Association Between Serum Thyroid-Stimulating Hormone Levels and Salivary Microbiome Shifts
- 1Department of Endodontics, Shanghai Ninth People’s Hospital, College of Stomatology, Shanghai Jiao Tong University, School of Medicine, Shanghai, China
- 2National Clinical Research Center for Oral Diseases, Shanghai, China
- 3Shanghai Key Laboratory of Stomatology, Shanghai, China
- 4Shanghai Research Institute of Stomatology, Shanghai, China
- 5Institute and Department of Endocrinology and Metabolism, Shanghai Ninth People’s Hospital, Shanghai Jiao Tong University School of Medicine, Shanghai, China
High serum thyroid-stimulating hormone (TSH) levels are linked to many metabolic disorders, but the effects of TSH levels on the oral microbiota are still largely unknown. This study aimed to explore the association between the salivary microbiome in adults and serum TSH levels. Saliva and fasting blood samples were obtained from a health census conducted in Southeast China. All participants were divided according to serum TSH levels. The microbial genetic profiles and changes were acquired by 16S rDNA sequencing and bioinformatics analysis. Relevant anthropometric and biochemical measurements such as insulin resistance, blood lipids, and body composition were evaluated with laboratory tests and physical examinations. The salivary microbiome in individuals with higher TSH level showed significantly higher taxa diversity. Principal coordinates analysis and partial least squares discriminant analysis showed distinct clustering in the Abnormal and Normal Groups (Adonis, P=0.0320). Granulicatella was identified as a discriminative genus for comparison of the two groups. Fasting serum insulin, Homeostatic Model Assessment for Insulin Resistance, and hemoglobin A1 were elevated in the Abnormal Group (P<0.05), showing the presence of insulin resistance in individuals with abnormal higher serum TSH levels. Distance-based redundancy analysis revealed the association of this distinctive difference with salivary microbiome. In conclusion, shifts in microbial profile were observed in the saliva of individuals with different serum TSH levels, and insulin resistance may play an important role in the biochemical and microbial alteration.
Introduction
The oral microbiome is a collection of microorganisms that colonize the human oral cavity and constitute an important microenvironment (Zhang et al., 2015). The oral microbiome harbors 50–100 billion bacteria in the oral cavity, and it has attracted increased attention by researchers and clinicians for its accessibility compared with microbiomes at other sites (Krishnan et al., 2017). Located at the beginning of the digestive tract, the oral microbiome plays a critical role in regulating nutrient absorption, substance metabolism and immune responses (Gao et al., 2018). The oral microbiome is dependent on the environment, and it is subject to changes in the environment.
Shifts in the oral microbiome are observed in some diseases or when the environment changes. Extensive studies have shown major changes in bacteria in most oral diseases, such as dental caries and periodontitis. Changes are also commonly seen in various systemic disorders, such as gastrointestinal, nervous, endocrine, immune, and cardiovascular diseases (Si et al., 2017). The oral microbiome is also related to various forms of metabolic dysbiosis, such as diabetes, hyperglycemia, and obesity (Saeb et al., 2019; De Andrade et al., 2020; Wei et al., 2020). Diabetes is reported to cause a shift in the oral microbiome with 16S rRNA sequencing and the reason for this shift may be enhanced expression of interleukin-17 in diabetes (Xiao et al., 2017). Our previous work has detected decreased richness and diversity of the oral microbiome during and after radiotherapy (Gao et al., 2015), and major shifts in supragingival microbiota during pregnancy (Lin et al., 2018).
Thyroid-stimulating hormone (TSH) is secreted by the adenohypophysis. TSH plays a critical regulatory role in the maintenance of normal thyroid function and is regulated by the hypothalamic–adenohypophysis–thyroid axis (Kopp, 2001). Thyroid hormone (TH) plays a significant role in maintaining metabolic homeostasis throughout life and is involved in the regulation of body composition, lipid metabolism, and insulin resistance (Asvold et al., 2013; Mullur et al., 2014; Martinez and Ortiz, 2017). The proportion of individuals with increased TSH level in the general population has increased in recent years. Hypothyroidism, subclinical hypothyroidism and thyroid hormone resistance syndrome are becoming common diseases in the general population (Lefevre, 2015). Elevated serum TSH level may cause organ damage and metabolic disorder (Ruhla et al., 2010). Various changes in physiological indicators and metabolic levels are associated with high TSH levels. Lipid metabolism [including total cholesterol (TC), triglyceride (TG), and low-density lipoprotein cholesterol (LDL-C)] deteriorates with increasing TSH level (Teixeira Pde et al., 2008; Ren et al., 2019). High TSH level is also associated with other cardiovascular risk factors, such as alteration in blood pressure and increased atherosclerosis (Delitala et al., 2017; Ren et al., 2019). Increasing TSH level is linked with insulin resistance and type 2 diabetes (Bos et al., 2017; Jun et al., 2017; Laclaustra et al., 2019).
The oral microbiome is affected by physiological processes and changes in the environment, while the microenvironment and various metabolic processes are disturbed by increased levels of TSH, which is assumed to cause shifts in the oral microbiome. However, there is limited information on the correlation between changes in the oral microbiome and levels of serum TSH. In this study, saliva samples were obtained from volunteers who were divided into Normal and Abnormal Groups according to their serum TSH levels. Illumina MiSeq PE300 sequencing and bioinformatics analysis were used to determine the shifts in salivary microbial profiles with different serum TSH levels. These findings may provide a deeper understanding of the oral ecological dysbiosis associated with high TSH levels.
Materials and Methods
Participant Recruitment
Participants aged 45–60 years were recruited from a health census conducted in Southwest China. The inclusion criteria were: (1) Han race; (2) residence in the same community for >5 years; (3) normal measured THs; and (4) no iodine contrast agent examination or amiodarone intake in the past 3 months. The exclusion criteria were: (1) type 1 or 2 diabetes mellitus; (2) symptomatic liver and cardiovascular diseases; (3) cancer or cancer history; (4) other chronic inflammatory disease; (5) oral diseases: untreated oral abscess, precancerous lesions, and oral cancer, oral fungal infection, missing more than eight teeth, etc.; (6) long-term heavy smoking and alcohol consumption; (7) taking antibiotics for >5 days in the last 6 months; and (8) pregnant or lactating women. This study followed the Declaration of Helsinki on medical protocols and ethics and obtained approval from the regional Ethical Review Board of Shanghai 9th Hospital affiliated to Shanghai Jiaotong University, School of Medicine (2019-T295-1). Written informed consent was obtained from all participants.
Anthropometric and Biochemical Measurements
All of the participants were required to fast overnight before physical examination. The anthropometric parameters included height, body mass index (BMI), waist circumference (WC), hip circumference (HC), and blood pressure. Questionnaires and fasting blood samples were collected for demographic information and biochemical measurements, respectively. The clinical variables included: TSH, triiodothyronine (T3), tetraiodothyronine (T4), TC, TG, LDL-C, high-density lipoprotein cholesterol (HDL-C), uric acid (UA), fasting blood glucose (FBG), fasting serum insulin (FSI), and hemoglobin A1C (HbA1C). Homeostatic Model Assessment for Insulin Resistance (HOMA-IR) was calculated according to the formula: HOMA-IR = FBG (mmol/L) × FSI (mU/L)/22.5 (Bos et al., 2017).
Group Definition
We recruited 114 participants. Those with serum TSH level >4.2 mIU/L were defined as the Abnormal Group (n=20, 4.20–9.22 mIU/L). The Normal Group (n=20, 0.6–4.20 mIU/L) was screened from participants with serum TSH level no more than 4.2 mIU/L, using MedCalc Statistical Software version 19.0.7 (MedCalc Software, Ostend, Belgium) with case–control matching. The variables were set to an exact match between the sexes and a maximum allowable age difference of 3. All participants included possessed normal T3 and T4. The reference ranges were 0.27–4.20 mIU/L for TSH, 1.30–3.10 nmol/L for T3, and 66.0–181.0 nmol/L for T4 as provided by the laboratory.
Saliva Sample Collection
Unstimulated saliva samples were collected between 08:00 and 10:00 h before eating in the morning, as described in the Manual of Procedures for the Human Microbiome Project (https://www.hmpdacc.org/hmp/doc/HMP_MOP_Version12_0_072910.pdf). Participants were asked to refrain from drinking, smoking, or oral hygiene procedures for at least 1 h before saliva collection. Saliva samples were collected in test tubes and stored in liquid nitrogen at minus 196 degrees immediately for 5 days until sequencing.
DNA Extraction, Amplification, and High-Throughput Sequences
Total bacterial DNA was extracted using the QIAamp DNA Mini Kit (Qiagen, Valencia, CA, USA) and then quantified using NanoDrop 2000 UV-vis spectrophotometer (Thermo Scientific, Wilmington, DE, USA) and 1% agarose gel electrophoresis. The V3–V4 region of 16S rRNA genes was amplified using the following primers: 338F forward primer (5′-ACTCCTACGGGAGGCAGCAG-3′) and 806R reverse primer (5′-GGACTACHVGGGTWTCTAAT-3′) by thermocycler PCR system (GeneAmp 9700; Applied Biosystems, Carlsbad, CA, USA) as described previously (Lin et al., 2018). The purified amplicons were sequenced using the Illumina Miseq PE300 platform (Illumina, San Diego, CA, USA) according to the standard protocol.
