Abstract
The satellite-derived wind from cloud and moisture features of geostationary satellites is an important data source for numerical weather prediction (NWP) models. These datasets and global positioning system radio occultation (GPSRO) satellite radiances are assimilated in the four-dimensional variational atmospheric data assimilation system of the UKMO Unified Model in India. This study focuses on the importance of these data in the NWP system and their impact on short-term 24-h forecasts. The quality of the wind observations is compared to the short-range forecast from the model background. The observation increments (observation minus background) are computed as the satellite-derived wind minus the model forecast with a 6-h lead time. The results show the model background has a large easterly wind component compared to satellite observations. The importance of each observation in the analysis is studied using an adjoint-based forecast sensitivity to observation method. The results show that at least around 50% of all types of satellite observations are beneficial. In terms of individual contribution, METEOSAT-7 shows a higher percentage of impact (nearly 50%), as compared to GEOS, MTSAT-2 and METEOSAT-10, all of which have a less than 25% impact. In addition, the impact of GPSRO, infrared atmospheric sounding interferometer (IASI) and atmospheric infrared sounder (AIRS) data is calculated. The GPSRO observations have beneficial impacts up to 50 km. Over the Southern Hemisphere, the high spectral radiances from IASI and AIRS show a greater impact than over the Northern Hemisphere. The results in this study can be used for further improvements in the use of new and existing satellite observations.
Similar content being viewed by others
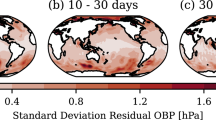
References
Aumann, H. H., and Coauthors, 2003: AIRS/AMSU/HSB on the AQUA mission: Design, science objectives, data products, and processing systems. IEEE Transactions on Geoscience and Remote Sensing, 41, 253–264.
Bonavita, M., 2014: On some aspects of the impact of GPSRO observations in global numerical weather prediction. Quart. J. Roy. Meteor. Soc., 140, 2546–2562, doi: 10.1002/qj.320.
Cardinali, C., 2009a: Forecast sensitivity to observation (FSO) as a diagnostic tool. ECMWF Tech. Memo. 599. ECMWF: Reading. [Available online at http://www.ecmwf.int/en/elibrary/8574-forecast-sensitivity-observation-fso-diagnostic-tool.]
Cardinali, C., 2009b: Monitoring the observation impact on the short-range forecast. Quart. J. Roy. Meteor. Soc., 135, 239–250, doi: 10.1002/qj.366.
Cardinali, C., and F. Prates, 2011: Performance measurement with advanced diagnostic tools of all-sky microwave imager radiances in 4D-Var. Quart. J. Roy. Meteor. Soc., 137, 2038–2046, doi: 10.1002/qj.865.
Collard, A. D., 2004: Assimilation of AIRS Observations at the Met Office. Proceedings of the ECMWF Workshop on Assimilation of High Spectral Resolution Sounders in NWP, 63–71. [Available online at http://www.ecmwf.int/sites/default/files/elibrary/2004/8754-assimilation-airs-observations-met-office.pdf.]
Collard, A. D., 2007: Selection of IASI channels for use in numerical weather prediction. Quart. J. Roy. Meteor. Soc., 133, 1977–1991.
Collard, A. D., and S. B. Healy, 2003: The combined impact of future space-based atmospheric sounding instruments on numerical weather-prediction analysis fields: A simulation study. Quart. J. Roy. Meteor. Soc., 129, 2741–2760.
Cotton, J., 2014: ldNWP SAF AMV monitoring: the 6th Analysis Report (AR6)” No NWPSAF-MO-TR-029 [Available online at https://nwpsaf.eu/monitoring/amv/nwpsaf_motr_029.pdf.]
Courtier, P., J. N. Thépaut, and A. Hollingsworth, 1994: A strategy for operational implementation of 4D-var, using an incremental approach. Quart. J. Roy. Meteor. Soc., 120, 1367–1387.
Dee, D. P., and S. Uppala, 2009: Variational Bias correction of satellite radiance data in the ERA-Interim reanalysis. Quart. J. Roy. Meteor. Soc., 135, 1830–1841.
Eyre, J. R., 2008: An introduction to GPS radio occultation and its use in numerical weather prediction. Proceedings of the ECMWF GRAS SAF Workshop on Applications of GPS Radio Occultation Measurements, 16–18 June, 1–10. [Available online at http://www.ecmwf.int/sites/default/files/elibrary/2008/9342-introduction-gps-radio-occultation-and-its-use-numerical-weather-prediction.pdf.]
Gelaro, R., R. H. Langland, S. Pellerin, and R. Todling, 2010: The THORPEX observation impact intercomparison experiment. Mon. Wea. Rev., 138, 4009–4025, doi: 10.1175/2010MWR3393.1.
George, J. P., and Coauthors, 2016: NCUM Data Assimilation System. NMRF/TR/01/2016, 21pp. [Available online at http://www.ncmrwf.gov.in/NCUM-Data%20Assimilation.pdf.]
