Causal associations between gut microbiota, gut microbiota-derived metabolites, and cerebrovascular diseases: a multivariable Mendelian randomization study
- 1School of Medicine, Jishou University, Jishou, China
- 2Department of Neurosurgery, The First Affiliated Hospital of Jishou University, Jishou, China
- 3Department of Neurology, The First Affiliated Hospital of Jishou University, Jishou, China
- 4Department of Orthopedics, The Affiliated Changsha Central Hospital, Changsha, China
Background: Mounting evidence has demonstrated the associations between gut microbiota, gut microbiota-derived metabolites, and cerebrovascular diseases (CVDs). The major categories of CVD are ischemic stroke (IS), intracerebral hemorrhage (ICH), and subarachnoid hemorrhage (SAH). However, the causal relationship is still unclear.
Methods: A two-sample Mendelian randomization (MR) study was conducted leveraging the summary data from genome-wide association studies. The inverse variance-weighted, maximum likelihood, weighted median, and MR.RAPS methods were performed to detect the causal relationship. Several sensitivity analyses were carried out to evaluate potential horizontal pleiotropy and heterogeneity. Finally, reverse MR analysis was conducted to examine the likelihood of reverse causality, and multivariable MR was performed to adjust the potential confounders.
Results: We collected 1,505 host single nucleotide polymorphisms (SNPs) linked to 119 gut microbiota traits and 1,873 host SNPs associated with 81 gut metabolite traits as exposure data. Among these, three gut bacteria indicated an elevated risk of IS, two of ICH, and one of SAH. In contrast, five gut bacteria were associated with a reduced risk of IS, one with ICH, and one with SAH. Our study also demonstrated the potential causal associations between 11 gut microbiota-derived metabolites and CVD.
Conclusions: This study provided evidence of the causal relationship between gut microbiota, gut microbiota-derived metabolites, and CVD, thereby offering novel perspectives on gut biomarkers and targeted prevention and treatment for CVD.
Introduction
Cerebrovascular diseases (CVDs) are characterized by pathological changes in cerebral blood vessels resulting in brain dysfunction, such as hemiplegia and language disorder (World Health Organization, 2021). The major categories are ischemic stroke (IS), intracerebral hemorrhage (ICH), and subarachnoid hemorrhage (SAH). Despite years of prevention and treatment, CVD continues to be the second leading cause of mortality and disability globally (DALYs, G.B.D., and Collaborators, H, 2016; Mortality, G.B.D., and Causes of Death, C, 2016). With the aging of the population, the prevalence of CVD increases annually, which poses a substantial hazard to human life and places a significant burden on healthcare systems (Collaborators, G.B.D.C.o.D, 2017; Feigin et al., 2017). Nevertheless, the exact pathogenesis of CVD, as well as effective strategies for its prevention and treatment, remained uncertain.
Gut microbiota is a complex bacterial community that resides in the intestine. A growing body of research suggests that the gut microbiota and microbiota-derived metabolites play a significant role in CVD through the microbiota–gut–brain axis (Peh et al., 2022; Zou et al., 2022). Dysbiosis of the gut microbiome leads to increased gut permeability and activation of the intestinal immune system, allowing it to penetrate brain tissue via the blood–brain barrier (Hu et al., 2022). Clinical cross-sectional studies have identified gut microbiota disorders in CVD patients (Huang et al., 2019; Li et al., 2020; Ling et al., 2020b). It is reported that Firmicutes displayed a growing trend, but Bacteroidetes had a declining tendency in stroke patients (Singh et al., 2016). However, existing studies have produced inconsistent results. For instance, Yin et al. observed a significant decrease in the abundance of Bacteroides and Prevotella in IS patients compared with healthy controls (Yin et al., 2015), while another small cross-sectional study (N = 10) reported the opposite results (Wang et al., 2018). The gut microbiota-derived metabolites are key actors in host–microbiota crosstalk, which influences the host’s brain function and behavior, such as short-chain fatty acids (SCFAs) (Liu et al., 2020), trimethylamine N-oxide (TMAO) (Schiattarella et al., 2017), and butyrate (Haak et al., 2021). However, most evidence came from observational studies, making it difficult to determine the temporal association between exposure and outcome. Furthermore, confounding factors of gut microbiota–CVD-related studies were difficult to measure and control due to the complicated environment of the intestine and cerebral blood vessels.
Mendelian randomization (MR) analysis integrates data from genome-wide association studies (GWAS) and utilizes genetic variations as instrumental variables (IVs) to assess the causal relationship between exposures and outcomes (Greenland, 2000). The MR approach should conform to three fundamental assumptions: 1) IVs should be highly associated with exposure, 2) IVs should be independent of confounders that affect exposure and outcome, and 3) IVs should affect the outcome only through exposure. With the advantages of controlling confounders and eliminating reverse causality, MR analysis has been extensively utilized to investigate the causality between gut microbiota and human complex diseases like T2DM (Sanna et al., 2019), chronic kidney disease (Xu et al., 2020), and Alzheimer’s disease (Hughes et al., 2020). In the study, using summary data from the most extensive and up-to-date GWAS datasets, we employed the MR method to investigate the causal relationship between gut microbiota, gut microbiota-derived metabolites, and CVD.
Materials and methods
Data sources
Summary data for gut microbiota serving as exposure were obtained from a multi-ethnic GWAS, which includes 18,340 individuals (Kurilshikov et al., 2021). 16S rRNA gene sequencing profiles and genotyping data were coordinated to perform the genome-wide meta-analysis of the association between human genetic variants and the gut microbiota. Employing SILVA as the reference database (Quast et al., 2013), all the data were annotated to genus and higher levels.
Pooled data for gut microbiota-derived metabolites were obtained from the most comprehensive metabolite GWAS (Shin et al., 2014) so far, which was carried out among people of European ancestry (Twins UK and KORA cohort, N = 7,824). The GWAS examined 486 human metabolites obtained from both cohorts at each single nucleotide polymorphism (SNP). Then, we manually searched the HMDB database (Wishart et al., 2018) to screen out human gut microbiota-derived metabolites.
The MEGASTROKE consortium provided genetic variations for IS including 34,217 cases and 406,111 controls (Malik et al., 2018). Summary data for ICH and SAH were obtained from the FinnGen consortium data (Kurki et al., 2022), which included 202,833 subjects (1,687 cases/201,146 controls) and 16,381,733 subjects (1,338 cases/16,380,395 controls), respectively. Genotyped by the Illumina platform and Affymetrix chip arrays, association studies were performed with sex, age, genotyping batch, and 10 main components adjusted.
All GWAS datasets were publicly available with limited sample overlap and already obtained ethical approvals in original studies. Details of GWAS utilized in this study are shown in Supplementary Table 1.
Selection of instrumental variables
In order to select optimal instrumental variables (IVs), several quality control steps were conducted. First, IVs were selected from SNPs associated with gut microbiota and gut microbiota-derived metabolites at the locus-wide significance level (P< 1 × 10−5) (Benjamini and Hochberg, 1995). Second, the PLINK clumping method on linkage disequilibrium (LD) with r2<0.001 and a clumping window of 10,000 kb was performed to identify the lead SNPs. Third, we calculated each exposure’s F-statistic using the formula , where R2, n, and k mean the estimated exposure variance explained by IVs, sample size, and the number of IVs, respectively (Palmer et al., 2012). If the F-statistics were below 10, the IVs would be excluded to prevent weak IVs’ bias (Staiger and Stock, 1997).