Data Processing and Bioinformatics Analyses
All anthropometric and biochemical comparisons were made between the Abnormal and Normal Groups using unpaired t-test, except for “Gender”, which was analyzed by χ2 test. Operational taxonomic units (OTUs) were clustered with 97% similarity using UPARSE (version 7.1, http://drive5.com/uparse/) and matched to a database (SILVA 106; https://www.arb-silva.de/) for taxonomic analysis. A modified OTUs table was generated for subsequent analysis after subsampling according to the minimum number of sample sequence. Alpha diversity indexes (Ace, Chao, Shannon and Simpson) and co-occurrence networks of the 50 most abundant genera of each group were calculated to evaluate the diversity and construction of community species using MOTHUR (version 1.30.2, https://www.mothur.org/wiki/Download_mothur). Community barplot analysis and heatmaps on genus level were created via R platform (version 3.6.1) to describe the species composition in different groups. The weighted UniFrac principal coordinates analysis (PCoA) (Lozupone et al., 2007) and partial least squares discriminant analysis (PLS-DA) (Lee et al., 2018) were performed to evaluate the variances of sample community composition using QIIME. The linear discriminant analysis (LDA) effect size analysis (LEfSe, http://huttenhower.sph.harvard.edu/galaxy) was applied to identify the most discriminatory taxa among groups from phylum to genus level with logarithmic LDA score >2.0 regarded as discriminative species (Segata et al., 2011). The unweighted Unifrac distance-based redundancy analysis (db-RDA) and Spearman’s rank correlation coefficient were operated to quantitatively evaluate the multicollinearity relationship between environmental/clinical factors and sample species composition (Roberts, 2009). Phylogenetic Investigation of Communities by Reconstruction of Unobserved States (PICRUSt2, version 1.1.0, http://picrust.github.io/picrust/) analysis and Wilcoxon rank sum test with a Benjamini–Hochberg false discovery rate (FDR) correction to adjust P values for multiple testing were performed to predict and compare the abundance of Kyoto Encyclopedia of Genes and Genomes (KEGG) pathways in different groups.
Data Access
All the raw sequences were deposited in the NCBI Sequence Read Archive database. The accession number is SRP273570.
Results
Participant Characteristics
There were no differences in sex and age between the Abnormal and Normal Groups, based on case–control matching. The anthropometric and biochemical variables of the two groups were summarized in Table 1. FSI, HOMA-IR, and HbA1C were significantly elevated in the Abnormal Group (P<0.05), demonstrating the presence of insulin resistance in participants with abnormally higher serum TSH levels. TG, which reflected lipid metabolism status and was affected by thyroid function, was significantly higher in the Abnormal compared with Normal Group (P<0.05) and TC was lower in the Abnormal Group.
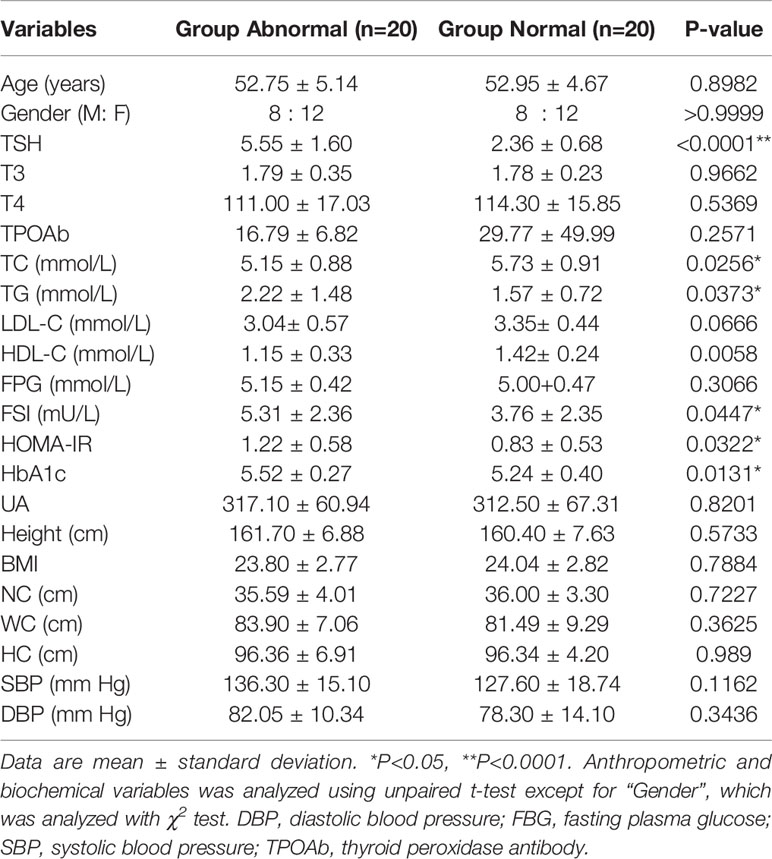
Table 1 Anthropometric and biochemical variables of the participants with normal/abnormal serum thyroid-stimulating hormone (TSH) levels.
Community Structure and Species Composition of Oral Microbiome
A total of 1,216,520 high-quality sequences were produced, and 21 phyla, 38 classes, 71 orders, 119 families, 258 genera, 514 species, and 978 OTUs were identified for the Abnormal and Normal Groups. The microbial community from the Abnormal Group indicated significantly greater community richness than the Normal Group (P<0.05, Figure 1A), but a smaller Simpson Index at the OUT level (Figure 1B). The samples from the Abnormal and Normal Groups shared a similar core microbiome (Gao et al., 2015). The top phyla of both groups (including Firmicutes, Bacteroidetes, Proteobacteria, Actinobacteria, Fusobacteria, Saccharibacteria, SR1 Absconditabateria, Spirochaetes, and Gracilibacteria) accounted for >99% of the total phyla found in the salivary samples of the Abnormal and Normal Groups and exhibited analogous community species composition (Figure 1E). The top 50 abundant genera in each sample are displayed in the heatmap (Figure 2). There was an obvious tendency for separation in the PC1 axis in PCoA and COMP 1 axis in PLS-DA, indicating the differences in sample community composition in the Abnormal and Normal Groups (Figures 1C, D). The co-occurrence network of the top 50 abundant genera in the salivary microbiome exhibited slightly different community structures, but both mainly consisted of Bacterioidetes and Firmicutes (Figure 3).
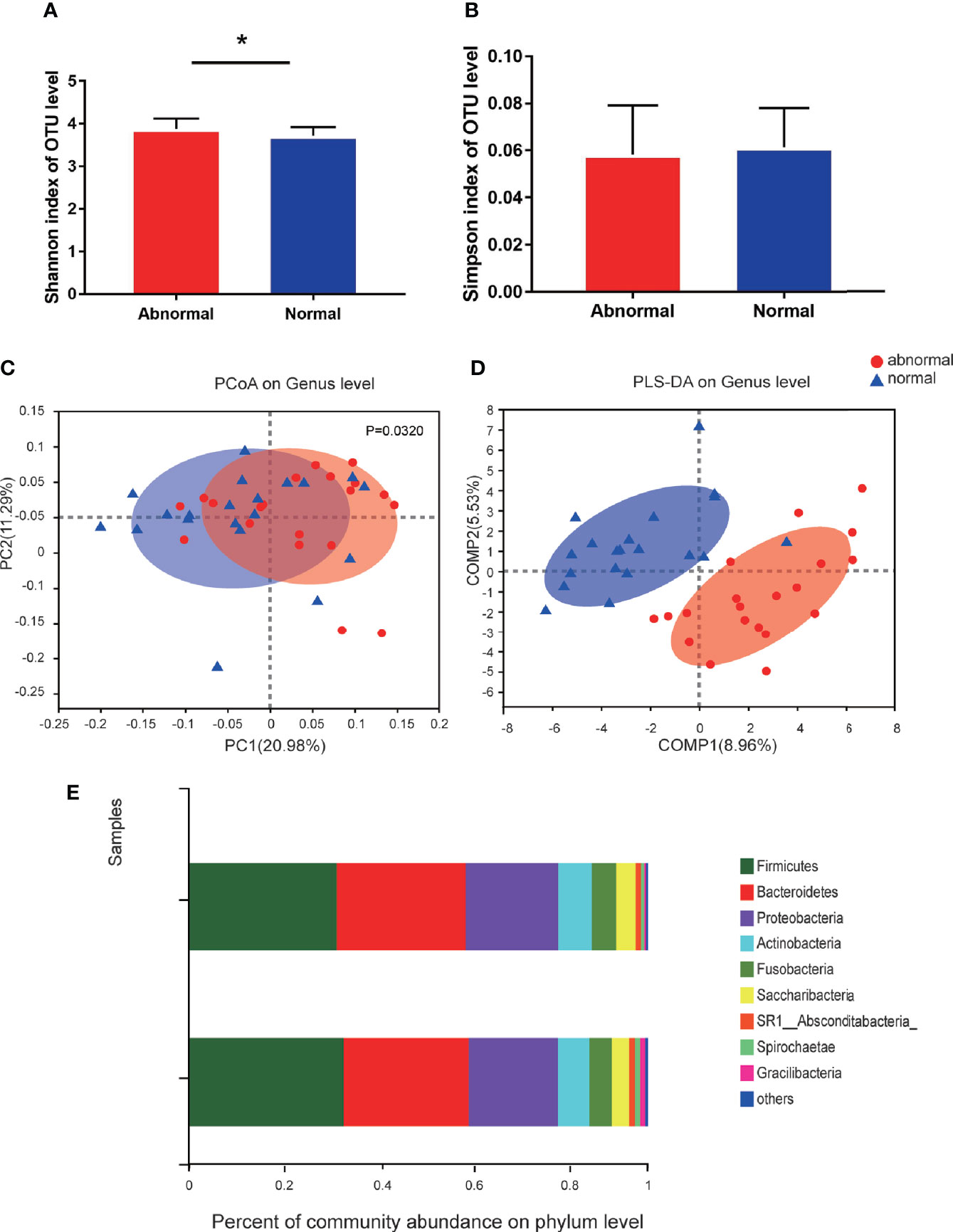
Figure 1 Diversity and species composition of salivary microbiome in the Abnormal and Normal Groups. (A) Chao Index of operational taxonomic unit (OTU) level, *P<0.05. (B) Simpson index of OTU level. (C) Weighted UniFrac PCoA of genus level, P<0.05. (D) partial least squares discriminant analysis (PLS-DA) of genus level showed more obvious separation in the horizontal coordinate axis. (E) Community bar plot analysis showing the top 10 phyla with highest abundance.
