Abstract
Background
Predicting total procedure time (TPT) entails several elements subject to variability, including the two main components: surgeon-controlled time (SCT) and anesthesia-controlled time (ACT). This study explores the effect of ACT on TPT as a proportion of TPT as opposed to a fixed number of minutes. The goal is to enhance the prediction of TPT and improve operating room scheduling.
Methods
Data from six university medical centres (UMCs) over seven consecutive years (2005-2011) were included, comprising 330,258 inpatient elective surgical cases. Based on the actual ACT and SCT, the revised prediction of TPT was determined as SCT × 1.33. Differences between actual and predicted total procedure times were calculated for the two methods of prediction.
Results
The predictability of TPT improved when the scheduling of procedures was based on predicting ACT as a proportion of SCT.
Conclusions
Efficient operating room (OR) management demands the accurate prediction of the times needed for all components of care, including SCT and ACT, for each surgical procedure. Supported by an extensive dataset from six UMCs, we advise grossing up the SCT by 33% to account for ACT (revised prediction of TPT = SCT × 1.33), rather than employing a methodology for predicting ACT based on a fixed number of minutes. This recommendation will improve OR scheduling, which could result in reducing overutilized OR time and the number of case cancellations and could lead to more efficient use of limited OR resources.
Résumé
Contexte
La prédiction du temps total de procédure (TTP) implique plusieurs éléments soumis à variabilité, notamment ses deux principaux composants: le temps contrôlé par le chirurgien (TCC) et le temps contrôlé par l’anesthésie (TCA). Cette étude explore l’effet du TCA sur le TTP en tant que pourcentage du TTP, par opposition à un nombre déterminé de minutes. L’objectif est d’améliorer la prédiction du TTP et d’améliorer la planification du programme opératoire.
Méthodes
Les données provenant de six centres médicaux universitaires (CMU) pendant sept années consécutives (2005-2011) ont été incluses, portant sur 330 258 patients hospitalisés qui y ont subi des interventions chirurgicales programmées. En s’appuyant sur les véritables TCA et TCC, la prédiction révisée du TTP a été déterminée par l’équation TCC × 1,33. Les différences entre le temps total réel de procédures et le temps total prédit ont été calculées avec les deux méthodes de prédiction.
Résultats
La prévisibilité du TTP a été améliorée quand la planification des procédures s’est basée sur le TCA en le considérant comme un pourcentage du TCC.
Conclusions
Une gestion efficace de la salle d’opération exige une prédiction précise du temps nécessaire à toutes les composantes des soins, y compris le TCC et le TCA de chaque procédure chirurgicale. Confortés par un abondant ensemble de données provenant de six CMU, nous préconisons d’ajuster le TCC par un facteur de 33 % pour prendre en compte le TCA (prédiction révisée du TTP = TCC × 1,33), plutôt que d’employer une méthodologie de prévision du TCA reposant sur un nombre fixe de minutes. Cette recommandation améliorera la planification de la salle d’opération, ce qui pourrait entraîner une diminution des temps de salles surutilisées et du nombre de cas d’annulations, et pourrait aboutir à une utilisation plus rationnelle de ressources limitées en salles d’opération.
Similar content being viewed by others
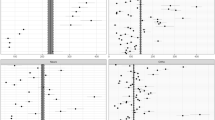
Operative surgical care is a costly multi-professional activity.1 Economic efficiency with regard to operative services is fundamentally tied to the efficient use of operative time, which in turn is tied to precision in scheduling surgical cases.
Among the hospitals participating in this study, approximately 60% of admitted patients received operative surgical care. Additionally, 40% of the revenues and expenses of the participating hospitals were related to operative surgical care, emphasizing the importance of economic and operational efficiency. Optimal scheduling of operating rooms (ORs) is one way to achieve effective and efficient use of their capacity.
Scheduling surgical procedures is a complex process. One difficulty associated with the development of accurate schedules for the OR is the uncertainty inherent in surgical procedures. Variability in the duration of surgery accounts for deviations between the predicted and the actual procedure time, which complicates surgical scheduling and reduces operational efficiency.2,3 When a procedure takes longer than predicted, procedures may be postponed or cancelled, and when the duration of surgery is shorter than predicted, valuable operating room time is wasted. Both of these situations are undesirable and contribute to suboptimal use of OR resources.4
Optimal scheduling depends on reliable predictions concerning the time needed for elective procedures. Total procedure time (TPT) is defined as the time from the patient’s entry into the OR until the patient’s departure from the OR. In this study, the term, “total procedure time”, characterizes one “session” and refers to one “surgical case”. Predicting TPT is challenging because it entails several elements subject to variability, such as room setup and takedown, patient positioning, prepping and draping, as well as the two principal components: surgeon-controlled time (SCT) and anesthesia-controlled time (ACT).
