Abstract
A large number of studies have been published examining the implications of climate change for agricultural productivity that, broadly speaking, can be divided into process-based modeling and statistical approaches. Despite a general perception that results from these methods differ substantially, there have been few direct comparisons. Here we use a data-base of yield impact studies compiled for the IPCC Fifth Assessment Report (Porter et al 2014) to systematically compare results from process-based and empirical studies. Controlling for differences in representation of CO2 fertilization between the two methods, we find little evidence for differences in the yield response to warming. The magnitude of CO2 fertilization is instead a much larger source of uncertainty. Based on this set of impact results, we find a very limited potential for on-farm adaptation to reduce yield impacts. We use the Global Trade Analysis Project (GTAP) global economic model to estimate welfare consequences of yield changes and find negligible welfare changes for warming of 1 °C–2 °C if CO2 fertilization is included and large negative effects on welfare without CO2. Uncertainty bounds on welfare changes are highly asymmetric, showing substantial probability of large declines in welfare for warming of 2 °C–3 °C even including the CO2 fertilization effect.
Export citation and abstract BibTeX RIS
Introduction
There is now a large literature examining the impact of climate change on agricultural yields that can be divided into two types of studies. One category uses process-based crop models that simulate the biological mechanisms of crop growth. A second category that has been developed more recently looks at statistical relationships between climate or weather and crop yields. The benefits of the former are that it is grounded in a mechanistic, bottom-up understanding of how plants grow. But process-based models are often calibrated to specific field settings which can be data intensive and means the generalizability of results to larger areas is unclear. Statistical models are typically based on observations of crop growth over large areas in real-world field settings. But the reduced-form relationship between weather variables and yield means the mechanisms driving model results are often unclear and that care should therefore be taken in using results for climate change projections extending beyond the historical record.
Despite the fact that both approaches seek to quantify the impacts of climate change on agricultural productivity, there have been relatively few attempts to systematically compare findings. A number of studies have compared process-based and empirical responses for individual crops in individual locations, such as maize and wheat in South Africa (Estes et al 2013) or maize in Switzerland (Holzkamper et al 2015). At the global level, Liu et al (2016) provide a systematic comparison of the temperature response of wheat yields estimated from regression models, upscaling point estimates from an ensemble of process-based models, and gridded process-based models, generally finding only small differences between the three methods. In this special issue, Lobell and Asseng (2017) compare individual published estimates of crop sensitivities to climate impacts from process-based and empirical yield models, finding little difference in the temperature response. Roudier et al (2011), Knox et al (2012), and Knox et al (2016) are perhaps most similar to the analysis presented here. These papers present meta-analyses of yield impacts for multiple crops in specific regions (West Africa, Africa and South Asia, and Europe respectively) and report average differences between process-based and empirical estimates. All papers find that the effect of impact estimation technique is small relative to other sources of variation. Our approach adds to these studies firstly by performing a global analysis (thereby giving us a larger set of data-points to work from) and secondly by using a multivariate regression for our meta-analysis, instead of simply splitting the sample of studies. This multivariate approach allows us both to control for potential confounding variables and to estimate continuous response functions that can be globally extrapolated in order to inform an economic analysis.
In the agricultural sector, the effect of climate change-induced yield shocks on more policy-relevant variables such as prices, consumption, food-security, and economic welfare will be mediated by the global trade in agricultural commodities and will depend on terms-of-trade effects and the interaction of climate change impacts with existing market distortions (Hertel and Randhir 2000). Though several papers have incorporated climate productivity shocks into partial- and general-equilibrium models (Nelson et al 2014b, 2014a, Wiebe et al 2015, Stevanović et al 2017) very few report welfare changes (Stevanović et al 2016 is an exception). Understanding the welfare impacts of climate change-induced productivity shocks on agriculture is important both for policy and because the simple integrated assessment models (IAMs) used to calculate the social cost of carbon (SCC) use damage functions that parameterize changes in economic welfare with temperature. Current agricultural damage functions in these IAMs use studies from the early-to-mid 1990s that are now dated and largely obsolete (NAS 2017, Rose et al 2014).
This paper contributes to the existing literature in two ways. It is the first systematic, multi-crop, global comparison between empirical and process-based crop models. Although the distinction between these approaches has been widely discussed, this paper quantifies this difference at the global scale and puts it in the context of other uncertainties in future climate change impacts, such as how quickly farmers are able to adapt to climate change. In addition, we advance the literature by examining not just yields but also economic welfare by incorporating estimated yield changes into the Global Trade Analysis Project (GTAP) CGE model, thereby producing results ready to inform IAM damage functions.