Statistical analyses
To estimate the causality between gut microbiota, gut microbiota-derived metabolites, and CVD, the two-sample MR analysis was performed. The inverse variance-weighted (IVW) method was performed as the primary MR analysis, which is a meta-analysis method that combines the Wald ratio estimates of each IV and restricts the intercept to zero. If there is no horizontal pleiotropy, results from IVW would be unbiased (Burgess et al., 2016). Effect estimates for causal associations were reported in odds ratios with 95% confidence intervals for binary outcomes (Dekking, 2007; Szumilas, 2010). In addition, P-values were adjusted for several comparisons at the significance level (q-value< 0.1) by false discovery rate (FDR) (Storey and Tibshirani, 2003). If exposures with P<0.05 while FDR corrected q-value >0.1, they were reported as potentially causal associations. Other sensitivity analyses were carried out to ensure the reliability of the results, including maximum likelihood (Thompson et al., 2005), weighted median (Bowden et al., 2016), and MR robust adjusted profile score (MR.RAPS) (Zhao et al., 2020). When heterogeneity and horizontal pleiotropy are assumed to be absent, the maximum likelihood method is comparable to IVW with smaller standard errors and more unbiased results (Pierce and Burgess, 2013). The weighted median method can obtain robust assumptions when less than 50% of SNPs are invalid (Bowden et al., 2016). MR.RAPS offers robust estimates to correct for systematic and idiosyncratic pleiotropy (Zhao et al., 2020).
Cochran Q statistic was performed to examine the heterogeneity, and the leave-one-out sensitivity analysis was employed to examine if each SNP was accountable for the causative outcomes (Hemani et al., 2017). Moreover, the MR-Egger intercept and the Mendelian randomization pleiotropy residual sum and outlier (MR-PRESSO) global test were applied to detect the potential horizontal pleiotropy between IVs and the outcome (Hemani et al., 2017).
Reverse MR analysis was also conducted to investigate the reverse causality between CVD and gut microbiota as well as gut microbiota-derived metabolites, which used the same setting and datasets as in the forward MR analysis except for changing the original exposure to outcome. Finally, we performed a multivariable MR (MVMR) analysis to distinguish each confounder’s direct influence (Burgess and Thompson, 2015). Three confounders, smoking (IEU number: ieu-b-4877), alcohol drinking (IEU number: ukb-b-5779), and hypertension (IEU number: ukb-a-61), were considered for the MVMR analysis by using the IVW method (Burgess and Thompson, 2015).
The flowchart of this study is displayed in Figure 1. All statistical analyses were performed using “TwoSampleMR,” “MRPRESSO,” and “qvalue” packages in R software.
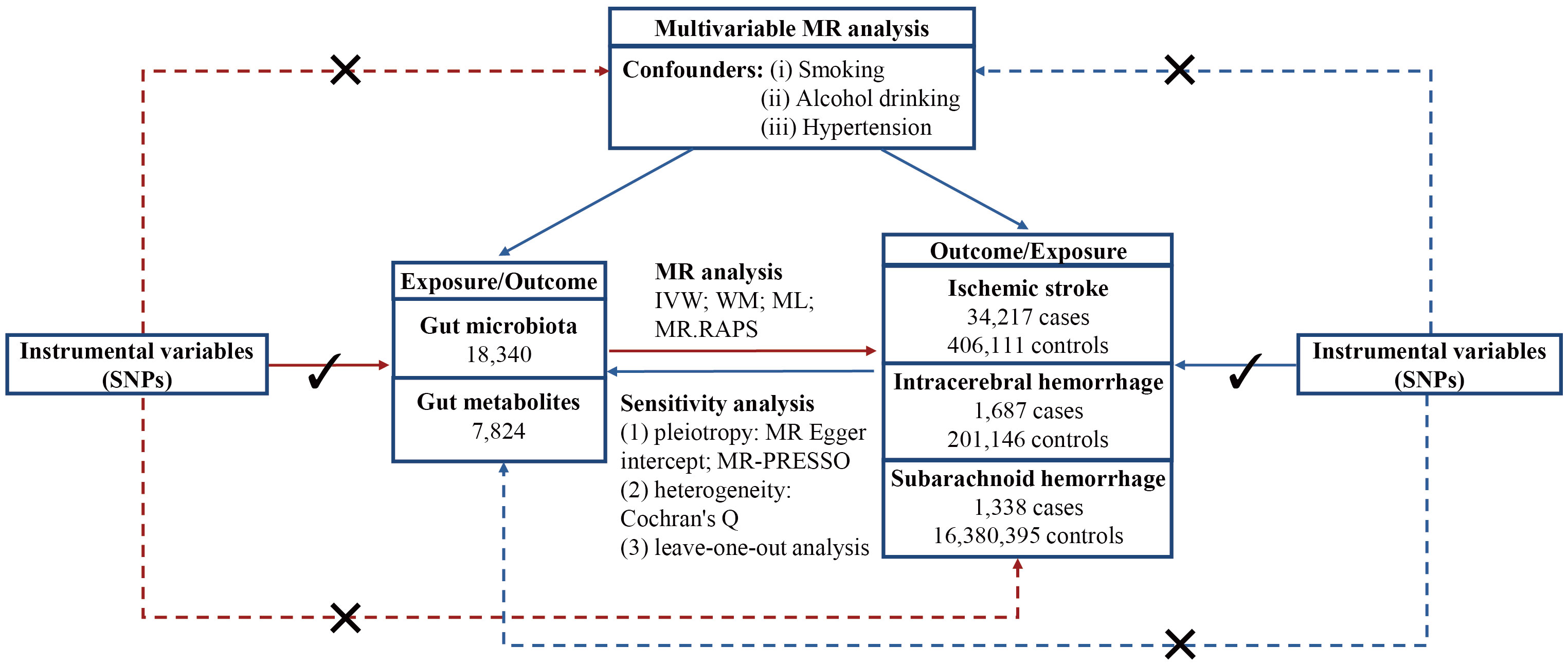
Figure 1 Flowchart of this study. MR, Mendelian randomization; SNP, single nucleotide polymorphism; IVW, inverse variance weighted; WM, weighted mean; ML, maximum likelihood; MR.RAPS, Mendelian randomization robust adjusted profile score; MR-PRESSO, Mendelian randomization pleiotropy residual sum and outlier.
Results
A total of 1,505 lead SNPs associated with 119 bacterial genera were included for gut microbiota, and 1,873 lead SNPs associated with 81 traits were identified for gut microbiota-derived metabolites. The characters of the selected IVs are presented in Supplementary Tables 2-5.
Association between gut microbiota and cerebrovascular diseases
The IVW estimations showing higher genetically predicted Allisonella (OR, 1.08; 95% CI, 1.019–1.150; P = 0.011), Paraprevotella (OR, 1.094; 95% CI, 1.022–1.172; P = 0.010), and Streptococcus (OR, 1.166; 95% CI, 1.049–1.297; P = 0.004) were associated with a higher risk of IS, while genetically increased Barnesiella (OR, 0.899; 95% CI, 0.809–0.999; P = 0.048), Intestinimonas (OR, 0.889; 95% CI, 0.820–0.963; P = 0.004), LachnospiraceaeFCS020group (OR, 0.905; 95% CI, 0.823–0.995; P = 0.039), LachnospiraceaeNK4A136group (OR, 0.893; 95% CI, 0.816–0.977; P = 0.014), and RuminococcaceaeUCG004 (OR, 0.913; 95% CI, 0.835–0.999; P = 0.048) were associated with a lower risk of IS. However, there is no significant causal association after q-value adjustment (Figure 2).
