Comprehensive profiling of cell subsets of gastric cancer and liver metastasis based on single cell RNA-sequencing analysis
Highlight box
Key findings
• Natural killer cells with high TNFAIP3 expression were located at the gastric cancer primary tumor (PT) site and corresponded to a poor prognosis, and HLA-E-CD94/NKG2A of natural killer cells was only activated in the PT and not in the liver metastasis (Li).
What is known and what is new?
• Li is one of the most common distant metastatic sites for gastric cancer.
• The aim of this study was to use single cell RNA sequencing (scRNA-seq) to evaluate cell subtypes and understand the molecular mechanism of gastric cancer Li.
What is the implication, and what should change now?
• The present study revealed the difference between Li and gastric PT by scRNA-seq. Our study provides new insights into gastric cancer and Li.
Introduction
Patients with solid cancers mostly die because of metastasis. The clinical presence of metastasis detected by clinical imaging is an indication of an almost certain death in most patients; thus, the prevention of metastatic tumors is a clinical priority (1). Gastric cancer is the third most common cause of cancer death and the fifth most common cancer worldwide (2,3). Liver metastasis (Li) remains a major obstacle in the treatment of malignant diseases, especially for tumors giving metastasis in this organ, such as gastric and intestinal cancer and breast cancer (4,5). Although improvements in the existing diagnostic techniques and treatments improved the outcomes of patients with gastric cancer, the survival when Lis are present is extremely poor, with a median overall survival of only 2 months (6). Therefore, the understanding of the biological and molecular mechanisms of gastric cancer and Li is an urgent priority.
Single cell RNA sequencing (scRNA-seq) is a promising high-throughput approach to study cell subtypes in tumor tissue samples (7-10). The development of this technology has enabled a comprehensive analysis of the human immune system (9,11-13). scRNA-seq enables the finding of the links and differences between primary tumors (PTs) and metastasis by characterizing tumor cell populations as well as by establishing developmental “trajectories” of tumor cells (14-16). Therefore, performing single-cell sequencing of gastric orthotopic tumors and Li with deep data mining may allow the discovery of biological molecular mechanisms.
Metastatic tumor cell survival and proliferation in the liver depend on the outcome of complex cellular interactions between tumor cells and subpopulations in the microenvironment (4,17,18).
Our work characterized and analyzed different cell populations of gastric orthotopic tumors and Lis using existing single-cell datasets. Key genes related to prognosis in metastatic samples were identified by pseudo-time analysis of malignant epithelial cell subsets. In addition, the immune cell subsets infiltrated into the Li and orthotopic tumor were compared to explore the similarities and differences between Li sites and gastric orthotopic tumor sites by analyzing cell-cell interactions. Therefore, our work might provide a new understanding of gastric cancer Li from a bioinformatics analysis perspective. We present this article in accordance with the MDAR reporting checklist (available at https://tcr.amegroups.com/article/view/10.21037/tcr-23-1532/rc).
Methods
Data sources
Gene Expression Omnibus (GEO, http://www.ncbi.nlm.nih.gov/geo/) stores researchers’ raw and processed sequencing data and is a powerful public database (19). Data were downloaded from the GEO database with the serial number GSE163558. The 3 samples GSM5004180, GSM5004181, and GSM5004182 from gastric PTs and the 2 samples GSM5004188 and GSM5004189 from Lis were downloaded for integration and analysis.
Integration of single cell data
The R package Seurat 4.3.0 was downloaded from Seurat net (https://satijalab.org/seurat/) and installed for single-cell data integration analysis. Seurat is a powerful software for single-cell data analysis, enabling an efficient processing of data from the 10× genomics platform (20).
Pseudotime analysis of cell progression
Pseudotime analysis is a method to simulate time. Monocle utilizes advanced machine learning techniques to learn explicit master graphs from single-cell data to rank cells, which can robustly and accurately resolve complex biological processes (21). The pseudotime analysis of epithelial cells by monocle2 was used combined with Seurat to evaluate gene changes. The R package monocle2.22.0, based on R 4.1.1 was downloaded from the functional net (http://cole-trapnell-lab.github.io/monocle-release/).
Gene survival analysis
GEPIA2 (http://gepia2.cancer-pku.cn/) integrated data from The Cancer Genome Atlas (TCGA) and GEO has information on multiple disease subtypes (22). The GEPIA2 online analysis platform was used for survival analysis of the genes.
Immunomarker analysis
The expression of immune markers of different subtypes of T cells was based on the results of previous academic studies on cytotoxic markers NKG7, GZMA, GZMB, and GNLY (23). The results of immunomarker analysis were performed using the R package pheatmap visualization tool.
Gene set variation analysis (GSVA)
The R package BiocManager was used to download the R package GSVA (24). The correlation between the hallmark of tumors and samples was obtained through the enrichment analysis of a single sample gene set of GSVA.
Gene set enrichment analysis (GSEA)
Functional enrichment analysis of significant hallmark gene sets comparing myeloid cells was performed using Hallmark iterative system, and fgsea (1.16.0) and plotGseaTable () were used for the analysis and plot.