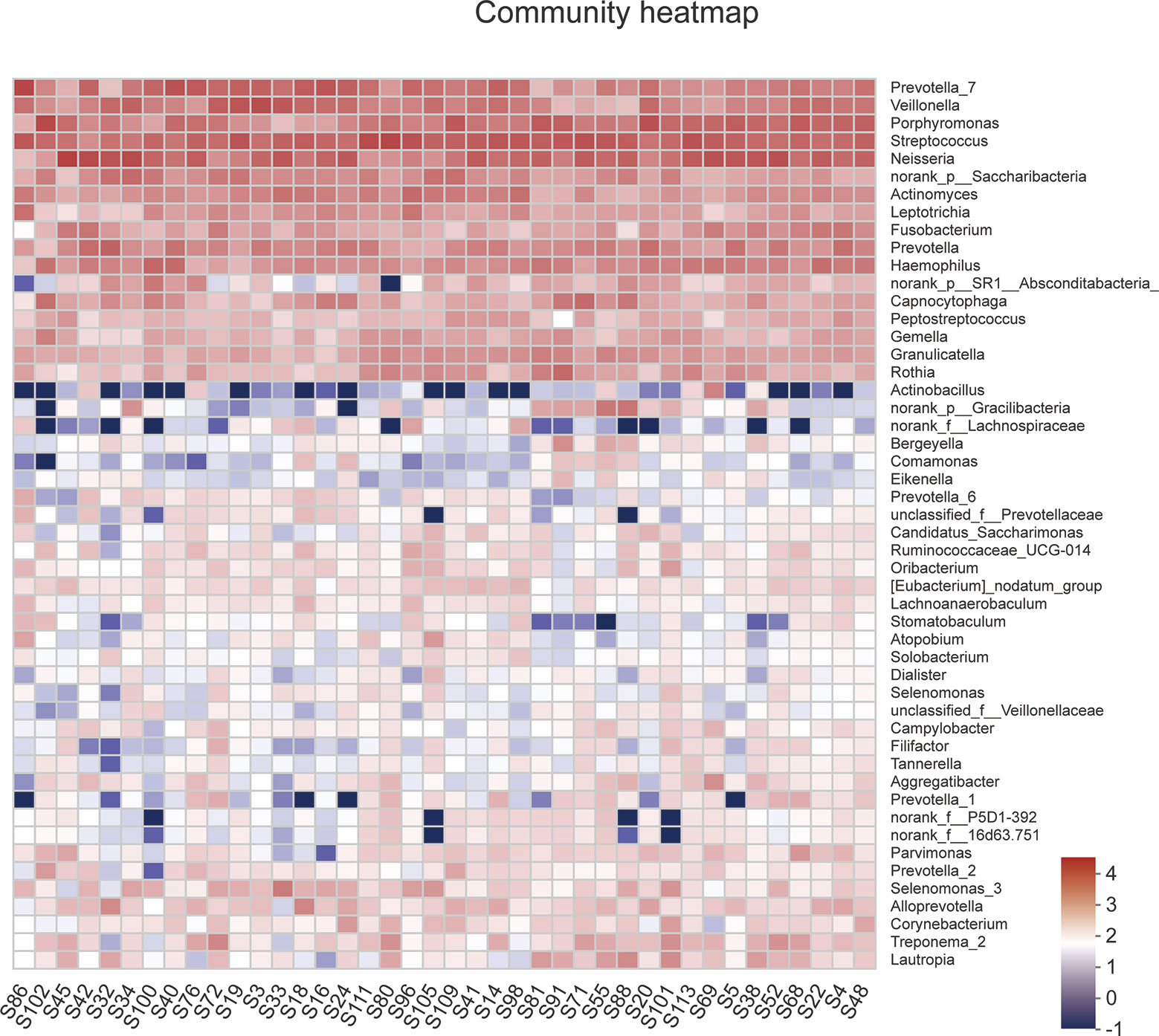
Figure 2 A heatmap of the abundance of top 50 abundant genera in each sample. The right side of the legend shows the color range of different R values. The color gradient of the color block was used to show the variation in abundance of different species in the sample.
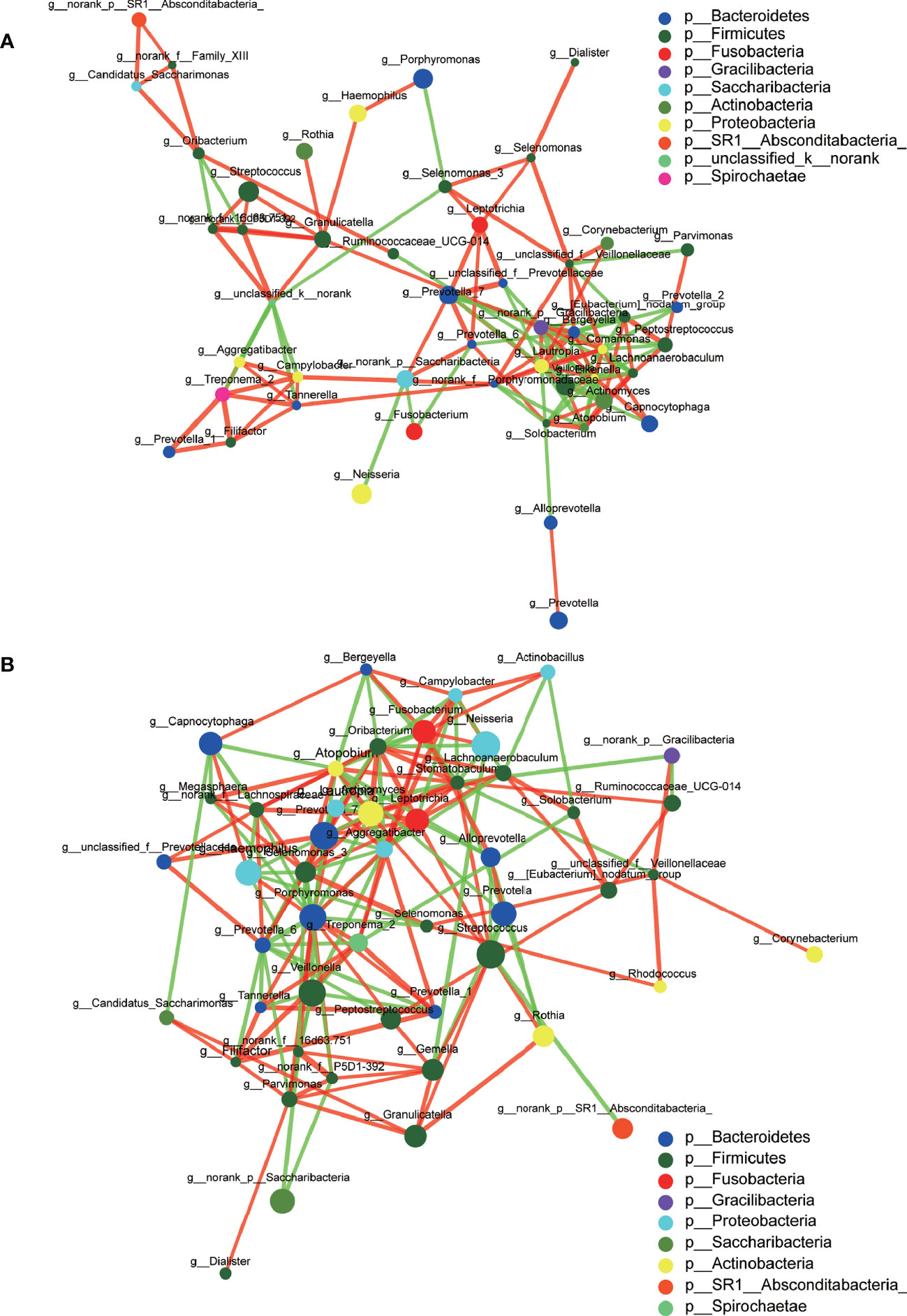
Figure 3 Co-occurrence networks of the top 50 abundant genera in the Abnormal (A) and Normal (B) Groups. The size of the node indicates the mean relative abundance of the corresponding genus. The same color represents the genera belonging to the same phylum. The thickness of connecting lines corresponds to the coefficient values. The red and green lines indicate a positive and negative correlation, respectively.