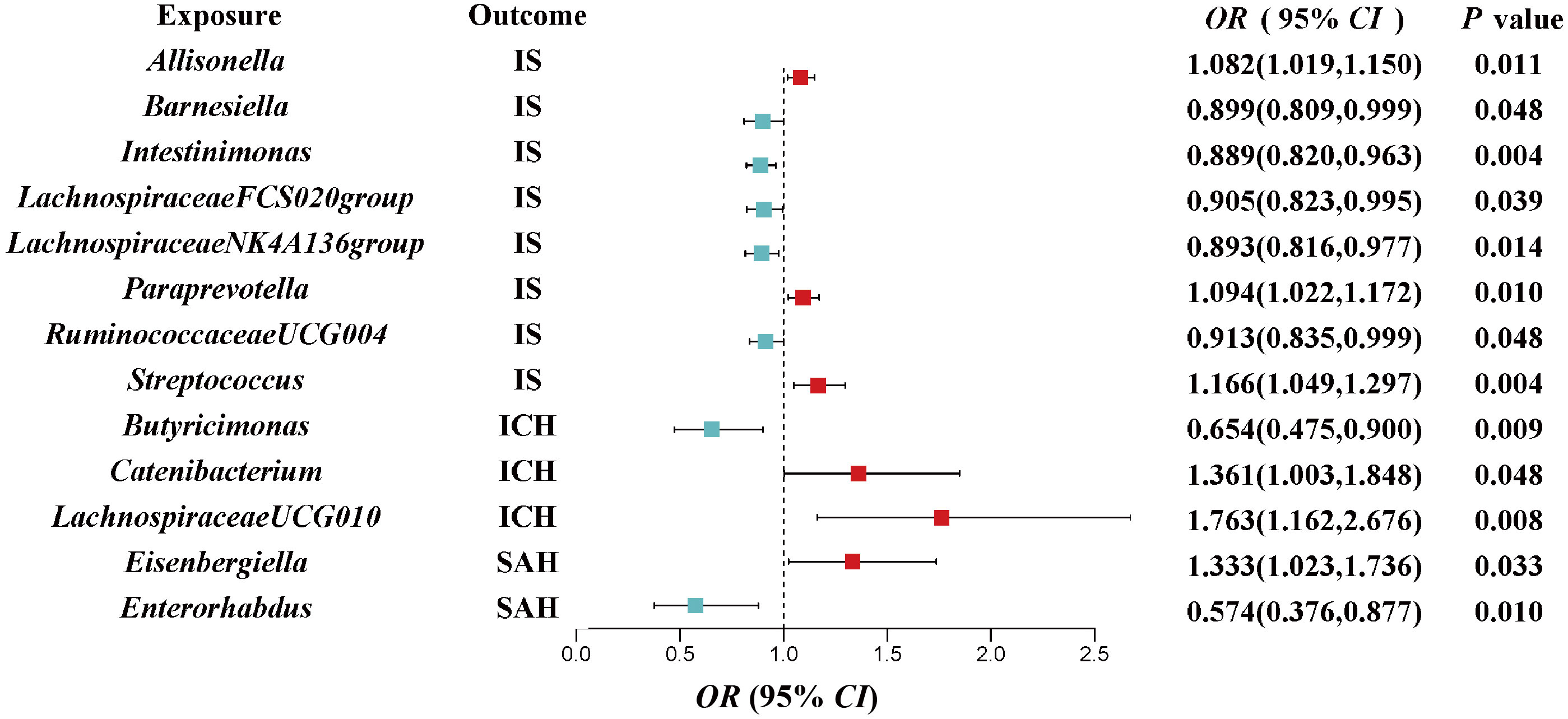
Figure 2 Associations of genetically predicted gut microbiota with risk of CVD using the IVW method. The colored block represents the point estimation of OR and the horizontal black line represents the 95% CI of OR. CVD, cerebrovascular disease; IVW, inverse variance weighted; IS, ischemic stroke; ICH, intracerebral hemorrhage; SAH, subarachnoid hemorrhage; OR, odds ratio; CI, confidence interval.
We also investigated the potential causality between gut microbiota and ICH as well as SAH, using the same methods, respectively. Our results indicated that genetically higher abundances of Catenibacterium (OR, 1.361; 95% CI, 1.003–1.848; P = 0.0423) and LachnospiraceaeUCG010 (OR, 1.763; 95% CI, 1.162–2.676; P = 0.008) were associated with a higher risk of ICH, but higher abundances of Butyricimonas (OR, 0.654; 95% CI, 0.475–0.9; P = 0.009) were associated with a protective effect against ICH. Additionally, an increase in Eisenbergiella (OR, 1.333; 95% CI, 1.023–1.736; P = 0.033) was related to a greater risk of SAH, but an increase in Enterorhabdus (OR, 0.574; 95% CI, 0.376–0.877; P = 0.01) was associated with a decreased probability of SAH (Figure 2).
In the sensitivity analyses, the association between gut microbiota and CVD remained consistent (Table 1, Figures 3–5). F-statistics varied from 18.41 to 59.85, indicating that no weak IV bias was observed. Additionally, Cochran Q statistic showed no notable heterogeneity, and the leave-one-out analysis indicated that the causal relationship cannot be driven by any single SNP. Moreover, the results of MR-PRESSO suggested that there was no significant horizontal pleiotropy (Supplementary Table 6, Supplementary Figures 1-3).
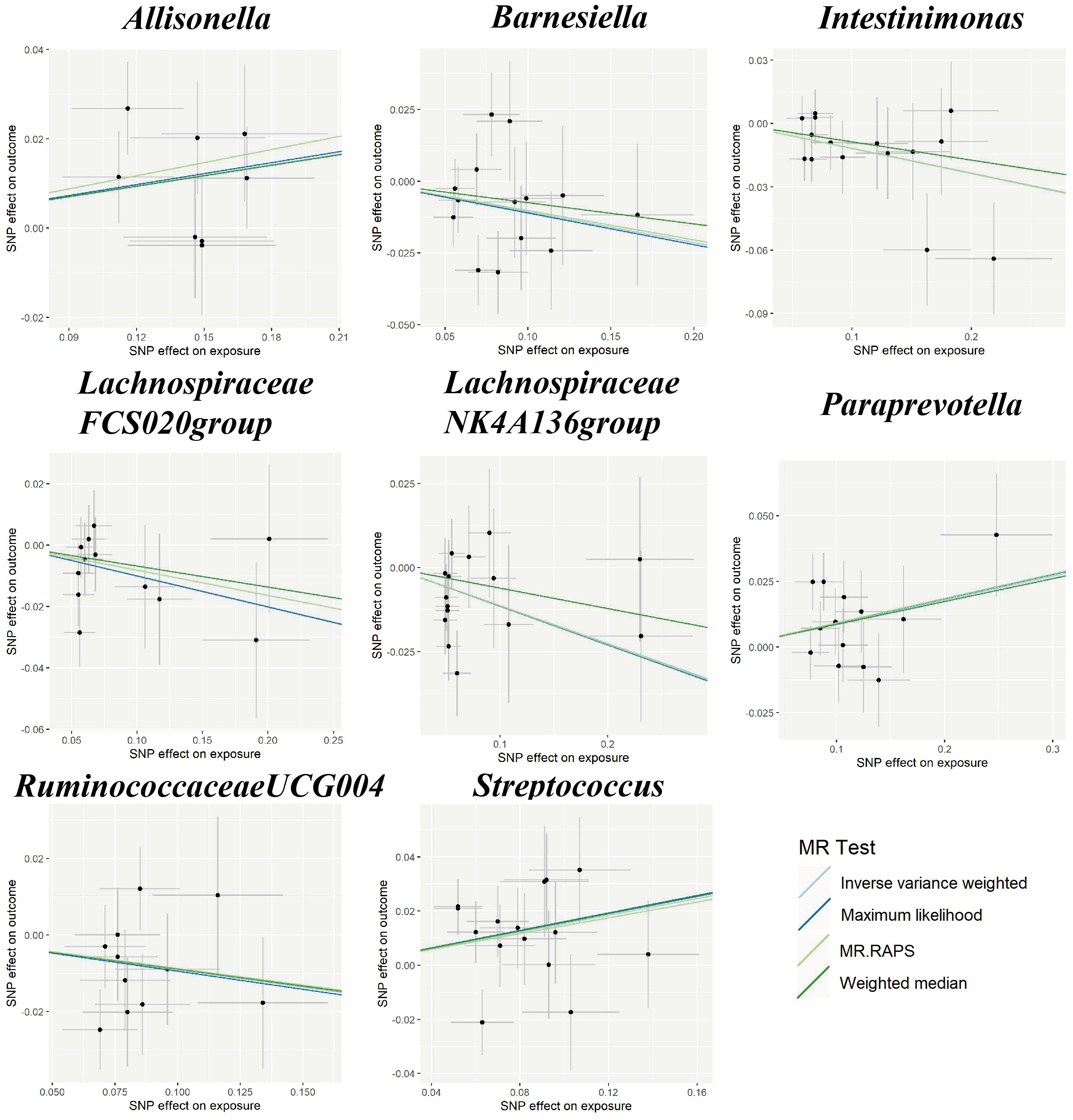
Figure 3 Scatter plots for the causal association between gut microbiota and IS. SNP effects were plotted into lines for the inverse variance-weighted test (light blue line), maximum likelihood (dark blue line), MR.RAPS (light green line), and weighted median (dark green line). The slope of the line corresponded to the causal estimation. IS, ischemic stroke; MR.RAPS, Mendelian randomization robust adjusted profile score.
