Abstract
Complex systems in the real world often operate through multiple kinds of links connecting their constituents. In this paper we propose an opinion formation model under bounded confidence over multiplex networks, consisting of edges at different topological and temporal scales. We determine rigorously the critical confidence threshold by exploiting probability theory and network science when the nodes are arranged on the integers, , evolving in continuous time. It is found that the existence of 'multiplexity' impedes the convergence, and that working with the aggregated or summarized simplex network is inaccurate since it misses vital information. Analytical calculations are confirmed by extensive numerical simulations.
Export citation and abstract BibTeX RIS
1. Introduction
Much work has been done over the past few decades to probe varied social contagion processes underpinning voting behaviors as well as innovation diffusion [1–4]. At the heart of such processes are the opinion formation models characterizing a wide range of collective social phenomena, including adoption of a belief, collective decision making, minority opinion survival, emergence of extremism or fads, rise and fall of political parties, etc In a model of opinion formation, each agent holds an opinion which can change when she becomes aware of the opinions of her neighbors in a social network. According to whether the variable that represents the opinion of an agent is discrete or continuous, opinion formation models are classified into two types. In the discrete case, most established examples include the voter model [5], the Galam majority-rule model [6], and the Sznajd model [7]. A unifying frame for discrete opinion dynamics was proposed in [8]. Among continuous models, two models presented by Hegselmann and Krause [9, 10] (HK model) and Deffuant et al [11, 12] (Deffuant model) have raised significant attention. In these two models, each agent is only willing to adjust her opinion towards those opinions that differ less than a certain bound of confidence d from her own opinion. An excellent survey for such continuous opinion dynamics under bounded confidence can be found in [13].
The assumption of bounded confidence in opinion interaction may find its origin in the psychological concept of selective exposure, where people are inclined to perceive their environment in favor of their own opinions. In the initial studies of bounded confidence models, agents in a network are assumed to be homogeneous and have the same confidence level. For example, it was shown in [11, 14] that there exists a universal critical confidence threshold dc for the homogeneous Deffuant model, above which complete consensus is reached (namely, a single opinion cluster emerges) while below which opinions diverge (namely, two or more opinion clusters are observed) through simulations on diverse networks, be them complete graphs, lattices, or scale-free networks. Later on, the idea of agent-dependent confidence bounds is introduced to interpret the heterogeneity/disparity of people's knowledge, experience, and personality, etc [15–20]; agents are thereby divided into open-minded, closed-minded, and stubborn, according to their confidence levels. The effects of heterogeneous bounds of confidence were analyzed experimentally for a group of open-minded and closed-minded agents in both HK and Deffuant models [16]. Other more sophisticated models, for example, incorporating competitive influence of social media [21], have also been reported.
In all the aforementioned work, the opinion negotiation processes take place on networks containing edges of the same type and at the same temporal and topological scale. However, several papers showed that the presence of temporal co-evolving network structures can drastically change the convergence threshold; see e.g. [22–24]. Moreover, the real agents usually simultaneously engage in multiple types of interactions, which can make a non-additive effect on network dynamics [25]. People in a society, for example, interact through a variety of relationships: friendship, partnership, kinship, work-related acquaintanceship, collaboration and colocation, to name just a few. Admittedly, a natural and more appropriate description of such systems is in terms of multiplex networks [26–28], the networks made up of different layers containing the same nodes and a given type of edges in each layer (see figure 1 for an illustration). A considerable amount of effort has been devoted recently to the modeling and structure of multiplex networks in the sociophysics community, with the aim to understand emergent properties ranging from epidemics [29, 30] to cooperation [33], from diffusion [31, 32] to synchronization [34] to cascades [35, 36].