Cell-cell interaction analysis
The tumor microenvironment consists of a variety of cell types that communicate through ligand receptor interactions. Targeted ligand receptor interaction can be analyzed by CellChat, which is a single cell data analysis method (25). Therefore, the R package CellChat 1.4.0 was used to analyze the cell interaction between gastric PT and Li cells.
Differential gene analysis
Differential gene analysis was used to analyze the differences among NK cell subsets and among myeloid cell subsets, which was performed using the function FindMakers in the R package Seurat, R 4.1.1. Statistical significance was set as logfc >1 and corrected P less than 0.05.
Patients and immunohistochemistry (IHC)
The study was reviewed and approved by the Ethics Committee of The Second Affiliated Hospital of Wannan Medical College (Wuhu, China) and the ethical number is WYEFYLS2023138. The study was conducted in compliance with the principles of the Declaration of Helsinki (as revised in 2013). All participants provided full written informed consent.
The case inclusion criteria included age 18 years or above; newly diagnosed primary gastric tumor and Li confirmed by histology; without other treatments. The exclusion criteria included history of other malignancies. Three patients with newly diagnosed primary gastric tumor and Li who attended The Second Affiliated Hospital of Wannan Medical College were invited to participate in this study. All patients provided full written informed consent. We obtained three pairs of fresh tissue samples which contained primary gastric tumor tissues and Li tissues from these patients.
IHC was performed as previously described (26) on clinical specimens of gastric orthotopic tumor and Li using the primary antibodies against APOD (1:100, ab108191, Abcam, Cambridge, UK), JUN (1:100, ab40766, Abcam), CXCL5 (1:100, ARG66029, Arigo, Hsinchu, Taiwan, China), TNFAIP3 (1:100, ab270264, Abcam), IL7R (1:100, AG2272, Beyotime, Shanghai, China) and CD94 (1:100, ab235441, Abcam).
Statistical analysis
When appropriate, statistical differences between groups were evaluated using Student’s t-test or Wilcoxon test, depending on the data distribution. Survival analysis was performed using the log-rank test.
Results
Single cell landscape of gastric carcinoma in situ and Li
A total of 23,409 cells from gastric PT (n=3) and Li (n=2) from the dataset GSE163558 were subjected to integrative analysis to investigate the differences between gastric primary cancer and Li (Figure 1A). After clustering for cell dimensionality reduction, subpopulation identification was performed, and T cells, epithelial cells, myeloid cells, NK cells, B cells, and stromal cells were sorted (Figure 1B). RNA expression was normalized using SCTransform. Gene count and feature abundance are shown in Figure 1C,1D. In addition, mitochondrial gene content, erythrocyte gene content, and ribosomal RNA content were analyzed (Figure 1E and Figure S1A,S1B). Erythroid genes contain very little mitochondrial gene cluster in epithelial cells, and ribosomal RNA is expressed in T and B cells. The high gene expression and high quality of cell data for each sample facilitated the subsequent analysis (Figure S1C,S1D). The top 5 highly expressed genes in T cell clusters were IL7R, CD2, CCL5, CD3D, and TRAC. High expression of KRT8, KRT18, and KRT19 was found in epithelial cells, consistent with previous findings. Myeloid cells showed a high expression of S100A8, S100A9, CXCL8, IL1B and NAMPT. The top 5 genes of the NK cell cluster were NKG7, KLRD1, CCL4, TRDC, and CMC1. A high expression of the antigen presenting genes HLA-DRA, IGKC and MS4A1 was found in the B cell cluster. The genes with the highest expression in the stromal cell clusters were IGFBP7 and the fibroblast associated genes COL1A1, COL1A2 and COL3A1 (Figure 1F). The identification of cell subpopulations was based on gene expression using cell markers previously confirmed (Figure 1G) (27): T cells (CD2, CD3D, CD3E, and CD3G), epithelial cells (EPCAM, KRT18, KRT19, and MUC1), myeloid cells (CD68, CSF1R, TPSAB1, and S100A8), NK cells (GNLY, KLRF1, and KLRD1), B cells (CD79A, MS4A1, and IGHG1), and stromal cells (MCAM, VWF, PECAM1, and DCN) were identified. Next, the proportion of cell clusters was analyzed (Figure 1H,1I); T cells were mainly present in the Li sample Li2, epithelial and stromal cells were mainly present in the PT2, NK cells were mainly present in the Li1 and Li2, myeloid cells were present in the PT1 and PT2, while B cells were not specific. The proportion of T cells was 60.9% in the whole samples. Thus, cell subpopulations in the single-cell dataset landscape of gastric PTs and Lis were identified. The proportion of epithelial cells, T cells, NK cells, and myeloid cells were different between PTs and Lis.
