Modeling Tsunamis Generated by Submarine Landslides at Stromboli Volcano (Aeolian Islands, Italy): A Numerical Benchmark Study
- 1Istituto Nazionale di Geofisica e Vulcanologia, Sezione di Pisa, Pisa, Italy
- 2EDANYA Group, Universidad de Málaga, Málaga, Spain
We present a benchmark study aimed at identifying the most effective modeling approach for tsunami generation, propagation, and hazard in an active volcanic context, such as the island of Stromboli (Italy). We take as a reference scenario the 2002 landslide-generated tsunami event at Stromboli simulated to assess the relative sensitivity of numerical predictions to the landslide and the wave models, with our analysis limited to the submarine landslide case. Two numerical codes, at different levels of approximation, have been compared in this study: the NHWAVE three-dimensional non-hydrostatic model in sigma-coordinates and the Multilayer-HySEA model. In particular, different instances of Multilayer-HySEA with one or more vertical discretization layers, in hydrostatic and non-hydrostatic formulation and with different landslide models have been tested. Model results have been compared for the maximum runup along the shores of Stromboli village, and the waveform sampled at four proximal sites (two of them corresponding to the locations of the monitoring gauges, offshore the Sciara del Fuoco). Both rigid and deformable (granular) submarine landslide models, with volumes ranging from 7 to 25 million of cubic meters, have been used to trigger the water waves, with different physical descriptions of the mass movement. Close to the source, the maximum surface elevation and the resulting runup at the Stromboli village shores obtained with hydrostatic and non-hydrostatic models are similar. However, hydrostatic models overestimate (with respect to non-hydrostatic ones) the amplitude of the initial positive wave crest, whose height increases with the distance. Moreover, as expected, results indicate significant differences between the waveforms produced by the different models at proximal locations. The accurate modeling of near-field waveforms is particularly critical at Stromboli in the perspective of using the installed proximal sea-level gauges, together with numerical simulations, to characterize tsunami source in an early-warning system. We show that the use of non-hydrostatic models, coupled with a multilayer approach, allows a better description of the waveforms. However, the source description remains the most sensitive (and uncertain) aspect of the modeling. We finally show that non-hydrostatic models, such as Multilayer-HySEA, solved on accelerated GPU architectures, exhibit the optimal trade-off between accuracy and computational requirements, at least for the envisaged problem size and for what concerns the proximal wave field of tsunamis generated by volcano landslides. Their application and future developments are opening new avenues to tsunami early warning at Stromboli.
1. Introduction
The generation of large tsunamis is a relatively rare phenomenon at volcanic islands on a decadal scale (Latter, 1981; Béget, 2000; Tinti et al., 2003a), but it represents a remarkable risk, in reason of the catastrophic impact it may have along the nearby coasts (Auker et al., 2013; Paris et al., 2013; Paris, 2015). The most common phenomena capable to generate tsunami on volcanic islands are submarine and subaerial landslides (Harbitz et al., 2013; Løvholt et al., 2015; Yavari-Ramshe and Ataie-Ashtiani, 2016). Landslides are particularly frequent at active volcanoes during periods of intense eruptive activity, resulting in overloading and instability in both the submarine and subaerial portions of the volcano flanks (cf. Tibaldi, 2001; Pistolesi et al., 2020), especially on the parts of the edifice characterized by unconsolidated pyroclastic deposits and steep slopes (Bisson et al., 2007; Pistolesi et al., 2020). Rapid pyroclastic avalanches are a special type of subaerial mass flow composed of air and hot pyroclastic particles (ash, lapilli, and blocks produced during explosive eruptions). They are peculiar of volcanic settings and differ from other subaerial landslide by their generation mechanism, which can be associated with the collapse of eruptive jets and/or lava domes, or by the impulsive directional ejection of pyroclasts (Branney and Kokelaar, 2002). Moreover, they are characterized by an initially higher momentum, finer granulometry, and higher temperature, facilitating the built-up of pore pressure (Roche et al., 2011; Lube et al., 2020). For these features, the tsunamigenic capacity of pyroclastic avalanches is still only partially understood (De Lange et al., 2001; Freundt, 2003; Walder, 2003; Watts and Waythomas, 2003; Bougouin et al., 2020).
At Stromboli Island (Aeolian Islands, Southern Tyrrhenian Sea, Italy), the generation of tsunamis represents one among the several relevant hazards associated with ordinary and extraordinary volcanic activity (Rosi et al., 2013) for the shores of the island, for the nearby Aeolian Archipelago, and for the Southern Tyrrhenian Sea (Figure 1).
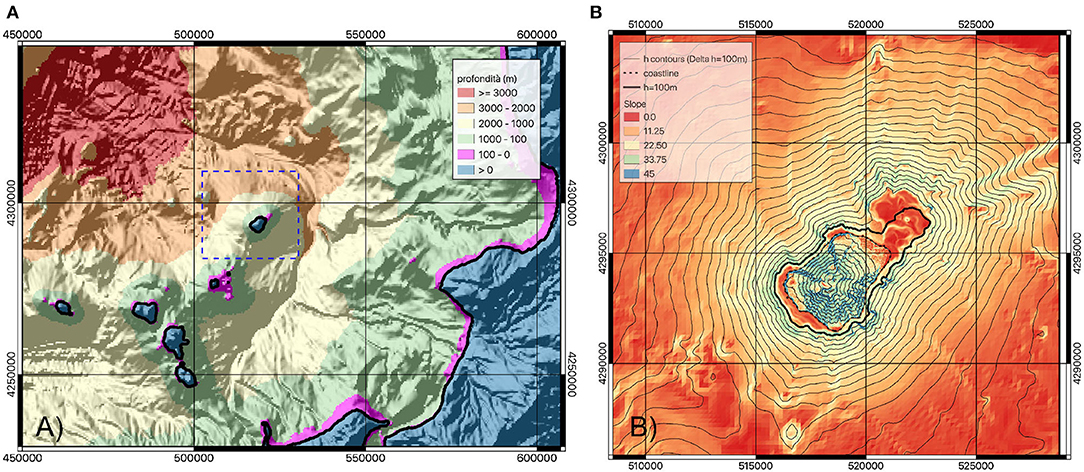
Figure 1. (A) Topo-bathymetry of the Southern Tyrrhenian sea, with the Aeolian archipelago and the island of Stromboli (dashed inset frame). (B) Map of proximal topographic and bathymetric slopes of the Stromboli volcano.
All known tsunami events at Stromboli were associated with intense explosive and/or effusive eruptions and subsequent landslides associated with gravitational instabilities of the Sciara del Fuoco (SdF) (Tinti et al., 2008; Casalbore et al., 2011; Pistolesi et al., 2020) (Figure 2). At least eight events of tsunami have been recognized since 1900 CE (Maramai et al., 2005b; Rosi et al., 2019; Pistolesi et al., 2020). The largest one was initiated on 30 December 2002 by two landslides (with total volume of the order of 10 × 106 m3 Chiocci et al., 2008) that detached from the submarine and subaerial flanks of the SdF scar (Bonaccorso et al., 2003; Maramai et al., 2005a; Tinti et al., 2006; Marani et al., 2008). The 2002 event is presently taken as a reference for emergency planning by the Italian Civil Protection. Two smaller but more recent events were associated with the July 3rd and August 28th, 2019, paroxysmal events (i.e., eruptions with exceedingly high mass eruption rate, with respect to the ordinary Strombolian activity; Rosi et al., 2013; Giordano and De Astis, 2020; Giudicepietro et al., 2020). Both events generated pyroclastic avalanches along the SdF, whose entrance into the sea triggered two sequences of tsunamis (INGV, 2019; LGS, 2019a,b). Although they did not have significant impact on the island shores (with maximum surface elevation of a few centimeters), they provided first-hand evidence of the capability of relatively small rapid pyroclastic avalanches to trigger water waves (Freundt, 2003; Watts and Waythomas, 2003; Bougouin et al., 2020). The analysis of the witnessed cases of the 20th century suggests in any case a dominant submarine component of the tsunami source mechanism at Stromboli (Maramai et al., 2005b; Rosi et al., 2013). Although probability of occurrence of submarine/subaerial landslides at SdF is not rigorously established yet, in this work we preliminary address submarine landslides and leave the study of subaerial landslides and pyroclastic avalanches for a future work.
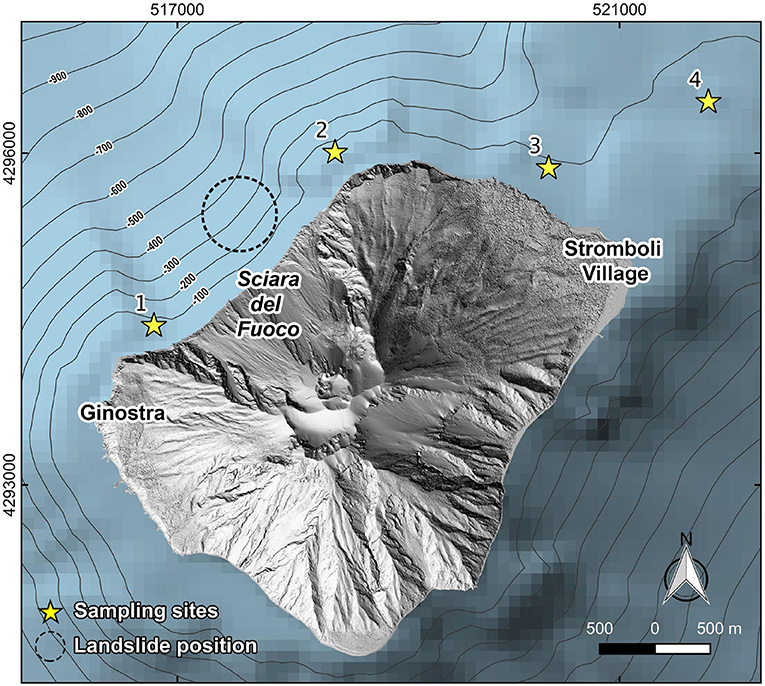
Figure 2. Topography and bathymetry of the island of Stromboli. Stars indicate the positions of the actual (1 and 2) and virtual gauges (3 and 4), where simulated waves are sampled. The initial position of the 2002 submarine landslide identified by Fornaciai et al. (2019) is indicated by the circle.
Modeling of tsunamis generated by submarine landslides entails different levels of complexity. Modeling of the tsunamigenic source requires description of the mechanisms of landslide triggering (Harbitz et al., 2006, 2013; Masson et al., 2006; Clare et al., 2018), propagation (Hungr et al., 2005; Pudasaini and Mergili, 2019), and deformation (Løvholt et al., 2015). These difficulties are common also for volcanic mass flows, whose capability to transfer energy to water waves, involving complex multiphase processes and dissipative phenomena, is still largely unknown (Tinti and Bortolucci, 2000; Ruff, 2003; Watts and Waythomas, 2003; Bougouin et al., 2020). For what concerns wave dynamics, volcanic tsunamis share some of the complexities that make the assumptions underlying the wave equations at the open sea fail: the source of the tsunami is almost always close to the shore, where non-linear shoaling effects are significant (cf. Guyenne and Grilli, 2003); the interaction with the coast and with a steep and rapidly varying bathymetry induces significant reflection and refraction effects (cf. Glimsdal et al., 2013); non-hydrostatic effects (i.e., frequency dispersion) are significant due to steep slopes and for the high-frequency component of generated waves on the shallow bathymetry (cf. Grilli and Watts, 2005). For these reasons, non-linear, non-hydrostatic wave dispersive models are recognized to be essential components to simulate landslide-generated tsunamis, including those at volcanic islands (Yavari-Ramshe and Ataie-Ashtiani, 2016).
At Stromboli, numerical modeling of tsunamis has been carried out and reported in a number of previous works, for the 2002 event (Tinti et al., 2006) and for potential scenarios generated outside the SdF area (Tinti et al., 2008), including considerations about extremely large volume landslides with tsunami (Tinti et al., 2000). These simulations have been carried out by means of a Lagrangian block model to compute the motion of the collapsing mass, and a finite-element, shallow water (hydrostatic) model to compute the propagation of the tsunami. The impact on Stromboli Island, on the Aeolian Archipelago and on the Southern Tyrrhenian Sea have been addressed (Tinti et al., 2003b) with a scenario approach based on the knowledge of past volcanic and tsunamigenic activity at Stromboli. However, the use of shallow-water models for landslide-generated tsunamis is nowadays known to suffer severe limitations, due to non-dispersive features and because of relevant three-dimensional effects associated with propagation along steep slopes (Yavari-Ramshe and Ataie-Ashtiani, 2016). For these reasons, Fornaciai et al. (2019) proposed a new numerical simulation work of the 2002 event at Stromboli, in which several landslide scenarios were studied by coupling a rigid landslide with a non-hydrostatic wave model to study the near-shore wave generation and propagation, including wave dispersion, shoaling and diffraction effects. To compute further wave propagation in the Southern Tyrrhenian Sea and the potential inundation, Fornaciai et al. (2019) have used a depth-averaged, dispersive Boussinesq wave model. That study allowed setting further constraints to the magnitude of either submarine and subaerial landslide phases for scenarios compatible with the 2002 event at Stromboli, and highlighted the importance of shoaling and diffraction phenomena which can increase the waves heights locally, with initial wave as large as 10 m and runups on the shores as high as 5–10 m. However, the high computational cost of the used three-dimensional solver (even when run on clusters of parallel processors) made its use for hazard assessment purposes problematic, limiting its use to single scenario analysis and to relatively small computational domains.