Figure 1. An example scenario of multiplex network as a duplex network consisting of layers. The two layers indicate two different types of intralayer connections, while the interlayer connections (dashed links) map each node to itself.
Download figure:
Standard image High-resolution imageThe goal of this paper is to investigate analytically the Deffuant model of opinion formation in multiplex networks. Due to the essential nonlinearity rooted in the action of confidence bound, the Deffuant model has mostly been studied using the tools borrowed from statistical physics and through agent-based simulations [1]. Remarkably, when the underlying interaction network is modeled as an infinite one-dimensional lattice, rigorous analyses based on probability theory have been performed and the critical confidence bounds have been identified by several mathematicians recently [37–40]. The current work can be viewed as an extension in this direction from a single-layer (or simplex) network to a multiplex one. In particular, we are able to identify the critical confidence threshold as a function of interaction rates and parameters capturing confidence bounds in multiple layers capitalizing on the marked Poisson process theory. Our results reveal that working with a simplex aggregated network, ignoring the existence of multiple edges in topological and temporal scales, is inaccurate. Similar observation that the aggregation procedure tends to lose important information has also been shared in recent studies of structure and dynamics of complex systems [29, 41, 42], highlighting the significance of exploring multilayer networks.
We mention that some work of bounded confidence model on multilayer networks has been done very recently. For example, in [21] the authors address the media competition and social influence issues by involving an inner dynamics of the multiple actors of social media. A coupled network of two layers is considered therein: one is the layer of gossipers and the other is that of media. Such networks are conventionally called as interdependent systems, which fall in a different category of ours (see [25] for more backgrounds). Another work similar in spirit is [43], where the effect of multiple communities on opinion formation is investigated. It is shown that increasing the multiple layers is similar to promoting coupling between different communities. Unfortunately, this model is not amenable to rigorous analytical treatment due to its involved community complications.
The remainder of the paper is organized as follows. In section 2, we introduce the opinion model on general multiplex networks. For clarity, we first focus on the case of one-dimensional duplex model and present our main results for the critical confidence threshold in section 3. The results are then extended in section 4 to the one-dimensional multiplex networks. Section 5 is devoted to numerical simulations illustrating the theoretical results. Finally, we conclude the paper in section 6 with some remarks.
2. Multiplex opinion dynamics model
Given an integer , a multiplex network is a pair
, made of ℓ layers
such that each layer is a simple graph
with node set V and edge set
(
). The edge set of G consists of ℓ types of edges:
. We assume that each layer Gi has bounded degrees, namely, the set of node degrees is bounded. Thus, each agent in the network G has a bounded number of neighbors and at most ℓ types of relationship.
In the Deffuant model, two agents compromise as per the following rules. Initially at time t = 0, each agent is assigned an opinion
uniformly distributed on the interval
independent of others. Independent of this, each edge
is independently assigned a Poisson process with rate (or intensity)
with
and
for
. We assume that
without loss of generality. Let Xt(u) represent the opinion value of agent u at time
, which remains unchanged as long as no Poisson event happens for any of the edges incident to u. Let
,
and
for
. (Here,
implies that
is the largest one in
after a possible normalization without loss of generality.) When at some time t the Poisson event occurs at edge
for some i, such that the pre-meeting opinions of the two agents are
and
, we set