Alterations of the Salivary Microbial Phylotypes Associated With Serum TSH Levels
The significance level of the difference in species abundance was evaluated with Wilcoxon rank-sum test according to the obtained community abundance data, and species with significant variances between groups were obtained at the genus level. Granulicatella was found to possess significantly higher abundance in the Abnormal Group compared with the Normal Group (Figure 4A). The most discriminatory taxa between groups from phylum to genus level were further identified using LEFSe with logarithmic LDA score >2.0. Forty-five taxa showed differential distribution in the two groups. Lactobacillales at the order level, Porphyromonadaceae, Carnobacteriaceae, and Spirochaetae at the family level, and Granulicatella, Treponema, and Streptobacillus at the genus level were more prevalent in the Abnormal Group, while Rhodococus and Nocardiaceae were more abundant in the Normal Group (Figure 4B).
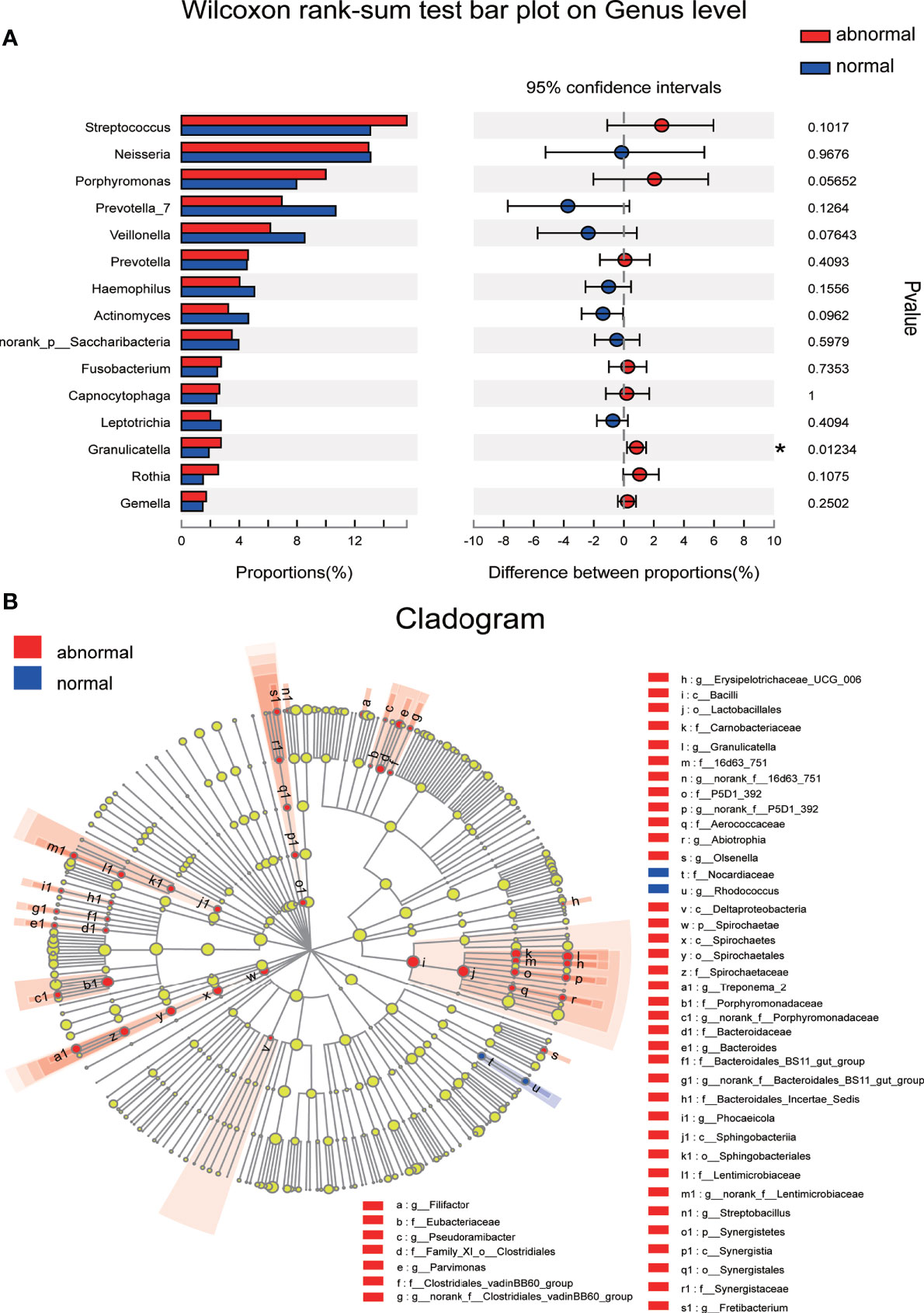
Figure 4 Discriminative species with maximum abundance difference in the Abnormal and Normal Groups. (A) Discriminative species at genus level were identified using Wilcoxon rank-sum test, *P < 0.05. (B) A cladogram for taxonomic representation based on LEfSe. Red indicates enrichment in samples from the Abnormal Group, and blue indicates the taxa enriched in samples from the Normal Group (LDA>2.0, P<0.05).
Associations Between Clinical Variables and Salivary Microbiome
Unweighted Unifrac db-RDA was performed to analyze the relation between the microbial community and clinical variables. The degree of explanation of obesity-related [BMI, neck circumference (NC), WC and HC] and glucose-metabolism-related (HOMA-IR, HbA1C, and FSI) variables occupied the main part (Figure S2). HOMA-IR contributed most to the degree of explanation with r2 = 0.3165, which had significance, followed by FSI (r2 = 0.2844), BMI (r2 = 0.2282), WC (r2 = 0.1889), NC (r2 = 0.1589), and HC (r2 = 0.1538). Spearman correlation coefficient of the top 50 abundant genera and clinical variables was visualized using a heatmap (Figure 5). R value is shown in different colors; red indicates positive correlation and blue negative correlation.
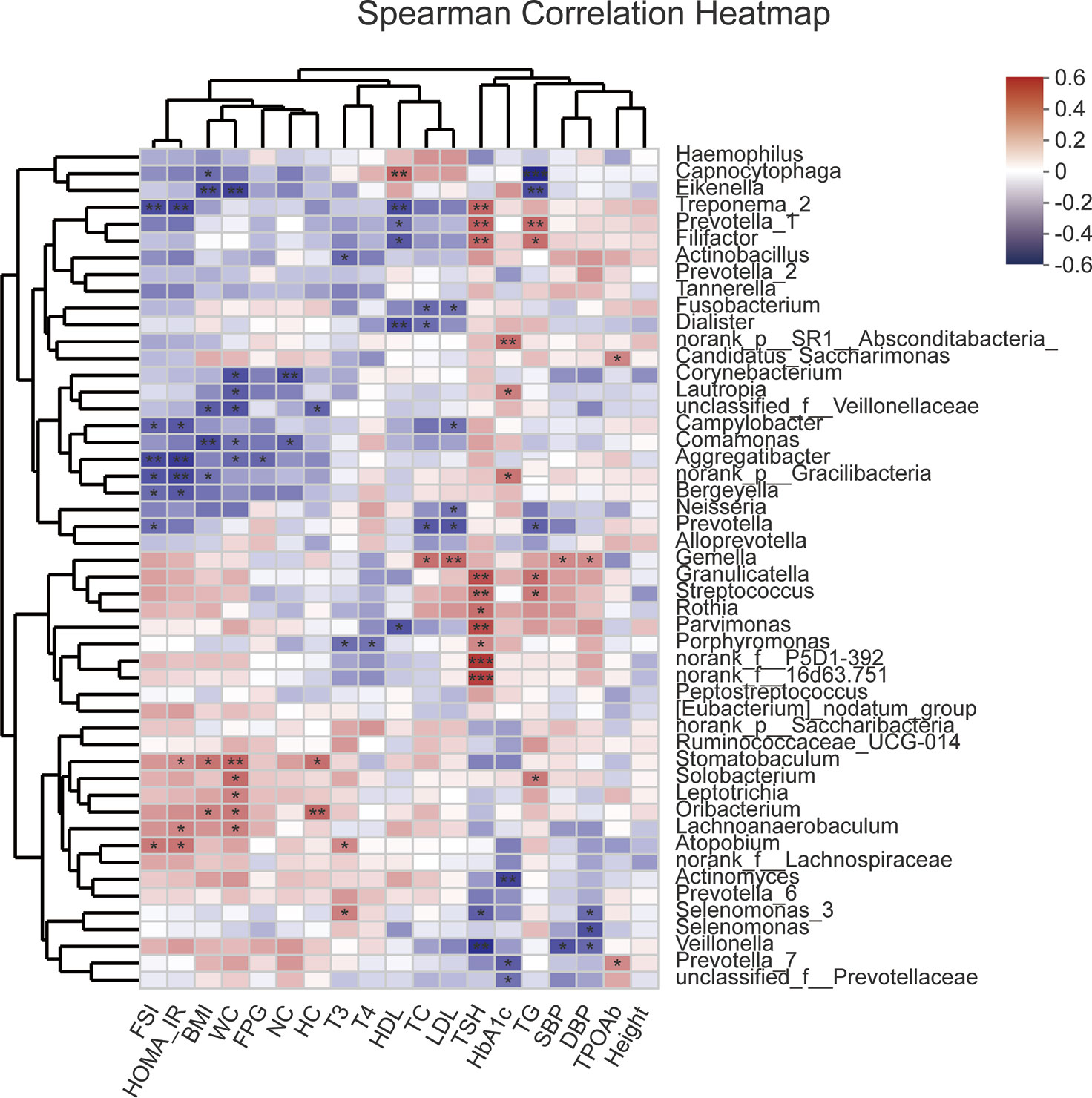
Figure 5 Heatmap of Spearman correlation analysis between the top 50 abundant salivary microbiota and clinical variables. R value shows in different colors, red indicates positive correlation while blue indicates negative correlation. The darker the color, the greater the correlation coefficient. Species clustering trees were presented in the left side of the heat map. *P<0.05; **P<0.01; ***P<0.001.