Hayashi, M., and K. Shimoji, 2012: Recent status and development of Atmospheric Motion Vector at JMA. The Proceedings Eleventh International Winds Workshop, Auckland, New Zealand [Available online at http://www.eumetsat.int/website/home/News/ConferencesandEvents/DAT_2039311.html?lang=EN.]
Ingleby, N. B., and A. C. Lorenc, 1993: Bayesian quality control using multivariate normal distributions. Quart. J. Roy. Meteor. Soc., 119, 1195–1225.
Isaksen, L., M. Fisher, E. Andersson, and J. Barkmeijer, 2005: The structure and realism of sensitivity perturbations and their interpretation as “Key Analysis”. Quart. J. Roy. Meteor. Soc., 131, 3053–3078.
Johnson, C., B. J. Hoskins, N. K. Nichols, and S. P. Ballard, 2006: A singular vector perspective of 4DVAR: The spatial structure and evolution of baroclinic weather systems. Mon. Wea. Rev., 134, 3436–3455, doi: 10.1175/MWR3243.1.
Joo, S., A. Lorenc, and R. Marriott, 2012: Diagnosis of exaggerated impacts in adjoint-based sensitivity studies. Forecasting Research Tech. Rep. 564, Met Office, 20pp. [Available online at http://www.metoffice.gov.uk/media/pdf/s/m/FRTR564.pdf.]
Joo, S., J. Eyre, and R. Marriott, 2013: The impact of MetOp and other satellite data within the Met Office global NWP system using an adjoint-based sensitivity method. Mon. Wea. Rev., 141, 3331–3342, doi: 10.1175/MWR-D-12-00232.1.
Kursinski, E. R., G. A. Hajj, J. T. Schofield, R. P. Linfield, and K. R. Hardy, 1997: Observing Earth’s atmosphere with radio occultation measurements using the Global Positioning System. J. Geophys. Res., 102, 23429–23465, doi: 10.1029/97JD01569.
Le Marshall, J., and Coauthors, 2005: Airs hyperspectral data improves Southern Hemisphere forecasts. Aust. Meteor. Mag., 54, 57–60.
Lorenc, A. C., 2003: Modelling of error covariances by 4D-Var data assimilation. Quart. J. Roy. Meteor. Soc., 129, 3167–3182.
Lorenc, A. C., and O. Hammon, 1988: Objective quality control of observations using Bayesian methods. Theory, and a practical implementation. Quart. J. Roy. Meteor. Soc., 114, 515–543.
Lorenc, A. C., and R. T. Marriott, 2014: Forecast sensitivity to observations in the Met Office Global numerical weather prediction system. Quart. J. Roy. Meteor. Soc., 140, 209–224, doi: 10.1002/qj.2122.
Lupu, C., C. Cardinali, and A. P. McNally, 2015: Adjoint-based forecast sensitivity applied to observation-error variance tuning. Quart. J. Roy. Meteor. Soc., 141, 3157–3165, doi: 10.1002/qj.2599.
Mallick, S., S. Indira Rani, D. Srinivas, and J. P. George, 2016: Assimilation of CrIS hyperspectral radiances in a 4D-VAR assimilation system. Proc. SPIE 9880, Multispectral, Hyperspectral, and Ultraspectral Remote Sensing Technology, Techniques and Applications VI, 98800Q, New Delhi, India, doi: 10.1117/12.2222775.
Marriott, R., et al., 2012: Adjoint-based forecast sensitivity to observation (FSO) and observation impacts. VAR Scientific Documentation Papers 63, Met-Office, UK.
Nerry, F., J. Labed, and M. P. Stoll, 1988: Emissivity signatures in the thermal IR band for remote sensing: calibration procedure and method of measurement. Appl. Opt., 27, 758–764.
Polkinghorne, R., and T. Vukicevic, 2011: Data assimilation of cloud-affected radiances in a cloud-resolving model. Mon. Wea. Rev., 139, 755–773, doi: 10.1175/2010MWR3360.1.
Prasad, V. S., 2014: Satellite Data Processing for NCMRWF Unified Model (NCUM), NMRF/RR/02/2014, 17pp. [Available online at http://www.ncmrwf.gov.in/radiance_obstore_ver2.pdf.]
Prasad, V. S., and S. Indira Rani, 2014: Data Pre-Processing for NCMRWF Unified Model (NCUM): Version 2. [Available online at http://www.ncmrwf.gov.in/ncum_obstore_v2.pdf.]
Prunet, P., J.-N. Thépaut, and V. Cassé, 1998: The information content of clear sky IASI radiances and their potential for numerical weather prediction. Quart. J. Roy. Meteor. Soc., 124, 211–241, doi: 10.1002/qj.49712454510.
Rajagopal, E. N., and Coauthors, 2012: Implementation of Unified Model based Analysis-Forecast System at NCMRWF. Internal Report, NMRF/TR/2/2012, National Centre for Medium Range Weather Forecasting, Ministry of Earth Sciences, India, 1–45. [Available online at http://www.ncmrwf.gov.in/UM_OPS_VAR_Report.pdf.]