Data and methods
The basis of the yield-temperature response functions in this paper is a database of studies estimating the climate change impact on yield compiled for the IPCC 5th Assessment Report (Porter et al 2014), also described in a meta-analysis by Challinor et al (2014). This database contains over 1700 point estimates of the impacts of changes in temperature, rainfall, and CO2 concentrations on the yield of 17 different crops compiled from 94 different studies. These studies include a wide range of process-based crop models as well as empirical papers, published between the late 1990s and 2012, and they vary substantially in the geographic regions examined as well as the extent to which they include on-farm adaptations. In this paper we focus on maize, rice, soy and wheat, four crops that make up a major part of the scientific literature on climate impacts on crops. These crops collectively account for approximately 20% of the value of global agricultural production, 65% of harvested crop area, and just under 50% of calories directly consumed (FAO 2016). For these four crops, the database contains 1010 data-points (344, 238, 336, and 92, for maize, rice, wheat and soybeans respectively). Of these, 451 are reported as including some form of on-farm, within-crop agronomic adaptation. The majority of these adaptations involve adjusting either planting date (10%), cultivar (12%) or both (44%). In total, 28 models are represented in the 56 studies used for the estimation, made up of 17 process-based model families5 and 11 statistical models.
For this analysis we have complemented the existing database in two ways. Firstly, we coded each study based on whether a process-based or empirical approach was used. Secondly we added baseline growing-season temperatures to the database. To do this, each data point was assigned to a country. 86% of points come from studies located in a single country. For the remaining 14% coming from studies with an international scope the assigned country was the country with the highest production of the relevant crop. Average growing season temperatures were calculated using planting and harvest dates from Sacks et al (2010) and gridded monthly temperature from the Climate Research Unit (CRU 2016).
We treat the database of studies as a kind of 'ensemble of opportunity' (Stone et al 2007, Tebaldi and Knutti 2007). The benefits of this approach are that predictions from multi-model ensembles have been shown to consistently out-perform individual models in both climate modeling and, increasingly, in agricultural modeling (Asseng et al 2014, Bassu et al 2014, Li et al 2015). Though not derived from a formal ensemble modeling project, the universe of individual studies contained in the database can be thought of as draws from a set of possible models, each of which captures the response of crops to changing climate conditions with some error. The sampling of models is not systematic or random, but instead has emerged from scientific research and associated peer review of work on climate effects on crop yields over the last two decades. A difficult question in estimating multi-model ensembles is always how to weight different models (Tebaldi and Knutti 2007). The approach described here takes advantage of an implicit weighting derived from representation of models within the scientific literature. To the extent that this representation reflects researchers' judgements about the best model to use for particular crops in particular locations, it may be that this implicit weighting emerging from the scientific literature is preferred to simpler one-model one-vote aggregation schemes (for instance Asseng et al 2014).
We use multiple regression to aggregate the results from individual studies to an ensemble average response function. This approach allows us to estimate common response functions at an appropriate level of aggregation. For example, every study in the database examines the effect of change in temperatures on yields, allowing us to estimate separate yield response functions by crop and by baseline temperature, as well as by type of study. Fewer studies examine the effect of CO2 fertilization or adaptation, limiting our ability to model heterogeneity in response to changes in these variables.
Our regression specification is:
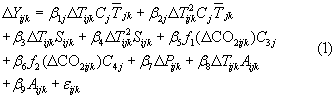
where ΔYijk is the change in yield from data-points for crop j in country k (in % relative to the 1995–2005 baseline)6. ΔTijk, ΔCO2ijk and ΔPijk are the changes in temperature (in degrees C), CO2 concentration (in parts per million (ppm)) and rainfall (in percent) for point-estimate ijk, Sijk is a dummy variable indicating whether point-estimate ijk came from a statistical study, and Aijk is a dummy variable indicating whether authors report the point-estimate as incorporating any on-farm adaptation. Cj is a dummy variable taking the value 1 if the point-estimate is for crop j and 0 otherwise and is the baseline growing-season temperature for crop j in country k. C3j and C3j are dummy variables indicating whether crop j is a C3 or C4 crop. The β parameters in equation (1) are estimated using OLS regression. εijk gives the residual variability in yield changes in the database unexplained by the variables in equation (1). We allow for correlations between data-points from the same study by estimating standard errors using 750 block bootstraps, blocking at the study level. In results that follow, error bars show the 95% confidence interval based on these block bootstraps. This regression model has an adjusted R2 of 39%. One thousand leave-one-out cross validations gave a median root mean squared error of 9.9% (95% CI: 0.4% to 39.1%). For comparison, the standard deviation of the dependent variable is 20.1%.