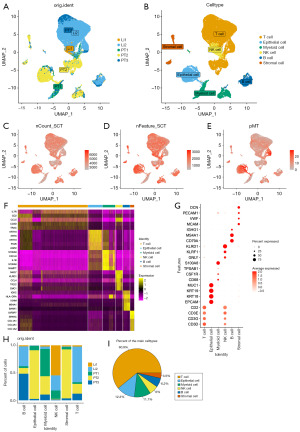
Identification of key genes in the metastatic progression of epithelial cells
The data of 2,878 epithelial cells from gastric PT (n=3) and Li (n=2) from the dataset GSE163558 were extracted for analysis to further investigate the metastatic progression of epithelial cells. After cluster dimension reduction, the links among epithelial cells of different origins were identified, with Li1 being closer to PT1 and PT3, while Li2 was closer to PT2 (Figure 2A). Pseudotime analysis was performed using monocle2 (21), to evaluate hypermutated genes (Figure S2A). Node predictions of the evolutionary tree were consistent with UMAP clustering (Figure 2B). The metastasis of the PT to the liver was in the direction of pseudotime (Figure 2C). Combining the occupancy across samples, pseudotime was PT2 in the early phase and Li1 and Li2 in the late phase (Figure 2D). Notably, the JUN gene was expressed in both the PT and Li, with a trend toward higher expression in metastases than in the PT (Figure 2E). As regards the analysis of the key genes involved in the pseudotime progression, the top 10 most significant genes were APOD, CXCL5, GC, JUN, REG1A, REG3A, S100A12, S100A4, S100A8, and S100A9 (Figure 2F). Cluster analysis was performed on the pseudo temporal differential genes, and the expression of the JUN gene was high in Li, consistent with the previous result (Figure 2G). The survival analysis of stomach adenocarcinoma (STAD) and liver hepatocellular carcinoma (LIHC) from the TCGA dataset were integrated, and the prognostic results of APOD [P<0.001, hazard ratio (HR) >1], CXCL5 (P<0.001, HR >1), GC (P=0.0036, HR <1), and JUN (P=0.0087, HR >1) genes were statistically analyzed (Figure 2H-2K), revealing that results of these four genes in STAD and LIHC were consistent with those of the integrative analysis (Figure S2B-S2I). Thus, the analysis of the epithelial pseudotime suggested that the genes APOD, CXCL5, and JUN might play promoting roles during the progression of Li from gastric primary carcinoma. The Jun gene, in particular, was expressed at higher levels throughout the progression of metastasis in epithelial cells and had a trend towards higher expression accompanied by metastasis.
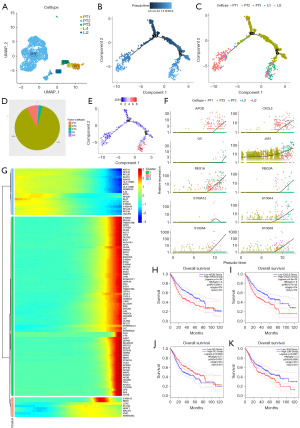
Distinct T cell subsets and immune effector analysis
T-cell clusters contained 14,259 cells, representing the 60.9% of the total sample. T-cell infiltration in PT and Li tumors was analyzed. First, the dimensionality reduction clustering of T cells into new cell clusters was performed, and the cell clusters were renamed according to the top 5 highly expressed genes of each cluster as well as according to an additional single-cell database CellMarker (28). The T cells were divided into primary (left, PT) and metastatic (right, Li) and divided into 10 clusters (Figure 3A) to better distinguish the T cell distribution in the PT from that in the Li. Combining the proportion of sample source and cell type, the results revealed that CD8Tem (effector memory T cell) and CD8T-HSPA1A high and CD4Tm-IL7R high were more numerous in the Li compared with that in the PT. Conversely, exhausted T cells, CD8T-cytotoxic cells and naive T cells were more numerous in the PT samples (Figure 3A-3C). The results of differential gene analysis for each cell cluster indicated differences in each subpopulation, with the CD4Tm-IL7R subpopulation highly expressing both LTB and TXNIP, and the CD8Tem subpopulation highly expressing NKG7, GZMA and CD8A genes, which was similar to the CD8T-cytotoxic subset. Notably, a class of epithelioid T cells highly expressing KRT8, KRT18 and KRT19 was identified (Figure 3D). Immune cell cytotoxicity, depletion as well as co-stimulation are crucial events in the tumor microenvironment. The exhausted T cell cluster and the Treg-MKI67 cell cluster showed a high expression of the ICOS, TNFRSF4, CD27 and TNFSF9 costimulatory markers, and expressed the exhaustion markers ENTPD1, LAYN, PDCD1, TIGIT and LAG3. CD8T-cytotoxic and CD8Tem highly expressed the cytotoxic markers NKG7 and GZMA, and among them, CD8T-cytotoxic showed a higher expression of the GZMB, GNLY and PRF1 genes compared to other clusters (Figure 3E). These results suggested that the distribution of the infiltrated T cells was different in the PT and the Li, with cytotoxic CD8 T cells more in the PT than in the Li. Exhausted CD8 T cells were also more enriched in the PT. CD4 T memory and CD8 T effector memory with partial cytotoxicity and co-stimulation were enriched in the Li. Notably, a class of epithelium-like T cells appeared in the PT, and this class of cells promoted the malignant epithelial progression rather than promoting tumor immunity.