If TPT could be better predicted, this could lead to a reduction in overutilized OR time and fewer case cancellations.2,5,6 While progress has been made in the methodology of OR scheduling over the past years, opportunities still remain for improvement in this area of research. Previous studies have examined methods to predict TPT and have aimed to develop predictive tools using statistical modelling of procedure times.3,4,6-14 Most of these studies have focused on simulation of data, mathematical modelling, the selection of procedures from a few surgical departments, or consideration of a single procedure. Although the number of papers about modelling has grown substantially over recent years, few have reported on the outcomes of implementation of these models. Consequently, the value of implementing the suggested model remains unassessed. The validation of various models that have been identified remains to be published.15
There is a scarcity of multicentre studies employing an extensive empirical dataset of OR scheduling systems. In this paper, we present empirical data from six different university medical centres (UMCs) in the Netherlands over seven years. In these centres, the time allocated in an OR schedule for a particular session is the sum of ACT and SCT. The former has been considered to be a fixed number of minutes regardless of the surgical time. This study attempts to determine if this approach survives scrutiny or if an alternative approach to allocating ACT could better predict TPT to be allocated on a schedule. The goal is to enhance prediction of TPT and improve OR scheduling.
Methods
Operating room departments of all eight UMCs in the Netherlands established a nationwide benchmarking collaborative in 2005, which is still active.16,17 The objective of this collaborative is to improve OR performance by learning from each other through exchanging best practices. Each UMC provides data records for all surgical cases performed to a central OR benchmark database. This extensive database, presently comprising more than one million records of surgical cases, is used to calculate key performance indicators related to utilization of OR capacity and to perform research on OR scheduling issues. An independent data management centre enters the longitudinal data collection into the central OR benchmark database. This centre provides professional expertise to facilitate processing the data records and performing reliability checks before data are ready for analysis.
The central OR benchmark database with 940,381 cases in total consisted of records of all surgical cases performed at eight UMCs over a seven-year period from 2005 up to and including 2011. To define a consistent dataset for analysis, we excluded all non-elective (emergency) cases, surgical departments with an OR caseload of less than 100 per year, cases missing registration for a specific surgical department, and all outpatient cases. In Dutch UMCs, outpatient surgical cases are allocated to a specific organizational OR unit (a separate “day surgery centre”). The outpatient surgery workflow varies from the inpatient surgery workflow and has a different planning methodology. If the OR department of a UMC is divided into a main location and sub-locations, such as a children’s hospital, cancer centre, or thorax centre, the main (largest) inpatient OR location was included because these sub-locations have a different planning methodology.
During the session, OR nursing staff prospectively registered (electronically in the hospital information system in each UMC) the times for each case occupying the OR, and the surgeon and anesthesiologist in charge validated the times after completion of the session. Four time intervals were registered in the central OR benchmark database:
-
1.
total procedure time (predicted total procedure time as well as actual total procedure time),
-
2.
anesthesia induction time (actual time),
-
3.
surgeon-controlled time (actual time), and
-
4.
anesthesia emergence time (actual time).
Anesthesia-controlled time is the sum of 2 and 4. Total procedure time consists of 2, 3, and 4, in other words, ACT plus SCT. According to Dexter,18 anesthesia-controlled time is defined as “the sum of 1) the time starting when the patient enters the OR to the time when positioning or skin preparation can begin plus 2) the time starting when the surgical dressing is completed and ending when the patient leaves the OR,” in other words, ACT is the sum of 1) anesthesia induction time plus 2) anesthesia emergence time. Dexter also defined SCT18 as “the time starting when patient positioning and/or skin preparation can begin to when surgical dressing is completed” (see Fig. 1).