This specification allows estimation of different quadratic response functions for each crop and for the effect of warming to differ according to baseline temperature. But the estimated effect of statistical as opposed to process-based modeling (β3 and β4 in equation (1)) is common across crops. We are limited in our ability to model heterogeneity in the effect of statistical modeling due to limited representation of statistical studies in the database. Figure 1 shows the number of observations by crop, country, and level of warming coming from process-based and empirical papers. There are approximately ten times more observations from process-based models than from empirical models. Moreover, observations from empirical papers are tightly clustered around 1 °C warming with very few studies reporting results for 2 °C warming or more. This is because while statistical studies estimate a continuous response function between yield and temperature, they frequently only report the marginal effect of a 1° increase in temperature, even when the estimated response function is non-linear. Results from process-based studies are much more widely dispersed between 1 and 5 °C.
Figure 1 (a) Maps of the distribution of individual data-points included in the yield impacts database by crop and geographic region. (b) Distribution of individual data-points by level of warming.
Download figure:
Standard image High-resolution imageThe effect of CO2 on crop yields is modelled as a concave function, allowing for a declining marginal effect of CO2, consistent with a number of field studies (Long et al 2006, Tubiello et al 2007). Specifically the function takes the form where A is a free parameter that is allowed to differ between C3 and C4 crops. It is set at 100 ppm for C3 and 50 ppm for C4 based on a comparison of the R2 across models using multiple possible values. The changes in CO2 are adjusted so that all are relative to a modern baseline of 360 ppm (the most common baseline value for studies included in the analysis).
The effect of adaptation on crop yields is modelled with both an intercept term (β9 and an interaction with warming (β8). This is prompted by the observation that in many studies that report including on-farm agronomic adaptations, adaptation is represented by changing management practices that would improve yields even in the current climate (Lobell 2014). Failing to include an adaptation intercept in this context will lead to an over-estimation of the potential of the adaptation actions included in these studies to reduce the negative impacts of a warming climate. We therefore include an adaptation intercept in the estimating equation but then subtract it out to produce a damage function that goes through the origin7. The true effect of adaptation is captured by the interaction with temperature change, given by the β8 term in equation (1). This term reflects the effect of management changes that are not beneficial today but that will be beneficial under a changed climate, the standard definition of adaptation8.
To estimate the impact of local warming for a pre-specified increase in global mean temperature we use pattern-scaling between local and global temperature changes based on the CMIP5 multi-model mean for RCP8.5 (Taylor et al 2012). The multi-model mean was calculated using the Climate Explorer tool (KNMI 2015) using methods documented in van Oldenborg et al (2013). For each grid cell we take the change in temperature between a future (2035–2065) and baseline (1861–1900) period and divide by the mean global warming over this time period. Local warming is greater than global average warming over land areas and is larger at high latitudes and in continental interiors. Gridded local temperature changes are combined with the response functions estimated using equation (1) and baseline growing season temperatures based on CRU (2016) and Sacks et al (2010) to give projected changes in yield with warming on a 0.5 degree grid. Global average yield changes are calculated by production-weighting the gridded data using production data for the relevant crop in the year 2000 (Monfreda et al 2008).
In presenting results, we focus on global temperature changes ranging from 1 to 3 °C and use 4 cases to examine the importance of different variables. The reference case is based on the temperature response curves for process-based models, including CO2 and adaptation. The 'No CO2' case is the same as the reference except without CO2 fertilization. 'No Adaptation' is the same as the reference except excluding adaptation. And finally, the 'Statistical' case is the same as the reference (i.e. includes CO2 fertilization and adaptation) except the temperature response comes from statistical studies.
In order to assess the economic implications of alternative studies of climate impacts on crop yields, we use the Global Trade Analysis Project (GTAP) model (Hertel, 1997). GTAP is a global, computable general equilibrium model which seeks to predict changes in bilateral trade flows, production, consumption, intermediate use and welfare, owing to changes in technology, policies or other exogenous shocks. In this case, we treat the climate-induced yield changes as Hicks-neutral productivity changes. Thus, a 10% yield loss would mean that, if farmers did not alter their practices in the face of the changing climate, application of the same inputs to the same amount of land would result in 10% less output. The economic model does allow for changes in area planted, as well as input intensities, in response to changing relative prices, so actual yields will not change by 10%. In this sense, all of the economic results reported here allow for economic adaptation (table S1).
