7):575–9. doi: 10.1007/s10654-005-7835-x
30. Mackinnon DP, Warsi G, Dwyer JH. A Simulation Study of Mediated Effect Measures. Multivariate Behav Res (1995) 30(1):41. doi: 10.1207/s15327906mbr3001_3
31. Hou W, Meng X, Zhao A, Zhao W, Pan J, Tang J, et al. Development of Multimarker Diagnostic Models From Metabolomics Analysis for Gestational Diabetes Mellitus (GDM). Mol Cell Proteomics MCP (2018) 17(3):431–41. doi: 10.1074/mcp.RA117.000121
32. Dudzik D, Zorawski M, Skotnicki M, Zarzycki W, Kozlowska G, Bibik-Malinowska K, et al. Metabolic Fingerprint of Gestational Diabetes Mellitus. J Proteomics (2014) 103:57–71. doi: 10.1016/j.jprot.2014.03.025
33. Liang Z, Wang L, Liu H, Chen Y, Zhou T, Heianza Y, et al. Genetic Susceptibility, Lifestyle Intervention and Glycemic Changes Among Women With Prior Gestational Diabetes. Clin Nutr (Edinburgh Scotland) (2020) 39(7):2144–50. doi: 10.1016/j.clnu.2019.08.032
34. Kir S, Beddow SA, Samuel VT, Miller P, Previs SF, Suino-Powell K, et al. FGF19 as a Postprandial, Insulin-Independent Activator of Hepatic Protein and Glycogen Synthesis. Sci (New York NY) (2011) 331(6024):1621–4. doi: 10.1126/science.1198363
35. Trabelsi MS, Daoudi M, Prawitt J, Ducastel S, Touche V, Sayin SI, et al. Farnesoid X Receptor Inhibits Glucagon-Like Peptide-1 Production by Enteroendocrine L Cells. Nat Commun (2015) 6:7629. doi: 10.1038/ncomms8629
36. Drucker DJ. Mechanisms of Action and Therapeutic Application of Glucagon-Like Peptide-1. Cell Metab (2018) 27(4):740–56. doi: 10.1016/j.cmet.2018.03.001
37. Pascoe L, Tura A, Patel SK, Ibrahim IM, Ferrannini E, Zeggini E, et al. Common Variants of the Novel Type 2 Diabetes Genes CDKAL1 and HHEX/IDE are Associated With Decreased Pancreatic Beta-Cell Function. Diabetes (2007) 56(12):3101–4. doi: 10.2337/db07-0634
38. Liang J, Pei Y, Liu X, Qiu Q, Sun Y, Zhu Y, et al. The CDKAL1 Gene is Associated With Impaired Insulin Secretion and Glucose-Related Traits: The Cardiometabolic Risk in Chinese (CRC) Study. Clin Endocrinol (Oxf) (2015) 83(5):651–5. doi: 10.1111/cen.12838
39. Qi Y, Jiang C, Cheng J, Krausz KW, Li T, Ferrell JM, et al. Bile Acid Signaling in Lipid Metabolism: Metabolomic and Lipidomic Analysis of Lipid and Bile Acid Markers Linked to Anti-Obesity and Anti-Diabetes in Mice. Biochim Biophys Acta (2015) 1851(1):19–29. doi: 10.1016/j.bbalip.2014.04.008
40. Sun L, Pang Y, Wang X, Wu Q, Liu H, Liu B, et al. Ablation of Gut Microbiota Alleviates Obesity-Induced Hepatic Steatosis and Glucose Intolerance by Modulating Bile Acid Metabolism in Hamsters. Acta Pharm Sin B (2019) 9(4):702–10. doi: 10.1016/j.apsb.2019.02.004
41. Chen H, Ma J, Liu J, Dou L, Shen T, Zuo H, et al. Lysophosphatidylcholine Disrupts Cell Adhesion and Induces Anoikis in Hepatocytes. FEBS Lett (2022). doi: 10.1002/1873-3468.14291
Keywords: CDKAL1, rs7747752, glycoursodeoxycholic acid, deoxycholic acid, gestational diabetes mellitus
Citation: Wang H, Li J, Leng J, Li W, Liu J, Yan X, Yu Z, Hu G, Ma RCW, Fang Z, Wang Y and Yang X (2022) The CDKAL1 rs7747752-Bile Acids Interaction Increased Risk of Gestational Diabetes Mellitus: A Nested Case-Control Study. Front. Endocrinol. 13:808956. doi: 10.3389/fendo.2022.808956
Received: 04 November 2021; Accepted: 16 February 2022;
Published: 10 March 2022.
Edited by:
Ihtisham Bukhari, Fifth Affiliated Hospital of Zhengzhou University, ChinaReviewed by:
Dazhi Fan, Foshan Women and Children Hospital, ChinaFarooq Rashid, Southern Medical University, China
Copyright © 2022 Wang, Li, Leng, Li, Liu, Yan, Yu, Hu, Ma, Fang, Wang and Yang. This is an open-access article distributed under the terms of the Creative Commons Attribution License (CC BY). The use, distribution or reproduction in other forums is permitted, provided the original author(s) and the copyright owner(s) are credited and that the original publication in this journal is cited, in accordance with accepted academic practice. No use, distribution or reproduction is permitted which does not comply with these terms.
*Correspondence: Xilin Yang, yxl@hotmail.com; yangxilin@tmu.edu.cn; Ying Wang, wangying@gdmu.edu.cn; Zhongze Fang, fangzhongze@tmu.edu.cn
†These authors have contributed equally to this work