In the tsunami community there has been a continuous effort to identify criteria and appropriate validation experiments for the assessment of numerical model reliability. This was aimed especially to seismically induced tsunamis (Synolakis et al., 2007; Horrillo et al., 2015; Lynett et al., 2017), but the need of better understanding landslide-generated tsunamis recently stimulated a comparable effort. In this context, a set of experiments have been proposed as benchmarks for landslide-induced tsunami by Kirby et al. (2018). For conical islands, a specific benchmark based on laboratory experiments has been proposed by Romano et al. (2016), to be used for validation of numerical models (Montagna et al., 2011). Analysis of experimental data allowed Romano et al. (2013) and Bellotti and Romano (2017) to accurately characterize the physical properties of the wave generated by a subaerial, rigid landslide, the inundation mechanism (controlled by the trapped edge-wave) and the energy content of the radiating waves. However, it is still challenging to compare models against natural phenomena, due to the scarcity of the observations, the uncertainty on initial and boundary conditions and complex interactions between subsystems.
In this paper, we present a synthetic benchmark (or model inter-comparison) study aimed at quantifying the impact of different physical and numerical approximations on the resulting waveforms and tsunami inundation patterns at Stromboli, and identifying the most effective trade-off between computational cost and model accuracy. The Material and Methods section describes the landslide and wave models used for the benchmark and the simulation conditions. We take as a reference the 2002 scenario described by Fornaciai et al. (2019) and assess the relative sensitivity of numerical predictions to the landslide and the wave models. In the Results section we present the model results, emphasizing the comparison among proximal waveforms, sampled at the same locations of the two elastic gauges recently installed by Università di Firenze and the Italian Department of Civil Protection near the shoreline of the SdF (http://lgs.geo.unifi.it/; Lacanna and Ripepe, 2020). We also discuss differences in the inundation patterns at Stromboli village, by comparing the maximum wave height on the shoreline with the field data collected after the 2002 event, for different landslide volumes and models. In the context of the development of an early warning system, it is of primary importance to provide a reliable and effective (from the point of view of accuracy and computational time) model able to interpret proximal wave signals, and to potentially assimilate them into a predictive wave propagation model. Such aspects are addressed in the Discussion section, where we also discuss our results in the framework of the recent scientific literature. Finally, in the Conclusion section, we provide a short summary of the main results and an outline of future work.
2. Materials and Methods
The two models used in our study (named NHWAVE and Multilayer-HySEA) and shortly described below have been tested against validation laboratory experiments proposed by Kirby et al. (2018) during the Landslide Tsunami Model Benchmarking Workshop (LTMBW, 2017). Their formulation, implementation and validation are documented in the referenced literature. Both numerical codes include a wave generation mechanism describing the landslide and its interaction with the water (Table 1) and implement different approximations of the wave dynamics (Table 2). Their implementation is here described and summarized in Table 3.
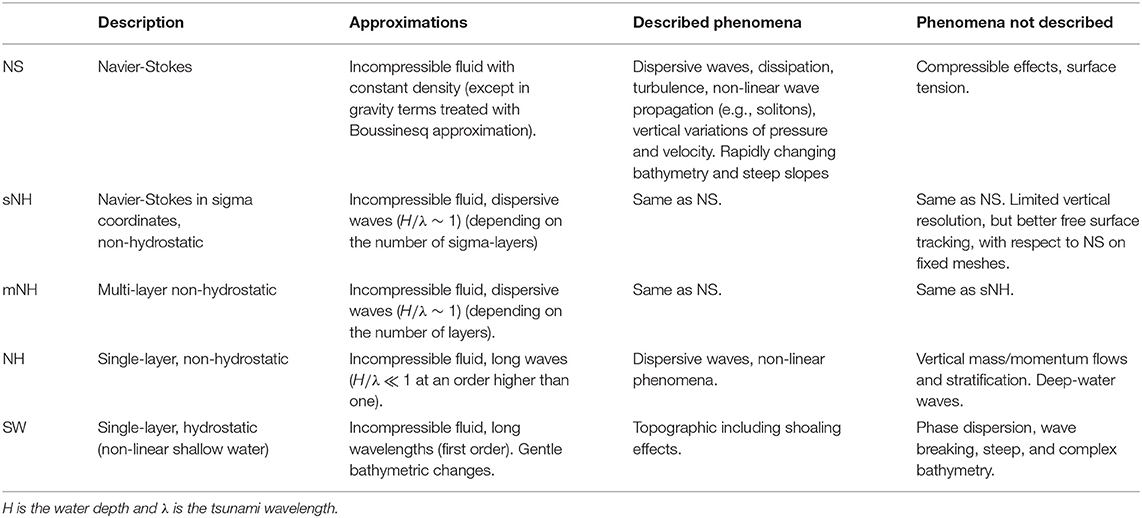
Table 2. Acronym and hierarchy of the different approaches to the modeling of water waves, ordered from top to bottom by decreasing complexity.
2.1. Rigid Landslide Model
In most of the presented numerical results, we adopt a simple conceptualization of the landslides, which considers a rigid sliding mass whose center of mass has prescribed kinematics. The slide has a nearly elliptical footprint on the slope and vertical cross sections varying according to truncated hyperbolic secant functions in the two orthogonal directions (the analytical expression is reported in the Supplementary Material), and it is identified by its length, width and maximum thickness, defining its volume (Enet and Grilli, 2007; Fornaciai et al., 2019). The Rigid Landslide model (abbreviated by the acronym RL in Tables 1, 2) considers the balanced effects of inertia, gravity, buoyancy, Coulomb friction, hydrodynamic friction, and drag forces. These are described by the equation of motion defined by Enet and Grilli (2007) and proposed by Kirby et al. (2018) at the Landslide Tsunami Model Benchmarking Workshop (LTMBW, 2017). They have been adopted by Fornaciai et al. (2019) for the study of the 2002 scenario at Stromboli:
where s is the spatial coordinate, θ is the slope angle, Cm is the added mass coefficient, is the landslide over water density ratio, Cd is the global drag coefficient, Cn is the basal Coulomb friction coefficient, g is the gravity acceleration, Ab and Vb are the landslide cross section and volume, calculated from the analytical expression reported in the Supplementary Material. Analytical integration of this equation on a constant slope and for large times gives the semi-empirical prognostic equation proposed by Grilli and Watts (2005) and Enet and Grilli (2007),
In this expression s0 and t0 are the characteristic distance and time, defined as , and , with ut (terminal velocity for large slides) and a0 (initial acceleration) defined as in Grilli and Watts (2005). On the 3D topography, we solve Equation (1) numerically by integrating the x and y components in time with a backward Euler scheme. With the RL model, coupling between landslide and water is essentially due to the transient modification of the bathymetry.
2.2. Granular Landslide Model
The Granular Landslide (GL) model (Savage and Hutter, 1989; Fernández-Nieto et al., 2008), describes the landslide as a deformable, incompressible granular medium with constant average density (i.e., constant porosity) which moves under the competing effect of gravity and frictional forces (Ma et al., 2013, 2015; Macías et al., 2015; Macías et al., 2020b; González-Vida et al., 2019). With the GL model, the landslide-wave coupling is two-way: the bottom landslide movement affects the water column by changing the bathymetry, and the two fluids (the landslide and the water) are coupled through friction terms. On the contrary, the fluctuation of pressure due to the variations of the free-surface can be neglected in the momentum equation of the granular material, thus simplifying the system of equations (Macías et al., 2020b). The comparison between the RL and GL model is carried out by imposing that the granular volume has the same initial shape of the rigid slide and using friction coefficients and density contrast within a comparable range. The rheology of the granular landslide is the most difficult part of the model to calibrate. In this work, we only analyze the results of a granular landslide with one of the two codes, Multilayer-HySEA. The model assumes a Coulomb rheology, with the friction coefficient depending on the landslide Froude number (Pouliquen and Forterre, 2002; Macías et al., 2020b). The friction law is thus characterized by three parameters (three friction angles) μ1, μ2, μ3. We set μ1 = μ3 and as a preliminary study we assessed the sensitivity of the results to variations of μ1 (static friction) and μ2 (reduced friction) in the ranges 0.02–0.18. The uncertainty of the resulting wave amplitude is always <10%. However, it is worth remarking that NHWAVE also includes the possibility of using a deformable granular model (Ma et al., 2013, 2015). Its most recent version (Zhang et al., 2021a,b) includes the possibility to simulate arbitrary bathymetry, viscous or granular slides, and also has non-hydrostatic pressure included in the slide layer.
2.3. Water Wave Models
In our study, we compare results of the two numerical solvers, run with the same initial and boundary conditions, to assess the influence of different physical and numerical approximations, for the specific natural case of Stromboli. Part of our analysis is dedicated to a comparison between hydrostatic and non-hydrostatic approximations. In the former, the condition of pressure being everywhere hydrostatic derives from the assumption of negligible vertical acceleration in the equation of vertical momentum. This is usually a good approximation for shallow-water (thin) flows (having horizontal wavelengths much larger than the flow thickness) and on mild slopes. It is nowadays recognized that non-linear, non-hydrostatic wave dispersive models are essential components to forecast landslide-generated tsunamis (Yavari-Ramshe and Ataie-Ashtiani, 2016), because their wavelengths are smaller and they are generated on steep slopes. However, legacy shallow-water models are still widely used by practitioners and researchers for assessing tsunami risk and impact (e.g., Liu et al., 2020). For this reason, we analyze the hydrostatic limit at Stromboli, in order to quantify the uncertainty associated with such an approximation.
NHWAVE is a 3D shock-capturing non-hydrostatic wave model developed by Ma et al. (2012), which solves the incompressible Navier-Stokes equations using a small number of vertical, boundary fitted, σ-layers. NHWAVE simulates wave generation by either rigid or deformable slides (Ma et al., 2012, 2013, 2015; Zhang et al., 2021a,b), including frequency dispersion effects (associated with vertical acceleration and non-hydrostatic pressure distribution). Terms describing the viscous and turbulent stress can be included in the NHWAVE model, but they were set to zero in the presented simulations. The code has been modified by Fornaciai et al. (2019) with respect to the original landslide treatment (imposing that the rigid body only follows straight trajectories on a constant slope and therefore with a fixed kinematics) in order to solve the landslide motion equation along the bathymetry. NHWAVE has been widely applied to simulate the wave generation stage of some real landslide-generated tsunami events with a RL model (Tappin et al., 2014; Grilli et al., 2015; Fornaciai et al., 2019). More recently, NHWAVE has been used to simulate the 2018 collapse of Anak Krakatau volcano and subsequent tsunami (Grilli et al., 2019), the 1908 Messina (Schambach et al., 2020), and the 2018 Palu (Schambach et al., 2021) tsunamis with a GL model. The NHWAVE model is parallelized using Message Passing Interface (MPI) with non-blocking communication, with domain-decomposition using ghost-cells.
The Multilayer-HySEA model implements one of the multilayer, non-hydrostatic models of the family introduced and described in Fernández-Nieto et al. (2018), in which the three-dimensional model equations are depth-averaged across a number of vertical layers. The governing equations, obtained by a process of depth-averaging, correspond to a semi-discretization for the vertical variable of the Euler equations and are mathematically equivalent to those of the NHWAVE model. The total pressure is decomposed into a sum of hydrostatic and non-hydrostatic pressures. In this process, the horizontal and vertical velocities are assumed to have a constant vertical profile. The proposed model admits an exact energy balance and, when the number of layers increases, the linear dispersion relation of the linear model converges to the same of Airy's theory (Fernández-Nieto et al., 2018). The motion of the bottom surface can be taken into account as a boundary condition. Therefore, this model can simulate the interaction with a slide in the case that the motion of the bottom is prescribed by a function, given by a set of data, or simulated by a numerical model. In the latter case, the bottom layer can represent the motion of either a rigid (RL) or granular (GL) landslide. The new version of Multilayer-HySEA incorporates the possibility of simulating the generation of tsunami produced by subaerial or submarine deformable landslides. The GL motion is modeled by a shallow-water Savage-Hutter type (Fernández-Nieto et al., 2008) model that is weakly coupled with the non-hydrostatic multilayer model through the boundary conditions (i.e., the modification of the pressure term associated with the wave height is neglected). Model description and validation tests are detailed in Macías et al. (2020a,b). The Multilayer-HySEA model can also be run in hydrostatic approximation (in which case, the multilayer formulation is equivalent to the non-linear shallow-Water equations). The Multilayer-HySEA numerical code is designed to run on Graphic Processing Unit (GPU) accelerated High-Performance Computing (HPC) architectures (Escalante et al., 2018, 2019).
Table 2 presents a list of the modeling approaches considered in this study, defining a hierarchy based on the complexity of the underlying physical model and highlighting the approximations and limitations. Table 3 shows the numerical codes tested in this study and the different computational approaches implemented.
2.4. Computational Efficiency
The evaluation of the computational efficiency in the numerical simulation of a tsunami is a fundamental component, together with the accuracy of the approximations and of the numerical solution algorithm, for the choice of the strategy and the numerical model for early warning. Evaluating the suitability of a numerical application requires the identification of specific metrics to compare different models, considering three main factors: (1) accuracy of the model in the description of the phenomenon (physical problem); (2) accuracy of numerical approximations (mathematical problem); (3) efficiency of algorithms (implementation problem). It is indeed always possible to obtain extremely fast computational models by sacrificing the accuracy of the physical representation and/or by using coarser numerical methods, both in terms of spatial/temporal resolution and in terms of mathematical accuracy. In the present case, we make the choice of comparing execution times of the different solvers with the same physics, and on the same physical problem representing a real case. It is worth remarking, however, that such a comparison might be incomplete, because the models might have a different convergent rate to the solution (at decreasing grid size). To check this, a comparison with an analytical test solution should be done, which is left for a future study.