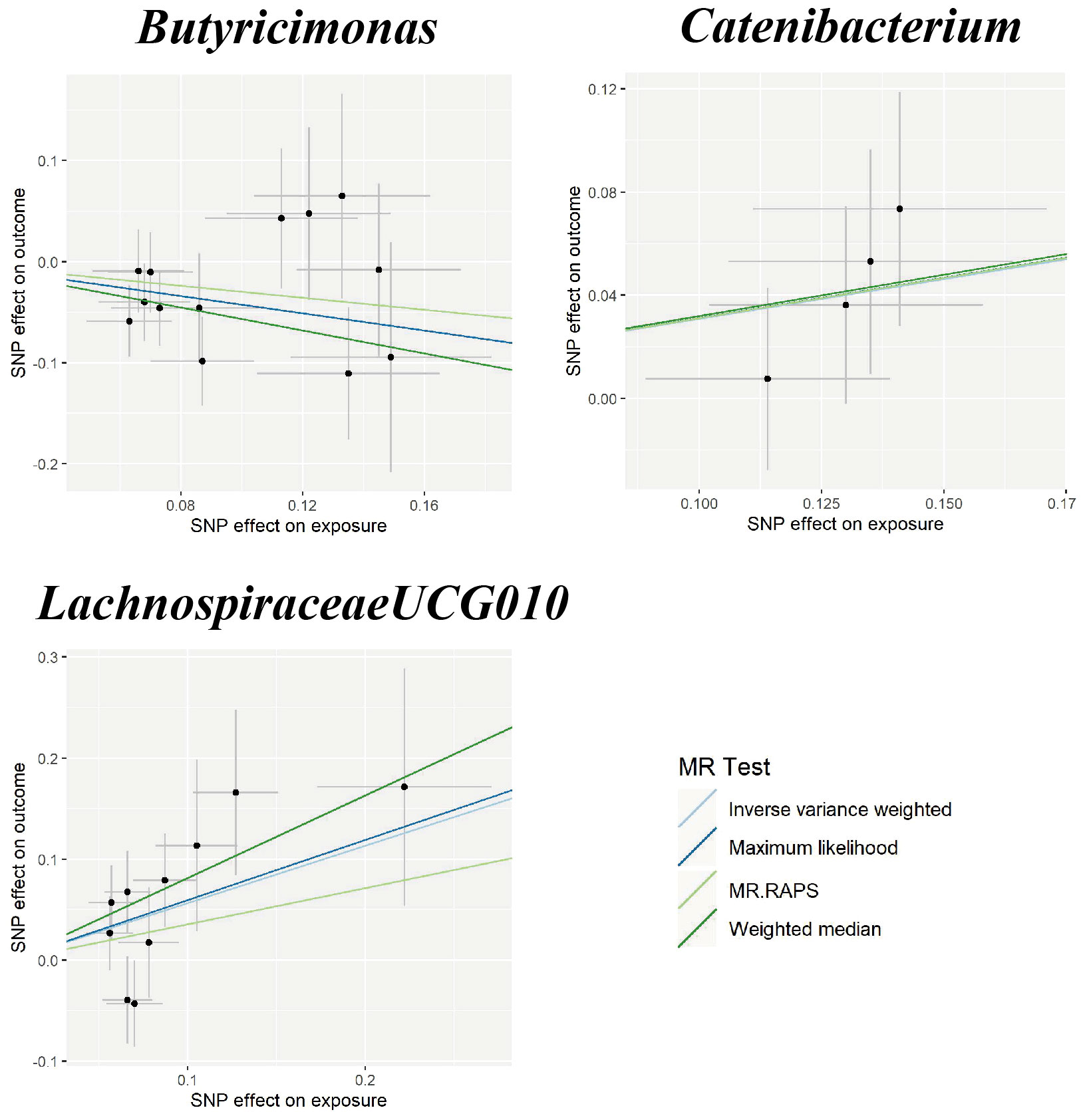
Figure 4 Scatter plots for the causal association between gut microbiota and ICH. SNP effects were plotted into lines for the inverse variance-weighted test (light blue line), maximum likelihood (dark blue line), MR.RAPS (light green line), and weighted median (dark green line). The slope of the line corresponded to the causal estimation. ICH, intracerebral hemorrhage; MR.RAPS, Mendelian randomization robust adjusted profile score.
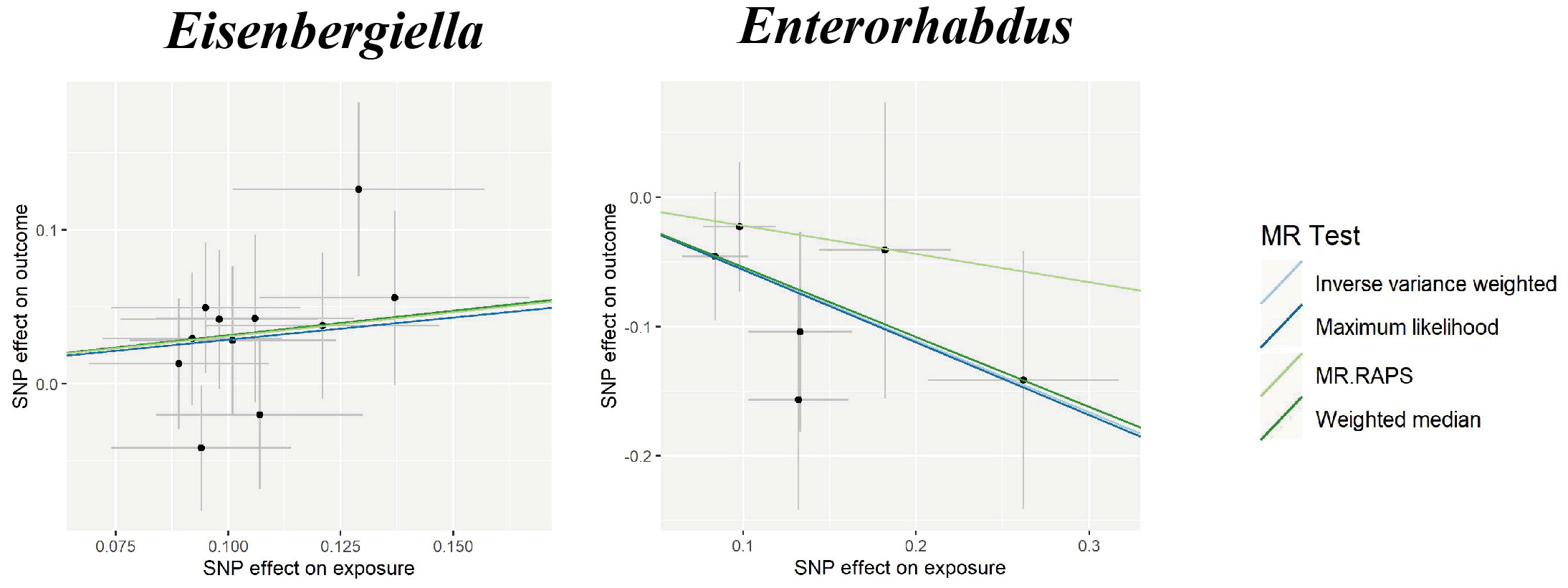
Figure 5 Scatter plots for the causal association between gut microbiota and SAH. SNP effects were plotted into lines for the inverse variance-weighted test (light blue line), maximum likelihood (dark blue line), MR.RAPS (light green line), and weighted median (dark green line). The slope of the line corresponded to the causal estimation. SAH, subarachnoid hemorrhage; MR.RAPS, Mendelian randomization robust adjusted profile score.