Total procedure time is subdivided into anesthesia induction time, surgeon-controlled time, and anesthesia emergence time. The sum of induction time and emergence time is anesthesia-controlled time. Predicting total procedure time entails several elements subject to variability, including the two main components: surgeon-controlled time and anesthesia-controlled time
In the current prediction method, the surgeon’s prediction of SCT (pSCT) is routinely determined before each procedure. For a prediction of ACT (pACT), a fixed time period of 20 min (general anesthesia) or 40 min (regional anesthetic technique) was added to (pSCT). This provided the predicted TPT (pTPT), which was used for OR scheduling. The actual TPT (aTPT), actual ACT (aACT), and actual SCT (aSCT) were registered in the database. The difference between predicted and actual TPT was assessed.
Data from six UMCs and seven consecutive years (2005-2011) were included. Two UMCs were excluded because these centres were not able to record the predicted TPT due to the non-availability of an adequate recording system. Considering purely inpatient and elective surgical cases, 330,258 cases from the six UMCs were subjected to statistical analysis. First, the status quo of the relationship between the independent variable, pTPT, and the dependent variable, aTPT, was assessed. Second, the proportion of TPT attributed to ACT was calculated, as well as the ratio SCT/ACT. Third, based on this ratio, a revised predicted ACT (rpACT) was computed, and TPT was revised based on this rpACT plus the surgeon’s prediction of SCT. Finally, the difference between revised predicted TPT (rpTPT) and actual TPT was assessed. Table 1 illustrates the calculations that were performed.
Statistical analysis
Data analysis was performed using SPSS® Statistics 19 (IBM SPSS Statistics for Windows, version 19.0, IBM Corp. Released 2010.; Armonk, NY, USA). Ordinary least-squares regression analysis was used to determine the regression line of aTPT on pTPT as well as the regression line of aTPT on rpTPT. Scatter plots of actual vs predicted TPT and prediction error vs predicted TPT were constructed. Finally, stacked histograms of the prediction errors (current prediction method and revised prediction strategy) were assembled.
Results
There were 330,258 inpatient elective surgical cases (2005-2011) selected for inclusion in the study. The six centres showed a mean (SD) aTPT of 158 (119) min and a median of 124 min for all inpatient elective surgical cases (Table 2). The mean (SD) aSCT was 121 (106) min and the median aSCT was 90 min. The mean (SD) aACT was 37 (22) min and the median aACT was 31 min.
Figure 2A shows a scatter plot depicting the aTPT and pTPT (current prediction) in minutes of all observations at all six UMCs. The estimated regression line of the current prediction method is: aTPT = 10.94 + (1.06*pTPT). For example, if TPT were predicted to take 140 min, the duration of aTPT would be 159 min. TPT was underestimated by 19 min.
(A) Current prediction method (fixed ACT): scatter plot of actual vs predicted total procedure time in minutes. ACT = anesthesia-controlled time. (B) Current prediction method (fixed ACT): scatter plot of prediction error (actual − predicted total procedure time [TPT]) vs predicted total procedure time in minutes. ACT = anesthesia-controlled time
The analysis of the empirical data from six UMCs (n = 330,258) showed a mean (SD) ratio aSCT/aACT of 3.61 (2.91) and a median of 2.90. These results indicate that aSCT was approximately three times greater than aACT. The mean of 3.61 was rounded down to 3 to correct for the influence of data outliers because the variables used in this study were not normally distributed. Hence, on an overall level: (aACT/aTPT) × 100 = 25% and (aACT/aSCT) × 100 = 33%. Based on these results, rpACT and rpTPT were computed as follows:
The conducted calculations are illustrated in Table 1.
Figure 3A shows a scatter plot depicting the aTPT and rpTPT in minutes of all observations at all six UMCs. The estimated regression line of this revised prediction strategy is: aTPT = 23.3 + (0.83*rpTPT). For example, if TPT were predicted to take 140 min, the duration of aTPT would be 139.5 min. TPT was overestimated by 0.5 min.
(A) Revised prediction strategy (relative ACT): scatter plot of actual vs revised predicted total procedure time in minutes. ACT = anesthesia-controlled time. (B) Revised prediction strategy (relative ACT): scatter plot of prediction error (actual − revised predicted total procedure time [TPT]) vs revised predicted TPT in minutes. ACT = anesthesia-controlled time
Figures 2B and 3B both illustrate the prediction errors and predicted values in minutes. These plots show that the prediction errors increased rapidly in the current situation and varied with a wider range than in the revised prediction strategy. This is also emphasized by Fig. 4 showing stacked histograms of the prediction errors for both prediction methods. In the current situation, prediction errors ranged from −688 to +488 min, and in the revised strategy, prediction errors ranged from −219 to +231 min. Figure 4 simply shows the prediction errors for both methods on the scale from −300 to +300 min.