In Table 4 we compare the execution time of the models described above for the simulation of the tsunami generation and propagation in the proximal domain around the Stromboli island. As expected, the hydrostatic models, with the same resolution, are much faster (by a factor of almost 10), since they can exploit efficient computational techniques for hyperbolic systems and do not require the solution of the more complex Poisson equation for the pressure. As for multilayer models, a linear dependence is observed between the number of layers and the execution time. Although MPI-based parallelization has the potential advantage of being more scalable for larger, memory-intensive applications, intrinsic speed-up limits are always associated with the overhead of the MPI, especially for relatively small-size problems. On the other hand, while GPUs are known to perform extremely fast for HPC problems, this is achieved at the price of a more complex programming paradigm and less flexibility in terms of memory usage. For the type and size of problem addressed in this work, resolution on GPU-based accelerated architectures is significantly more efficient. For this reason, we base most of the model sensitivity analysis on Multilayer-HySEA on GPUs.
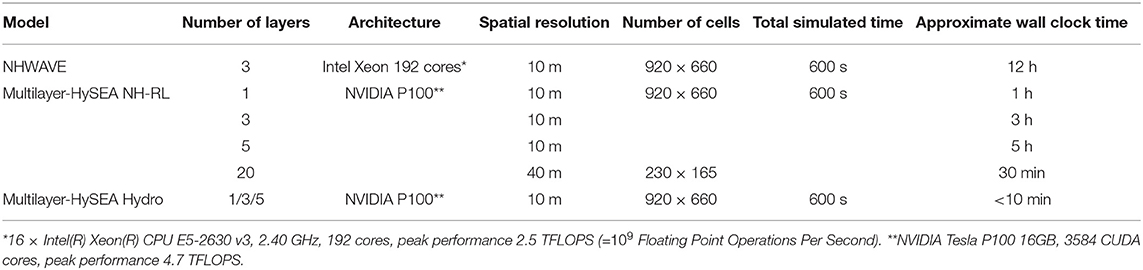
Table 4. Computational effort for the benchmark test using different numerical models and grid resolutions.
2.5. Simulated Scenarios
We compare the simulation results with different wave and landslide models. Although a complete study on landslide modeling and parameterization is beyond the scope of the present work, we emphasize the importance of the source model in tsunami predictions, and we present a comparison of the influence of the landslide model on the resulting tsunami. The benchmark has been designed to ensure consistency with previous studies by Fornaciai et al. (2019). It relates to the initiation and propagation of the tsunami due to a submarine landslide, described as a rigid body of fixed volume propagating along the trajectory of maximum bathymetric slope, with a kinematic law prescribed by Equation (1). It is worth remarking that, although the volume of the rigid landslide remains the same during propagation, its shape may locally slightly vary because of the bathymetry changes on its bottom. As part of the work, the RL model was implemented in the Multilayer-HySEA code to ensure compatibility with the scenarios simulated by Fornaciai et al. (2019), in which the reference scenario for Stromboli was produced using the NHWAVE (3 layers) model. The geometric and physical parameters used to characterize the landslide kinematics are reported in Table 5. More details can be found in Fornaciai et al. (2019) and in the Supplementary Material. Please notice that the volumes reported in Table 5 are those actually implemented in the simulation model. The values computed with the analytical formula proposed by Enet and Grilli (2007) in Fornaciai et al. (2019) (who reported 6, 10, and 15 × 106 m3), were underestimated by about 15%. It is worth remarking that Enet and Grilli (2007)'s analytical formula has been recently corrected by Schambach et al. (2019).
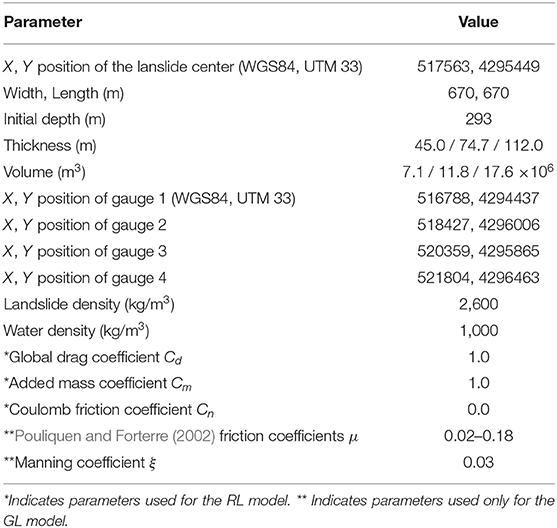
Table 5. Geometric and physical parameters characterizing the three scenarios of a submarine landslide studied by Fornaciai et al. (2019).
Most of the numerical benchmark tests have been performed on a 10 m resolution mesh on a 9.2 × 6.6 km2 domain, but the effect of the numerical resolution has also been tested. In particular, the error in maximum wave height moving from a horizontal resolution of 20–10 m was <5% in all simulated cases, whereas a grid of 40 m can lead to underestimate the wave height by about 30%. In Table 4, a list of tested resolutions is reported.
For each scenario, and for each model, the following outputs were compared: (1) the sampled waveforms at four different positions, corresponding to the two gauges installed in Stromboli offshore of Punta dei Corvi (gauge 1) and Punta Labronzo (gauge 2) and to two virtual gauges located one in front of Porto dei Balordi (gauge 3) and one near the Strombolicchio reef (gauge 4); (2) the runup, i.e., maximum height reached by the wave in the stretch that goes from Spiaggia Lunga to Porto; (3) the code execution time. The positions of the sampling points are indicated in Figure 2 and reported in Table 5.
3. Results
3.1. Waveforms
Because we take as a reference for our benchmark the scenarios discussed and simulations performed by Fornaciai et al. (2019) with the rigid landslide model, we first analyse the sensitivity of the numerical results to the wave model with the same rigid landslide source. Figure 3 reports the simulated waves at the Punta dei Corvi proximal gauge (gauge 1), for the three simulated volumes of the rigid submarine landslide (7.1, 11.8, and 17.6 × 106 m3). We first observe that the waveform amplitude is directly correlated with the landslide volume, whereas the frequency content is almost independent, showing almost identical sequences of local maxima and minima in the three scenarios. Independency of the wave form on the landslide volume is also observable at the more distal gauge (gauge 4 at Strombolicchio; Figure 4). Waveforms at gauges 2 and 3 are shown in the Supplementary Material.
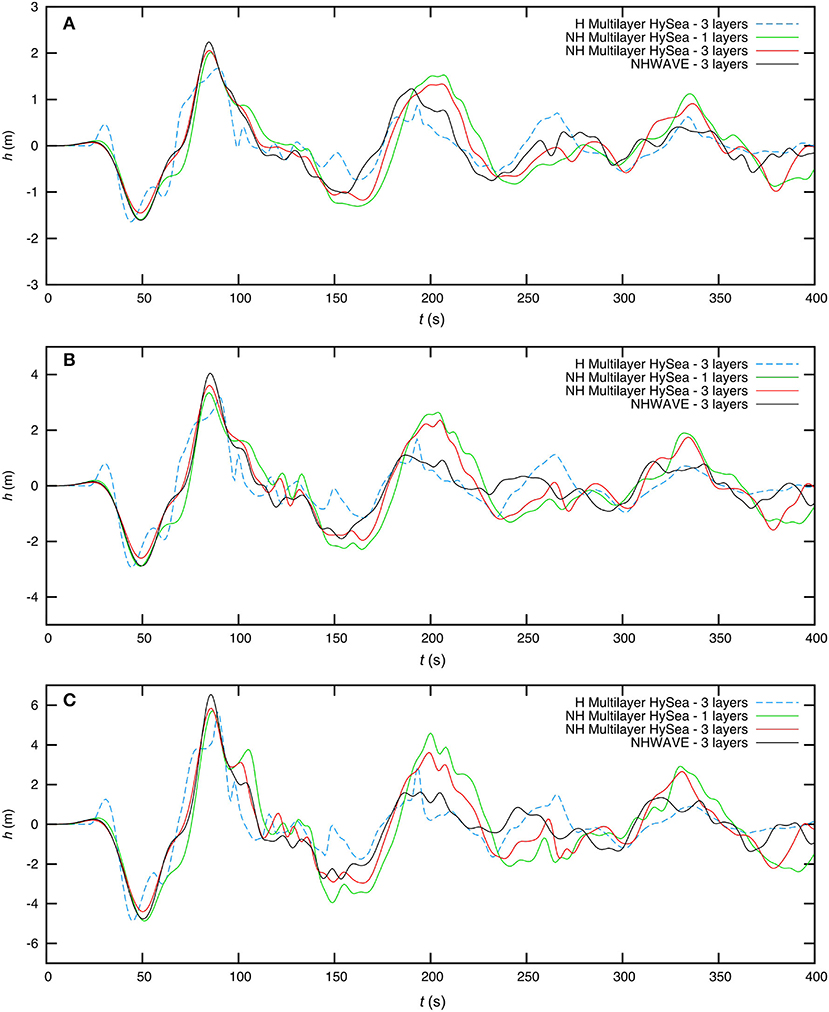
Figure 3. Wave forms at gauge 1 simulated with different wave models with a rigid landslide (A) V = 7.1 × 106 m3, (B) V = 11.8 × 106 m3, and (C) V = 17.6 × 106 m3.
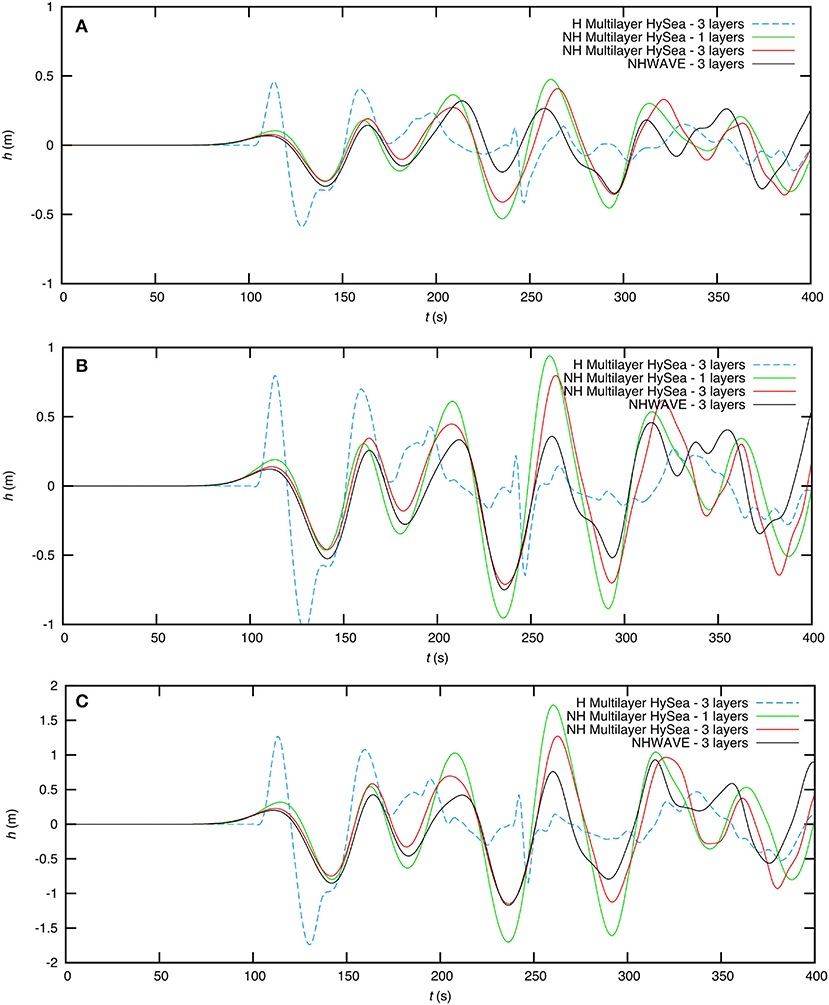
Figure 4. Wave forms at gauge 4 simulated with different wave models with a rigid landslide (A) V = 7.1 × 106 m3, (B) V = 11.8 × 106 m3, and (C) V = 17.6 × 106 m3.
3.1.1. Effect of the Algorithm and Implementation: NHWAVE vs. Multilayer-HySEA With 3 Layers
As described above, NHWAVE and Multilayer-HySEA models are equivalent from the point of view of the physical formulation (both for the wave and for the source). In particular, the viscous and turbulent viscosity terms (not present in Multilayer-HySEA) are set to zero in this application of NHWAVE. The numerical approximations can also be demonstrated to be mathematically equivalent (cf. Fernández-Nieto et al., 2018; Escalante et al., 2019). Differences between the results are therefore attributable mostly to the different numerical implementations, the accuracy of the discretization and the resolution algorithm.
Continuous black and red lines in Figures 3, 4 compare the results obtained, at a resolution of 10 m, with both models using 3 layers (as in Fornaciai et al., 2019), sampled at gauges 1 and 4. The waveforms obtained with the NHWAVE 3-layers and Multilayer-HySEA 3-layers models are very similar both in amplitude and over time, at least as regards the first peaks and the absolute maximum/minimum, for the three simulated triggering volumes. The same is observed for the two other sampling points shown in the Supplementary Material. This result was expected since the two models are mathematically equivalent. Differences observable in the rest of the wavetrain are possibly associated with reflections and the combined effect of the threshold of minimum depth for wet/dry condition, which was 1 m for NHWAVE and 0.01 m for Multilayer-HySEA. This is especially true at gauge 1, close to the shoreline. At gauge 4, wave oscillations are less influenced by near-shore effects but are influenced by diffraction by the corner of the island (at Punta Labronzo, near gauge 2—Figure 2).