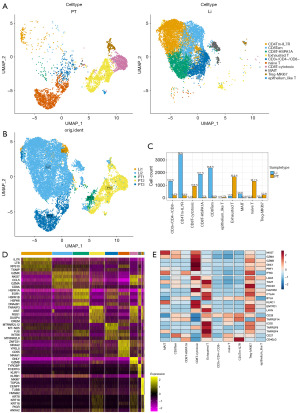
NK cells promote immunity in Li while promoting tumor development in the primary site
NK cells are involved in tumor immunity. A total of 1,419 NK cells were isolated from the total sample to distinguish PTs from Lis. Next, cell clusters were redefined as class 8 cells after dimensionality reduction clustering with 2 NKT cell clusters (Figure 4A). A class of NK cells with high TNFAIP3 expression derived from gastric PT samples was identified combining sample source and cell type occupancy, as well as a class of NKT cells with high IL7R expression mainly derived from Li (Figure 4B and Figure S3A,S3B). The results of top 5 highly expressed genes per cell cluster were found: IL7R high cluster highly expressed the cytotoxic related gene GNLY, TNFAIP3 high cluster expressed the cell cycle mediator RGCC, heat shock protein family gene HSPA1A, and the tumor progression promoting gene CXCR4 (Figure 4C). The TNFAIP3 high cluster highly expressed heat shock protein family genes as revealed by the comparison of the TNFAIP3 high cluster with the IL7R high cluster. The IL7R high cluster then expressed the tumor suppressor associated genes TXNIP and RIPOR2 (Figure 4D). The GSVA used to perform the hallmark enrichment analysis of the 8 NK cell clusters revealed that the TNFAIP3 high cluster showed an enrichment of hallmark pathways associated with tumors compared with the other clusters (Figure 4E). Next, the survival analysis from TCGA indicated that IL7R was a good prognostic factor for the survival of patients with LIHC (P=0.017, HR <1), while the high expression of TNFAIP3 was a poor prognostic factor (P=0.39, HR >1) (Figure 4F-4G). The survival analysis of patients with STAD also similarly showed that patients with high TNFAIP3 expression had a worse prognosis (P=0.13, HR >1) (Figure S3C-S3D). Thus, there were two distinct NK cell populations, TNFAIP3 high and IL7R high, in the PT and Li samples. The former promoted the progression of the tumors, and the latter inhibited tumor progression.
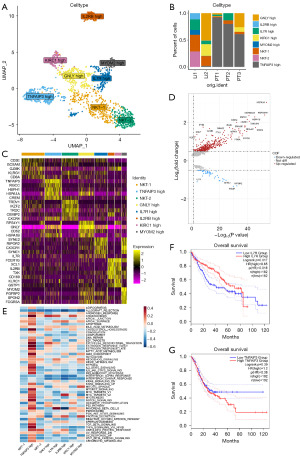
Difference in myeloid cell composition between primary gastric tumor and Li
Myeloid cells play a crucial role in the tumor microenvironment. Myeloid cells (n=2,589) from the gastric primary cancer and the Li were collected and subsets were analyzed for differences in composition. Myeloid cells were subdivided into nine cell clusters based on the expression of genes and markers (Figure 5A). The results of the UMAP dimension reduction clustering were similar for Li1 and Li2, and similar for PT1 and PT3 (Figure 5B). The high expression top gene parts of the 9 cell clusters were similar, but some differences were found between PT and Li (Figure 5C-5F). DCs (CD1c high) mainly existed in Li samples, as revealed by the combination with the results of cell proportion. In contrast, CXCL8 + and DCs (PDF4B high) cell clusters were mainly found in PT samples. Notably, neutrophils were present in each sample. Although the PT and Li sites contained many of the same myeloid cells, such as DCs (VCAN high), mono/macrophages, and DCs (S100A12 high), the differential genes of PT compared to Li showed that PT highly expressed the LITAF, IL1RN, CTSL, and NAMPT genes, which were associated with tumor immunity. Li highly expressed ITGA4 and POUF2 related to tumor migration and proliferation (Figure 5G). The differential gene sets of PT and Li were further subjected to GSEA. The results showed that PT was associated with angiogenesis, coagulation and inflammatory response, while Li was associated with MYC targets, p53 pathway, myogenesis and allograft rejection (Figure 5H). Therefore, the different cellular composition and gene expression of myeloid cells in the PT and in the metastasis in the liver might have a different contribution to the tumor. Our study provided an a priori reference for the analysis in future research.