Nevertheless, the results of reverse MR did not reveal any correlations between CVD and gut microbiota (Supplementary Table 7). Detailed results of the sensitivity analyses are listed in Supplementary Table 8. We conducted an MVMR analysis to examine the cause–effect of gut microbiota on CVD after adjustment of three confounding factors (smoking, alcohol drinking, and hypertension). For the genus Streptococcus, after adjusting for alcohol drinking (OR = 1.182; 95% CI, 1.070–1.306; P = 0.001), smoking (OR = 1.189; 95% CI, 1.045–1.354; P = 0.009), and hypertension (OR = 1.168; 95% CI, 1.052–1.298; P = 0.004), the Streptococcus remained causally associated with CVD. We found that other gut microbiota continued to be causally related to CVD and had a more substantial impact than the causal effect found by univariable MR (Table 2).
Association between gut metabolites and cerebrovascular diseases
We obtained 11 suggestive estimates of the effects of gut metabolites on CVD among the 81 gut microbiota-derived metabolites that were included in our study. Increased abundances of cholesterol (OR, 1.639; 95% CI, 1.053–2.55; P = 0.028), leucine (OR, 1.548; 95% CI, 1.04–2.303; P = 0.031), and taurodeoxycholate (OR, 1.138; 95% CI, 1.005–1.289; P = 0.041) were found to be associated with a higher risk of IS, while increased glycerate (OR, 0.562; 95% CI, 0.319–0.993; P = 0.047), indolelactate (OR, 0.608; 95% CI, 0.396–0.936; P = 0.024), ornithine (OR, 0.384; 95% CI, 0.172–0.857; P = 0.019), and threonate (OR, 0.783; 95% CI, 0.617–0.994; P = 0.044) were protective factors for IS. Furthermore, 7α-hydroxy-3-oxo-4-cholestenoate (7-Hoca) (OR, 0.091; 95% CI, 0.013–0.659; P = 0.018), choline (OR, 0.02; 95% CI, 0.001–0.367; P = 0.008), and glycine (OR, 0.343; 95% CI, 0.147–0.8; P = 0.013) were revealed as protective factors of SAH, while tyrosine (OR, 5.908; 95% CI, 1.178–29.641; P = 0.099) was demonstrated to enhance the risk of ICH (Table 3).
Additionally, following the sensitivity analyses, those aforementioned results were deemed to be trustworthy without pleiotropy (Table 4, Supplementary Table 6, and Supplementary Figures 4-6), but we did not find a significant association after the q-value adjustment. Reverse MR analysis demonstrated that CVD had no causal association with gut microbiota-derived metabolites except for the association between IS and cholesterol, leucine, and taurodeoxycholate (Supplementary Tables 7, 8). We conducted an MVMR analysis to examine the causality of gut microbiota-derived metabolites on CVD after adjusting for confounding factors. For the protective factor ornithine, after adjusting for alcohol drinking (OR = 0.547; 95% CI, 0.326–0.916; P = 0.022), smoking (OR = 0.527; 95% CI, 0.348–0.798; P = 0.002), and hypertension (OR = 0.548; 95% CI, 0.311–0.967; P = 0.038), we found it continued to be causally associated with CVD and had a more significant impact than the causal relationship found by univariable MR (Table 5).
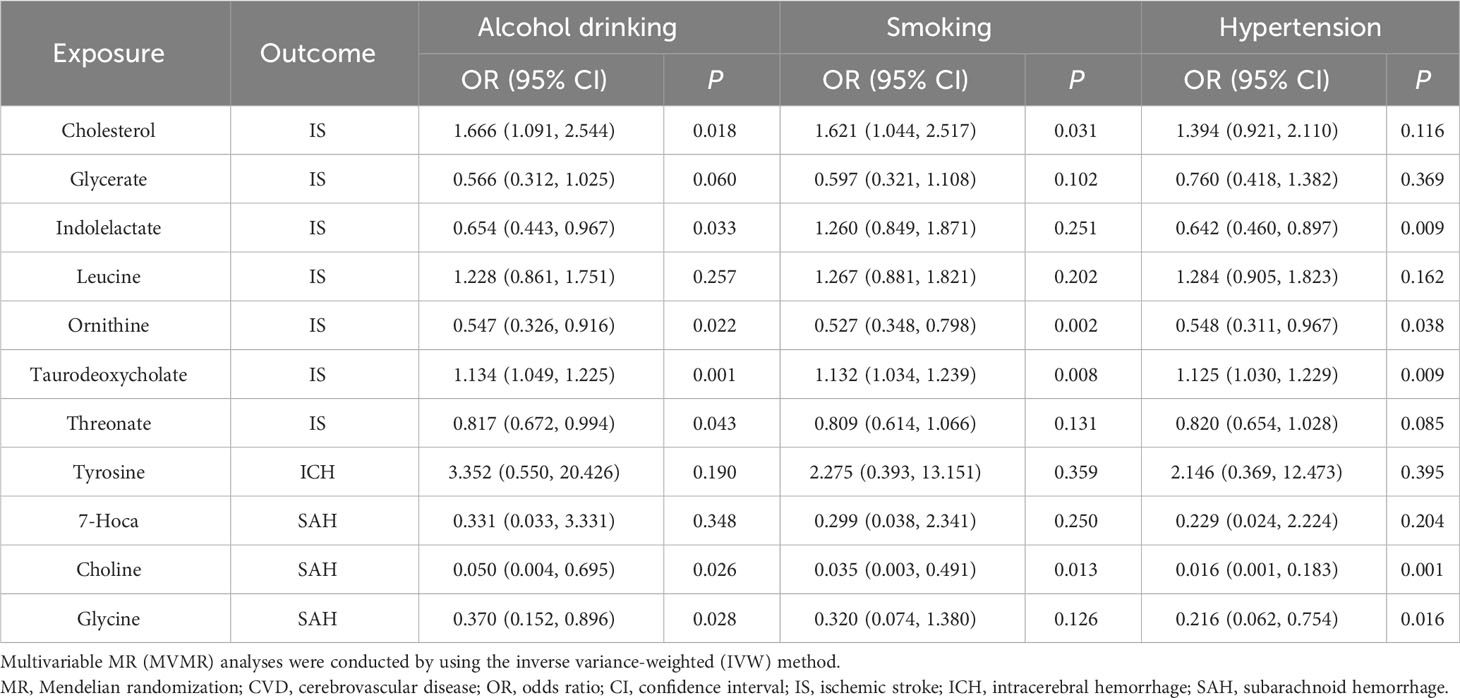
Table 5 Multivariable MR analyses of gut microbiota-derived metabolites on CVD after adjusting confounding factors.