and

where is the so-called convergence parameter. Hence, two meeting agents adjust their opinions by moving towards each other by a relative amount μ (
implies that the two agents meet halfway) if their difference of opinion is smaller in magnitude than a threshold. Note that our assumption of bounded degrees guarantees that none of the Poisson events will be simultaneous for any node set V, be it finite or infinite. Indeed, for a finite node set V, there will be only finitely many Poisson events in any finite time interval; while for an infinite V, the prevention of simultaneous events can be shown following a standard denseness argument (see [44, p 28]). Therefore, the model is well-defined.
The multiplexity in the above opinion model lies in two aspects. First, the interaction rates in each layer can be different. Second, the confidence bounds
in each layer can be different too. We might as well consider distinct convergence parameters, for example,
for the ith layer. However, it has been confirmed that μ plays no role in the qualitative behavior of the opinion dynamics; it only affects the convergence time (see e.g. [11, 13, 38]).
In what follows, we will consider the opinion model on the real line following [37–40]. More specifically, we take
with
and
for
. When
, G becomes a simplex network with only one type of edges. For this interaction network, the critical confidence threshold for opinion formation is identified to be
in [38]. This can be viewed as a special case of theorems 1 and 2 below.
3. Critical confidence bound in one-dimensional duplex networks
For simplicity, in this section we first present the results in the case of . Namely, we take
,
, and
. With these notations the main result concerning the critical confidence threshold for the one-dimensional duplex model reads as follows.
Theorem 1. Consider the above continuous opinion model on with parameters
,
, and
. Suppose
. Then
is the critical confidence threshold in the following sense
- If
, then with probability 1, the limiting value
exists and
for every
;
- If
, then with probability 1,
for every
.
Several remarks are in order. First, the result indicates that when , the opinions of all agents will converge to 1/2, the average of the initial opinion distribution. This reproduces the results for simplex network (i.e.,
) [37, 38], and also agrees with the results for general initial opinion distributions [39, 40]. Second, when
, the limiting configuration is piecewise constant interrupted by jumps of size at least d. This is nontrivial and essentially different from the situation in [45], where the random confidence bound D is set once and for all. In our setup, the two bounds d and
take effect alternatively as time t tends to infinity. Third, the assumption
is for technical reason (see the proof of proposition 3), which does not have counterpart in the case of simplex network where μ only influences the convergence time of negotiation process. It would be interesting to sort out whether this condition can be dropped, which is the case suggested by our numerical simulations.
In the following we show theorem 1 in two regimes and
separately. Our proof heavily relies on the technique called sharing a drink (SAD) proposed in [38]. We briefly review the SAD process in the appendix for completeness.
3.1. Subcritical regime
Given and the initial opinion configuration
, a node
is said to be an
-flat point to the right if for all
,

By analogy, is said to be an
-flat point to the left if for all
,

and two-sidedly -flat if for all
,

If u is -flat to the right, the Kolmogorov strong law of large numbers indicates that
Employing the translation invariance of the configuration
and a coupling argument, we can show that all the following three events occur with positive probability; see [38].
Define a Boolean random variable A by A = 1 with probability p and with probability
. It follows from the decomposition of the Poisson stream that the Poisson events in our opinion model constitute a marked Poisson process with rate λ. Recall that a marked Poisson process
is composed of a Poisson stream
and a sequence of associate random variables
[46]. (More precisely, we should write At explicitly as a function of t when a Poisson event occurs at time t. We can safely omit t by using an independent coupling when necessary.) Suppose that
. Take
. For
, define the following events
,
,
,
, and
.
Proposition 1. Under the assumption of theorem 1, if , then for any
,
.
Proof. For , define
. It follows from lemma 1 that
The proposition will follow if we can show that

Suppose that and
hold. Let
be the first time that opinion adjustment happens across any of the four edges
and
(i = 1,2). Obviously,
for any
. We will show by contradiction that T does not exist. Indeed, on one hand, there must exist some
such that either (i)
, or (ii)
takes place.
On the other hand, for any we obtain from lemma A2, by replacing 0 with
using translation invariance,
and
for all
. Lemma A1 gives
for some integer N with
. Set
for
. Arguing as in [38, equations (19) and (20)], we have
and

Since holds, it follows that

Similarly, . These contradict with (i) and (ii). Hence,
is concluded.
The events and
in conjunction with (4) yield, for all
,

and holds. Hence, (3) is established, which completes the proof.
Due to the ergodicity of the indicator processes and
, we are led to the following corollary (see [47, theorem 1.3] and [38, lemma 5.2]).
Corollary 1. With probability 1, there are infinitely many nodes u to the left (and right) of 0 such that happens. The same thing holds for
.
Proposition 2. Under the assumption of theorem 1, if , then with probability 1 the limiting value
exists and
for all
.
Proof. Given the initial opinion configuration , let u1 be a node such that
happens, and let
. It follows from (3) that the opinions in the interval
will not be influenced by nodes outside and vice versa. Corollary 1 indicates that every node
is located in some such interval. Therefore, it suffices to prove the proposition for every
.
Define the energy of the interval at time t as

If two nodes u and in the interval exchange opinions at time t,
drops by an amount of
. Therefore,
is a decreasing function of t. We claim that