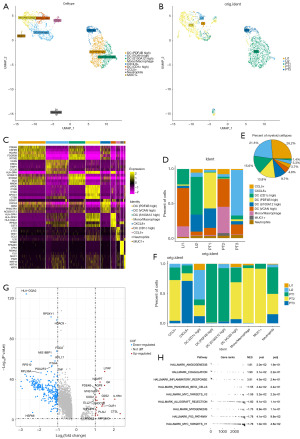
Differences in cell communication at PT compared to Li
The interaction between cells was analyzed to further investigate the tumor microenvironment in both locations. First, the cellular interaction of PT and Li was integrated by CellChat (25), and the number and strength of cellular interaction indicated that epithelial cell interaction with other types of cells was strong (Figure 6A and Figure S4A). Furthermore, the splitting of PT and Li showed that the PT had more cellular interaction compared to the Li (Figure 6B). The strength of epithelial, NK and myeloid cells as receptors were high in PT, the strength of stromal cells as legends were the highest, while T cell and B cell interaction was less strong. The strength of NK cells, myeloid cells, B cells as both legends and receptors became stronger in Li, whereas epithelial cells weakly interacted with other cells. Stromal cells increased the intensity of incoming compared to PT (Figure 6C,6D). Despite the large variation in intensity, the number of cellular interaction was substantial. Epithelial cells in the PT had more cell interaction pathways such as APP, MIF, CD46, GALECTIN, and OCLN. MIF promoted tumors by activating downstream pathways, such as proliferation, angiogenesis, and tumor invasiveness. Epithelial cells in the Li had more enriched pathways such as complement, NECTIN, SPP1, GDF and VTN. SPP1 contributed to tumor epithelial mesenchymal transition, NECTIN is a receptor of TIGIT. Notably, an activation of the NECTIN pathway in PT samples was also observed (Figure S4B). Next, we compared Li to PT by analyzing the activated signaling pathways in different cell clusters (Figure 6E-6G and Figure S4C-S4E). The activation of antigen presentation class II (MHC-II) signaling in Li was present in stromal cells, NK cells, B cells, and myeloid cells, while epithelial cells, NK cells and myeloid cells had activation of CXCL or CCL signaling in the PT group. Combining the overall significant signaling pathways for top ranking, CXCL, ICAM, CCL, and IL1 signaling pathways were activated in PT, while MHC-II, CD48 and SPP1 were activated in Li (Figure 6H). In addition, the information flow count of CXCL and CCL was low, whereas that of MHC-II was high in Li (Figure S4F). Taken together, the differential cellular interactions of PT versus Li were mainly in MHC-II, CXCL and CCL signaling pathways, and the number and strength of interactions differed among cell types.
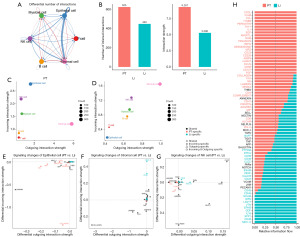
Activation of distinct signaling axes between cell types in PT versus Li
The interaction between different cell types was analyzed to further study the signaling axis between various types of cells (Figure 7 and Figure S5). The interaction among epithelial cells was the highest in PT (n=42), followed by NK cells (n=31), myeloid cells (n=25) and stromal cells (n=25). These signal interactions were weakened in Li, but the interaction with stromal cells (n=32) became stronger (Figure 7A). A detailed signaling axis analysis showed the increase of signal interaction between epithelial cells and stromal cells in the Li, such as VEGFA-VEGFR1/R2 and GDF15-TGFBR2. However, MIF-CD74/CXCR4/CXCR2/CD44, CXCL1/2/3/8-CXCR1/2 signaling in epithelial cells and myeloid cells decreased, and MDK-NCL and HLA-A/B/C/E/F signaling in epithelial cells and NK cells also decreased (Figure 7E,7F). NK cells had less interaction with epithelial cells and more interaction with stromal cells in the Li than in the PT (Figure 7B). The increased PTPRC-MRC1, GZMA-F2R and CD99 signaling axes were between NK cells and stromal cells. The reduced SEMA4D-PLXNB2, MIF-CD74/CD44 and ADGRE5-CD55 signaling axes were between NK and epithelial cells (Figure 7G-7H). In addition, the interaction of NK cells became less in Li, and LAMA4-ITGA2 + ITGB1 and COL1A1-ITGA1 + ITGB1 signaling were weakened or absent in stromal cells and epithelial cells/NK cells (Figure 7C and Figure S5C). The interaction between myeloid cells and stromal cells was increased, and the interaction between myeloid cells and epithelial cells was decreased (Figure 7D). The increase of the MHC-II signaling and the decrease of SEM4D-PLXNB2 signaling and MHC-I signaling in the myeloid cells were found in Li (Figure S5). Furthermore, the interactions between T cells, B cells and other cells were few at Li (Figure S5A,S5B), with a decrease in the signaling axis of mainly MHC-I and an increase in MHC-II at Li but not PT site (Figure S5F-S5H). Notably, the HLA-E-CD94/NKG2A signaling between T cells, B cells, and myeloid cells to NK cells was found in the PT rather than in the Li.
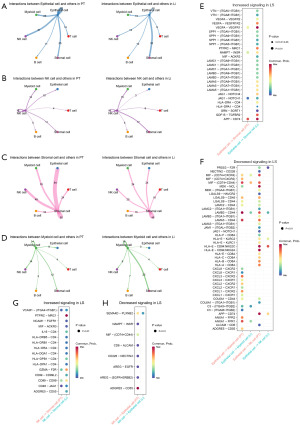
Genes associated with Li in gastric cancer differentially express in histological level
The results of IHC of an independent cohort of patients on the expression of APOD, JUN, and CXCL5 proteins revealed a low APOD expression in PT tissues and a high expression in Li tissues (Figure 8A). The expression of JUN was low in PT tissues and medium to high in Li (Figure 8B). The expression of CXCL5 was low or not expressed in PT, while it showed a high expression in Li tissues (Figure 8C). The expression of the above proteins was significantly different in PT compared to Li tissues. These results are consistent with our scRNA-seq analysis showing that APOD, JUN, and CXCL5 were upregulated during the pseudotime course of Li in gastric cancer.