3.1.2. Vertical Resolution Effects: Non-hydrostatic Multilayer-HySEA 1 vs. 3 Layers, With a Rigid Landslide
The comparison between the results of the Multilayer-HySEA non-hydrostatic model with different numbers of layers is aimed at evaluating the physical approximations (in particular, that of “long waves”) in the presence of steep bathymetric slopes where three-dimensional effects and dispersive terms should be relevant. Indeed, adding more layers usually allow to relax the shallow-water approximation (Macías et al., 2020a). Figures 3, 4 show the comparisons obtained with the high-resolution models (10 m). Simulations with 20 layers (computationally more demanding) are carried out only at low resolution (40 m) to ascertain the numerical convergence of the models to almost indistinguishable waveforms for N > 3 (cf. the Supplementary Material). Changing between 1 and 3 layers, differences in non-hydrostatic model results are relatively small for the first positive and negative peaks, but slightly increase for the subsequent oscillations in the proximal and more distal regions (for t > 300 s).
All non-hydrostatic models display a growing water crest above the submarine landslide, which moves at the same velocity and along the same trajectory of the landslide, without propagating in other directions. This effect is associated with the deepening of the bathymetry along the landslide trajectory, which makes the long-wave approximation weaker. It is due to the approximate dispersion laws in the dispersive, non-hydrostatic model, occurring for short wavelengths (H/λ > 1) (Escalante et al., 2019; Macías et al., 2020a). The phenomenon is greatly reduced by the use of a higher number of layers and, in this case and for the simulated domain, it almost disappears for N > 5.
3.1.3. Dispersive Effects: Multilayer-HySEA 3 Layers, Non-hydrostatic vs. Hydrostatic
The comparison between Multilayer-HySEA non-hydrostatic vs. hydrostatic, both with three vertical layers, is aimed at assessing the suitability of the hydrostatic model (which is much more efficient from a computational point of view and attractive in the perspective of early-warning applications) for the simulation of near fields and waveforms. It should be noted that simulations with the 1, 3, or 5 layers hydrostatic model produce identical results, as regards to both the inundation height and waveforms. As expected, the waveforms generated by the hydrostatic models are significantly different from those obtained with the non-hydrostatic models. In particular, a significant increase of the first relative maximum of the leading crest is observed for the hydrostatic models (at about 40 s at gauge 1; Figure 3). This maximum is progressively amplified and becomes the absolute maximum at the most distal sampling points (Figure 4). Moreover, a general divergence of the waveforms is observed for longer times, as associated with the different phase velocity with respect to non-hydrostatic models.
To quantify the effect of the hydrostatic/non-hydrostatic approximation on the proximal (near-shore) waveforms (where monitoring gauges are installed), we have extracted the amplitude of wave minima and maxima and their time of arrival after the triggering of the landslide. In this analysis, we have not considered the positive local maximum of the first crest, since we have already noticed that hydrostatic models have the tendency to largely increase its amplitude. Moreover, to avoid considering all the local amplitude fluctuations, we have set the minimum amplitude fluctuation to 0.66 m (0.55 m for hydrostatic models), which is approximately equal to the amplitude of the last maximum. Table 6 reports the values of the first negative minimum and first positive maximum, and the amplitude and half period of the first, second, and third waves oscillations. Inspection of the results suggests that, beyond increasing the amplitude of the leading positive crest, the hydrostatic model overestimates the period of the first and largest oscillation, and it significantly decreases the amplitude of the second and third ones at the proximal locations.
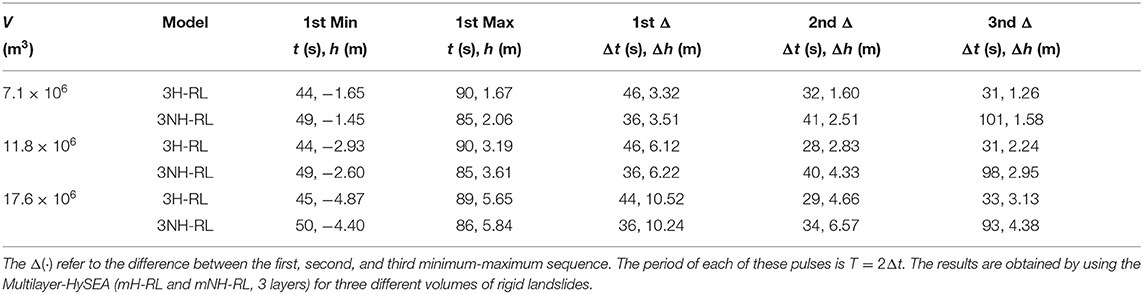
Table 6. Wave minima and maxima with respect to the average sea level at gauge 1, characterized by the time after landslide release (t) and surface elevation (h).
3.1.4. Source Effects: Multilayer-HySEA Hydrostatic/Non-hydrostatic With Rigid or Granular Landslide
Figure 5 shows the comparison between waveforms obtained with either a RL or a GL model coupled with either the hydrostatic and non-hydrostatic Multilayer-HySEA wave model, at gauge 1, close to the landslide source. The initial geometric conditions (the volume and shape of the sliding mass, initially at rest) and vertical discretization (3 layers) are the same for the two models, and the friction and density contrasts are set within a comparable range: the differences between the simulated waveforms are only due to the different slide dynamics. For the RL model, the kinematics is prescribed by Equation (1) whereas the GL model computes the motion of a deformable granular fluid with Coulomb rheology.
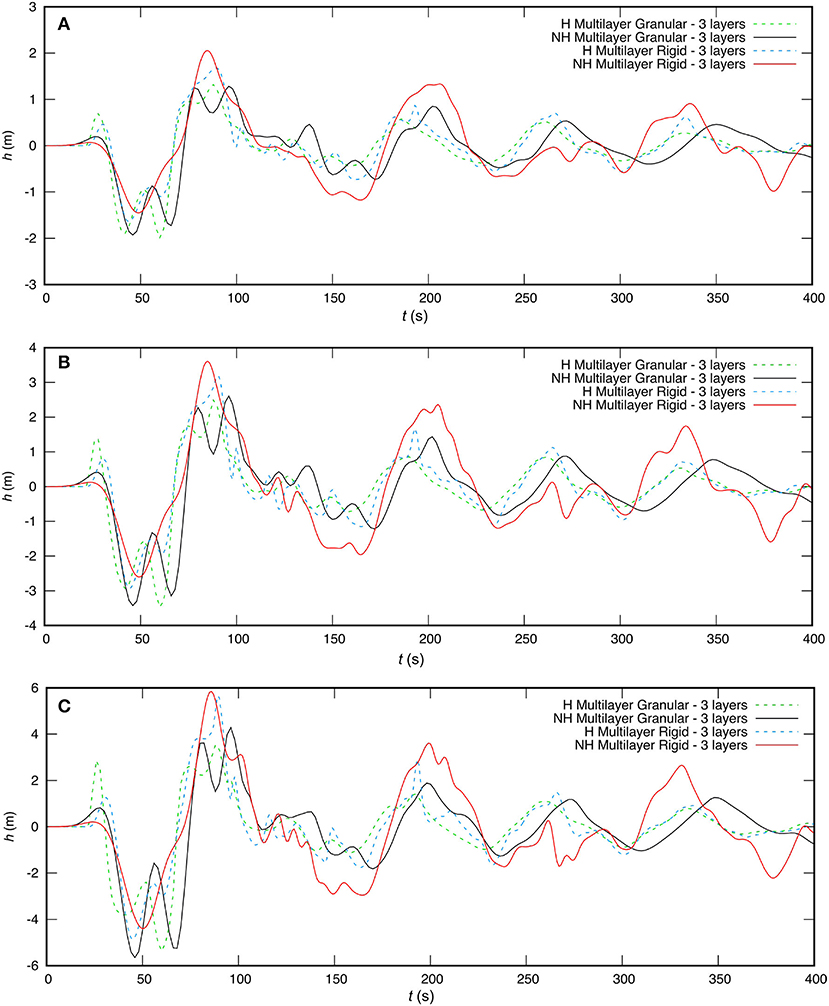
Figure 5. Wave forms at gauge 1 simulated with a hydrostatic and non-hydrostatic Multilayer-HySEA model with either a rigid or a granular landslide, and three vertical layers. The three subplots are for different landslide volumes: (A) V = 7.1 × 106 m3, (B) V = 11.8 × 106 m3, and (C) V = 17.6 × 106 m3.
The difference associated with the landslide (rigid or granular) source model is comparable to (but somehow larger than) that between the hydrostatic and non-hydrostatic results. At the most proximal gauge (Figure 5), the outcoming wave features a small leading crest followed by an intense depression, typical of submarine landslides. As expected, the leading crest is amplified by the hydrostatic model, more pronouncedly for the rigid landslide case. For the granular model, the first wave depression is deeper, but it is followed by a lower positive peak, resulting in a comparable wave height during the first oscillation. The main wave period appears to be comparable between the two models. As already discussed for Figure 3, the hydrostatic approximation, in both cases, produces a strong amplification of the leading crest.
3.1.5. Vertical Resolution Effects: Non-hydrostatic Multilayer-HySEA 3, 5, 10 Layers, With a Granular Landslide
As for the RL model, the use of many layers N > 3 for the GL model does not significantly change the waveform and the wave height, although some variations in the amplitude of minima and maxima can be noticed. Table 7 reports the amplitude and time of the relative and absolute maxima and minima. To better quantify the influence of the vertical discretization on the wave features, in the Supplementary Material we show the waveforms obtained with Multilayer-HySEA with a granular landslide at gauge 1, with different vertical discretization from 3 to 10 layers. Amplitude of the first maximum can be up to 30% higher using 10 layers, but this is partly balanced by a slightly higher negative minimum. On the contrary, the time of the first maximum/minimum is almost identical in the three cases.
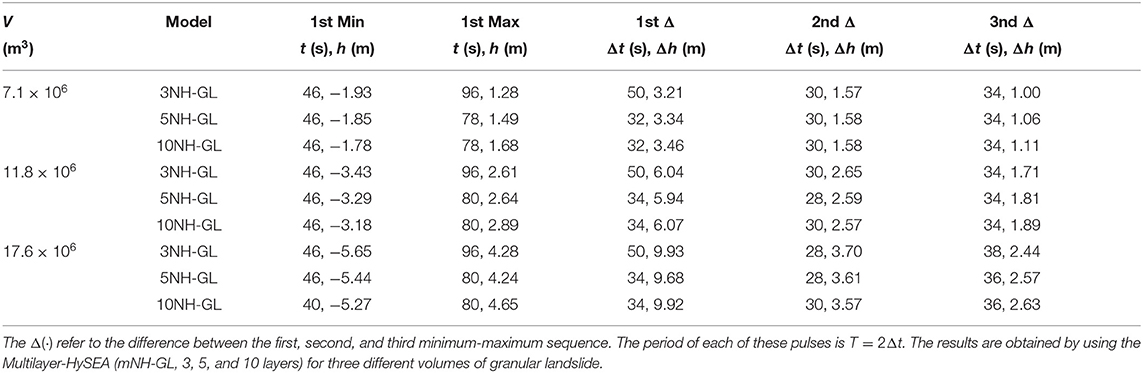
Table 7. Wave minima and maxima with respect to the average sea level at gauge 1, characterized by the time after landslide release (t) and surface elevation (h).
Figure 6 displays a rendering of the wave propagation and landslide position from 100 to 400 s, for the 17.6 × 106 m3 granular landslide. The comparison among the three different volumes can be seen in the animated results provided in the Supplementary Material.
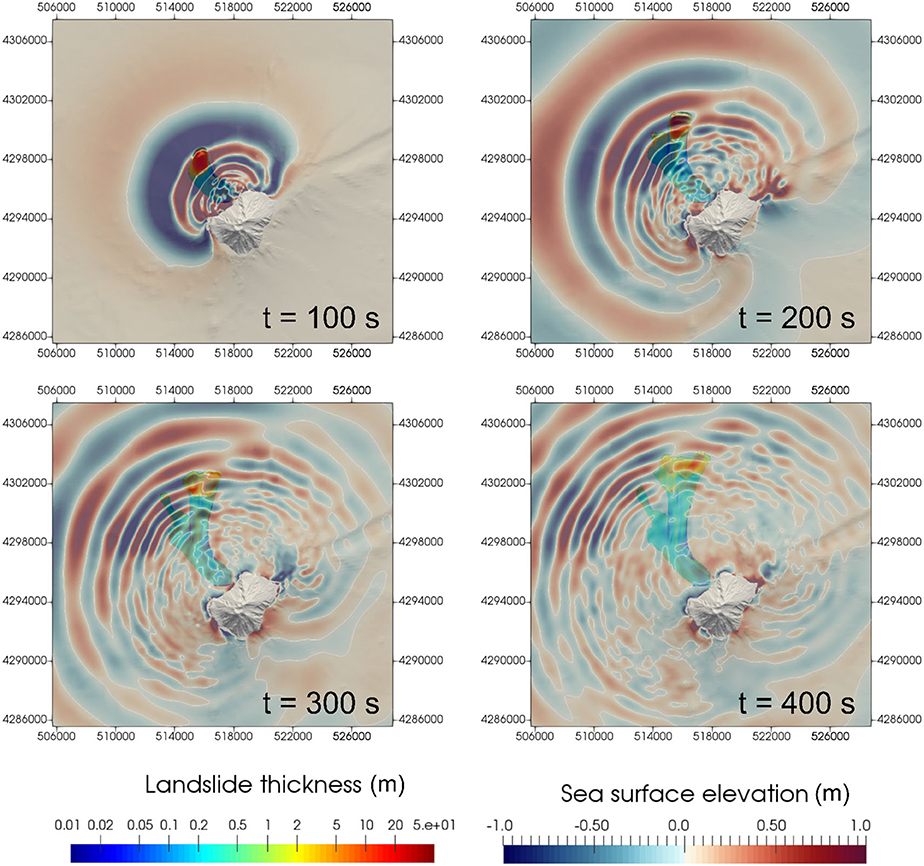
Figure 6. Sea surface elevation and landslide thickness simulated with the Multilayer-HySEA-GL model, with a landslide volume of 17.6 × 106 m3 and three vertical layers. At t = 400 s the landslide has reached its maximum runout and has almost completely stopped.