Discussion
Previous studies suggested that the gut microbiome exhibits high heritability and plays a pivotal role in cardiovascular disease (Kurilshikov et al., 2021; Zou et al., 2022), emphasizing the need for conducting microbial genome-wide association studies (mGWAS) in CVD. In the present study, we performed MR analyses to explore the causal relationship between gut microbiota, gut microbiota-derived metabolites, and CVD. Using summary data from the largest and latest GWAS, we detected the causal associations between 13 gut microbial genera and CVD subtypes. Moreover, it was suggested that the increased concentration of 11 metabolites was potentially protective or risk factors for different CVD subtypes, respectively.
As for gut microbiome and IS, a previous MR study reported that the bacterial genera Intestinimonas and LachnospiraceaeNK4A136group play significant protective roles in more than one IS subtype (Meng et al., 2023), which supported our results. Consistent with other previous observational studies (Denes et al., 2014; Huang et al., 2019; Ling et al., 2020a), Streptococcus was demonstrated to be related to a higher risk of IS in this study. In-vitro experiments also found that Streptococcus infection promoted atherosclerosis and aggravated ischemic brain damage through platelet and IL-1-mediated systemic inflammation (Denes et al., 2014). In a population-based study, Zeng et al. observed significantly lower levels of butyrate-producing bacteria Lachnospiraceae and Ruminococcaceae in the high-risk stroke group (Zeng et al., 2019), which was in line with our study. It is noteworthy that our results indicate varying effects of LachnospiraceaeNK4A136group, LachnospiraceaeFCS020group, and LachnospiraceaeUCG010 on IS and ICH. This highlights the importance of conducting studies at a more specific species level and across different CVD subtypes to elucidate the potential mechanisms from the perspective of gut microbiota. As for ICH, a mouse model demonstrated a notably higher abundance of Butyricimonas in the exercise group (Liu et al., 2017). Regular exercise is known to reduce the risk of ICH (Carpenter et al., 2016), which supported our result that Butyricimonas exhibits a protective effect on the risk of ICH. Our findings indicated that Catenibacterium is associated with an elevated risk of ICH. Multiple observational studies have reported increased levels of Catenibacterium in obese patients (Gallardo-Becerra et al., 2020; Pinart et al., 2021). Meanwhile, a multicenter case–control study demonstrated that obesity can increase the risk of ICH (Pezzini et al., 2013). This suggests that Catenibacterium might influence ICH through the pathway of obesity. As for SAH, a previous study observed an increase in the relative abundance of Enterorhabdus following a low-calorie Mediterranean diet intervention (Pagliai et al., 2020). The Mediterranean diet is advised for preventing the development and rupture of cerebral aneurysms, which accounts for 85% of SAH cases (Czekajlo, 2019). The evidence above showed that Enterorhabdus may contribute to SAH risk with their function to the rupture of cerebral aneurysms.
Regarding the connection between gut microbiota-derived metabolites and CVD, consistent with our findings, a rat experiment using middle cerebral artery occlusion as the model confirmed the protective effect of ornithine against IS (Barakat et al., 2018). The NLRP3 inflammasome, characterized by leucine-rich repeat (LRR) domains at the C-terminus, promotes the initiation of an inflammatory response (Schroder and Tschopp, 2010). An experimental mouse model confirmed that NLRP3 inflammasome activation in neurons triggers neuroinflammation during acute IS. Early inhibition of NLRP3 reduces inflammation and stabilizes the blood–brain barrier, providing protection against ischemia/reperfusion injury (Franke et al., 2021). The evidence presented above supports our study’s conclusion that leucine is a risk factor for IS. Nagata et al. conducted a clinical trial to compare the cerebrospinal fluid of 6 aneurysmal SAH patients with 11 healthy controls and observed a higher concentration of 7-Hoca in patients (Nagata et al., 1995), which contradicted our findings. The limited sample size and sample heterogeneity are likely responsible for the conflicting results.
This study has several advantages. First, this study is the first to evaluate the causality between gut microbiota, gut microbiota-derived metabolites, and CVD. Second, the application of the MR method decreased the interference of confounding factors and the reverse causality of the results. Finally, based on the dataset from the largest GWAS up-to-date, we performed reverse MR, MVMR, and several sensitivity analyses to support the results. However, it has to be admitted that there are some limitations. First, the results should be interpreted with caution due to the inadequate IVs under genome-wide significance, which is why we utilized a loose cutoff for exposure-related SNPs at a threshold of P< 1 × 10−5. Second, bacterial taxa were only analyzed at the genus level rather than at a more specific species level. Finally, previous studies have demonstrated the contentious nature of gene–microbial interactions across diverse ethnicities. Therefore, the findings of this study may not be fully generalizable to other ethnic populations, given that the original GWAS primarily enrolled individuals of European descent.
In conclusion, by performing the two-sample MR analysis, we assessed the potential causality between gut microbiota, gut microbiota-derived metabolites, and CVD. Our findings could offer new perspectives on novel biomarkers for the targeted prevention and treatment of CVD. However, further randomized clinical trials and functional experimental studies are required to verify these findings and clarify the potential mechanism.
Data availability statement
The original contributions presented in the study are included in the article/Supplementary Material. Further inquiries can be directed to the corresponding author.
Author contributions
DL: Investigation, Writing – original draft. YZ: Writing – review & editing. ZT: Writing – review & editing. YT: Formal Analysis. CL: Formal Analysis. XP: Software, Visualization. JL: Software, Funding acquisition. XW: Writing – review & editing, Conceptualization, Funding acquisition.
Funding
The author(s) declare financial support was received for the research, authorship, and/or publication of this article. This work was supported by the National Natural Science Foundation (82360665), the Natural Science Foundation of Hunan Province (2022JJ40343), the Scientific Research Foundation of Hunan Provincial Education Department (21B0513), the Scientific Research Project of Hunan Provincial Health Commission (202212053368), Changsha Bureau of Science and Technology Plan Projects (kq2004171), the National Undergraduate Innovation Training Program Project (S202310531011), and the Scientific Research Project of Jishou University (Jdx22035).
Acknowledgments
We appreciate the genetics consortiums making the GWAS summary data accessible to the public and are grateful to the Bioinformatics Center, Xiangya Hospital, Central South University for partial support of this work.
Conflict of interest
The authors declare that the research was conducted in the absence of any commercial or financial relationships that could be construed as a potential conflict of interest.
Publisher’s note
All claims expressed in this article are solely those of the authors and do not necessarily represent those of their affiliated organizations, or those of the publisher, the editors and the reviewers. Any product that may be evaluated in this article, or claim that may be made by its manufacturer, is not guaranteed or endorsed by the publisher.