Alterative Predictive Metagenome Functional Profiling Along With Serum TSH Levels
The functional profile of the salivary microbiome along with changes in serum TSH levels was further explored using PICRUSt 2 based on high-quality amplicon sequencing. Wilcoxon rank sum test with a Benjamini–Hochberg FDR correction to adjust P values for multiple testing was used for detection. Carbohydrate metabolism accounted for the highest proportion of the functional composition of the microbial community in the saliva samples at KEGG level 2 (Figure S3A). The top 10 functional components of abundance were mainly concerned with carbohydrate, nucleotide, amino acid and energy metabolism and material transport at KEGG levels 2 and 3 (Figure S3A, B), but no significant difference was found between the two groups after multiple testing.
Discussion
The salivary microbiome comprises a mixture of microorganisms from all oral sites and is more accessible than the microbiome of other sites, which makes saliva an easy and non-invasive alternative to other sampling options (Belstrøm, 2020). The salivary microbiome is shaped by the surrounding environment to some extent, and any fluctuation in that environment may cause variation in the microbiome. Shifts in the salivary microbiome have been reported in many oral and non-oral diseases (Said et al., 2014; Wu et al., 2018; Yang J. et al., 2018). Disturbances in hormone and metabolic levels often lead to changes or even dysbiosis in the oral microbiome. Sex hormones have been confirmed to exert a critical influence on the oral microbiome, and fluctuations in sex hormones can change the composition and structure of oral microbiota, with puberty and pregnancy being most typical (Kumar, 2013). In our previous study that focused on the shifts in oral microbiota associated with pregnancy, 53 OTUs were observed to have positive correlations with sex hormones, especially Prevotella spp. and Treponema spp. (Lin et al., 2018). Several studies have reported an association of the salivary microbiome with diabetes and detected decreased bacterial diversity in patients with diabetes (Sabharwal et al., 2019; Saeb et al., 2019).
An elevated serum TSH level may cause various changes in physiological indicators and metabolic levels (Mullur et al., 2014; Martinez and Ortiz, 2017). Therefore, it is easy to imagine that the salivary microbiome changes with increasing TSH levels and accompanying physiological changes. Elevated microbial abundance reflected by greater Ace and Chao Index was observed in the Abnormal Group, which may indicate changes in the microenvironment and the original microbiota. Microbial diversity is generally considered to be tightly associated with the health status of the host. Increased diversity of oral microbiota is often reported in individuals with poor oral conditions (caries, periodontitis, or mucosal diseases) (Pozhitkov et al., 2015; Takeshita et al., 2016) and several metabolic diseases (Si et al., 2017; De Andrade et al., 2020).
The discrepancy in the community structure of the salivary microbiome between the Abnormal and Normal Groups indicated greater microbial shifts once the serum TSH levels exceed the upper limit of normal. Granulicatella was distinguished as the discriminative genus between the groups using LEfSe and Wilcoxon rank-sum test. Granulicatella is normally associated with health (Sanz et al., 2017). It is considered as a suspicious periodontal disease pathogen, and 16S sequencing technology has confirmed its high detection rate in periodontitis (Lourenço et al., 2014). Decreased abundance of Granulicatella has been linked with asthma (Wang et al., 2018), and significantly greater abundance can be detected in many systemic disorders such as obesity (Wu et al., 2018), infective endocarditis (Téllez et al., 2018) and pancreatic cancer (Gaiser et al., 2019). A comprehensive analysis of the oral microbiome found that Granulicatella and Neisseria were highly enriched in patients with metabolic syndrome (Si et al., 2017). Porphyromonas, Parvimonas, and Streptococcus are also significantly correlated with abnormal TSH levels. Porphyromonas is a key pathogen in periodontitis and is closely related to many systemic diseases. A number of studies have explored the role of Porphyromonas in atherosclerosis (Giles et al., 2020), Alzheimer’s disease (Miller and Scott, 2020), rheumatoid arthritis (Cheng et al., 2020), diabetes (Minty et al., 2019), and adverse pregnancy outcomes (Chopra et al., 2020) and nonalcoholic fatty liver disease (Clos-Garcia et al., 2020; Wang et al., 2020; Zhou et al., 2020). Parvimonas have been reported to be a potential carcinogenic pathogen, which is positively associated with oral squamous cell carcinoma (OSCC) (Zhou et al., 2020), colorectal cancer (Clos-Garcia et al., 2020), and gastric cancer (Gantuya et al., 2020). Streptococcus is one of the most common bacteria in the oral cavity, and is predominant in the saliva of patients with liver diseases (Li et al., 2020) and is associated with OSCC (Yang C. Y. et al., 2018). A significantly higher relative abundance of Streptococcus has been observed in type 1 diabetes (Leiva-Gea et al., 2018) and nonalcoholic fatty liver disease (Ponziani et al., 2019).
We used co-occurrence networks of the Top 50 abundant genera to predict inter-genera correlations of saliva in the two groups, which exhibited different community structures, but both mainly consisted of Bacterioidetes and Firmicutes. The degree of interconnectedness among the genera in the Normal Group was higher than that in the Abnormal Group. This indicates that the increase in TSH level was related to the decrease in species richness, as well as the decrease in the interaction among these bacteria. In contrast, the genera within the Abnormal Group formed smaller clusters with fewer interconnections, suggesting an unknown antagonistic or mutually exclusive relationship, which is in accordance with previous research (Qiao et al., 2018).
TH is necessary for normal development as well as regulating metabolism in humans. It modulates hepatic insulin sensitivity, which is especially important for the suppression of hepatic gluconeogenesis (Mullur et al., 2014). Thyroid disease and diabetes mellitus are the two major endocrine disorders that are treated concurrently nowadays (Waring et al., 2012), so it is not surprising that TH and insulin signaling are related to each other. In the present study, several biochemical variables were demonstrated to differ significantly once serum TSH levels were beyond the normal range, which were mainly clinical indicators of glucose metabolism (including FSI, HOMA-IR, and HbA1C) and lipid metabolism (TC and TG). Insulin resistance is a metabolic disorder that affects many insulin-regulated pathways, and it is characterized by reduced action of insulin, usually described with HOMA-IR (Artunc et al., 2016). Several studies have reported that elevation in TSH levels is correlated with insulin resistance (Vyakaranam et al., 2014; Ujwal Upadya et al., 2015). Our study suggested the presence of insulin resistance in individuals with abnormally higher serum TSH levels, which is consistent with previous reports (Kouidhi et al., 2013; Vyakaranam et al., 2014; Ujwal Upadya et al., 2015). Higher TG and lower HDL-C were detected in the Abnormal Group, supporting the presence of insulin resistance with comparatively higher TG/HDL-C ratio (Uruska et al., 2018).
db-RDA also indicated that HOMA-IR contributed most to the main explanatory variables affecting distribution of the salivary microbiome. Insulin resistance means that the normal insulin concentration is not sufficient to produce the desired response to its target tissues. In this case, the pancreatic β cells secrete more insulin to overcome hyperglycemia (Onyango, 2018). Insulin exerts its physiological function by binding to tyrosine kinase receptors. Two main parallel pathways are activated after insulin binding: phosphatidylinositol-3 kinase and mitogen-activated protein kinase pathways, while the balance between these two pathways is disrupted under insulin resistance (Kim et al., 2006), which may have an effect on various metabolic processes. Although no significant differences were detected between our two groups with different TSH levels, carbohydrate metabolism was confirmed to have the highest proportion of the functional composition of the microbial community in the saliva samples. This may explain why HOMA-IR contributed most to the main explanatory variables affecting distribution of the salivary microbiome. Lower community diversity was observed in participants with higher TSH levels, which coincides with the suggestion that greater gene richness implies better health (Zhao et al., 2018). Decreased gene functional abundance in the salivary microbiome of individuals with abnormally higher TSH levels may result in decreased biosynthesis of essential amino acids, nucleotides, vitamins, and utilization of carbohydrate and energy, which may be involved in the tendency of insulin resistance. Considering that 16S rDNA sequencing does not yield robust metabolic and functional pathways, several significant variances may be detected using further metagenomic sequencing.