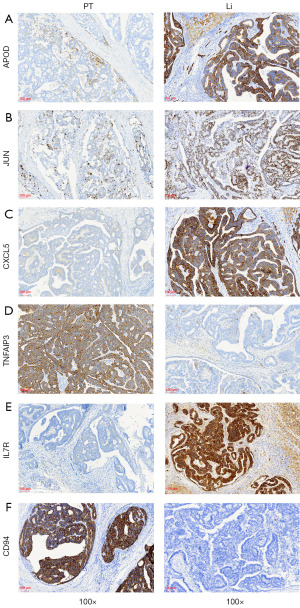
Next, TNFAIP3 protein expression, a marker of TNFAIP3 high NK in PT, and IL7R protein expression, a marker of IL7R high NK in Li were evaluated and the results showed a high TNFAIP3 expression in PT compared to Li (Figure 8D), while high IL7R expression was found in Li tissues compared to PT (Figure 8E). These results were consistent with our scRNA-seq analysis results. HLA-E-CD94/NKG2A signaling was enriched in PT based on cell-cell communication analysis. CD94 protein expression was high in PT tissues (Figure 8F).
The IHC of these additional sample cohorts further strengthened our notion that APOD, JUN and CXCL5 promoted gastric cancer Li and that the subtypes of NK were different in PT and Li.
Discussion
The progression of gastric cancer as it spreads to other organs might vary due to interactions between cancer cells and immune cells residing in the tumor. Our results enhanced the understanding of metastatic progression of malignant epithelial cells and the influence of the PT, Li tissue, on their phenotype. The simultaneous analysis of immune cells from multiple tissues of PT and Li patients with gastric PTs and Lis delineated a comprehensive landscape across different tissues. Our study revealed that different cell types orchestrated organ phenotypes through cell-cell signaling interactions. The PT and Li sites harbor distinct immune cell populations, comprising Exhausted-T cells and TNFAIP3 high NK cells within the PT microenvironment. Functionally, these cells exhibit immunosuppressive traits or are associated with tumor progression.
At the sample level, our results showed that the potential tissue metastasis progression of epithelial cells was regulated by genes such as CXCL5, APOD, GC and JUN. These genes also affected patient survival. JUN is a transcription factor of proteins that regulate gene expression and function particularly important during tumor evolution (29). The analysis of previous scRNA-seq identified genes associated with JUN during gastric cancer evolution (30). scRNA-seq also explains that JUN has a high expression pattern upon leukemia progression that regresses after a treatment but reoccurs at relapse and death (31). The results of JUN protein expression by IHC supported our view at the protein level. Our study revealed that JUN also had high expression during Li of gastric cancer and regulated metastasis.
At the cluster level, PT also had a very higher proportion of CD8 T cells with high expression of cytotoxic than concurrently exhausted T cells, in comparison to Li. CD8 T cells are one of the main effector cells that exert anticancer immune effects, and exhausted CD8 T cells are a barrier for a successful cancer elimination (32). The results of cell communication suggested a class of MHC-I in epithelial cell interaction with other immune cells in PT. Immune escape is essential for the progression of cancer. It has been reported that the expression of HMC-I is low in pancreatic ductal adenocarcinoma and infiltrating CD8 T cells are absent, thus promoting tumor progression (33). Our study found that gastric cancer PT tissues had higher MHC-I than Li, and our conclusion was that gastric cancer highly expressed MHC-I and had infiltration of CD8 T cells, leading to a better immune efficacy compared with Li.
Distant metastasis of malignant cells is a major cause of cancer-related death. NK cells can exert killing effects on malignant cells (34). Our study identified a cluster of NK cells that highly expressed TNFAIP3 in primary gastric cancer, and it was closely related to the regulation of tumor metastasis, such as epithelial mesenchymal transition and hypoxia. Immunohistochemical staining for TNFAIP3 indicated its expression in PT tissue. Previous studies reported that the heterozygous deletion of TNFAIP3 improves the survival of patients with NK cell lymphoma (35). Our study demonstrated that NK with high expression of TNFAIP3 promoted tumor progression.
Cell-cell communication showed how PT and Li differed in antigen presentation. MHC-I had signalling activation mainly in PT. MHC-II had signaling activation mainly in Li. MHC-I presents antigen to CD8 T cells, and MHC-II primarily presents antigen to CD4 T cells (36). Our study revealed that the primary site of gastric cancer contributed to the recognition and killing of tumor cells by CD8 T cells because antigen presentation by MHC-I occurred between the malignant epithelium and T cells. It was mainly the presentation by MHC-II at the site of Li that mediated the regulatory effect of CD4 T cells.