3.2. Maximum Surface Elevation and Potential Inundation
The simulation of the inundation process and the actual tsunami runup is very sensitive to the topo-bathymetric resolution, to sub-grid models describing turbulent processes at a scale smaller than the grid size (in our model, this is not considered), and to the minimum thickness threshold specified for the resolution of the wet/dry threshold. The use of a high threshold parameter (1 m thickness) was necessary in our study to ensure the convergence of the NHWAVE model on the complex topo-bathymetry of Stromboli, whereas the Multilayer-HySEA model converged with a thickness threshold of 0.01 m. Figure 7 reports the maximum tsunami runup (i.e., the maximum surface elevation along a transect perpendicular to the coastline) along the coastline, simulated with Multilayer-HySEA and NHWAVE with a rigid landslide, for the three analyzed scenarios. The comparison between the maximum surface elevation simulated with NHWAVE 3 layers and Multilayer-HySEA 3 layers shows a good consistency in their average values. However, these models present some local differences where Multilayer-HySEA 3 layers, with respect to NHWAVE, seems to predict lower values.
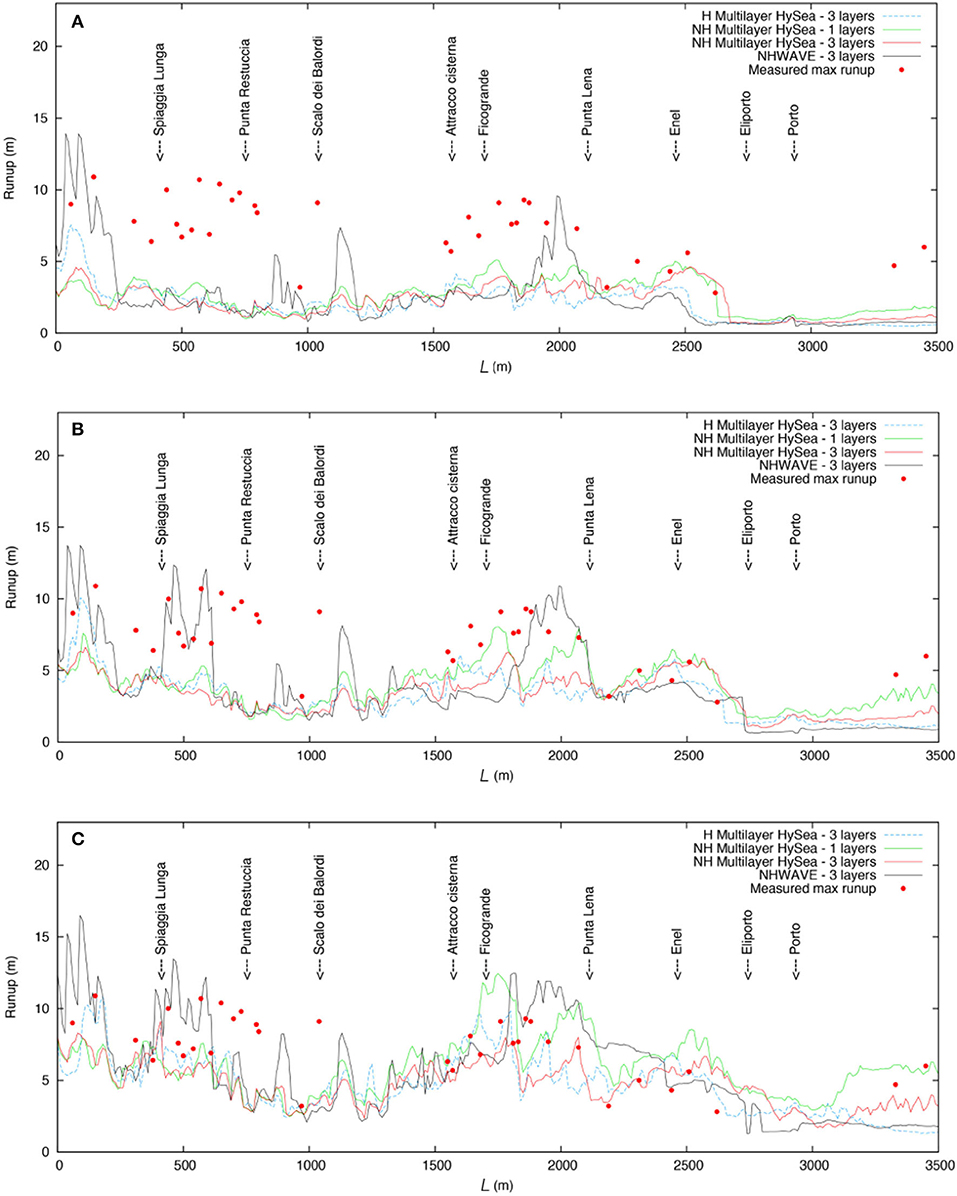
Figure 7. Tsunami maximum runup along the stretch of coastline represented by the white solid line in Figure 8, obtained with different wave models with a RL model (A) V = 7.1 × 106 m3, (B) V = 11.8 × 106 m3, and (C) V = 17.6 × 106 m3.
At a qualitative level, it is observed that in both cases the best agreement with the observations on the field data (Maramai et al., 2005a; Tinti et al., 2005) is obtained with a volume of 17.6 × 106 m3 (Fornaciai et al., 2019), consistent with the field estimates (Chiocci et al., 2008). In this region of the island, just behind Punta Labronzo headland, the wave height is very sensitive to the mechanism of reflection/diffraction, which in turn depends sensibly on the wet/dry threshold. Uncertainty of the results is therefore difficult to estimate. A detailed future study of the runups near the coast will clarify the role of this phenomenon in the runup estimates in Stromboli.
Results obtained with hydrostatic and non-hydrostatic formulations are comparable in amplitude and average value even if they are partially out of phase. The non-hydrostatic 1-layer model, compared to the 3-layer model in Figure 7, produces higher runups, probably due to inaccurate approximation of the phase velocity for short wavelengths onshore (Escalante et al., 2019; Macías et al., 2020a).
The GL model predicts smaller waves than the RL model, and a minor coastal inundation. To reproduce the inundation data reported by Fornaciai et al. (2019), a higher landslide volume of 25 × 106 m3 was necessary. Figure 8A presents a map of maximum surface elevation and arrival times for a granular landslide of 17.6 × 106 m3, to be compared with Figure 4 by Fornaciai et al. (2019). Figure 8B reports the maximum surface elevation at the coastline, together with the sampled runup at a number of sites (Maramai et al., 2005a; Tinti et al., 2005). Accordingly to this result, the best agreement with the observations is obtained with a volume of 25 × 106 m3 (Fornaciai et al., 2019), slightly larger but still consistent with the field estimates (Chiocci et al., 2008).
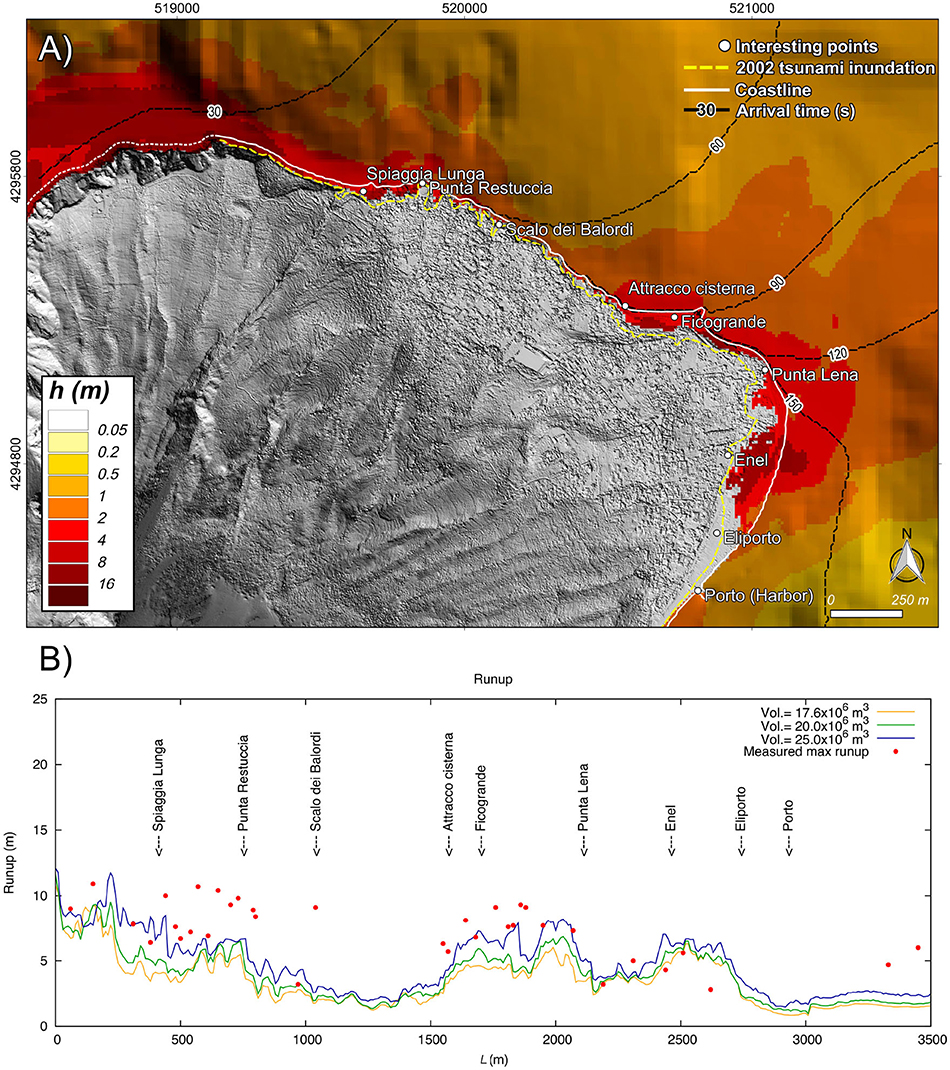
Figure 8. (A) Maximum surface elevation of the tsunami generated by a submarine granular landslide of volume V = 17.6 × 106 m3. (B) Maximum runup obtained with Multilayer-HySEA and granular landslide volumes of V = 17.6, 20.0, and 25.0 × 106 m3, with 3 layers. Red points represent the measurements reported by Tinti et al. (2006) of the tsunami runup for the 2002 event at Stromboli.
4. Discussion
The comparison study carried out in this work is aimed at identifying the most effective modeling strategy to simulate the waveforms generated by submarine landslides occurring at the SdF, a necessary preliminary step to calibrate a warning system based on proximal sea level measurements. In the case of a tsunamigenic event, this approach would be used to reconstruct the source of the detected waves, and to quickly forecast the subsequent impact on the Island of Stromboli, on the nearby Aeolian Archipelago and on the Southern Tyrrhenian Sea shores.
The observation of a correlation between wave height and landslide volume is consistent with some historical observations (Murty, 2003) and theoretical predictions of landslide-generated tsunamis. In particular, Ruff (2003) demonstrated that submarine landslides produce wave heights related to block height, and have wavelengths that scale with block width. By considering a block with uniform thickness moving on a horizontal seabed with constant velocity, Haugen et al. (2005) also showed that the length of the block affects only the wavelength, while the wave height is determined by the thickness of the block, the landslide velocity, and the wave speed (which depends on the water depth). The same dependency was found by Løvholt et al. (2005), for landslides characterized by slow propagation or occurring in sufficiently deep water (i.e., low Froude number; Harbitz et al., 2006). The new results indicate that such a correlation might hold also for deformable (granular) landslides, whose dynamics is governed by gravity, internal and bottom friction, and interaction with the water column. In particular, the wave height scales with the landslide volume (or initial thickness), as predicted by simpler theories, whereas the wavelength is almost independent of the volume. The influence of the initial submergence of the slide, which is a key parameter for the tsunami generation (Løvholt et al., 2005), has not been addressed in this work (the initial position was fixed following the indications given by Chiocci et al., 2008, for the 2002 event).