Supplementary material
The Supplementary Material for this article can be found online at: https://www.frontiersin.org/articles/10.3389/fcimb.2023.1269414/full#supplementary-material
References
Barakat, W., Fahmy, A., Askar, M., El-Kannishy, S. (2018). Effectiveness of arginase inhibitors against experimentally induced stroke. Naunyn Schmiedebergs Arch. Pharmacol. 391 (6), 603–612. doi: 10.1007/s00210-018-1489-1
Benjamini, Y., Hochberg, Y. (1995). Controlling the false discovery rate: A practical and powerful approach to multiple testing. J. R. Stat. Society: Ser. B (Methodological) 57 (1), 289–300. doi: 10.1111/j.2517-6161.1995.tb02031.x
Bowden, J., Davey Smith, G., Haycock, P. C., Burgess, S. (2016). Consistent estimation in mendelian randomization with some invalid instruments using a weighted median estimator. Genet. Epidemiol. 40 (4), 304–314. doi: 10.1002/gepi.21965
Burgess, S., Dudbridge, F., Thompson, S. G. (2016). Combining information on multiple instrumental variables in Mendelian randomization: comparison of allele score and summarized data methods. Stat. Med. 35 (11), 1880–1906. doi: 10.1002/sim.6835
Burgess, S., Thompson, S. G. (2015). Multivariable Mendelian randomization: the use of pleiotropic genetic variants to estimate causal effects. Am. J. Epidemiol. 181 (4), 251–260. doi: 10.1093/aje/kwu283
Carpenter, A. M., Singh, I. P., Gandhi, C. D., Prestigiacomo, C. J. (2016). Genetic risk factors for spontaneous intracerebral haemorrhage. Nat. Rev. Neurol. 12 (1), 40–49. doi: 10.1038/nrneurol.2015.226
Collaborators, G.B.D.C.o.D (2017). Global, regional, and national age-sex specific mortality for 264 causes of death 1980-2016: a systematic analysis for the Global Burden of Disease Study 2016. Lancet 390 (10100), 1151–1210. doi: 10.1016/S0140-6736(17)32152-9
Czekajlo, A. (2019). Role of diet-related factors in cerebral aneurysm formation and rupture. Rocz Panstw Zakl Hig. 70 (2), 119–126. doi: 10.32394/rpzh.2019.0061
DALYs, G.B.D., and Collaborators, H (2016). Global, regional, and national disability-adjusted life-years (DALYs) for 315 diseases and injuries and healthy life expectancy (HALE), 1990-2015: a systematic analysis for the Global Burden of Disease Study 2015. Lancet 388 (10053), 1603–1658. doi: 10.1016/S0140-6736(16)31460-X
Dekking, M. (2007). A Modern Introduction to Probability and Statistics: Understanding Why and How. Greenland, S. (2020) An introduction to instrumental variables for epidemiologists. Int J Epidemiol 29 (4), 722–729. doi: 10.1093/ije/29.4.722
Denes, A., Pradillo, J. M., Drake, C., Sharp, A., Warn, P., Murray, K. N., et al. (2014). Streptococcus pneumoniae worsens cerebral ischemia via interleukin 1 and platelet glycoprotein Ibalpha. Ann. Neurol. 75 (5), 670–683. doi: 10.1002/ana.24146
Feigin, V. L., Norrving, B., Mensah, G. A. (2017). Global burden of stroke. Circ. Res. 120 (3), 439–448. doi: 10.1161/CIRCRESAHA.116.308413
Franke, M., Bieber, M., Kraft, P., Weber, A. N. R., Stoll, G., Schuhmann, M. K. (2021). The NLRP3 inflammasome drives inflammation in ischemia/reperfusion injury after transient middle cerebral artery occlusion in mice. Brain Behav. Immun. 92, 223–233. doi: 10.1016/j.bbi.2020.12.009
Gallardo-Becerra, L., Cornejo-Granados, F., Garcia-Lopez, R., Valdez-Lara, A., Bikel, S., Canizales-Quinteros, S., et al. (2020). Metatranscriptomic analysis to define the Secrebiome, and 16S rRNA profiling of the gut microbiome in obesity and metabolic syndrome of Mexican children. Microb. Cell Fact 19 (1), 61. doi: 10.1186/s12934-020-01319-y
Greenland, S. (2000). An introduction to instrumental variables for epidemiologists. Int. J. Epidemiol. 29 (4), 722–729. doi: 10.1093/ije/29.4.722
Haak, B. W., Westendorp, W. F., van Engelen, T. S. R., Brands, X., Brouwer, M. C., Vermeij, J. D., et al. (2021). Disruptions of anaerobic gut bacteria are associated with stroke and post-stroke infection: a prospective case-control study. Transl. Stroke Res. 12 (4), 581–592. doi: 10.1007/s12975-020-00863-4
Hemani, G., Tilling, K., Davey Smith, G. (2017). Orienting the causal relationship between imprecisely measured traits using GWAS summary data. PloS Genet. 13 (11), e1007081. doi: 10.1371/journal.pgen.1007081
Hu, W., Kong, X., Wang, H., Li, Y., Luo, Y. (2022). Ischemic stroke and intestinal flora: an insight into brain-gut axis. Eur. J. Med. Res. 27 (1), 73. doi: 10.1186/s40001-022-00691-2
Huang, L., Wang, T., Wu, Q., Dong, X., Shen, F., Liu, D., et al. (2019). Analysis of microbiota in elderly patients with Acute Cerebral Infarction. PeerJ 7, e6928. doi: 10.7717/peerj.6928
Hughes, D. A., Bacigalupe, R., Wang, J., Ruhlemann, M. C., Tito, R. Y., Falony, G., et al. (2020). Genome-wide associations of human gut microbiome variation and implications for causal inference analyses. Nat. Microbiol. 5 (9), 1079–1087. doi: 10.1038/s41564-020-0743-8
Kurilshikov, A., Medina-Gomez, C., Bacigalupe, R., Radjabzadeh, D., Wang, J., Demirkan, A., et al. (2021). Large-scale association analyses identify host factors influencing human gut microbiome composition. Nat. Genet. 53 (2), 156–165. doi: 10.1038/s41588-020-00763-1
Kurki, M. I., Karjalainen, J., Palta, P., Sipilä, T. P., Kristiansson, K., Donner, K., et al. (2022). FinnGen: Unique genetic insights from combining isolated population and national health register data. medRxiv. doi: 10.1101/2022.03.03.22271360
Li, H., Zhang, X., Pan, D., Liu, Y., Yan, X., Tang, Y., et al. (2020). Dysbiosis characteristics of gut microbiota in cerebral infarction patients. Transl. Neurosci. 11 (1), 124–133. doi: 10.1515/tnsci-2020-0117
Ling, Y., Gong, T., Zhang, J., Gu, Q., Gao, X., Weng, X., et al. (2020a). Gut microbiome signatures are biomarkers for cognitive impairment in patients with ischemic stroke. Front. Aging Neurosci. 12. doi: 10.3389/fnagi.2020.511562
Ling, Y., Gu, Q., Zhang, J., Gong, T., Weng, X., Liu, J., et al. (2020b). Structural change of gut microbiota in patients with post-stroke comorbid cognitive impairment and depression and its correlation with clinical features. J. Alzheimers Dis. 77 (4), 1595–1608. doi: 10.3233/JAD-200315
Liu, Y., Kong, C., Gong, L., Zhang, X., Zhu, Y., Wang, H., et al. (2020). The association of post-stroke cognitive impairment and gut microbiota and its corresponding metabolites. J. Alzheimers Dis. 73 (4), 1455–1466. doi: 10.3233/JAD-191066
Liu, Z., Liu, H. Y., Zhou, H., Zhan, Q., Lai, W., Zeng, Q., et al. (2017). Moderate-intensity exercise affects gut microbiome composition and influences cardiac function in myocardial infarction mice. Front. Microbiol. 8. doi: 10.3389/fmicb.2017.01687
Malik, R., Chauhan, G., Traylor, M., Sargurupremraj, M., Okada, Y., Mishra, A., et al. (2018). Multiancestry genome-wide association study of 520,000 subjects identifies 32 loci associated with stroke and stroke subtypes. Nat. Genet. 50 (4), 524–537. doi: 10.1038/s41588-018-0058-3
Meng, C., Deng, P., Miao, R., Tang, H., Li, Y., Wang, J., et al. (2023). Gut microbiome and risk of ischemic stroke: a comprehensive mendelian randomization study. Eur. J. Prev. Cardiol. 30(7), 613–620. doi: 10.1093/eurjpc/zwad052