Indeed, the maximum value in (5) should be larger than some for all sufficiently large t if (5) is not true. But for such large t that a Poisson event occurs, with probability at least
some edge
with
will exchange opinions. Then,
will decrease by at least
, and this will happen infinitely often almost surely in view of the conditional version of the Borel–Cantelli lemma [47, corollary 3.2]. This yields
, a contradiction, which establishes (5).
For any edge in the interval
, a single opinion adjustment can only increase
by at most
. In the light of (5), we have either
for all sufficiently large t or
. To see this, just note that otherwise there would exist some
so that
jumps back and forth between
and
infinitely often for arbitrarily small σ. However,
for small σ, which leads to a contradiction.
Now, arguing as in [38, theorem 5.3], the fact that the quantity remains unchanged over time readily implies the existence of
. The proof is complete.
3.2. Supercritical regime
The following result informs us what values can be achieved for a two-sidedly flat node regardless of all future Poisson events. It can be proved exactly as [33, lemma 6.3].
Lemma 2. Given and the initial configuration
. If
is two-sidedly
-flat, then
for all
.
Define the energy at node as
. Define an auxiliary continuous-time step function
by
with
increasing by an amount of
if and only if opinion exchange occurs on the edge
at time t. The following lemma can be shown exactly as [38, lemma 6.2].

In other words, the expected sum of is constant over time. The following proposition holds for all
.
Proposition 3. With probability 1, for any , either
for all sufficiently large t, or
.
Proof. Given a node and a sufficiently small σ with
, we claim that, with probability 1,

for all sufficiently large t.
Indeed, when a Poisson event occurs on any of the edges ,
or
, the next Poisson event will occur at
with probability 1/3. If
, then
will increase at least
if a Poisson event occurs at
. In view of the conditional Borel–Cantelli lemma as before, this will happen infinitely often with probability 1. Hence,
, contradicting lemma 3. Equation (6) is established.
What remains to show is that for small enough ,
cannot jump back and forth between
and
infinitely often. This is true because a single Poisson event cannot increase
by more than
, which for small enough σ, is always less than the span of the gap
that needs to be crossed since
.
Proposition 4. Under the assumptions of theorem 1, if , then with probability 1,
for every
.
Proof. Choose an such that
. We first show that with probability 1,

for any . In the light of proposition 3, we only need to show that for each node u,

Suppose for contradiction that the probability in (8) is strictly positive. Then the event in (8) happens for infinitely many u on and
with probability 1 by using the ergodicity again. We will show that the limit