Table 3 shows the mean (SD) ratio aSCT/aACT and median ratio aSCT/aACT differentiated per surgical department using the data of all six UMCs. Cardiothoracic Surgery and Neurosurgery, both characterized by significantly longer aSCT than other surgical departments, showed an aSCT/aACT ratio of 4. Orthopedic Surgery and Plastic Surgery also showed a ratio of four, indicating that the recommended scheduling rule, rpTPT = pSCT × 1.33, is preferably differentiated per surgical department, e.g., adjusted to rpTPT = pSCT × 1.25 for Cardiothoracic-, Neuro-, Orthopedic, and Plastic Surgery.
Discussion
This Dutch nationwide multicentre study in a university hospital environment shows that grossing up SCT by 33% to account for rpACT could improve the prediction of TPT if this methodology were adopted. This study confirms that employing a fixed time period for ACT (e.g., 20 min) is unsuitable because, like SCT, ACT is subject to variability. The results affirm that ACT is a considerable component of TPT, which should be scheduled just as realistically as SCT. Robust OR schedules need to anticipate SCT as well as ACT. Instead of predicting one time period for TPT, anesthesia-controlled time should be predicted apart from SCT as a separate time period within TPT.
In a previous study in 1998 from the Medical University of South Carolina, a 550-bed teaching hospital and tertiary referral centre (n = 1,881 cases), Overdyk et al. found that “surgeon-controlled time is approximately four and a half times greater than anesthesia-controlled time”.19 These authors suggested grossing up SCT by 22% to account for ACT.19
Our study, which is based on an extensive database collected in a considerable research setting of six centres, shows that this proportion should be higher and at least 33% (rpTPT = pSCT × 1.33). More accurate prediction rules may lead to reducing the amount of overutilized OR time and the number of case cancellations.2,5
Potential reasons for the difference between Overdyk’s study results19 and these recent results are the size of the hospitals and the number of cases investigated. All UMCs have a bed capacity that varies from 715 to 1,339 beds, and our study investigated 330,258 cases. Both figures are considerably larger than previous research.
Figures 2A, 2B, and 4 show that the current prediction method frequently underestimated aTPT, leading to the risk of OR days running late and case cancellations. Figures 3A, 3B, and 4 show that employing our recommended scheduling rule results in lower prediction errors and especially less underestimation of aTPT. Moreover, the increasing size of prediction errors with increasing predicted values suggests that using a proportion of time for ACT would be preferable to using the current methodology based on a fixed number of minutes.
In many hospitals, surgeons make a routine prediction of the procedure time needed, though it has been shown that surgeons tend to underestimate the time needed to perform a procedure.20-22 In some facilities, historical procedure times are taken as a reference to predict the duration of future cases. This methodology has been recommended by earlier studies.14,23-27 Some centres employ an approximation of pACT in the pTPT,10 often as a fixed number of minutes. All types of anesthesia, including general, regional, local, combined general with epidural, and monitored anesthesia care, were included in the study. The participating UMCs do not use anesthetic induction rooms distinct from the operating room. In these Dutch centres, all activities related to anesthesia care are provided in the OR and therefore influence OR utilization. In one centre, however, the epidural catheter is occasionally inserted in the preoperative holding area, but this is not always possible due to the large number of patients. Referring to the use of a fixed number of minutes for pACT, Escobar et al.28 found significant variation in anesthesia release time and concluded that assigning a constant fixed time for anesthetic induction is inappropriate for OR scheduling purposes. This recent study produced results that corroborate that conclusion.
There are, however, valid limitations to this study. Although the revised prediction strategy has decreased the size of prediction errors (see Fig. 4), it is obvious, from Fig. 3A, that there is still substantial variability in the actual total procedure time for any given predicted total procedure time. Scheduling surgical procedures will remain a multifactorial and therefore complex process.
Since data were gathered in tertiary referral centres only, general applicability of the findings may be restricted. The mean (SD) aTPT of 158 (119) min and the median of 124 min in the present study reflect that the complexity of procedures is potentially greater than in other facilities. The application of this scheduling rule in non-academic facilities has yet to be studied.