In order to implement the yield shocks under the different climate scenarios, we aggregate the gridded impacts for each of the four crops to the level of the 140 countries/regions in the version 9 GTAP data base (Aguiar et al 2016). Since maize and soybeans are part of larger crop aggregates in the GTAP data base (coarse grains in the case of maize and oilseeds in the case of soybeans), the climate-induced yield shocks are diluted by multiplying them by the share of the country-commodity aggregate made up of maize and soybeans, respectively. Thus in a region which does not produce soybeans, the climate shock would be zero, whereas a country in which maize was the only coarse grain produced would experience precisely the productivity shock specified by the aggregated maize results for that geographic region. To be consistent with these incomplete agricultural yield shocks, when it comes to reporting the welfare losses, we report the losses as a share of that incomplete production value (i.e. change in welfare/sum of rice, maize, wheat and soybean output values at 2001 prices).
Results
Figure 2 shows the temperature response functions estimated from equation (1). Warming has a negative impact on yields that is worse for maize and wheat than for the more heat-tolerant rice. It is striking that we find very little evidence for any yield benefits from warming over most growing areas—our point estimates are negative even for warming less than 1 °C and even in the 25th percentile of growing season temperatures (i.e. relatively cool areas). This negative impact is generally statistically significant for process-based model results at warming above 2 °C. Standard errors for results from statistical models are much larger and bracket zero in almost all cases. The interaction with baseline temperature is in the expected direction: warming is less damaging for crops in cooler locations. Figure S1 shows the gridded yield responses to 2 °C of global average warming for each crop. While many areas see negative impacts, there are some positive effects in the boreal zone and in cooler temperate areas.
Figure 2 Yield-temperature response functions derived from process-based and statistical models for maize (a), rice (b), soybeans (c) and wheat (d) estimated using equation (1). Response curves are plotted for three baseline growing season temperatures based on the 25th, 50th and 75th percentile of temperatures for regions represented in the yield impacts database. In each graph the lightest color corresponds to the coolest baseline temperature and the darkest color to the warmest. Dashed lines show the 95% confidence intervals based on 750 block bootstraps, blocking at the study level. For the GTAP analysis, any grid cell with more than 99% yield loss was set to 99% yield loss. Temperature changes are relative to a 1995–2005 baseline.
Download figure:
Standard image High-resolution imageIn figure S2 we show a comparison between our estimated response to a 1 °C warming and the mean of multiple process-based crop models calibrated to specific locations as part of the Agricultural Modeling Inter-comparison and Improvement Project (AgMIP) for the three crops that are available: maize (Bassu et al 2014), wheat (Asseng et al 2013), and rice (Li et al 2015). The results from these two very different methods are close for both wheat and maize while the findings for rice show more variability. In all cases the AgMIP data are well within the 95% confidence interval produced in this study.
Figure 3 shows how the type of study (β3 and β4 in equation (1)), inclusion of adaptation (β8), and the CO2 fertilization effect (β5 and β6) affect the climate change response. Point estimates for β3 and β4 suggest that on average results from statistical studies are slightly more optimistic than results from process-based models for small amounts of warming and more pessimistic for higher levels of warming. Error bars for the statistical model are extremely large though, particularly for warming beyond 2 °C, which is perhaps unsurprising given the concentration of empirical results at 1° warming documented in figure 1.
Figure 3 Effects of type of study, inclusion of adaptation, and CO2 fertilization. Dashed lines show the 95% confidence intervals based on 750 block bootstraps, blocking at the study level. Temperature changes are relative to a 1995–2005 baseline.
Download figure:
Standard image High-resolution imageThe point estimate for the effect of adaptation is in the opposite direction from what would be expected (slightly negative), but the error bars are large and the effect is not distinguishable from zero. Studies that include adaptation do have more positive yield outcomes than studies that don't, but those benefits are captured entirely in the adaptation intercept term (β9 = 7.05%, p > 0.05). Therefore, these findings suggest that most within-crop agronomic adaptation measures represented in process-based modeling studies would provide the same benefit under the current climate as they would under future climates. In other words, they are actions that shift the supply curve out to the right but do not change the marginal impacts of future warming, consistent with the 'adaptation illusion' identified by Lobell (2014).