Differently from subaerial landslides, which produce a first large positive wave, submarine slides produce a first negative wave that propagates as an edge wave around the island causing water to first withdraw (Romano et al., 2016). The animations provided in the Supplementary Material clearly show, as expected, a first negative wave propagating around the island, followed by the arrival of positive waves. The analysis of the waveforms indicates that non-hydrostatic models produce coherent predictions among each other, and that the use of more than 3 layers does not significantly change the features of the proximal waves. The hydrostatic model predicts a first minimum and maximum of the wave at the proximal gauges (which is usually the first peak) consistently with the non-hydrostatic models, but on the contrary it delays the wave propagation, producing different waveforms at later times and lower amplitudes. In addition, the hydrostatic model predicts a larger leading crest, whose amplitude increases with the distance. Although, a priori, it is difficult to evaluate which solution is physically better in a comparison study, the tests carried out on the LTMBW (2017) model benchmark (Macías et al., 2020a) confirm that the form of landslide-generated waves cannot be accurately reproduced by a hydrostatic model (shallow water equations) or even with a one-layer non-hydrostatic model. Schambach et al. (2019) also compared the landslide tsunami simulations with and without dispersion (i.e., hydrostatic vs. non-hydrostatic results for both NHWAVE and FUNWAVE) in the near- ad far-field. For the very large slide volumes considered, they showed moderate dispersive effects in the near-field but very large differences caused by dispersion in the far-field. In our simulations, non-hydrostatic models can introduce spurious shoaling phenomena when the water depth changes in response to bathymetric variations, due to the increase of the relative error in the phase dispersion relations when H/λ increases. This phenomenon (which locally causes wave maxima) is however greatly reduced by using more than 5 layers, reducing the error to about 0.1% for kH up to 15 (k being the wavenumber), or H/λ < 2.4 (cf. Macías et al., 2020a) and it almost completely disappears with 10 layers.
The maximum surface elevation near the coastline is strongly affected by refraction and diffraction processes, and by shoaling effects (Ma et al., 2012), which justify the use of non-hydrostatic models able to account for a vertical component of the velocity (Zijlema and Stelling, 2008; Young and Wu, 2009). However, accurate modeling of near-shore dynamics might require the introduction of a turbulent stress term, to represent three-dimensional shear cascade and breaking of the fronts (Grilli and Watts, 2005). This will be the subject of future investigations.
The main comparisons in this work were made using a RL model. However, comparison of the results obtained with the RL and GL models shows that the two landslide models are not equivalent (although describing the same mobilized volume), and that the uncertainty associated with the trigger model is as relevant as that introduced by the water wave model (cf. Figure 5). The kinematic model for the rigid landslide was originally proposed by Watts (1998) not only for solid landslides but also for a deformable granular mass, with laboratory experiments (e.g., Grilli and Watts, 2005) suggesting that the center of mass motion in a deformable landslide moves in the same manner as a solid block. More recent results however report that RL models generally predict higher waves (Yavari-Ramshe and Ataie-Ashtiani, 2016; Schambach et al., 2019). Also in our simulations, for both the hydrostatic and non-hydrostatic cases the GL model shows a lower tsunamigenic potential. However, it is worth remarking that the amount of energy available to excite the wave obviously depends on the prescribed kinematics of the submarine rigid landslide. Analogously, for submarine granular landslides the rheological parameters (the angle of friction, the Manning coefficient; Table 5) would require prior calibration. Whereas, for subaerial granular flows several experimental (Fritz et al., 2004; Heller and Hager, 2011; Mohammed and Fritz, 2012; Heller and Spinneken, 2013; Bougouin et al., 2020) and numerical (Ruffini et al., 2019) works exist, systematic studies on subacqueous deformable granular landslides are less developed (Yavari-Ramshe and Ataie-Ashtiani, 2017). Grilli et al. (2017) presented and modeled lab experiments for underwater granular flows. They and Schambach et al. (2019) compared the generation of tsunamis by solid and granular slides of same initial geometry and compared also their center of mass motion. They showed that solid slides cause larger waves and runup. Although it is likely that the granular model provides a better representation of flow processes potentially generated by submarine landslides at Stromboli, it is still difficult to define a priori (in the absence of direct measures on the real phenomenon) which one is more realistic. For this reason, current line of research for future studies has been oriented toward developing validation studies for both the RL and GL models implemented in Multilayer-HySEA (Macías et al., 2020a,b).
Distal wave fields (>10 km) have not yet been addressed in this work. Extension of the domain to the whole Southern Tyrrhenian Sea would require nesting of different computational approaches, models and grid resolution to be effective (Fornaciai et al., 2019; Grilli et al., 2019). Simple scaling considerations suggest that the non-hydrostatic model would be necessary also to simulate the wave propagation stage of landslide-generated tsunamis from Stromboli. Indeed, it is generally considered (cf. Bowden, 1983) that the long-wave approximation leading to the shallow-water (hydrostatic) model can be applied to regimes of H/λ < 0.05, i.e., in the case of the Southern Tyrrenian sea (with depths as those of Figure 1), a wavelength larger than 50–100 km. As seen, e.g., in Figure 6, a landslide from the Sciara del Fuoco would generate tsunamis with shorter wavelengths, increasing up to about 6 km on the domain boundaries. Even considering the further development of long-wave components by the effects of bathymetric variations and frequency dispersion, most of the wave propagation in the Southern Tyrrhenian Sea would likely occur in an intermediate regime 0.05 < H/λ ~ 1. Fornaciai et al. (2019) estimated that waves generated by landslide at the SdF would be able to reach the first Stromboli populated beaches in just over 1 min and the harbor in <7 min. After about 30 min the whole Aeolian Arc would be impacted by maximum waves. After 1 h and 20 min, waves would encompass the whole Southern Tyrrhenian Sea arriving at Capri island. This leaves very little time for evacuating the coastal population, and makes it difficult to design an effective Tsunami Early Warning Systems (TEWS) (Selva et al., 2021).
Possible alternative approaches to the three-dimensional solution of the water equations and the granular landslide have been proposed by others, especially in the light of designing effective TEWS for landslide-generated tsunamis, also for volcanic islands. Ward (2001) has first proposed a linear solution for landslide-generated tsunami, based on the superposition of many small simple “square” slides for which a Green's function can be calculated analytically. This has been applied also to potential collapse of the Cumbre Vieja volcano (Ward and Day, 2001). A similar approach has recently been adopted by Wang et al. (2019) to reproduce the tsunami induced by the 1792 Unzen-Mayuyama mega-slide in Japan. Cecioni and Bellotti (2010) have proposed a near-field extension of the Bellotti et al. (2008)'s far-field propagation model based on the Mild Slope Equations, applied to tsunami propagation at Stromboli. Such an approach, based on the inclusion of a new wave source term representing the moving bottom boundary, has the advantage of giving an accurate solution in the far-field without needing many layers and to be accurate enough in the near-field. This is constrained by the approximate description of the source model and it has been tested only for a rigid landslide model. Another alternative approach is to use analytical models to describe the tsunami source, and using single layer wave models for the distal propagation (Liu et al., 2020). However, nowadays, numerical solvers exploiting GPUs allow to achieve unprecedented simulation speed-up and make it possible to approach three-dimensional (multilayer) simulations of tsunamis generated by a granular landslide at Stromboli, and their propagation across the Southern Tyrrhenian Sea. At the same time, three-dimensional simulations will potentially provide a way to interpret proximal waveforms registered by the two elastic gauges installed offshore the Sciara del Fuoco.
Data presented in Table 4 are relative to simulations performed at high resolution and on a relatively small domain of a few square kilometers encompassing the island of Stromboli. Despite execution times are still too large for early-warning (real-time) applications, recent developments of multi-GPU computing for the numerical tsunami models (Escalante et al., 2018) have allowed to significantly speed-up the code execution. These developments will likely make the faster-than-real-time simulation of volcano-landslide-tsunami generation and propagation possible in the future, opening new avenues to urgent tsunami computing, probabilistic tsunami hazard assessment and tsunami early warning (Løvholt et al., 2019; Macías and de la Asunción, 2019).
5. Conclusion
We have presented a synthetic benchmark (or model inter-comparison) study aimed at quantifying the impact of different physical and numerical approximations on the resulting waveforms and tsunami inundation patterns at Stromboli, and identifying the most effective trade-off between computational cost and model accuracy. We have taken as a reference the 2002 scenario described by Fornaciai et al. (2019) and assessed the relative sensitivity of numerical predictions to the landslide and the wave models. Present results and comparison with previously published data clearly indicate that dispersive non-hydrostatic models are better suited to reproduce proximal wave forms of landslide-generated tsunamis, and that using multiple layers (three being the optimal compromise in our tests) improves the quality of predictions. To extend numerical prediction to larger domains (characterized by deeper water), different strategies should be adopted. Further studies and technological developments are needed to address this point. In any case, it is apparent that the uncertainty of numerical model predictions associated with the landslide source model is as large as that of the wave model approximation: the comparison between a RL and GL model produces sensibly different results, in terms of wave amplitude, frequencies and attenuation. In the perspective of assimilating in a numerical model the measurement of sea level at the two gauges near the shore of the SdF, to trigger a Stromboli Tsunami Early Warning, it would therefore be important to adopt a coupled non-hydrostatic, multilayer wave model (implemented on GPU to reduce the time of simulation) with an accurate GL model. Future research should particularly focus on validation and calibration of the latter. The results of numerical simulations can be used as a preliminary calibration of the detection system currently operating at Stromboli Island, when direct measurements of waves generated by submarine landslides are not available.
During the course of this study, on July 3rd and August 28th 2019, two paroxysmal events (Giordano and De Astis, 2020; Giudicepietro et al., 2020) generated a sequence of pyroclastic avalanches along the SdF, which entered the sea. Water waves as high as 1 m near the entrance point were detected by the two gauges operated by Università di Firenze, and by a low-frequency mareometer off the coast of the Ginostra village. In a forthcoming work, we will report about the use of the results of the present study to analyze the waveforms produced by the entrance in the sea of pyroclastic avalanches.
Data Availability Statement
The raw data supporting the conclusions of this article will be made available by the authors, upon request without undue reservation.
Author Contributions
TE, MM, and AF have conceived the study and coordinated the project with the Italian Department of Civil Protection. MC and BC have contributed to the analysis of the physical approximations, simulation runs, and data analysis. MF, AF, and LN have run the NHWAVE model and provided comparison with previously published data. JM, MJC, SO, JG-V, and CE have developed and made available the HySEA software, their expertise in the interpretation of numerical model results and some computer time. All authors contributed to the article and approved the submitted version.
Funding
This work has been produced within the 2012–2021 agreement between Istituto Nazionale di Geofisica e Vulcanologia (INGV) and the Italian Presidenza del Consiglio dei Ministri, Dipartimento della Protezione Civile (DPC), Convenzione B2, Ob. 5 Task 4 (2017), Ob. 4 Task 4 (2018) and WP2 Task 12 (2019-2021). HySEA codes development is supported by the Spanish Government-FEDER funded project MEGAFLOW (RTI2018-096064-B-C21).
Conflict of Interest
The authors declare that the research was conducted in the absence of any commercial or financial relationships that could be construed as a potential conflict of interest.
Acknowledgments
We thank: S. Calvari and G. Macedonio (INGV) for the coordination of activities at INGV Centro di Pericolosità Vulcanica; A. Neri (Director of INGV Departments of Volcanoes) for supporting the project activities; D. Mangione and A. Ricciardi (Italian Department of Civil Protection) for discussion about implications for tsunami hazard and risk mitigation; M. Ripepe and G. Lacanna (University of Florence) for information about the gauges installation, the discussion about the Stromboli tsunami alert system and the requirements for its calibration. We thank the Editor JB and three referees for their meticulous reviews and further reading suggestions.
Supplementary Material
The Supplementary Material for this article can be found online at: https://www.frontiersin.org/articles/10.3389/feart.2021.628652/full#supplementary-material
The Supplementary material reports additional plots, videos, and data to support the paper's conclusions. In particular:
• the benchmark results at gauges 2 and 3 for a rigid landslide;
• the waveforms predicted by Multilayer-HySEA at gauges 1, 2, 3, and 4 for a rigid landslide and 1, 3, 5, and 10 layers;
• animated results of Multilayer-HySEA with a granular landslide, for different slide volumes.
References
Auker, M. R., Sparks, R. S. J., Siebert, L., Crosweller, H. S., and Ewert, J. (2013). A statistical analysis of the global historical volcanic fatalities record. J. Appl. Volcanol. 2, 57–24. doi: 10.1186/2191-5040-2-2
Béget, J. (2000). “Volcanic tsunamis,” in Encyclopedia of Volcanoes, eds H. Sigurdsson, B. Houghton, H. Rymer, J. Stix, and S. McNutt (London, UK: Academic Press), 1005–1014.
Bellotti, G., Cecioni, C., and De Girolamo, P. (2008). Simulation of small-amplitude frequency-dispersive transient waves by means of the mild-slope equation. Coast. Eng. 55, 447–458. doi: 10.1016/j.coastaleng.2007.12.006
Bellotti, G., and Romano, A. (2017). Wavenumber-frequency analysis of landslide-generated tsunamis at a conical island. Part II: EOF and modal analysis. Coast. Eng. 128, 84–91. doi: 10.1016/j.coastaleng.2017.07.008
Bisson, M., Pareschi, M. T., Zanchetta, G., Sulpizio, R., and Santacroce, R. (2007). Volcaniclastic debris-flow occurrences in the Campania region (Southern Italy) and their relation to Holocene–Late Pleistocene pyroclastic fall deposits: implications for large-scale hazard mapping. Bull. Volcanol. 70, 157–167. doi: 10.1007/s00445-007-0127-4
Bonaccorso, A., Calvari, S., Garfì, G., Lodato, L., and Patanè, D. (2003). Dynamics of the December 2002 flank failure and tsunami at Stromboli volcano inferred by volcanological and geophysical observations. Geophys. Res. Lett. 30:1941. doi: 10.1029/2003GL017702
Bougouin, A., Paris, R., and Roche, O. (2020). Impact of fluidized granular flows into water: implications for tsunamis generated by pyroclastic flows. J. Geophys. Res. Sol. Earth 125, 319–317. doi: 10.1029/2019JB018954
Branney, M. J., and Kokelaar, B. P. (2002). Pyroclastic Density Currents and the Sedimentation of Ignimbrites. London, UK: Geological Society of London.