Another issue with this and past studies related to OR scheduling and OR efficiency lies in the way data are collected.19 In the Netherlands, the OR departments of all eight UMCs established a benchmarking collaborative in 2004, continuing to this day. Each UMC submits the data records of all surgical cases performed to a central OR benchmark database. All data are prospectively electronically entered in real-time by the OR nursing staff into a hospital information system per UMC and subsequently confirmed by the surgeon and anesthesiologist in charge. The individual databases of each of the eight UMCs were originally intended for administrative and managerial purposes. We acknowledge the potential virtually unavoidable biases stemming from this data collection source (administrative/nursing database) and agree with Overdyk’s19 remark that it might even be impossible to exclude bias when data collection depends on human individuals instead of automatic electronic time recording systems.
The estimated magnitude of this “human bias” in our longitudinal study is considered to have a small impact because of the long-term stable nature of data capture. It involves repeated and continuous measurement of the same parameters over a long period of time. In this respect, we have assessed the OR benchmark data and found that parameters over all these years (2005-2011) show a consistent picture over the years, either a gradual increase or a gradual decrease. Furthermore, the differences between the UMCs also show a consistent picture, which does not indicate that human bias is of imperative importance.
Scheduling surgical procedures is complex because a procedure entails several elements subject to variability, including room setup and takedown, patient positioning, prepping and draping, as well as the two principal components, SCT and ACT. More factors of variability, such as OR team member characteristics and their experience (attending, fellow, resident, trainee, and experience in years), can influence aTPT. Even for experienced anesthesiologists, it is often difficult to predict how long the anesthetic induction for a specific surgical case will take.9 Factors such as American Society of Anesthesiologists’ physical status, age, anesthetic technique (e.g., monitors, lines, and pain management procedures), working with trainees and residents in a teaching setting, and surgical procedure have shown to affect aACT and aTPT significantly.3,9,10,29 Since the central OR benchmark database was not designed to register all of these variability factors, this study could not investigate their impact on aTPT. In our view, however, these factors are most likely equally distributed among the different UMCs and therefore are of limited impact on our conclusions.
Future studies that take additional variability factors into account are needed. On an individual hospital level, these factors are partially available. It would also be interesting to compare aSCT among surgeons regarding the same procedure as well as aACT among anesthesiologists regarding the same anesthetic technique. Using historical times per surgeon and per procedure to schedule pSCT is not new,14,23-27 but using historical times per anesthesiologist is not common in the Netherlands. Recently, one Dutch UMC adopted a system of scheduling pACT based on historical times per anesthesiologist and per anesthetic technique. The implementation of this process started at the end of 2012, and further research is needed to assess the value and effects of this methodology in practice.
If readers consider implementing the suggested scheduling rule (rpTPT = SCT × 1.33), it is recommended to calculate the relevant proportion aSCT/aACT using their own specific historical procedure data. Additionally, it is recommended to refine the scheduling rule per surgical department, which is indicated by the differentiation of the ratio in the results section (Table 2).
Efficient OR management demands the accurate prediction of the times needed for all components of care (including the two main elements SCT and ACT) for each surgical procedure.3,10,14,30 Supported by an extensive dataset from six UMCs, we advise grossing up the pSCT by 33% to account for pACT (rpTPT = pSCT × 1.33), rather than employing a methodology based on a fixed number of minutes. Thirty-three percent is a higher proportion than reported in earlier research.19 This recommendation will improve OR scheduling, which could result in reducing the amount of overutilized OR time and the number of case cancellations and therefore lead to more efficient use of limited OR resources.2,5,6
References
Marjamaa R, Vakkuri A, Kirvela O. Operating room management: why, how and by whom? Acta Anaesthesiol Scand 2008; 52: 596-600.
Cardoen B, Demeulemeester E, Belien J. Operating room planning and scheduling: a literature review. European Journal of Operational Research 2010; 201: 921-32.
Strum DP, Sampson AR, May JH, Vargas LG. Surgeon and type of anesthesia predict variability in surgical procedure times. Anesthesiology 2000; 92: 1454-66.
Eijkemans MJ, van Houdenhoven M, Nguyen T, Boersma E, Steyerberg EW, Kazemier G. Predicting the unpredictable: a new prediction model for operating room times using individual characteristics and the surgeon’s estimate. Anesthesiology 2010; 112: 41-9.
Tyler DC, Pasquariello CA, Chen CH. Determining optimum operating room utilization. Anesth Analg 2003; 96: 1114-21.