Finally, the CO2 response functions show statistically-significant benefits of CO2 fertilization that asymptote at 17.3% for C3 crops and 10.6% for C4 crops. Given the functional form assumption, this translates to yield gains of 11.5% (C3) and 8.5% (C4) for a doubling of CO2 from pre-industrial levels. For C3 crops, this value is close to that obtained from FACE experiments which range between 12 and 14% yield gains for a doubling of CO2 (Long et al 2006). (It may well be that some process-based models have used FACE data for calibration of the CO2 fertilization effect (particularly those published more recently) and therefore this may not be a fully independent validation of the meta-analysis results.) Fewer FACE experiments have been performed for C4 crops but available experimental data, as well as theory, suggest C4 crops will benefit less from CO2 except under water-stressed conditions (Leakey et al 2006, Long et al 2006)9.
Figure 4 shows global production-weighted yield losses for a global temperature change of 1 °C–3 °C for four cases. Except for soybeans, the reference case that includes CO2 fertilization and adaptation shows positive effects on yields at low levels of warming, becoming negative between 2 °C–3 °C of warming. Variation between the different cases reflects what might be expected given the response curves shown in figure 3. CO2 fertilization is most important– excluding the CO2 effect produces substantial losses for all crops ranging from 14% (rice) to 25% (wheat) at 3 °C of warming. The effect of excluding adaptation is very small. The effect of statistical as opposed to process-based studies is small and slightly positive for 1 °C–2 °C, becoming slightly negative at 3 °C. At higher levels of temperature change we would expect this effect to become more negative (figure 3). The 95% confidence intervals are large and mostly bracket zero, with the exception of the No CO2 case at 3 °C of global average warming. Uncertainties are particularly large for soybeans and for the statistical case at 3 °C of warming—both instances where the number of data-points in the meta-analysis are limited.
Figure 4 Global production-weighted average yield changes for global temperature change of 1 °C–3 °C for four different cases. The reference case is for response functions derived from process-based models including both adaptation and the CO2 fertilization effect. Temperature changes are relative to a 1995–2005 baseline.
Download figure:
Standard image High-resolution imageWe use our estimates of the response of global crop productivity to temperature change as an input to the GTAP CGE model in order to determine how national economic welfare is affected by climate impacts on crop yields. Figure 5 gives the global damage functions (% change in welfare with warming) within the impacted sectors. Among all the cases that include CO2 fertilization, welfare changes are negligible at 1–2 degrees of warming, becoming negative at 3 degrees. In contrast, the No-CO2 case shows substantial global welfare losses, even at 1–2 degrees. Uncertainty bounds are large meaning no cases are statistically different from the reference case at the 95% confidence level. But the uncertainty in potential yield losses is also highly asymmetric: the possibility of large welfare losses is substantial whereas welfare gains are both smaller and less likely, particularly for a warming of 2–3 degrees. These welfare changes depend on modeled changes in harvested crop areas, production intensity, and consumption. Some of these changes are shown in figures S3 and S4 and are discussed more extensively in the supplemental information available at stacks.iop.org/ERL/12/065008/mmedia.
Figure 5 Change in welfare measured in equivalent variation for the four cases and temperature change of 1–3 degrees. Welfare changes are normalized by output value in the affected sectors to give % change. Error bars show welfare changes based on using the upper or lower bounds of the 95% confidence interval of yield changes. Temperature changes are relative to a 1995–2005 baseline.
Download figure:
Standard image High-resolution imageDiscussion and conclusions
We believe there is a general perception that empirical studies give more pessimistic estimates of crop response to warming than do process-based models (Lobell and Asseng 2017). However, there is a lack of systematic comparisons between the two methods. In particular, because empirical studies do not include CO2 fertilization whereas process-based studies generally do, it is important to account for this difference in comparing the temperature response from the two methods. Here we are able to do this statistically, showing that once CO2 (and to a lesser extent adaptation) are controlled for, differences between empirical and process-based responses may be smaller than generally believed. Though the point-estimates do show some evidence of more negative impacts from statistical studies at higher temperatures (4–5°), the effect is not precisely estimated and error bars are large.
The poor representation of empirical studies within the yield impacts database, particularly at higher levels of warming, is a major limitation of this analysis. Inclusion of more recent studies would help with this, but this is not always straightforward. Many recent papers report the marginal effect of growing degree days rather than average growing season temperature and converting from one to the other is not simple (for example, Burke and Emerick 2016, Butler and Huybers 2012). Standardized reporting of the impacts of a 1 °C increase in average temperature (and higher levels of warming for non-linear response functions) in empirical papers would help with this and should be encouraged. In addition, as noted above, the number of points at which the continuous response function estimated in empirical papers should be sampled for inclusion in the database is inevitably arbitrary. Some standardization would be useful and would help with interpretation in the future.