Mortality, G.B.D., and Causes of Death, C (2016). Global, regional, and national life expectancy, all-cause mortality, and cause-specific mortality for 249 causes of death 1980-2015: a systematic analysis for the Global Burden of Disease Study 2015. Lancet 388 (10053), 1459–1544. doi: 10.1016/S0140-6736(16)31012-1
Nagata, K., Seyama, Y., Shimizu, T. (1995). Changes in the level of 7 alpha-hydroxy-3-oxo-4-cholestenoic acid in cerebrospinal fluid after subarachnoid hemorrhage. Neurol. Med. Chir (Tokyo) 35 (5), 294–297. doi: 10.2176/nmc.35.294
Pagliai, G., Russo, E., Niccolai, E., Dinu, M., Di Pilato, V., Magrini, A., et al. (2020). Influence of a 3-month low-calorie Mediterranean diet compared to the vegetarian diet on human gut microbiota and SCFA: the CARDIVEG Study. Eur. J. Nutr. 59 (5), 2011–2024. doi: 10.1007/s00394-019-02050-0
Palmer, T. M., Lawlor, D. A., Harbord, R. M., Sheehan, N. A., Tobias, J. H., Timpson, N. J., et al. (2012). Using multiple genetic variants as instrumental variables for modifiable risk factors. Stat. Methods Med. Res. 21 (3), 223–242. doi: 10.1177/0962280210394459
Peh, A., O'Donnell, J. A., Broughton, B. R. S., Marques, F. Z. (2022). Gut microbiota and their metabolites in stroke: A double-edged sword. Stroke 53 (5), 1788–1801. doi: 10.1161/STROKEAHA.121.036800
Pezzini, A., Grassi, M., Paciaroni, M., Zini, A., Silvestrelli, G., Iacoviello, L., et al. (2013). Obesity and the risk of intracerebral hemorrhage: the multicenter study on cerebral hemorrhage in Italy. Stroke 44 (6), 1584–1589. doi: 10.1161/STROKEAHA.111.000069
Pierce, B. L., Burgess, S. (2013). Efficient design for Mendelian randomization studies: subsample and 2-sample instrumental variable estimators. Am. J. Epidemiol. 178 (7), 1177–1184. doi: 10.1093/aje/kwt084
Pinart, M., Dötsch, A., Schlicht, K., Laudes, M., Bouwman, J., Forslund, S. K., et al. (2021). Gut microbiome composition in obese and non-obese persons: A systematic review and meta-analysis. Nutrients 14 (1), 12. doi: 10.3390/nu14010012
Quast, C., Pruesse, E., Yilmaz, P., Gerken, J., Schweer, T., Yarza, P., et al. (2013). The SILVA ribosomal RNA gene database project: improved data processing and web-based tools. Nucleic Acids Res. 41 (Database issue), D590–D596. doi: 10.1093/nar/gks1219
Sanna, S., van Zuydam, N. R., Mahajan, A., Kurilshikov, A., Vich Vila, A., Vosa, U., et al. (2019). Causal relationships among the gut microbiome, short-chain fatty acids and metabolic diseases. Nat. Genet. 51 (4), 600–605. doi: 10.1038/s41588-019-0350-x
Schiattarella, G. G., Sannino, A., Toscano, E., Giugliano, G., Gargiulo, G., Franzone, A., et al. (2017). Gut microbe-generated metabolite trimethylamine-N-oxide as cardiovascular risk biomarker: a systematic review and dose-response meta-analysis. Eur. Heart J. 38 (39), 2948–2956. doi: 10.1093/eurheartj/ehx342
Schroder, K., Tschopp, J. (2010). The inflammasomes. Cell 140 (6), 821–832. doi: 10.1016/j.cell.2010.01.040
Shin, S. Y., Fauman, E. B., Petersen, A. K., Krumsiek, J., Santos, R., Huang, J., et al. (2014). An atlas of genetic influences on human blood metabolites. Nat. Genet. 46 (6), 543–550. doi: 10.1038/ng.2982
Singh, V., Roth, S., Llovera, G., Sadler, R., Garzetti, D., Stecher, B., et al. (2016). Microbiota dysbiosis controls the neuroinflammatory response after stroke. J. Neurosci. 36 (28), 7428–7440. doi: 10.1523/JNEUROSCI.1114-16.2016
Staiger, D., Stock, J. H. (1997). Instrumental variables regression with weak instruments. Econometrica 65 (3), 557–586. doi: 10.2307/2171753
Storey, J. D., Tibshirani, R. (2003). Statistical significance for genomewide studies. Proc. Natl. Acad. Sci. U.S.A. 100 (16), 9440–9445. doi: 10.1073/pnas.1530509100
Szumilas, M. (2010). Explaining odds ratios. J. Can. Acad. Child Adolesc. Psychiatry 19 (3), 227–229.
Thompson, J. R., Minelli, C., Abrams, K. R., Tobin, M. D., Riley, R. D. (2005). Meta-analysis of genetic studies using Mendelian randomization–a multivariate approach. Stat. Med. 24 (14), 2241–2254. doi: 10.1002/sim.2100
Wang, W., Li, X., Yao, X., Cheng, X., Zhu, Y. (2018). The characteristics analysis of intestinal microecology on cerebral infarction patients and its correlation with apolipoprotein E. Med. (Baltimore) 97 (41), e12805. doi: 10.1097/MD.0000000000012805
Wishart, D. S., Feunang, Y. D., Marcu, A., Guo, A. C., Liang, K., Vazquez-Fresno, R., et al. (2018). HMDB 4.0: the human metabolome database for 2018. Nucleic Acids Res. 46 (D1), D608–D617. doi: 10.1093/nar/gkx1089
World Health Organization (2021) Cardiovascular diseases (CVDs) fact sheets. Available at: https://www.who.int/news-room/fact-sheets/detail/cardiovascular-diseases-(cvds).
Xu, F., Fu, Y., Sun, T. Y., Jiang, Z., Miao, Z., Shuai, M., et al. (2020). The interplay between host genetics and the gut microbiome reveals common and distinct microbiome features for complex human diseases. Microbiome 8 (1), 145. doi: 10.1186/s40168-020-00923-9
Yin, J., Liao, S. X., He, Y., Wang, S., Xia, G. H., Liu, F. T., et al. (2015). Dysbiosis of gut microbiota with reduced trimethylamine-N-oxide level in patients with large-artery atherosclerotic stroke or transient ischemic attack. J. Am. Heart Assoc. 4 (11), e002699. doi: 10.1161/JAHA.115.002699
Zeng, X., Gao, X., Peng, Y., Wu, Q., Zhu, J., Tan, C., et al. (2019). Higher risk of stroke is correlated with increased opportunistic pathogen load and reduced levels of butyrate-producing bacteria in the gut. Front. Cell Infect. Microbiol. 9. doi: 10.3389/fcimb.2019.00004
Zhao, Q., Wang, J., Hemani, G., Bowden, J., Small, D. S. (2020). Statistical inference in two-sample summary-data Mendelian randomization using robust adjusted profile score. Ann. Stat 48 (3), 1742–1769. doi: 10.1214/19-aos1866
Keywords: Mendelian randomization, gut microbiota, cerebrovascular diseases, gut microbiota-derived metabolites, causal association
Citation: Lin D, Zhu Y, Tian Z, Tian Y, Liang C, Peng X, Li J and Wu X (2023) Causal associations between gut microbiota, gut microbiota-derived metabolites, and cerebrovascular diseases: a multivariable Mendelian randomization study. Front. Cell. Infect. Microbiol. 13:1269414. doi: 10.3389/fcimb.2023.1269414
Received: 30 July 2023; Accepted: 17 October 2023;
Published: 08 November 2023.
Edited by:
Prabu Paramasivam, University of New Mexico, United StatesReviewed by:
Gautam Pandey, University of North Carolina at Chapel Hill, United StatesKamakshi Mehta, University of Pittsburgh, United States
Copyright © 2023 Lin, Zhu, Tian, Tian, Liang, Peng, Li and Wu. This is an open-access article distributed under the terms of the Creative Commons Attribution License (CC BY). The use, distribution or reproduction in other forums is permitted, provided the original author(s) and the copyright owner(s) are credited and that the original publication in this journal is cited, in accordance with accepted academic practice. No use, distribution or reproduction is permitted which does not comply with these terms.
*Correspondence: Xinrui Wu, wuxinrui99@qq.com