exists for any .
Fix a node . Note that we can always pick two nodes
with
such that
are the nodes locating between edges
and
which never exchange opinions for any
with some
. To show (9) we consider two scenarios: (a) no edge
in
gets stuck with the event
happening for any
; and (b) some edge
in
gets stuck with this event at some time
. If (a) happens, we obtain
for all v in this interval by proposition 3. Since
remains constant over time,
must all converge to the average value
. If (b) happens, u will still belong to some subinterval
such that
for all v in this subinterval and that no opinion adjustment will occur on
and
from some time
onwards. Here we allow that
. Likewise, we obtain that the opinion of any node in the subinterval converges to the average value
. Equation (9) is established.
Lemma 1 plus ergodicity implies that with probability 1 there exists a node w which is two-sidedly -flat. We know from (9) and lemma 2 that
. Proposition 3 implies either
or
. However, the first option leads to (i)
, or (ii)
. Since
, it follows from (i) that the event
happens with positive probability. This of course is impossible. Likewise, (ii) gives rise to the event
happening with positive probability, which is impossible either. Consequently, we deduce that
. In an iterative manner, we have
for all
. This, however, contradicts the assumption that the event in (8) holds with positive probability. Therefore, (8) is proved and (7) follows.
For the node w chosen above, we obtain by using lemma 2 that for all
. For any
, we obtain with probability 1 that
for sufficiently large t invoking (7), as there are only finitely many edges between u and w. We conclude the proof by taking
.
Combining propositions 2 and 4 we finally complete the proof of theorem 1.
4. Generalization to multiplex networks
The techniques developed in the previous section can be used to cope with a more general interaction network, a multiplex network with ℓ layers. The following theorem can be proved in the same way as in the previous section but the details are left to the reader.
Theorem 2. Consider the continuous opinion model on with parameters
,
,
for
,
for
, and
. Suppose
,
. Then
is the critical confidence threshold in the following sense
- If
, then with probability 1, the limiting value
exists and
for every
;
- If
, then with probability 1,
for every
.
We give a couple of comments here. First, recall that we assume , and therefore
unless the underlying network is simplex, i.e.,
. This suggests that it is more difficult to reach agreement in multiplex networks, going some way to explaining the commonly seen nonconsensus or polarization in real-life social systems [48]. This also seems to resonate with the recent finding in [36] that response heterogeneity in multiplex networks may slow down the cascade progress.
Second, if there exists some k such that for all
, then
. This means that, if a frequently interaction layer exists, the critical threshold, in line with intuition, will be dominated by its confidence bound. Third, if we consider an aggregated simplex network based on average confidence bound, we would have
, which is not equivalent to dc in general. Indeed, the aggregation procedure missed important information in inherent multiplex systems leading to inaccurate results.
5. Simulation results
In this section, we conduct agent-based simulations on large rings to illustrate and test our obtained theoretical results.
To start with, we show the time evolution of opinions among a population of n = 1000 agents distributed on a duplex ring, namely, a ring with perimeter 1000 and layers. Take
,
,
, and p = 0.8. By theorem 1, we deduce the critical threshold dc = 0.54. The opinion dynamics is performed by monitoring 2000 independent Poisson processes, each of which determines the meeting time of a pair of agents as described in the model. For the sake of clarity, we plot in figure 2 the opinion behavior of a randomly selected subset of 100 agents, and discretize the time axis by compressing 40000 times of Poisson jumps into one time unit.
Figure 2. Evolution of opinions with ,
,
, p = 0.8 on a duplex ring consisting of n = 1000 agents. Only a subset of 100 agents out of 1000 is displayed. Each opinion is represented by a diamond. Each plot corresponds to one realization of the simulation.
Download figure:
Standard image High-resolution imageFrom figure 2 we observe that the opinions converge to 1/2, the average of the initial opinions, when (see figure 2(a)); while they diverge when
with a gap of opinion clusters at least d (see figure 2(b)). These are in line with our theoretical predictions of theorem 1. We performed extensive tests by using different parameters α and p, and different size n of nodes varying from 1000 to 5000, and they confirm the theoretical results. Here, we mention that the shape of the envelop of opinions' trajectories has a relatively large change from one simulation to another. It is sensitive to the initial opinion distribution and the underpinning Poisson process.
Next, we determine the critical confidence bound dc through Monte Carlo simulations. Choose ,
and
as above. Given parameters α, p and the number n of agents, we run the duplex opinion model algorithm on 1000 samples. The algorithm proceeds until no agent changes her opinion by more than 10−4 for 106 times of consecutive Poisson events. We qualify this regime as quasi-steady state. Once the system evolves into the quasi-steady state, we examine whether all agents belong to the same cluster or not. Define Pc as the fraction of samples which reaches a compete consensus at one-half. In figure 3 we show Pc as a function of confidence bound d for different α, p and n.
Figure 3. Fraction of samples with complete consensus as a function of confidence bound d in a quasi-steady state regime. Three different numbers of individuals located on rings are considered: n = 5000 (red squares), 10 000 (blue circles), and 20 000 (green triangles). We fix ,
and
. (a)
; (b)
; (c)