Another finding from this paper is that there is little evidence in the existing literature that farm-level adaptations will substantially reduce the negative impacts of climate change on yields. The results presented here suggest that many actions described as adaptation in yield modeling studies would raise yields both in the current and in the future climate, meaning they do not necessarily reduce the negative impacts of future warming. If actions would confer benefit in the current climate but are not being adopted, economic logic suggests that models may be either over-estimating benefits or they may be missing important costs of implementation. In either case, the potential for within-crop, farm-level adaptations that improve yields in the future climate more than in the present climate appears limited, at least as currently represented within the studies included in the meta-analysis.
This paper confirms the importance of CO2 fertilization in determining the average global impacts of changing temperature over the 21st century. Our results show the question of whether or not CO2 effects are included is more important than either the inclusion of adaptation or the type of study used to estimate the temperature response. For both maize, wheat, and rice, CO2 fertilization fully offsets negative impacts of warming up to 1–2° for the global average yield effect. This demonstrates the importance of future work to better constrain the magnitude of this benefit. While we find good agreement between our results and those derived from FACE experiments, at least for the C3 crops (Long et al 2006), there is evidence that the fertilization effect depends critically on water and nutrient availability (McGrath and Lobell 2013, Zhu et al 2016, Reich et al 2014). Capturing this heterogeneity in CO2 fertilization by crop and farming intensity could be important in improving estimates of the yield impacts of climate change at both global and regional scales. Because of the importance of the CO2 fertilization effect, it should be clearly communicated when climate change impacts are presented without CO2 fertilization, which is often the case with statistical papers and sometimes with process-based models (for example, Nelson et al 2014b).
Finally, this paper makes the connection between models of crop productivity and economic welfare. This is an essential step for informing damage functions in the simple Integrated Assessment Models (IAMs) such as DICE, PAGE, and FUND used to calculate the SCC (NAS 2017). The economic impact results further underscore the importance of the CO2 fertilization effect: global welfare effects at 1–2 degrees of warming are negative without the CO2 fertilization effect but slightly positive for cases that include it. These results also show the complex connection between yield and welfare change. Despite error bounds on yield impacts being more or less symmetric, these same yield impacts give rise to highly asymmetric distributions over welfare changes, with substantial probability of large welfare losses. This asymmetry arises, despite the fact that the GTAP modeling framework allows for a large number of economic adaptations to moderate the adverse consequences of productivity shocks including changing inputs, shifting crop areas, trade adjustments, and consumption switching.
Acknowledgments
The authors acknowledge support for the underlying research into the climate-food-energy-land-water nexus from US Department of Energy, Office of Science, Office of Biological and Environmental Research, Integrated Assessment Research Program Grand No. DE-SC005171 as well as from USDA-NIFA project IND01053G2, Hatch project 1003642, and USDA-NIFA award 2017-67003-26058.
Footnotes
- 5
This count treats CERES-maize, CERES-rice and CERES-wheat as a single model family, for example.
- 6
Each observation in this regression is a combination of an estimated change in yield given certain changes in climate. A single study in the database may include multiple observations (for example, for different locations, different levels of temperature change, or with and without adaptation).
- 7
In other words, we calculate the effect of a change in temperature of X on yields to be the yield change predicted from equation (1) for a temperature change of X minus the yield change predicted for a temperature change of zero (i.e. β9Adaptijk).
- 8
Note that the meta-analysis is capturing only the representation of agronomic, within-crop adaptations. Other adaptations such as crop switching, movement of agricultural areas, trade adjustments, and consumption switching are dealt with in the GTAP modeling section. Additional discussion is provided in table S1.
- 9
Equation (1) also includes a linear control for rainfall (β7 term in equation 1). This shows that, on average, higher rainfall improves yields. A 1% increase in precipitation increases yields by 0.22% (std. error = 0.11, p < 0.05). This is consistent with the initial meta-analysis of Challinor et al (2014) who, with a different meta-analysis regression specification, found a 1% increase in rainfall increased yields by 0.53%. In the welfare analysis we do not further consider rainfall changes meaning our results should be interpreted as the marginal effect of only temperature (and CO2 changes in the relevant cases) on yields.