Casalbore, D., Romagnoli, C., Bosman, A., and Chiocci, F. L. (2011). Potential tsunamigenic landslides at Stromboli Volcano (Italy): insight from marine DEM analysis. Geomorphology 126, 42–50. doi: 10.1016/j.geomorph.2010.10.026
Cecioni, C., and Bellotti, G. (2010). Inclusion of landslide tsunamis generation into a depth integrated wave model. Nat. Hazards Earth Syst. Sci. 10, 2259–2268. doi: 10.5194/nhess-10-2259-2010
Chiocci, F. L., Romagnoli, C., Tommasi, P., and Bosman, A. (2008). The Stromboli 2002 tsunamigenic submarine slide: characteristics and possible failure mechanisms. J. Geophys. Res. 113, 173–111. doi: 10.1029/2007JB005172
Clare, M. A., Le Bas, T., Price, D. M., Hunt, J. E., Sear, D., Cartigny, M. J. B., et al. (2018). Complex and cascading triggering of submarine landslides and turbidity currents at volcanic islands revealed from integration of high-resolution onshore and offshore surveys. Front. Earth Sci. 6:223. doi: 10.3389/feart.2018.00223
De Lange, W. P., Prasetya, G. S., and Healy, T. R. (2001). Modelling of tsunamis generated by pyroclastic flows (ignimbrites). Nat. Hazards 24, 251–266. doi: 10.1023/A:1012056920155
Enet, F., and Grilli, S. T. (2007). Experimental study of tsunami generation by three-dimensional rigid underwater landslides. J. Waterway Port Coast. Ocean Eng. 133, 442–454. doi: 10.1061/(ASCE)0733-950X(2007)133:6(442)
Escalante, C., de Luna, T. M., and Castro, M. J. (2018). Non-hydrostatic pressure shallow flows: GPU implementation using finite volume and finite difference scheme. Appl. Math. Comput. 338, 631–659. doi: 10.1016/j.amc.2018.06.035
Escalante, C., Fernández-Nieto, E. D., de Luna, T. M., and Castro, M. J. (2019). An efficient two-layer non-hydrostatic approach for dispersive water waves. J. Sci. Comput. 79, 273–320. doi: 10.1007/s10915-018-0849-9
Fernández-Nieto, E. D., Bouchut, F., Bresch, D., Castro Díaz, M. J., and Mangeney, A. (2008). A new Savage–Hutter type model for submarine avalanches and generated tsunami. J. Comput. Phys. 227, 7720–7754. doi: 10.1016/j.jcp.2008.04.039
Fernández-Nieto, E. D., Parisot, M., Penel, Y., and Sainte-Marie, J. (2018). A hierarchy of dispersive layer-averaged approximations of Euler equations for free surface flows. Comm. Math. Sci. 16, 1169–1202. doi: 10.4310/CMS.2018.v16.n5.a1
Fornaciai, A., Favalli, M., and Nannipieri, L. (2019). Numerical simulation of the tsunamis generated by the Sciara del Fuoco landslides (Stromboli Island, Italy). Sci. Rep. 9:18542. doi: 10.1038/s41598-019-54949-7
Freundt, A. (2003). Entrance of hot pyroclastic flows into the sea: experimental observations. Bull. Volcanol. 65, 144–164. doi: 10.1007/s00445-002-0250-1
Fritz, H. M., Hager, W. H., and Minor, H. E. (2004). Near field characteristics of landslide generated impulse waves. J. Waterway Port Coast. Ocean Eng. 130, 287–302. doi: 10.1061/(ASCE)0733-950X(2004)130:6(287)
Giordano, G., and De Astis, G. (2020). The summer 2019 basaltic Vulcanian eruptions (paroxysms) of Stromboli. Bull. Volcanol. 83:1. doi: 10.1007/s00445-020-01423-2
Giudicepietro, F., pez, C. L. x., Macedonio, G., Alparone, S., Bianco, F., Calvari, S., et al. (2020). Geophysical precursors of the July-August 2019 paroxysmal eruptive phase and their implications for Stromboli volcano (Italy) monitoring. Sci. Rep. 10:10296. doi: 10.1038/s41598-020-67220-1
Glimsdal, S., Pedersen, G. K., Harbitz, C. B., and Løvholt, F. (2013). Dispersion of tsunamis: does it really matter? Nat. Hazards Earth Syst. Sci. 13, 1507–1526. doi: 10.5194/nhess-13-1507-2013
González-Vida, J. M., Macías, J., Castro, M. J., Sánchez-Linares, C., de la Asunción, M., Ortega-Acosta, S., et al. (2019). The Lituya Bay landslide-generated mega-tsunami–numerical simulation and sensitivity analysis. Nat. Hazards Earth Syst. Sci. 19, 369–388. doi: 10.5194/nhess-19-369-2019
Grilli, S. T., O'Reilly, C., Harris, J. C., Bakhsh, T. T., Tehranirad, B., Banihashemi, S., et al. (2015). Modeling of SMF tsunami hazard along the upper US East Coast: detailed impact around Ocean City, MD. Nat. Hazards 76, 705–746. doi: 10.1007/s11069-014-1522-8
Grilli, S. T., Shelby, M., Kimmoun, O., Dupont, G., Nicolsky, D., Ma, G., et al. (2017). Modeling coastal tsunami hazard from submarine mass failures: effect of slide rheology, experimental validation, and case studies off the US East Coast. Nat. Hazards 86, 353–391. doi: 10.1007/s11069-016-2692-3
Grilli, S. T., Tappin, D. R., Carey, S., Watt, S. F. L., Ward, S. N., Grilli, A. R., et al. (2019). Modelling of the tsunami from the December 22 2018 lateral collapse of Anak Krakatau volcano in the Sunda Straits, Indonesia. Sci. Rep. 9:11946. doi: 10.1038/s41598-019-48327-6
Grilli, S. T., and Watts, P. (2005). Tsunami generation by submarine mass failure. I: modeling, experimental validation, and sensitivity analyses. J. Waterway Port Coast. Ocean Eng. 131, 283–297. doi: 10.1061/(ASCE)0733-950X(2005)131:6(283)
Guyenne, P., and Grilli, S. T. (2003). Computations of three-dimensional overturning waves in shallow water: dynamics and kinematics, in Proceedings of The Thirteenth International Offshore and Polar Engineering Conference (Honolulu, HI), 1–6.
Harbitz, C. B., Løvholt, F., and Bungum, H. (2013). Submarine landslide tsunamis: how extreme and how likely? Nat. Hazards 72, 1341–1374. doi: 10.1007/s11069-013-0681-3
Harbitz, C. B., Løvholt, F., Pedersen, G. K., and Masson, D. G. (2006). Mechanisms of tsunami generation by submarine landslides: a short review. Norw. J. Geol. 86, 255–264.
Haugen, K. B., Løvholt, F., and Harbitz, C. B. (2005). Fundamental mechanisms for tsunami generation by submarine mass flows in idealised geometries. Mar. Petrol. Geol. 22, 209–217. doi: 10.1016/j.marpetgeo.2004.10.016
Heller, V., and Hager, W. H. (2011). Wave types of landslide generated impulse waves. Ocean Eng. 38, 630–640. doi: 10.1016/j.oceaneng.2010.12.010
Heller, V., and Spinneken, J. (2013). Improved landslide-tsunami prediction: effects of block model parameters and slide model. J. Geophys. Res. Oceans 118, 1489–1507. doi: 10.1002/jgrc.20099
Horrillo, J., Grilli, S. T., Nicolsky, D., Roeber, V., and Zhang, J. (2015). Performance benchmarking tsunami models for NTHMP's inundation mapping activities. Pure Appl. Geophys. 172, 869–884. doi: 10.1007/s00024-014-0891-y
Hungr, O., Corominas, J., and Eberhardt, E. (2005). Estimating landslide motion mechanism, travel distance and velocity, in Landslide Risk Management (London, UK: CRC Press), 109–138. doi: 10.1201/9781439833711-7
INGV (2019). Comunicato Straordinario Stromboli 04/07/2019. Press release n.16, Istituto Nazionale di Geofisica e Vulcanologia. Available online at: http://www.ingv.it/it/stampa-e-urp/stampa/comunicati-stampa-1/2019/37-comunicato-straordinario-stromboli-04--07-2019--09-00-utc-aggiornamento-sul-fenomeno-in-corso/file
Kirby, J., Grilli, S., Zhang, C., Horrillo, J., Nicolsky, D., and Liu, P. L. F. (2018). The NTHMP Landslide Tsunami Benchmark Workshop, Galveston, January 9–11 2017. Technical Report, Center for Applied Coastal Research.
Lacanna, G., and Ripepe, M. (2020). Genesis of tsunami waves generated by pyroclastic flows and the early-warning system, in Rittmann Conference 2020, Session S13. The Summer 2019 Stromboli Paroxysms:A Precious Opportunity to Expand the Knowledge on the Volcano (Catania).
Latter, J. H. (1981). Tsunamis of volcanic origin: summary of causes, with particular reference to Krakatoa 1883. Bull. Volcanol. 44, 467–490. doi: 10.1007/BF02600578
LGS (2019a). Esplosione parossistica del vulcano stromboli del 03/07/2019. Report to the Italian Department of Civil Protection, Università di Firenze. Available online at: http://lgs.geo.unifi.it/index.php/reports/comunicati?view=document&id=7:esplosione-parossistica-03-07-2019&catid=14
LGS (2019b). Esplosione parossistica del vulcano stromboli del 28/08/2019. Report to the Italian Department of Civil Protection, Università di Firenze. Available online at: http://lgs.geo.unifi.it/index.php/reports/comunicati?view=document&id=8:esplosione-parossistica-28-08-2019&catid=14
Liu, P. L. F., Higuera, P., Husrin, S., Prasetya, G. S., Prihantono, J., Diastomo, H., et al. (2020). Coastal landslides in Palu Bay during 2018 Sulawesi earthquake and tsunami. Landslides 17, 2085–2098. doi: 10.1007/s10346-020-01417-3
Løvholt, F., Harbitz, C. B., and Haugen, K. B. (2005). A parametric study of tsunamis generated by submarine slides in the Ormen Lange/Storegga area off western Norway. Marine Petrol. Geol. 22, 219–231. doi: 10.1016/j.marpetgeo.2004.10.017
Løvholt, F., Lorito, S., Macías, J., Volpe, M., Selva, J., and Gibbons, S. (2019). Urgent tsunami computing, in 2019 IEEE/ACM HPC for Urgent Decision Making (UrgentHPC) (Denver, CO: IEEE), 45–50. doi: 10.1109/UrgentHPC49580.2019.00011
Løvholt, F., Pedersen, G., Harbitz, C. B., Glimsdal, S., and Kim, J. (2015). On the characteristics of landslide tsunamis. Philos. Trans. R. Soc. A Math. Phys. Eng. Sci. 373:20140376. doi: 10.1098/rsta.2014.0376
LTMBW (2017). Landslide Tsunami Model Benchmarking Workshop, Galveston, Texas 2017. Available online at: http://www1.udel.edu/kirby/landslide/index.html
Lube, G., Breard, E. C. P., Esposti Ongaro, T., Dufek, J., and Brand, B. (2020). Multiphase flow behaviour and hazard prediction of pyroclastic density currents. Nat. Rev. Earth Environ. 1, 348–365. doi: 10.1038/s43017-020-0064-8
Lynett, P. J., Gately, K., Wilson, R., Montoya, L., Arcas, D., Aytore, B., et al. (2017). Inter-model analysis of tsunami-induced coastal currents. Ocean Model. 114, 14–32. doi: 10.1016/j.ocemod.2017.04.003
Ma, G., Kirby, J. T., Hsu, T. J., and Shi, F. (2015). A two-layer granular landslide model for tsunami wave generation: theory and computation. Ocean Model. 93, 40–55. doi: 10.1016/j.ocemod.2015.07.012
Ma, G., Kirby, J. T., and Shi, F. (2013). Numerical simulation of tsunami waves generated by deformable submarine landslides. Ocean Model. 69, 146–165. doi: 10.1016/j.ocemod.2013.07.001
Ma, G., Shi, F., and Kirby, J. T. (2012). Shock-capturing non-hydrostatic model for fully dispersive surface wave processes. Ocean Model. 43–44, 22–35. doi: 10.1016/j.ocemod.2011.12.002
Macías, J., and de la Asunción, M. (2019). Faster and faster tsunami simulations with ChEESE, in AGU Fall Meeting, Session NH33A-06 (San Francisco, CA).