Rawlins, F., S. P. Ballard, K. J. Bovis, A. M. Clayton, D. Li, G. W. Inverarity, A. C. Lorenc, and T. J. Payne, 2007: The Met Office global four-dimensional variational data assimilation scheme. Quart. J. Roy. Meteor. Soc., 133, 347–362, doi: 10.1002/qj.32.
Salisbury, J. W., and D. M. D’Aria, 1992: Infrared (8–14 μm) remote sensing of soil particle size. Remote Sens. Environ., 42, 157–165.
Saunders, R., 2001: Assimilation of IASI and AIRS data: Forward modelling. Proceedings of the ECMWF Seminar on Exploitation of the New Generation of Satellite Instruments for NumericalWeather Prediction, ECMWF, Shineld Park, Reading, Berkshire RG2 9AX, UK, 181–200. [Available online at: http://www.ecmwf.int/sites/default/files/elibrary/2000/12103-assimilation-iasi-and-airs-data-forward-modelling.pdf.]
Saunders, R., M. Matricardi, and A. Geer, 2010: RTTOV-9 user guide: Science and validation report. EUMETSAT, 31 pp. [Available online at http://nwpsaf.eu/oldsite/deliverables/rtm/rttov9_files/users_guide_9_v1.7.pdf.]
Seemann, S. W., E. E. Borbas, R. O. Knuteson, G. R. Stephenson, and H. L. Huang, 2008: Development of a global infrared land surface emissivity database for application to clear sky sounding retrievals from multispectral satellite radiance measurements. J. Appl. Meteor. Climatol., 47, 108–123.
Sharma, P., S. Indira Rani, S. Mallick, D. Srinivas, J. P. George, and M. Gupta, 2016: IASI hyperspectral radiances in the NCMRWF 4D-VAR assimilation system: OSE. Proc. SPIE 9880, Multispectral, Hyperspectral, and Ultraspectral Remote Sensing Technology, Techniques and Applications VI, 98800P, New Delhi, India, doi: 10.1117/12.2225868.
Sherlock, V., 1999: ISEM-6: Infrared Surface Emissivity Model for RTTOV-6. NWP SAF report. [Available online at http://www.metoffice.gov.uk/research/interproj/nwpsaf/rtm/papers/isem6.pdf.]
Snyder, W. C., Z. Wan, Y. Zhang, and Y.-Z. Feng, 1998: Classification-based emissivity for land surface temperature measurement from space. Int. J. Remote Sens., 19, 2753–2774.
Srinivas, D., S. Indira Rani, S. Mallick, J. P. George, and P. Sharma, 2016: Impact of AIRS radiance in the NCUM 4DVAR assimilation system. Proc. SPIE 9880, Multispectral, Hyperspectral, and Ultraspectral Remote Sensing Technology, Techniques and Applications VI, 98800O, New Delhi, India, doi: 10.1117/12.2223516.
Stiller, O., and S. P. Ballard, 2009: Efficient moist physics schemes for data assimilation. I: Large-scale clouds and condensation. Quart. J. Roy. Meteor. Soc., 135, 707–720.
Talagrand, O., and P. Courtier, 1987: Variational assimilation of meteorological observations with the adjoint vorticity equation. I: Theory. Quart. J. Roy. Meteor. Soc., 113, 1311–1328.
Vincensini, A., N. Fourrié, F. Rabier, and V. Guidard, 2012: Data assimilation of IASI radiances over land. Proc. the 18th International Study TOVS Conference, Toulouse, France. [Available online at: https://cimss.ssec.wisc.edu/itwg/itsc/itsc18/program/files/links/4.16_Vincensini_pa.pdf.]
Wilks, D. S., 2006: Statistical Methods in the Atmospheric Sciences. 2nd Ed., Academic Press, 35 pp.
Zhu, Y. Q., and R. Gelaro, 2008: Observation sensitivity calculations using the adjoint of the Gridpoint Statistical Interpolation (GSI) analysis system. Mon. Wea. Rev., 136, 335–351.
Acknowledgements
The first author acknowledges the advice and help received from the National Centre for Medium Range Weather Forecasting (NCMRWF) scientists while carrying out the initial work at NCMRWF, India. The authors also acknowledge Dr. Peter M. JERMEY from the UKMO and Dr. John P. GEORGE from NCMRWF for helpful discussion. This study was supported by the Korea Meteorological Administration Research and Development Program (Grant No. KMIPA 2015-1090). We appreciate the constructive comments from the two anonymous reviewers and the editor.
Author information
Authors and Affiliations
Corresponding author
Rights and permissions
About this article
Cite this article
Mallick, S., Dutta, D. & Min, KH. Quality assessment and forecast sensitivity of global remote sensing observations. Adv. Atmos. Sci. 34, 371–382 (2017). https://doi.org/10.1007/s00376-016-6109-8
Received:
Revised:
Accepted:
Published:
Issue Date:
DOI: https://doi.org/10.1007/s00376-016-6109-8