Lehtonen JM, Torkki P, Peltokorpi A, Moilanen T. Increasing operating room productivity by duration categories and a newsvendor model. Int J Health Care Qual Assur 2013; 26: 80-92.
Agnoletti V, Buccioli M, Padovani E, et al. Operating room data management: improving efficiency and safety in a surgical block. BMC Surg 2013; 13: 7.
Devi SP, Rao KS, Sangeetha SS. Prediction of surgery times and scheduling of operation theaters in ophthalmology department. J Med Syst 2012; 36: 415-30.
Dexter F, Dexter EU, Masursky D, Nussmeier NA. Systematic review of general thoracic surgery articles to identify predictors of operating room case durations. Anesth Analg 2008; 106: 1232-41.
Ehrenwerth J, Escobar A, Davis EA, et al. Can the attending anesthesiologist accurately predict the duration of anesthesia induction? Anesth Analg 2006; 103: 938-40.
Gillespie BM, Chaboyer W, Fairweather N. Factors that influence the expected length of operation: results of a prospective study. BMJ Qual Saf 2012; 21: 3-12.
Pandit JJ, Tavare A. Using mean duration and variation of procedure times to plan a list of surgical operations to fit into the scheduled list time. Eur J Anaesthesiol 2011; 28: 493-501.
Smith CD, Spackman T, Brommer K, et al. Re-engineering the operating room using variability methodology to improve health care value. J Am Coll Surg 2013; 216: 559-68.
Wright IH, Kooperberg C, Bonar BA, Bashein G. Statistical modeling to predict elective surgery time. Comparison with a computer scheduling system and surgeon-provided estimates. Anesthesiology 1996; 85: 1235-45.
Fone D, Hollinghurst S, Temple M, et al. Systematic review of the use and value of computer simulation modelling in population health and health care delivery. J Public Health Med 2003; 25: 325-35.
Kazemier G, van Veen-Berkx E. Comment on “Identification and use of operating room efficiency indicators: the problem of definition”. Can J Surg 2013; 56: E103-4.
Van Veen-Berkx E, Elkhuizen SG, Kalkman CJ, Buhre WF, Kazemier G. Successful interventions to reduce first-case tardiness in Dutch university medical centers. Results of a nationwide operating room benchmark study. Am J Surg 2014. DOI:10.1016/j.amjsurg.2013.09.025.
Dexter F, Coffin S, Tinker JH. Decreases in anesthesia-controlled time cannot permit one additional surgical operation to be reliably scheduled during the workday. Anesth Analg 1995; 81: 1263-8.
Overdyk FJ, Harvey SC, Fishman RL, Shippey F. Successful strategies for improving operating room efficiency at academic institutions. Anesth Analg 1998; 86: 896-906.
Alvarez R, Bowry R, Carter M. Prediction of the time to complete a series of surgical cases to avoid cardiac operating room overutilization. Can J Anesth 2010; 57: 973-9.
Dexter F, Dexter EU, Ledolter J. Influence of procedure classification on process variability and parameter uncertainty of surgical case durations. Anesth Analg 2010; 110: 1155-63.
Dexter F, Wachtel RE, Epstein RH, McIntosh C, O’Neill L. Allocative efficiency vs technical efficiency in operating room management. Anaesthesia 2007; 62: 1290-1; author reply 1-2.
Pandit JJ, Carey A. Estimating the duration of common elective operations: implications for operating list management. Anaesthesia 2006; 61: 768-76.
Zhou J, Dexter F, Macario A, Lubarsky DA. Relying solely on historical surgical times to estimate accurately future surgical times is unlikely to reduce the average length of time cases finish late. J Clin Anesth 1999; 11: 601-5.
Dexter F, Ledolter J, Tiwari V, Epstein RH. Value of a scheduled duration quantified in terms of equivalent numbers of historical cases. Anesth Analg 2013; 117: 205-10.
Dexter F, Ledolter J. Bayesian prediction bounds and comparisons of operating room times even for procedures with few or no historic data. Anesthesiology 2005; 103: 1259-67.
Dexter F, Traub RD, Fleisher LA, Rock P. What sample sizes are required for pooling surgical case durations among facilities to decrease the incidence of procedures with little historical data? Anesthesiology 2002; 96: 1230-6.
Escobar A, Davis EA, Ehrenwerth J, et al. Task analysis of the preincision surgical period: an independent observer-based study of 1558 cases. Anesth Analg 2006; 103: 922-7.