Macías, J., Escalante, C., and Castro, M. J. (2020a). Multilayer-HySEA model validation for landslide generated tsunamis. Part I rigid slides. Nat. Hazards Earth Syst. Sci. 21, 775–789. doi: 10.5194/nhess-21-775-2021
Macías, J., Escalante, C., and Castro, M. J. (2020b). Multilayer-HySEA model validation for landslide generated tsunamis. Part II Granular slides. Nat. Hazards Earth Syst. Sci. 21, 791–805. doi: 10.5194/nhess-21-791-2021
Macías, J., Vázquez, J. T., Fernández-Salas, L. M., González-Vida, J. M., Bárcenas, P., Castro, M. J., et al. (2015). The Al-Borani submarine landslide and associated tsunami. A modelling approach. Marine Geol. 361, 79–95. doi: 10.1016/j.margeo.2014.12.006
Maramai, A., Graziani, L., Alessio, G., Burrato, P., Colini, L., Cucci, L., et al. (2005a). Near- and far-field survey report of the 30 December 2002 Stromboli (Southern Italy) tsunami. Marine Geol. 215, 93–106. doi: 10.1016/j.margeo.2004.11.009
Maramai, A., Graziani, L., and Tinti, S. (2005b). Tsunamis in the Aeolian Islands (Southern Italy): a review. Marine Geol. 215, 11–21. doi: 10.1016/j.margeo.2004.03.018
Marani, M. P., Gamberi, F., Rosi, M., Bertagnini, A., and di Roberto, A. (2008). Subaqueous density flow processes and deposits of an island volcano landslide (Stromboli Island, Italy). Sedimentology 56, 1488–1504. doi: 10.1111/j.1365-3091.2008.01043.x
Masson, D. G., Harbitz, C. B., Wynn, R. B., Pedersen, G., and Løvholt, F. (2006). Submarine landslides: processes, triggers and hazard prediction. Philos. Trans. R. Soc. A 364, 2009–2039. doi: 10.1098/rsta.2006.1810
Mohammed, F., and Fritz, H. M. (2012). Physical modeling of tsunamis generated by three-dimensional deformable granular landslides. J. Geophys. Res. 117:C11015. doi: 10.1029/2011JC007850
Montagna, F., Bellotti, G., and Di Risio, M. (2011). 3D numerical modeling of landslide-generated tsunamis around a conical island. Nat. Hazards 58, 591–608. doi: 10.1007/s11069-010-9689-0
Murty, T. S. (2003). Tsunami wave height dependence on landslide volume. Pure Appl. Geophys. 160, 2147–2153. doi: 10.1007/s00024-003-2423-z
Paris, R. (2015). Source mechanisms of volcanic tsunamis. Philos. Trans. R. Soc. A 373:20140380. doi: 10.1098/rsta.2014.0380
Paris, R., Switzer, A. D., Belousova, M., Belousov, A., Ontowirjo, B., Whelley, P. L., et al. (2013). Volcanic tsunami: a review of source mechanisms, past events and hazards in Southeast Asia (Indonesia, Philippines, Papua New Guinea). Nat. Hazards 70, 447–470. doi: 10.1007/s11069-013-0822-8
Pistolesi, M., Bertagnini, A., di Roberto, A., Ripepe, M., and Rosi, M. (2020). Tsunami and tephra deposits record interactions between past eruptive activity and landslides at Stromboli volcano, Italy. Geology 48, 436–440. doi: 10.1130/G47331.1
Pouliquen, O., and Forterre, Y. (2002). Friction law for dense granular flows: application to the motion of a mass down a rough inclined plane. J. Fluid Mech. 453, 1–19. doi: 10.1017/S0022112001006796
Pudasaini, S. P., and Mergili, M. (2019). A multi-phase mass flow model. J. Geophys. Res. Earth 124, 2920–2942. doi: 10.1029/2019JF005204
Roche, O., Attali, M., Mangeney, A., and Lucas, A. (2011). On the run-out distance of geophysical gravitational flows: insight from fluidized granular collapse experiments. Earth Planet. Sci. Lett. 311, 375–385. doi: 10.1016/j.epsl.2011.09.023
Romano, A., Bellotti, G., and Di Risio, M. (2013). Wavenumber–frequency analysis of the landslide-generated tsunamis at a conical island. Coast. Eng. 81, 32–43. doi: 10.1016/j.coastaleng.2013.06.007
Romano, A., Di Risio, M., Bellotti, G., Molfetta, M. G., Damiani, L., and De Girolamo, P. (2016). Tsunamis generated by landslides at the coast of conical islands: experimental benchmark dataset for mathematical model validation. Landslides 13, 1379–1393. doi: 10.1007/s10346-016-0696-4
Rosi, M., Levi, S. T., Pistolesi, M., Bertagnini, A., Brunelli, D., x000F2, V. C., et al. (2019). Geoarchaeological evidence of middle-age tsunamis at Stromboli and consequences for the tsunami hazard in the Southern Tyrrhenian sea. Sci. Rep. 9:677. doi: 10.1038/s41598-018-37050-3
Rosi, M., Pistolesi, M., Bertagnini, A., Landi, P., Pompilio, M., and Di Roberto, A. (2013). Chapter 14 Stromboli volcano, Aeolian Islands (Italy): present eruptive activity and hazards. Geol. Soc. Lond. Mem. 37, 473–490. doi: 10.1144/M37.14
Ruff, L. J. (2003). Some aspects of energy balance and tsunami generation by earthquakes and landslides. Pure Appl. Geophys. 160, 2155–2176. doi: 10.1007/s00024-003-2424-y
Ruffini, G., Heller, V., and Briganti, R. (2019). Numerical modelling of landslide-tsunami propagation in a wide range of idealised water body geometries. Coast. Eng. 153:103518. doi: 10.1016/j.coastaleng.2019.103518
Savage, S. B., and Hutter, K. (1989). The motion of a finite mass of granular material down a rough incline. J. Fluid Mech. 199, 177–215. doi: 10.1017/S0022112089000340
Schambach, L., Grilli, S. T., Kirby, J. T., and Shi, F. (2019). Landslide tsunami hazard along the upper US east coast: effects of slide deformation, bottom friction, and frequency dispersion. Pure Appl. Geophys. 176, 3059–3098. doi: 10.1007/s00024-018-1978-7
Schambach, L., Grilli, S. T., and Tappin, D. R. (2021). New high-resolution modeling of the 2018 Palu tsunami, based on supershear earthquake mechanisms and mapped coastal landslides, supports a dual source. Front. Earth Sci. 8:598839. doi: 10.3389/feart.2020.598839
Schambach, L., Grilli, S. T., Tappin, D. R., Gangemi, M. D., and Barbaro, G. (2020). New simulations and understanding of the 1908 Messina tsunami for a dual seismic and deep submarine mass failure source. Marine Geol. 421:106093. doi: 10.1016/j.margeo.2019.106093
Selva, J., Amato, A., Armigliato, A., Basili, R., Bernardi, F., Brizuela, B., et al. (2021). Tsunami Risk Management From Crustal Earthquakes and Non-seismic Sources in Italy. La Rivista del Nuovo Cimento. doi: 10.1007/s40766-021-00016-9
Synolakis, C. E., Bernard, E. N., Titov, V. B., Kânoglu, U., and González, F. I. (2007). Standards, Criteria, and Procedures for NOAA Evaluation of Tsunami Numerical Models. Technical Report OAR PMEL-135, NOAA, Seattle, WA.
Tappin, D. R., Grilli, S. T., Harris, J. C., Geller, R. J., Masterlark, T., Kirby, J. T., et al. (2014). Did a submarine landslide contribute to the 2011 Tohoku tsunami? Marine Geol. 357, 344–361. doi: 10.1016/j.margeo.2014.09.043
Tibaldi, A. (2001). Multiple sector collapses at stromboli volcano, Italy: how they work. Bull. Volcanol. 63, 112–125. doi: 10.1007/s004450100129
Tinti, S., and Bortolucci, E. (2000). Energy of water waves induced by submarine landslides. Pure Appl. Geophys. 157, 281–318. doi: 10.1007/s000240050001
Tinti, S., Bortolucci, E., and Romagnoli, C. (2000). Computer simulations of tsunamis due to sector collapse at Stromboli, Italy. J. Volcanol. Geotherm. Res. 96, 103–128. doi: 10.1016/S0377-0273(99)00138-9
Tinti, S., Maramai, A., Armigliato, A., Graziani, L., Manucci, A., Pagnoni, G., et al. (2005). Observations of physical effects from tsunamis of December 30 2002 at Stromboli volcano, southern Italy. Bull. Volcanol. 68, 450–461. doi: 10.1007/s00445-005-0021-x
Tinti, S., Pagnoni, G., and Piatanesi, A. (2003a). Simulation of tsunamis induced by volcanic activity in the Gulf of Naples (Italy). Nat. Hazards Earth Syst. Sci. 3, 311–320. doi: 10.5194/nhess-3-311-2003
Tinti, S., Pagnoni, G., and Zaniboni, F. (2006). The landslides and tsunamis of the 30th of December 2002 in Stromboli analysed through numerical simulations. Bull. Volcanol. 68, 462–479. doi: 10.1007/s00445-005-0022-9
Tinti, S., Pagnoni, G., Zaniboni, F., and Bortolucci, E. (2003b). Tsunami generation in Stromboli island and impact on the south-east Tyrrhenian coasts. Nat. Hazards Earth Syst. Sci. 3, 299–309. doi: 10.5194/nhess-3-299-2003
Tinti, S., Zaniboni, F., Pagnoni, G., and Manucci, A. (2008). Stromboli island (Italy): scenarios of tsunamis generated by submarine landslides. Pure Appl. Geophys. 165, 2143–2167. doi: 10.1007/s00024-008-0420-y
Walder, J. S. (2003). Tsunamis generated by subaerial mass flows. J. Geophys. Res. 108:2236. doi: 10.1029/2001JB000707
Wang, J., Ward, S. N., and Xiao, L. (2019). Tsunami Squares modeling of landslide generated impulsive waves and its application to the 1792 Unzen-Mayuyama mega-slide in Japan. Eng. Geol. 256, 121–137. doi: 10.1016/j.enggeo.2019.04.020
Ward, S. N. (2001). Landslide tsunami. J. Geophys. Res. Oceans 106, 11201–11215. doi: 10.1029/2000JB900450
Ward, S. N., and Day, S. (2001). Cumbre Vieja Volcano—potential collapse and tsunami at La Palma, Canary Islands. Geophys. Res. Lett. 28, 3397–3400. doi: 10.1029/2001GL013110
Watts, P. (1998). Wavemaker curves for tsunamis generated by underwater landslides. J. Waterway Port Coast. Ocean Eng. 124, 127–137. doi: 10.1061/(ASCE)0733-950X(1998)124:3(127)
Watts, P., and Waythomas, C. F. (2003). Theoretical analysis of tsunami generation by pyroclastic flows. J. Geophys. Res. 108:2563. doi: 10.1029/2002JB002265
Yavari-Ramshe, S., and Ataie-Ashtiani, B. (2016). Numerical modeling of subaerial and submarine landslide-generated tsunami waves—recent advances and future challenges. Landslides 13, 1325–1368. doi: 10.1007/s10346-016-0734-2
Yavari-Ramshe, S., and Ataie-Ashtiani, B. (2017). A rigorous finite volume model to simulate subaerial and submarine landslide-generated waves. Landslides 14, 203–221. doi: 10.1007/s10346-015-0662-6
Young, C. C., and Wu, C. H. (2009). An efficient and accurate non-hydrostatic model with embedded Boussinesq-type like equations for surface wave modeling. Int. J. Numer. Methods Fluids 60, 27–53. doi: 10.1002/fld.1876
Zhang, C., Kirby, J. T., Shi, F., Ma, G., and Grilli, S. T. (2021a). A two-layer non-hydrostatic landslide model for tsunami generation on irregular bathymetry. 1. Theoretical basis. Ocean Model. 159:101749. doi: 10.1016/j.ocemod.2020.101749
Zhang, C., Kirby, J. T., Shi, F., Ma, G., and Grilli, S. T. (2021b). A two-layer non-hydrostatic landslide model for tsunami generation on irregular bathymetry. 2. Numerical discretization and model validation. Ocean Model. 160:101769. doi: 10.1016/j.ocemod.2021.101769
Keywords: landslide, tsunami, volcano, Stromboli, numerical simulation, benchmark, hazard assessment
Citation: Esposti Ongaro T, de' Michieli Vitturi M, Cerminara M, Fornaciai A, Nannipieri L, Favalli M, Calusi B, Macías J, Castro MJ, Ortega S, González-Vida JM and Escalante C (2021) Modeling Tsunamis Generated by Submarine Landslides at Stromboli Volcano (Aeolian Islands, Italy): A Numerical Benchmark Study. Front. Earth Sci. 9:628652. doi: 10.3389/feart.2021.628652
Received: 12 November 2020; Accepted: 30 March 2021;
Published: 07 May 2021.
Edited by:
Jörn Behrens, Universität Hamburg, GermanyReviewed by:
Paris Raphael, UMR6524 Laboratoire Magmas et Volcans (LMV), FranceStephan Grilli, University of Rhode Island, United States
Valentin Heller, University of Nottingham, United Kingdom
Copyright © 2021 Esposti Ongaro, de' Michieli Vitturi, Cerminara, Fornaciai, Nannipieri, Favalli, Calusi, Macías, Castro, Ortega, González-Vida and Escalante. This is an open-access article distributed under the terms of the Creative Commons Attribution License (CC BY). The use, distribution or reproduction in other forums is permitted, provided the original author(s) and the copyright owner(s) are credited and that the original publication in this journal is cited, in accordance with accepted academic practice. No use, distribution or reproduction is permitted which does not comply with these terms.
*Correspondence: Tomaso Esposti Ongaro, tomaso.espostiongaro@ingv.it