As our data only captured static RNA expression, future techniques that can visualize cells might help understanded better the cell dynamics in the primary and metastatic microenvironments. It is important to note that our study is a transcriptomic based alignment approach to analyze cellular phenotypes. For instance, pseudotime analysis does not fully represent the actual origin of cells and is used to infer changes in cellular states, although this approach provided insight into potential phenotypes. Our study might inspire future works to ultimately reveal how the properties of the tumor microenvironment of the primary gastric cancer and metastasis are shaped.
Conclusions
This study revealed the difference cell subsets between Li and gastric PT by scRNA-seq, demonstrating the impact of partial gene expression on patient prognosis. Despite the limitations in our study, it provides new insights into gastric cancer and Li.
Acknowledgments
We would like to thank the databases TCGA and GEO that give free access to many useful data. We appreciate the contributors of these public databases used in this study.
Funding: This work was funded and supported by
Footnote
Reporting Checklist: The authors have completed the MDAR reporting checklist. Available at https://tcr.amegroups.com/article/view/10.21037/tcr-23-1532/rc
Data Sharing Statement: Available at https://tcr.amegroups.com/article/view/10.21037/tcr-23-1532/dss
Peer Review File: Available at https://tcr.amegroups.com/article/view/10.21037/tcr-23-1532/prf
Conflicts of Interest: All authors have completed the ICMJE uniform disclosure form (available at https://tcr.amegroups.com/article/view/10.21037/tcr-23-1532/coif). The authors have no conflicts of interest to declare.
Ethical Statement: The authors are accountable for all aspects of the work in ensuring that questions related to the accuracy or integrity of any part of the work are appropriately investigated and resolved. The study was reviewed and approved by the Ethics Committee of The Second Affiliated Hospital of Wannan Medical College (Wuhu, China) and the ethical number is WYEFYLS2023138. The study was conducted in compliance with the principles of the Declaration of Helsinki (as revised in 2013). All participants provided full written informed consent.
Open Access Statement: This is an Open Access article distributed in accordance with the Creative Commons Attribution-NonCommercial-NoDerivs 4.0 International License (CC BY-NC-ND 4.0), which permits the non-commercial replication and distribution of the article with the strict proviso that no changes or edits are made and the original work is properly cited (including links to both the formal publication through the relevant DOI and the license). See: https://creativecommons.org/licenses/by-nc-nd/4.0/.
References
- Klein CA. Cancer progression and the invisible phase of metastatic colonization. Nat Rev Cancer 2020;20:681-94. [Crossref] [PubMed]
- Smyth EC, Nilsson M, Grabsch HI, et al. Gastric cancer. Lancet 2020;396:635-48. [Crossref] [PubMed]
- Xing S, Tian Z, Zheng W, et al. Hypoxia downregulated miR-4521 suppresses gastric carcinoma progression through regulation of IGF2 and FOXM1. Mol Cancer 2021;20:9. [Crossref] [PubMed]
- Brodt P. Role of the Microenvironment in Liver Metastasis: From Pre- to Prometastatic Niches. Clin Cancer Res 2016;22:5971-82. [Crossref] [PubMed]
- Yu D, Yang J, Jin M, et al. Fecal Streptococcus Alteration Is Associated with Gastric Cancer Occurrence and Liver Metastasis. mBio 2021;12:e0299421. [Crossref] [PubMed]
- Marte G, Tufo A, Steccanella F, et al. Efficacy of Surgery for the Treatment of Gastric Cancer Liver Metastases: A Systematic Review of the Literature and Meta-Analysis of Prognostic Factors. J Clin Med 2021;10:1141. [Crossref] [PubMed]
- Kester L, van Oudenaarden A. Single-Cell Transcriptomics Meets Lineage Tracing. Cell Stem Cell 2018;23:166-79. [Crossref] [PubMed]
- Tang F, Barbacioru C, Wang Y, et al. mRNA-Seq whole-transcriptome analysis of a single cell. Nat Methods 2009;6:377-82. [Crossref] [PubMed]
- Muraro MJ, Dharmadhikari G, Grün D, et al. A Single-Cell Transcriptome Atlas of the Human Pancreas. Cell Syst 2016;3:385-394.e3. [Crossref] [PubMed]
- Hashimshony T, Senderovich N, Avital G, et al. CEL-Seq2: sensitive highly-multiplexed single-cell RNA-Seq. Genome Biol 2016;17:77. [Crossref] [PubMed]
- Ren X, Zhang L, Zhang Y, et al. Insights Gained from Single-Cell Analysis of Immune Cells in the Tumor Microenvironment. Annu Rev Immunol 2021;39:583-609. [Crossref] [PubMed]