Urman RD, Sarin P, Mitani A, Philip B, Eappen S. Presence of anesthesia resident trainees in day surgery unit has mixed effects on operating room efficiency measures. Ochsner J 2012; 12: 25-9.
Dexter F, Macario A. Applications of information systems to operating room scheduling. Anesthesiology 1996; 85: 1232-4.
Acknowledgments
The authors sincerely thank all members of the steering and project committee of the Dutch Operating Room Benchmarking Collaborative for their participation and contribution.
Current Members of the Dutch Operating Room Benchmarking Collaborative (the Netherlands)
Academic Medical Centre Amsterdam: Ron Balm, MD, PhD, Department of Vascular Surgery; Diederich C.C. Cornelisse, MSc, Department of Operating Rooms;
Maastricht University Medical Centre: Wolfgang F. Buhre, MD, PhD, Department of Anesthesiology and Pain Medicine; Hub J. Ackermans, Department of Operating Rooms;
Erasmus University Medical Centre Rotterdam: Sam L.W. Schoch, LLM, MSc, Division of Emergency, Perioperative and Intensive Care; Jeanne Bezstarosti, MD, Department of Anesthesiology and Department of Operating Rooms;
Leiden University Medical Centre: Rob C.M. Pelger, MD, PhD, Department of Urology; Roald R. Schaad, MSc, Department of Anesthesiology and Department of Operating Rooms;
University Medical Centre Groningen: Irmgard Krooneman-Smits, MBA, Department of Operating Rooms; Peter Meyer, MD, PhD, Department of Anesthesiology and Department of Operating Rooms;
Radboud University Medical Centre Nijmegen: Hein G. Gooszen, MD, PhD, Department of Operating Rooms; Mirjam van Dijk-Jager, Department of Operating Rooms; Xandra Verhoeven-Buijs, Department of Operating Rooms;
University Medical Center Utrecht: Jeffrey Kanters, Department of Operating Rooms; A. Christiaan Kroese, MSc, Department of Anesthesiology and Department of Operating Rooms;
University of Twente: Johannes J. Krabbendam, PhD; Erwin W. Hans, PhD, Department of Operational Methods for Production and Logistics;
VU University Medical Center Amsterdam: Marinus F. Caljouw, Division of Anesthesiology, Intensive Care and Emergency Medicine; Derk P. Veerman, MD, PhD, Division of Anesthesiology, Intensive Care and Emergency Medicine; Kjeld H. Aij, MBA, Division of Anesthesiology, Intensive Care and Emergency Medicine.
The authors also thank Ewout W. Steyerberg, PhD, Professor of Medical Decision Making, Department of Public Health, Erasmus University Medical Center Rotterdam, for his statistical advice.
Conflicts of interest
None declared.
Author information
Authors and Affiliations
Corresponding author
Additional information
Author contributions
Elizabeth van Veen-Berkx had full access to all of the data in the study and takes responsibility for the integrity of the data and the accuracy of the data analysis. Study concept and design: Elizabeth van Veen-Berkx, Geert Kazemier. Acquisition of data: Elizabeth van Veen-Berkx, Geert Kazemier, participants Dutch Operating Room Benchmarking Collaborative. Analysis and interpretation of data: Elizabeth van Veen-Berkx, Geert Kazemier, Sylvia G. Elkhuizen. Drafting of the manuscripts: Elizabeth van Veen-Berkx, Geert Kazemier, Justin Bitter. Critical revision of the manuscript for important intellectual content: Geert Kazemier, Wolfgang F. Buhre, Hein G. Gooszen, Cor J. Kalkman. Statistical analysis: Elizabeth van Veen-Berkx, Sylvia G. Elkhuizen. Administrative, technical, or material support: participants Dutch Operating Room Benchmarking Collaborative. Study supervision: Geert Kazemier, Wolfgang F. Buhre, Hein G. Gooszen, Cor J. Kalkman, Elizabeth van Veen-Berkx.
Rights and permissions
About this article
Cite this article
van Veen-Berkx, E., Bitter, J., Elkhuizen, S.G. et al. The influence of anesthesia-controlled time on operating room scheduling in Dutch university medical centres. Can J Anesth/J Can Anesth 61, 524–532 (2014). https://doi.org/10.1007/s12630-014-0134-9
Received:
Accepted:
Published:
Issue Date:
DOI: https://doi.org/10.1007/s12630-014-0134-9