Abstract
Effective monitoring and prediction of PM2.5 concentration is of great practical significance to People's Daily life and social development. In order to facilitate accurate prediction of the average daily concentration of PM2.5, a Feature Selection Algorithm Based on Pseudo F Statistic (FSPF) was proposed in this paper, and a PM2.5 concentration prediction model was combined with support vector regression. When constructing the model, all features are not selected into the model, and the pseudo-F statistic featu re selection algorithm is used to obtain more important feature variables related to PM2.5 concentration. On this basis, support vector regression based on different kernel functions is used to predict PM2.5 concentration. Through real data analysis, the hyperbolic tangent kernel support vector regression model (FSPF-SVR) selected by pseudo-F statistics features has the highest prediction accuracy when predicting the average PM2.5 concentration of Beijing in November and December 2017, with mean square errors of 1.441⋅10−3mg2/m6 and 1.307⋅10−3mg2/m6 respectively, which is better than the all-variable prediction model. At the same time, it is also better than random forest, weighted K nearest neighbor, Bagging regression, artificial neural network and other current mainstream machine learning regression prediction algorithms.
Export citation and abstract BibTeX RIS
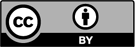
Content from this work may be used under the terms of the Creative Commons Attribution 3.0 licence. Any further distribution of this work must maintain attribution to the author(s) and the title of the work, journal citation and DOI.