Insulin resistance with abnormally higher serum TSH levels is accompanied with shifts in the salivary microbiome. Aggregatibacter, Treponema 2, norank p Gracilibacteria, Campylobacter, and Bergeyella were negatively associated with HOMA-IR, while Atopobium, Lachnoanaerobaculum, and Stomatobaculum were positively correlated with HOMA-IR. Aggregatibacter is prevalent at multiple oral sites and closely related to aggressive periodontitis (Åberg et al., 2015), and is associated with infective endocarditis (Nørskov-Lauritsen, 2014) and non-alcoholic fatty liver disease (Komazaki et al., 2017). Treponema denticola and Aggregatibacter actinomycetemcomitans are increased in healthy periodontal sites in patients with insulin-dependent diabetes mellitus compared with those without diabetes mellitus (Aemaimanan et al., 2013). Decreased abundance of Treponema 2 was recorded after treatment in type 2 diabetic Goto–Kakizaki rats (Zhang et al., 2019). Campylobacter is a Gram-negative bacillus and involved in many human diseases including gastroenteritis, abortion, septicemia, inflammatory bowel disease, and periodontitis (Lee et al., 2016). Campylobacter has a positive association with higher blood glucose levels, and likely contributes to the development of hyperglycemia (Wei et al., 2020). Bergeyella is correlated with volatile sulfur compounds among periodontally healthy adults (Ye et al., 2019), preterm birth (Han et al., 2006), and infective endocarditis (Mulliken et al., 2019). Atopobium is correlated with sarcoidosis (Zimmermann et al., 2017) and significantly increased in multiple polypoid adenomas and/or intramucosal carcinomas (Yachida et al., 2019). Atopobium and Stomatobaculum can also predicted greater fasting plasma glucose (FPG) change and insulin resistance according to a study among 230 diabetes-free adults (Demmer et al., 2019). Lachnoanaerobaculum can be isolated from gingivitis lesions (Lim et al., 2019) and is associated with recurrent aphthous stomatitis (Stehlikova et al., 2019). Chronic systemic inflammation has also been linked to increased risk of insulin resistance and the oral microbiome. Inflammation may cause oral dysbiosis and risk of diabetes (Demmer et al., 2017).
There were some limitations to our study. First, although 114 participants were recruited, only 40 were matched in two groups and underwent relevant bioinformatic analysis. A larger study needs to be carried out to verify our preliminary findings and the biological mechanisms involved require further experimental study. To further explore the variance in the genetics and function of the microbiome, metagenomics sequencing may be needed in our next study.
Conclusions
The salivary microbial profile shifted with serum TSH levels, which was reflected in the increase in taxa diversity and change of community structure and species composition, along with elevated TSH levels. Granulicatella was significantly more prevalent in individuals with abnormal TSH levels. Insulin resistance may play an important role in the accompanying biochemical and microbial alterations. These finding provide a new perspective to explore the association of oral ecological dysbiosis with high TSH levels and may contribute to the diagnosis and interventional treatment of hypothyroidism.
Data Availability Statement
The datasets presented in this study can be found in online repositories. The names of the repository/repositories and accession number(s) can be found below: https://www.ncbi.nlm.nih.gov/, SRP273570.
Ethics Statement
This study followed the Declaration of Helsinki on medical protocol and ethics and obtained the approval from the regional Ethical Review Board of Shanghai 9th Hospital affiliated to Shanghai Jiaotong University, School of Medicine (2019-T295-1). The patients/participants provided their written informed consent to participate in this study.
Author Contributions
DT and ZF performed the study design, data analysis, drafting and revising of the work. YKY and ZXH performed the bioinformatics analysis and sampling collection. WNJ, XFZ and LYL provided the relative clinical assessment. LYL and HZW contributed to the study design, clinical sample collection, data analysis, drafting, revising, final approval. All authors contributed to the article and approved the submitted version.
Funding
This work was supported by grants from the National Natural Science Foundation of China (82071104/81570964/81371143), the Shanghai Clinical Research Center for Oral Diseases (19MC1910600), and partly supported by the Shanghai Ninth People’s Hospital affiliated with Shanghai Jiao Tong University, School of Medicine (JYJC201806/JYLJ201908).
Conflict of Interest
The authors declare that the research was conducted in the absence of any commercial or financial relationships that could be construed as a potential conflict of interest.
Acknowledgments
Thanks are due to our co-workers for their assistance and all the study participants for their involvement. We thank Shanghai Majorbio Biopharm Technology Co. Ltd. for providing the free online Majorbio Cloud Platform and technical support for the bioinformatics analysis. We also thank International Science Editing (http://www.internationalscienceediting.com) for editing this manuscript.
Supplementary Material
The Supplementary Material for this article can be found online at: https://www.frontiersin.org/articles/10.3389/fcimb.2021.603291/full#supplementary-material
Supplementary Figure 1 | Rarefaction curve on the OTUs level. The curve tends to be flat, indicating that the sequencing data volume is reasonable.
Supplementary Figure 2 | Unweighted Unifrac db-RDA reflected the relationship between salivary microbiome and clinical variables. Red indicates samples from the Abnormal Group and blue indicates samples from the Normal Group. The red arrow indicates the quantitative clinical variables. The length of the arrow represents the degree of influence (interpretation) of the clinical variables on the species data; the angle between the arrows represents positive and negative correlation (acute angle: positive correlation; obtuse angle: negative correlation; right angle: no correlation).
Supplementary Figure 3 | Alterative predictive metagenome functional profiling along with serum TSH levels. (A) Relative abundance of predictive metagenome functional profiling of the top 10 abundant KEGG level 2 pathways (pathways with P(FDR)<0.05 are shown). (B) Relative abundance of predictive metagenome functional profiling of the top 10 abundant KEGG level 3 pathways (pathways with P(FDR)<0.05 are shown).
References
Åberg C. H., Kelk P., Johansson A. (2015). Aggregatibacter actinomycetemcomitans: virulence of its leukotoxin and association with aggressive periodontitis. Virulence 6 (3), 188–195. doi: 10.4161/21505594.2014.982428
Aemaimanan P., Amimanan P., Taweechaisupapong S. (2013). Quantification of key periodontal pathogens in insulin-dependent type 2 diabetic and non-diabetic patients with generalized chronic periodontitis. Anaerobe 22, 64–68. doi: 10.1016/j.anaerobe.2013.06.010
Artunc F., Schleicher E., Weigert C., Fritsche A., Stefan N., Häring H. U. (2016). The impact of insulin resistance on the kidney and vasculature. Nat. Rev. Nephrol. 12 (12), 721–737. doi: 10.1038/nrneph.2016.145
Asvold B. O., Bjøro T., Vatten L. J. (2013). Associations of TSH levels within the reference range with future blood pressure and lipid concentrations: 11-year follow-up of the HUNT study. Eur. J. Endocrinol. 169 (1), 73–82. doi: 10.1530/eje-13-0087
Belstrøm D. (2020). The salivary microbiota in health and disease. J. Oral. Microbiol. 12 (1):1723975. doi: 10.1080/20002297.2020.1723975
Bos M. M., Smit R. A. J., Trompet S., van Heemst D., Noordam R. (2017). Thyroid Signaling, Insulin Resistance, and 2 Diabetes Mellitus: A Mendelian Randomization Study. J. Clin. Endocrinol. Metab. 102 (6), 1960–1970. doi: 10.1210/jc.2016-2816
Cheng Z., Do T., Mankia K., Meade J., Hunt L., Clerehugh V., et al. (2021). Dysbiosis in the oral microbiomes of anti-CCP positive individuals at risk of developing rheumatoid arthritis. Ann. Rheum. Dis. 80 (2), 162–168. doi: 10.1136/annrheumdis-2020-216972
Chopra A., Radhakrishnan R., Sharma M. (2020). Porphyromonas gingivalis and adverse pregnancy outcomes: a review on its intricate pathogenic mechanisms. Crit. Rev. Microbiol. 46 (2), 213–236. doi: 10.1080/1040841x.2020.1747392
Clos-Garcia M., Garcia K., Alonso C., Iruarrizaga-Lejarreta M., D’Amato M., Crespo A., et al. (2020). Integrative Analysis of Fecal Metagenomics and Metabolomics in Colorectal Cancer. Cancers (Basel) 12 (5). doi: 10.3390/cancers12051142