- Vallejo J, Cochain C, Zernecke A, et al. Heterogeneity of immune cells in human atherosclerosis revealed by scRNA-Seq. Cardiovasc Res 2021;117:2537-43. [Crossref] [PubMed]
- Liang L, Yu J, Li J, et al. Integration of scRNA-Seq and Bulk RNA-Seq to Analyse the Heterogeneity of Ovarian Cancer Immune Cells and Establish a Molecular Risk Model. Front Oncol 2021;11:711020. [Crossref] [PubMed]
- Peng J, Sun BF, Chen CY, et al. Single-cell RNA-seq highlights intra-tumoral heterogeneity and malignant progression in pancreatic ductal adenocarcinoma. Cell Res 2019;29:725-38. [Crossref] [PubMed]
- Dong R, Yang R, Zhan Y, et al. Single-Cell Characterization of Malignant Phenotypes and Developmental Trajectories of Adrenal Neuroblastoma. Cancer Cell 2020;38:716-733.e6. [Crossref] [PubMed]
- Ren X, Zhou C, Lu Y, et al. Single-cell RNA-seq reveals invasive trajectory and determines cancer stem cell-related prognostic genes in pancreatic cancer. Bioengineered 2021;12:5056-68. [Crossref] [PubMed]
- Kmieć Z. Cooperation of liver cells in health and disease. Adv Anat Embryol Cell Biol 2001;161:III-XIII, 1-151. [Crossref] [PubMed]
- Smedsrød B, Le Couteur D, Ikejima K, et al. Hepatic sinusoidal cells in health and disease: update from the 14th International Symposium. Liver Int 2009;29:490-501. [Crossref] [PubMed]
- Barrett T, Wilhite SE, Ledoux P, et al. NCBI GEO: archive for functional genomics data sets--update. Nucleic Acids Res 2013;41:D991-5. [Crossref] [PubMed]
- Hao Y, Hao S, Andersen-Nissen E, et al. Integrated analysis of multimodal single-cell data. Cell 2021;184:3573-3587.e29. [Crossref] [PubMed]
- Qiu X, Mao Q, Tang Y, et al. Reversed graph embedding resolves complex single-cell trajectories. Nat Methods 2017;14:979-82. [Crossref] [PubMed]
- Tang Z, Kang B, Li C, et al. GEPIA2: an enhanced web server for large-scale expression profiling and interactive analysis. Nucleic Acids Res 2019;47:W556-60. [Crossref] [PubMed]
- Ji AL, Rubin AJ, Thrane K, et al. Multimodal Analysis of Composition and Spatial Architecture in Human Squamous Cell Carcinoma. Cell 2020;182:497-514.e22. [Crossref] [PubMed]
- Hänzelmann S, Castelo R, Guinney J. GSVA: gene set variation analysis for microarray and RNA-seq data. BMC Bioinformatics 2013;14:7. [Crossref] [PubMed]
- Jin S, Guerrero-Juarez CF, Zhang L, et al. Inference and analysis of cell-cell communication using CellChat. Nat Commun 2021;12:1088. [Crossref] [PubMed]
- Blakely CM, Pazarentzos E, Olivas V, et al. NF-κB-activating complex engaged in response to EGFR oncogene inhibition drives tumor cell survival and residual disease in lung cancer. Cell Rep 2015;11:98-110. [Crossref] [PubMed]
- Jiang H, Yu D, Yang P, et al. Revealing the transcriptional heterogeneity of organ-specific metastasis in human gastric cancer using single-cell RNA Sequencing. Clin Transl Med 2022;12:e730. [Crossref] [PubMed]
- Zhang X, Lan Y, Xu J, et al. CellMarker: a manually curated resource of cell markers in human and mouse. Nucleic Acids Res 2019;47:D721-8. [Crossref] [PubMed]
- Wang B, Zhang Y, Qing T, et al. Comprehensive analysis of metastatic gastric cancer tumour cells using single-cell RNA-seq. Sci Rep 2021;11:1141. [Crossref] [PubMed]
- Mansouri V, Rezaei Tavirani S, Zadeh-Esmaeel MM, et al. Comparative study of gastric cancer and chronic gastritis via network analysis. Gastroenterol Hepatol Bed Bench 2018;11:343-51. [PubMed]
- Zhao Z, Goldin L, Liu S, et al. Evolution of multiple cell clones over a 29-year period of a CLL patient. Nat Commun 2016;7:13765. [Crossref] [PubMed]
- He QF, Xu Y, Li J, et al. CD8+ T-cell exhaustion in cancer: mechanisms and new area for cancer immunotherapy. Brief Funct Genomics 2019;18:99-106. [Crossref] [PubMed]
- Cheung PF, Yang J, Fang R, et al. Progranulin mediates immune evasion of pancreatic ductal adenocarcinoma through regulation of MHCI expression. Nat Commun 2022;13:156. [Crossref] [PubMed]
- López-Soto A, Gonzalez S, Smyth MJ, et al. Control of Metastasis by NK Cells. Cancer Cell 2017;32:135-54. [Crossref] [PubMed]
- Liu F, Zheng JP, Wang L, et al. Activation of the NF-κB Pathway and Heterozygous Deletion of TNFAIP3 (A20) Confer Superior Survival in Extranodal Natural Killer/T-Cell Lymphoma, Nasal Type. Am J Clin Pathol 2019;152:243-52. [Crossref] [PubMed]
- Rock KL, Reits E, Neefjes J. Present Yourself! By MHC Class I and MHC Class II Molecules. Trends Immunol 2016;37:724-37. [Crossref] [PubMed]