Abstract
The insulator is an important component of the high voltage transmission line. Inspecting the defects of the insulator plays an important role in ensuring the normal operation of the transmission line. The cracked insulator is the most common type of insulator defects. The traditional cracked insulator detection method is to use image denoising, single insulator disk segmentation, and the adjacent European distance to locate and identify cracked insulators. Experiments show that the robustness of these methods is poor and their ability to adapt to the environment is not good. Image denoising and image segmentation with the complex environment in images are very difficult tasks. Based on the extracted insulator strings, we change the problem to cracked insulators detection under complex conditions with deep learning. Region-based Fully Convolutional Networks (R-FCN), a deep learning algorithm, is adopted. Firstly, we apply image correction and cropping to images with different sample sizes and directions. Secondly, we use image enhancement technology to expand the dataset of samples. Finally, sliding window and coordinate mapping method are used when testing samples. The experimental results after the algorithm being applied to UVA dataset show an average accuracy rate of 90.5%. The inspection time of a single image is less than 1 second, and the model proposed in this paper has strong robustness and environmental adaptability.
Export citation and abstract BibTeX RIS
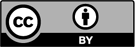
Content from this work may be used under the terms of the Creative Commons Attribution 3.0 licence. Any further distribution of this work must maintain attribution to the author(s) and the title of the work, journal citation and DOI.