de Andrade P. A. M., Giovani P. A., Araujo D. S., de Souza A. J., Pedroni-Pereira A., Kantovitz K. R., et al. (2020). Shifts in the bacterial community of saliva give insights on the relationship between obesity and oral microbiota in adolescents. Arch. Microbiol. 202 (5), 1085–1095. doi: 10.1007/s00203-020-01817-y
Delitala A. P., Fanciulli G., Maioli M., Delitala G. (2017). Subclinical hypothyroidism, lipid metabolism and cardiovascular disease. Eur. J. Intern. Med. 38, 17–24. doi: 10.1016/j.ejim.2016.12.015
Demmer R. T., Breskin A., Rosenbaum M., Zuk A., LeDuc C., Leibel R., et al. (2017). The subgingival microbiome, systemic inflammation and insulin resistance: The Oral Infections, Glucose Intolerance and Insulin Resistance Study. J. Clin. Periodontol. 44 (3), 255–265. doi: 10.1111/jcpe.12664
Demmer R. T., Trinh P., Rosenbaum M., Li G., LeDuc C., Leibel R., et al. (2019). Subgingival Microbiota and Longitudinal Glucose Change: The Oral Infections, Glucose Intolerance and Insulin Resistance Study (ORIGINS). J. Dent. Res. 98 (13), 1488–1496. doi: 10.1177/0022034519881978
Gaiser R. A., Halimi A., Alkharaan H., Lu L., Davanian H., Healy K., et al. (2019). Enrichment of oral microbiota in early cystic precursors to invasive pancreatic cancer. Gut 68 (12), 2186–2194. doi: 10.1136/gutjnl-2018-317458
Gantuya B., El Serag H. B., Matsumoto T., Ajami N. J., Uchida T., Oyuntsetseg K., et al. (2020). Gastric mucosal microbiota in a Mongolian population with gastric cancer and precursor conditions. Aliment. Pharmacol. Ther. 51 (8), 770–780. doi: 10.1111/apt.15675
Gao L., Hu Y., Wang Y., Jiang W., He Z., Zhu C., et al. (2015). Exploring the variation of oral microbiota in supragingival plaque during and after head-and-neck radiotherapy using pyrosequencing. Arch. Oral. Biol. 60 (9), 1222–1230. doi: 10.1016/j.archoralbio.2015.05.006
Gao L., Xu T., Huang G., Jiang S., Gu Y., Chen F. (2018). Oral microbiomes: more and more importance in oral cavity and whole body. Protein Cell 9 (5), 488–500. doi: 10.1007/s13238-018-0548-1
Giles J. T., Reinholdt J., Andrade F., Konig M. F. (2020). Associations of Antibodies Targeting Periodontal Pathogens with Subclinical Coronary, Carotid, and Peripheral Arterial Atherosclerosis in Rheumatoid Arthritis. Arthritis Rheumatol.. doi: 10.1002/art.41572
Han Y. W., Ikegami A., Bissada N. F., Herbst M., Redline R. W., Ashmead G. G. (2006). Transmission of an uncultivated Bergeyella strain from the oral cavity to amniotic fluid in a case of preterm birth. J. Clin. Microbiol. 44 (4), 1475–1483. doi: 10.1128/jcm.44.4.1475-1483.2006
Jun J. E., Jee J. H., Bae J. C., Jin S. M., Hur K. Y., Lee M. K., et al. (2017). Association Between Changes in Thyroid Hormones and Incident Type 2 Diabetes: A Seven-Year Longitudinal Study. Thyroid 27 (1), 29–38. doi: 10.1089/thy.2016.0171
Kim J. A., Montagnani M., Koh K. K., Quon M. J. (2006). Reciprocal relationships between insulin resistance and endothelial dysfunction: molecular and pathophysiological mechanisms. Circulation 113 (15), 1888–1904. doi: 10.1161/circulationaha.105.563213
Komazaki R., Katagiri S., Takahashi H., Maekawa S., Shiba T., Takeuchi Y., et al. (2017). Periodontal pathogenic bacteria, Aggregatibacter actinomycetemcomitans affect non-alcoholic fatty liver disease by altering gut microbiota and glucose metabolism. Sci. Rep. 7 (1), 13950. doi: 10.1038/s41598-017-14260-9
Kopp P. (2001). The TSH receptor and its role in thyroid disease. Cell Mol. Life Sci. 58 (9), 1301–1322. doi: 10.1007/pl00000941
Kouidhi S., Berhouma R., Ammar M., Rouissi K., Jarboui S., Clerget-Froidevaux M. S., et al. (2013). Relationship of thyroid function with obesity and type 2 diabetes in euthyroid Tunisian subjects. Endocr. Res. 38 (1), 15–23. doi: 10.3109/07435800.2012.699987
Krishnan K., Chen T., Paster B. J. (2017). A practical guide to the oral microbiome and its relation to health and disease. Oral. Dis. 23 (3), 276–286. doi: 10.1111/odi.12509
Kumar P. S. (2013). Sex and the subgingival microbiome: do female sex steroids affect periodontal bacteria? Periodontol. 2000 61 (1), 103–124. doi: 10.1111/j.1600-0757.2011.00398.x
Laclaustra M., Moreno-Franco B., Lou-Bonafonte J. M., Mateo-Gallego R., Casasnovas J. A., Guallar-Castillon P., et al. (2019). Impaired Sensitivity to Thyroid Hormones Is Associated With Diabetes and Metabolic Syndrome. Diabetes Care 42 (2), 303–310. doi: 10.2337/dc18-1410
Lee S., Lee J., Ha J., Choi Y., Kim S., Lee H., et al. (2016). Clinical relevance of infections with zoonotic and human oral species of Campylobacter. J. Microbiol. 54 (7), 459–467. doi: 10.1007/s12275-016-6254-x
Lee L. C., Liong C. Y., Jemain A. A. (2018). Partial least squares-discriminant analysis (PLS-DA) for classification of high-dimensional (HD) data: a review of contemporary practice strategies and knowledge gaps. Analyst 143 (15), 3526–3539. doi: 10.1039/c8an00599k
LeFevre M. L. (2015). Screening for thyroid dysfunction: U.S. Preventive Services Task Force recommendation statement. Ann. Intern. Med. 162 (9), 641–650. doi: 10.7326/m15-0483
Leiva-Gea I., Sánchez-Alcoholado L., Martín-Tejedor B., Castellano-Castillo D., Moreno-Indias I., Urda-Cardona A., et al. (2018). Gut Microbiota Differs in Composition and Functionality Between Children With Type 1 Diabetes and MODY2 and Healthy Control Subjects: A Case-Control Study. Diabetes Care 41 (11), 2385–2395. doi: 10.2337/dc18-0253
Li D., Xi W., Zhang Z., Ren L., Deng C., Chen J., et al. (2020). Oral microbial community analysis of the patients in the progression of liver cancer. Microb. Pathog. 149:104479. doi: 10.1016/j.micpath.2020.104479
Lim Y. K., Park S. N., Jo E., Shin J. H., Chang Y. H., Shin Y., et al. (2019). Lachnoanaerobaculum gingivalis sp. nov., Isolated from Human Subgingival Dental Plaque of a Gingivitis Lesion. Curr. Microbiol. 76 (10), 1147–1151. doi: 10.1007/s00284-019-01747-z
Lin W., Jiang W., Hu X., Gao L., Ai D., Pan H., et al. (2018). Ecological Shifts of Supragingival Microbiota in Association with Pregnancy. Front. Cell Infect. Microbiol. 8:24:24. doi: 10.3389/fcimb.2018.00024
Lourenço T. G., Heller D., Silva-Boghossian C. M., Cotton S. L., Paster B. J., Colombo A. P. (2014). Microbial signature profiles of periodontally healthy and diseased patients. J. Clin. Periodontol. 41 (11), 1027–1036. doi: 10.1111/jcpe.12302
Lozupone C. A., Hamady M., Kelley S. T., Knight R. (2007). Quantitative and qualitative beta diversity measures lead to different insights into factors that structure microbial communities. Appl. Environ. Microbiol. 73 (5), 1576–1585. doi: 10.1128/aem.01996-06
Martinez B., Ortiz R. M. (2017). Thyroid Hormone Regulation and Insulin Resistance: Insights From Animals Naturally Adapted to Fasting. Physiol. (Bethesda) 32 (2), 141–151. doi: 10.1152/physiol.00018.2016
Miller D. P., Scott D. A. (2021). Inherently and Conditionally Essential Protein Catabolism Genes of Porphyromonas gingivalis. Trends Microbiol. 29 (1), 54–64. doi: 10.1016/j.tim.2020.09.002
Minty M., Canceil T., Serino M., Burcelin R., Tercé F., Blasco-Baque V. (2019). Oral microbiota-induced periodontitis: a new risk factor of metabolic diseases. Rev. Endocr. Metab. Disord. 20 (4), 449–459. doi: 10.1007/s11154-019-09526-8
Mulliken J. S., Langelier C., Budak J. Z., Miller S., Dynerman D., Hao S., et al. (2019). Bergeyella cardium: Clinical Characteristics and Draft Genome of an Emerging Pathogen in Native and Prosthetic Valve Endocarditis. Open Forum Infect. Dis. 6 (4):ofz134. doi: 10.1093/ofid/ofz134
Mullur R., Liu Y. Y., Brent G. A. (2014). Thyroid hormone regulation of metabolism. Physiol. Rev. 94 (2), 355–382. doi: 10.1152/physrev.00030.2013
Nørskov-Lauritsen N. (2014). Classification, identification, and clinical significance of Haemophilus and Aggregatibacter species with host specificity for humans. Clin. Microbiol. Rev. 27 (2), 214–240. doi: 10.1128/cmr.00103-13
Onyango A. N. (2018). Cellular Stresses and Stress Responses in the Pathogenesis of Insulin Resistance. Oxid. Med. Cell Longev. 2018:4321714. doi: 10.1155/2018/4321714
Ponziani F. R., Bhoori S., Castelli C., Putignani L., Rivoltini L., Del Chierico F., et al. (2019). Hepatocellular Carcinoma Is Associated With Gut Microbiota Profile and Inflammation in Nonalcoholic Fatty Liver Disease. Hepatology 69 (1), 107–120. doi: 10.1002/hep.30036
Pozhitkov A. E., Leroux B. G., Randolph T. W., Beikler T., Flemmig T. F., Noble P. A. (2015). Towards microbiome transplant as a therapy for periodontitis: an exploratory study of periodontitis microbial signature contrasted by oral health, caries and edentulism. BMC Oral. Health 15, 125. doi: 10.1186/s12903-015-0109-4