. The critical threshold dc is indicated by a vertical dotted line in each plot.
Download figure:
Standard image High-resolution imageWe observe from figure 3 that Pc increases gradually for and then saturated to 1 for
in each plots. A further look into the growth of Pc for different population size n shows that it will converge to a step function in the large size limit
, implying a folding point at dc. This is in line with our theorem 1. Analogous qualitative results for simplex rings have been reported previously in [39]. Moreover, comparing figures 3(a), (b), and (c), we find that the sharpness of phase transition increases with respect to α but remains insensitive to p; for example, the increase of Pc from 0 to 1 in the case of n = 20 000 requires a raise of d by around 0.025 for
(figure 3(a)) while only around 0.015 for
(figure 3(c)). We contend that this correlation may find its origin in the higher level of homophily resulting from the more homogeneous confidence bounds, namely, larger α, as observed in wealthy social behaviors [45, 49].
Finally, we perform extensive simulations to examine the relationship between the critical confidence bound and the number of layers. Recall the comment below theorem 2 that the critical value as long as
. In figure 4 we pictorially show the evolution of dc with respect to the number of layers ℓ. We consider multiplex rings with perimeter
and
. Fix
and
. For each given ℓ and a confidence bound d, we run the multiplex opinion model algorithm with the following parameters
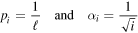
for . We first put
and run the algorithm until the system enters the quasi-steady state. Repeat this process for 1000 times to obtain the fraction of complete consensus Pc as before. We then start over by increasing d gradually and repeating the entire process until
. The corresponding value of d is regarded as dc.
Figure 4. The critical confidence bound dc as a function of the number ℓ of layers. Theoretical estimates (green crosses) are derived from theorem 2. The green line is drawn to aid the eye. Numerical results (upper and lower red bars) indicate the ranges of dc obtained from an ensemble of 100 independent samples.
Download figure:
Standard image High-resolution imageAccording to our choice of parameters, all the conditions in theorem 2 are satisfied. The simulation results reported in figure 4 agree with the prediction of dc in theorem 2. With the growth of ℓ, the critical threshold dc also increases, implying, as expected, a tougher situation for reaching consensus. For example, for a randomly selected , the possibility of consensus for
is almost cut in half as compared to that for
.
6. Conclusion
In this paper, we have studied a continuous opinion dynamics model of Deffuant et al under bound confidence. The underlying interaction network is modeled by an one-dimensional multiplex network, , with arbitrary ℓ layers. Based on the SAD process and probability theory, we obtained analytically the critical threshold dc for the opinion consensus, extending the previous results for simplex networks, i.e., the case of
. Our key findings, that the increase of 'multiplexity' hinders the opinion formation and the aggregated simplex network loses essential information, suggests a vital topological factor in opinion formation and further deepens our understanding of the multilayer impact on network dynamics. Our theoretical derivations are confirmed by extensive numerical simulations.
For future research, we note that the question put forward in [38] regarding the critical case remains open. Moreover, we believe it is hopeful to deal with the higher-dimensional lattice
for
by extending the framework developed here. However, the case of D = 1 with one or two boundaries, e.g.,
or
, may pose much greater challenges with exact confidence threshold being difficult to obtain. Towards more realistic network structure of populations, such as random networks and scale-free networks, a plausible intermediate step is to consider tree networks (Notice that the key SAD process has been studied in the case of Cayley tree in [39]).
Acknowledgments
This work has been supported by the Program for Young Excellent Talents in Tongji University (2014KJ036) and by Shanghai Pujiang Program (15PJ1408300). The author would like to thank the anonymous referees and the editor who provided valuable comments and advices resulting in improvements of this paper.
Appendix
In the appendix, we review the SAD process proposed in [38], which turns out to be closely related with the Deffuant model on .
Let be an integer. The SAD process, denoted by
, is a deterministic process defined iteratively as follows. Set

For a given sequence of nodes and
, we obtain the configuration
for
by setting

This procedure can be delineated as a liquid exchanging process on . A glass is put at each site
. At k = 0 only the glass located at the origin is full (signified by 1) while all others are empty (signified by 0). At each subsequent step k, one picks two neighboring glasses at uk and
, and pouring liquids from the glass with higher level to that with lower level by a relative amount μ. This leads to the SAD process. The following lemma can be easily shown.
Fix and consider our opinion model on
. Note that there exists a finite interval
containing 0 such that the Poisson events on the boundary edges
and
for all
have not happened yet up to time t. Let N be the number of opinion adjustments occur in
up to time t. The times of these adjustments are arranged in the chronological order as

where we set for convenience. For
, we write uk as the left endpoint of the edge
for which uk and
adjust opinions at time
. Given the sequence
(in this order) and
, we obtain a SAD process
as defined by (10) and (11).

In particular, .
Lemma A2 can be proved through induction over k similarly as [38, lemma 3.1]. It unveils that the opinion at the origin can be expressed as a linear combination of initial opinions over nodes of , with coefficients given by the associated SAD process.