Qiao Y., Wu M., Feng Y., Zhou Z., Chen L., Chen F. (2018). Alterations of oral microbiota distinguish children with autism spectrum disorders from healthy controls. Sci. Rep. 8 (1), 1597. doi: 10.1038/s41598-018-19982-y
Ren R., Ma Y., Deng F., Li T., Wang H., Wei J., et al. (2019). Association between serum TSH levels and metabolic components in euthyroid subjects: a nationwide population-based study. Diabetes Metab. Syndr. Obes. 12, 1563–1569. doi: 10.2147/dmso.S202769
Roberts D. W. (2009). Comparison of multidimensional fuzzy set ordination with CCA and DB-RDA. Ecology 90 (9), 2622–2634. doi: 10.1890/07-1673.1
Ruhla S., Weickert M. O., Arafat A. M., Osterhoff M., Isken F., Spranger J., et al. (2010). A high normal TSH is associated with the metabolic syndrome. Clin. Endocrinol. (Oxf) 72 (5), 696–701. doi: 10.1111/j.1365-2265.2009.03698.x
Sabharwal A., Ganley K., Miecznikowski J. C., Haase E. M., Barnes V., Scannapieco F. A. (2019). The salivary microbiome of diabetic and non-diabetic adults with periodontal disease. J. Periodontol. 90 (1), 26–34. doi: 10.1002/jper.18-0167
Saeb A. T. M., Al-Rubeaan K. A., Aldosary K., Udaya Raja G. K., Mani B., Abouelhoda M., et al. (2019). Relative reduction of biological and phylogenetic diversity of the oral microbiota of diabetes and pre-diabetes patients. Microb. Pathog. 128, 215–229. doi: 10.1016/j.micpath.2019.01.009
Said H. S., Suda W., Nakagome S., Chinen H., Oshima K., Kim S., et al. (2014). Dysbiosis of salivary microbiota in inflammatory bowel disease and its association with oral immunological biomarkers. DNA Res. 21 (1), 15–25. doi: 10.1093/dnares/dst037
Sanz M., Beighton D., Curtis M. A., Cury J. A., Dige I., Dommisch H., et al. (2017). Role of microbial biofilms in the maintenance of oral health and in the development of dental caries and periodontal diseases. Consensus report of group 1 of the Joint EFP/ORCA workshop on the boundaries between caries and periodontal disease. J. Clin. Periodontol. 44 Suppl 18, S5–s11. doi: 10.1111/jcpe.12682
Segata N., Izard J., Waldron L., Gevers D., Miropolsky L., Garrett W. S., et al. (2011). Metagenomic biomarker discovery and explanation. Genome Biol. 12 (6), R60. doi: 10.1186/gb-2011-12-6-r60
Si J., Lee C., Ko G. (2017). Oral Microbiota: Microbial Biomarkers of Metabolic Syndrome Independent of Host Genetic Factors. Front. Cell Infect. Microbiol. 7:516:516. doi: 10.3389/fcimb.2017.00516
Stehlikova Z., Tlaskal V., Galanova N., Roubalova R., Kreisinger J., Dvorak J., et al. (2019). Oral Microbiota Composition and Antimicrobial Antibody Response in Patients with Recurrent Aphthous Stomatitis. Microorganisms 7 (12). doi: 10.3390/microorganisms7120636
Takeshita T., Kageyama S., Furuta M., Tsuboi H., Takeuchi K., Shibata Y., et al. (2016). Bacterial diversity in saliva and oral health-related conditions: the Hisayama Study. Sci. Rep. 6:22164. doi: 10.1038/srep22164
Teixeira Pde F., Reuters V. S., Ferreira M. M., Almeida C. P., Reis F. A., Buescu A., et al. (2008). Lipid profile in different degrees of hypothyroidism and effects of levothyroxine replacement in mild thyroid failure. Transl. Res. 151 (4), 224–231. doi: 10.1016/j.trsl.2007.12.006
Téllez A., Ambrosioni J., Llopis J., Pericàs J. M., Falces C., Almela M., et al. (2018). Epidemiology, Clinical Features, and Outcome of Infective Endocarditis due to Abiotrophia Species and Granulicatella Species: Report of 76 Case-2015. Clin. Infect. Dis. 66 (1), 104–111. doi: 10.1093/cid/cix752
Ujwal Upadya B., Suma M., Srinath K., Akila P., Parveen D., Shilpa S. (2015). Effect of insulin resistance in assessing the clinical outcome of clinical and subclinical hypothyroid patients. J. Clin. Diagn. Res. 9, Oc01–Oc04. doi: 10.7860/JCDR/2015/9754.5513
Uruska A., Zozulinska-Ziolkiewicz D., Niedzwiecki P., Pietrzak M., Wierusz-Wysocka B. (2018). TG/HDL-C ratio and visceral adiposity index may be useful in assessment of insulin resistance in adults with type 1 diabetes in clinical practice. J. Clin. Lipidol. 12 (3), 734–740. doi: 10.1016/j.jacl.2018.01.005
Vyakaranam S., Vanaparthy S., Nori S., Palarapu S., Bhongir A. V. (2014). Study of Insulin Resistance in Subclinical Hypothyroidism. Int. J. Health Sci. Res. 4 (9), 147–153.
Wang L., de Ángel Solá D., Mao Y., Bielecki P., Zhu Y., Sun Z., et al. (2018). Family-based study reveals decreased abundance of sputum Granulicatella in asthmatics. Allergy 73 (9), 1918–1921. doi: 10.1111/all.13493
Wang Y., Zhang C., Hou S., Wu X., Liu J., Wan X. (2020). Analyses of Potential Driver and Passenger Bacteria in Human Colorectal Cancer. Cancer Manag. Res. 12, 11553–11561. doi: 10.2147/cmar.S275316
Waring A. C., Rodondi N., Harrison S., Kanaya A. M., Simonsick E. M., Miljkovic I., et al. (2012). Thyroid function and prevalent and incident metabolic syndrome in older adults: the Health, Ageing and Body Composition Study. Clin. Endocrinol. (Oxf) 76 (6), 911–918. doi: 10.1111/j.1365-2265.2011.04328.x
Wei Y. S., Hsiao Y. C., Su G. W., Chang Y. R., Lin H. P., Wang Y. S., et al. (2020). Identification of hyperglycemia-associated microbiota alterations in saliva and gingival sulcus. Arch. Biochem. Biophys. 682:108278. doi: 10.1016/j.abb.2020.108278
Wu Y., Chi X., Zhang Q., Chen F., Deng X. (2018). Characterization of the salivary microbiome in people with obesity. PeerJ 6, e4458. doi: 10.7717/peerj.4458
Xiao E., Mattos M., Vieira G. H. A., Chen S., Corrêa J. D., Wu Y., et al. (2017). Diabetes Enhances IL-17 Expression and Alters the Oral Microbiome to Increase Its Pathogenicity. Cell Host Microbe 22 (1), 120–128.e124. doi: 10.1016/j.chom.2017.06.014
Yachida S., Mizutani S., Shiroma H., Shiba S., Nakajima T., Sakamoto T., et al. (2019). Metagenomic and metabolomic analyses reveal distinct stage-specific phenotypes of the gut microbiota in colorectal cancer. Nat. Med. 25 (6), 968–976. doi: 10.1038/s41591-019-0458-7
Yang C. Y., Yeh Y. M., Yu H. Y., Chin C. Y., Hsu C. W., Liu H., et al. (2018a). Oral Microbiota Community Dynamics Associated With Oral Squamous Cell Carcinoma Staging. Front. Microbiol. 9:862:862. doi: 10.3389/fmicb.2018.00862
Yang J., Mu X., Wang Y., Zhu D., Zhang J., Liang C., et al. (2018b). Dysbiosis of the Salivary Microbiome Is Associated With Non-smoking Female Lung Cancer and Correlated With Immunocytochemistry Markers. Front. Oncol. 8:520:520. doi: 10.3389/fonc.2018.00520
Ye W., Zhang Y., He M., Zhu C., Feng X. P. (2019). Relationship of tongue coating microbiome on volatile sulfur compounds in healthy and halitosis adults. J. Breath Res. 14 (1), 016005. doi: 10.1088/1752-7163/ab47b4
Zhang X., Zhang D., Jia H., Feng Q., Wang D., Liang D., et al. (2015). The oral and gut microbiomes are perturbed in rheumatoid arthritis and partly normalized after treatment. Nat. Med. 21 (8), 895–905. doi: 10.1038/nm.3914
Zhang C., Wu W., Xin X., Li X., Liu D. (2019). Extract of ice plant (Mesembryanthemum crystallinum) ameliorates hyperglycemia and modulates the gut microbiota composition in type 2 diabetic Goto-Kakizaki rats. Food Funct. 10 (6), 3252–3261. doi: 10.1039/c9fo00119k
Zhao L., Zhang F., Ding X., Wu G., Lam Y. Y., Wang X., et al. (2018). Gut bacteria selectively promoted by dietary fibers alleviate type 2 diabetes. Science 359 (6380), 1151–1156. doi: 10.1126/science.aao5774
Zhou J., Wang L., Yuan R., Yu X., Chen Z., Yang F., et al. (2020). Signatures of Mucosal Microbiome in Oral Squamous Cell Carcinoma Identified Using a Random Forest Model. Cancer Manag. Res. 12, 5353–5363. doi: 10.2147/cmar.S251021
Keywords: thyroid stimulating hormone, salivary microbiome, 16S rDNA sequencing, insulin resistance, community shift
Citation: Dong T, Zhao F, Yuan K, Zhu X, Wang N, Xia F, Lu Y and Huang Z (2021) Association Between Serum Thyroid-Stimulating Hormone Levels and Salivary Microbiome Shifts. Front. Cell. Infect. Microbiol. 11:603291. doi: 10.3389/fcimb.2021.603291
Received: 12 September 2020; Accepted: 11 January 2021;
Published: 26 February 2021.
Edited by:
You Zhou, Cardiff University, United KingdomCopyright © 2021 Dong, Zhao, Yuan, Zhu, Wang, Xia, Lu and Huang. This is an open-access article distributed under the terms of the Creative Commons Attribution License (CC BY). The use, distribution or reproduction in other forums is permitted, provided the original author(s) and the copyright owner(s) are credited and that the original publication in this journal is cited, in accordance with accepted academic practice. No use, distribution or reproduction is permitted which does not comply with these terms.
*Correspondence: Zhengwei Huang, huangzhengwei@shsmu.edu.cn; Yingli Lu, luyingli2008@126.com
†These authors share first authorship